Peer Reviewed
Did the Musk takeover boost contentious actors on Twitter?
Article Metrics
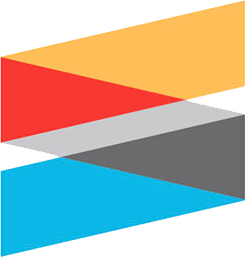
1
CrossRef Citations
Altmetric Score
PDF Downloads
Page Views
After his acquisition of Twitter, Elon Musk pledged to overhaul verification and moderation policies. These events sparked fears of a rise in influence of contentious actors—notably from the political right. I investigated whether these actors did receive increased engagement over this period by gathering tweet data for accounts that purchased blue-tick verification before and after the Musk takeover. Following the takeover, there was a large increase in post engagement for all users, but tweet engagement for accounts active in far-right networks outstripped any increased engagement for general user accounts. There is no obvious evidence that blue-tick verification conferred an additional engagement boost.
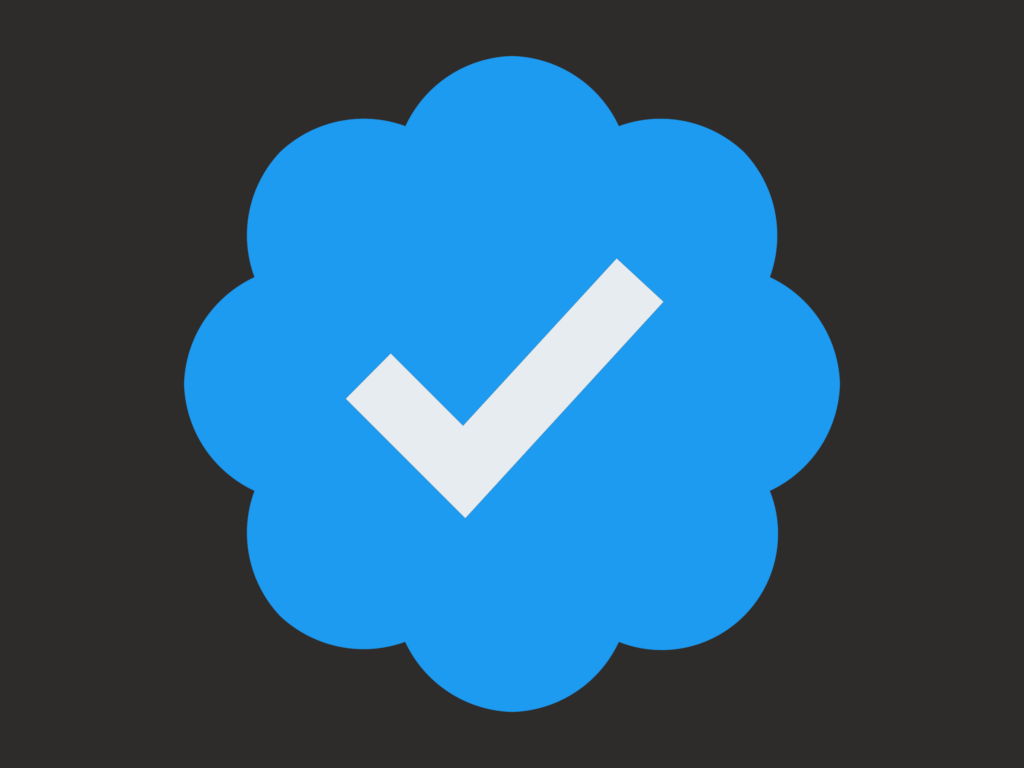
Research Questions
- Did the Musk takeover boost tweet engagement for general and contentious users?
- Did blue-tick verification boost tweet engagement for general and contentious users?
- Did the Musk takeover boost tweet engagement more for contentious users versus general users?
Essay Summary
- Platform moderation and verification are important for determining the credibility and suitability of information shared online. Questions of free speech and platform moderation have become ideologically polarized issues.
- The acquisition of Twitter by Elon Musk—a self-professed free-speech absolutist and critic of previous moderation policies on the platform—was seen by many as a threat to the safety and integrity of the platform.
- I used a list of ~138k Twitter users who purchased blue-tick verification in the aftermath of the Musk takeover. I gathered data on a random sample of contentious users, defined as users who have been observed as active within far-right and far-right adjacent online networks.
- I estimated over-time tweet engagement (retweets and likes) with a user-fixed effects model.
- Contentious actors saw a sizeable increase in contentious user engagement following the Musk acquisition: ~70% increase in retweets and 14% increase in likes. This outstripped any general engagement increase for other users.
Implications
Platform moderation and verification policies are important instruments in the fight against online harm and misinformation. They are also central to ongoing debates about free speech and its limits online. Twitter has been accused of having a moderation policy that disproportionately targets the political right by removing the verification status of right-wing accounts, removing tweets, or removing users from the platform altogether (Anyanwu, 2022; Huszár et al., 2022; Otala et al., 2021).
Elon Musk himself is one of those who has accused Twitter of harbouring such bias (Koenig, 2022). It was on this basis that, following his acquisition of Twitter in October 2022, he pledged to overhaul both content moderation and verification procedures. In a tweet posted on November 1, Musk wrote that “Twitter’s current lords & peasants system for who has or doesn’t have a blue checkmark is bullshit” and vowed to introduce a revamped, paid, “Twitter Blue” verification service to take its place.1See https://twitter.com/elonmusk/status/1587498907336118274 or Appendix Figure A1 for the full tweet thread. This new service allowed users to pay a monthly fee to receive a blue tick verification mark appearing by their screen name on the platform.
Separately, Musk vowed to overhaul moderation practices on the Twitter platform. A self-described “free speech absolutist,” Musk’s acquisition of Twitter was welcomed by many quarters critical of what they perceived as Twitter’s overzealous platform moderation policies (Anyanwu, 2022; Milmo, 2022). Elsewhere, Musk has accused Twitter of harbouring left-wing bias—both algorithmic and resulting from enforcement policies (Koenig, 2022). In the wake of his acquisition of Twitter, observers detected considerable spikes in hate speech on the platform (Anyanwu, 2022; Network Contagion Research Institute [@ncri_io], 2022). What is more, the platform has seen the return of numerous controversial actors who had previously been suspended on the platform (Brown, 2022). Subsequent research has confirmed that the Musk acquisition saw a sustained rise in hateful speech on the platform (Hickey et al., 2023).
in the period immediately following the Musk acquisition. Contentious actors, in this context, are actors who either express or are active in networks of far-right accounts on the Twitter platform. In asking this question, I am able to determine whether changes in platform content moderation policies have an effect on the activity and behaviour of particular types of users. By focusing on the immediate period after the Musk acquisition, I am able also to hold constant the policy changes introduced in this period—many of which would go on to change again significantly in subsequent months.
Moderation
Platform moderation policies, such as labelling or removal policies, have been effective in stemming the flow of conspiracy theories online and curbing extremist rhetoric (Ganesh & Bright, 2020; Papakyriakopoulos et al., 2020). Removing contentious users from platforms reduces online discussion about these users and reduces the toxicity of content produced by followers of these users (Jhaver et al., 2021).
Moderation policies such as these, however, also have potential adverse effects on the diversity of platform user bases. The perception of discriminatory moderation practices may push users toward other platforms (Cinelli et al., 2022). Empirical evidence from users of rival micro-blogging platform Gettr suggests that right-wing users were attracted to the platform thanks to its proclaimed free speech protections (Sharevski et al., 2022). Taken together, this means that the perception of biased moderation policies may attract or repel different user bases depending on the platform in question.
Verification
Web platform affordances such as verification, on the other hand, provide users with important heuristics for judging the credibility of information (Flanagin & Metzger, 2007; Metzger et al., 2010). Early research found that the blue tick on Twitter served as a shorthand for credibility and legitimacy (Morris et al., 2012). More recent experimental interventions suggest that the blue tick no longer serves as a marker of credibility, but that identity verification does boost follower engagement (Edgerly & Vraga, 2019; Taylor et al., 2023)
Whether or not verification provides a seal of information credibility for users, verified users continue to play a central role in online information flows. This is most obvious during periods of political unrest when users have to make additional efforts to determine the credibility of online content. Existing research finds that, in these contexts, unverified (automated or “bot”) users are far less visible than verified accounts during periods of political turbulence (González-Bailón & De Domenico, 2021; Rauchfleisch et al., 2017). Verification status, in other words, is an important determinant of visibility when online discussion is at its most febrile.
Taken together, existing evidence suggests that verification may provide: 1) a cognitive heuristic for online users to determine information credibility and 2) may lead to enhanced visibility online. Conferring verification in exchange for payment, therefore, has the potential falsely to boost the visibility and perceived reliability of contentious actors.
Hypotheses
Against this backdrop, I develop two hypotheses. Given the stated position of Musk against existing platform moderation policies and the alleged liberal bias of Twitter as a platform, I predict that his acquisition of Twitter will attract new or otherwise dormant users to the platform who are sympathetic to his views. In turn, this will boost engagement for contentious actors on Twitter who are aligned with these views. I, therefore, predict that any boost in tweet engagement following the Musk acquisition will be larger for contentious users than general users.
Hypothesis 1: The Musk acquisition of Twitter will disproportionately boost tweet engagement for contentious users.
Second, as described above, blue tick verification may provide a visibility and credibility boost for accounts. Given that any account was able to purchase blue tick verification from November 9–November 11, 2022, I, therefore, expect all verified users, whether contentious or not, to see a boost in tweet engagement after verification.
Hypothesis 2: Blue tick verification will boost tweet engagement.
In order to derive an appropriate sample of potentially sympathetic contentious users, I gathered tweets posted by a list of accounts that are active within far-right networks of Twitter users. This was an appropriate sample, given that content moderation is a politically coded and ideologically polarizing issue. Evidence from the United States demonstrates that Republicans are far less likely to support the removal of online speech than Democrats (Appel et al., 2023; Kozyreva et al., 2023). Additionally, a recent analysis of suspensions shows that right-wing accounts on Twitter were more likely to be suspended because of their higher propensity to share low-quality content (Mosleh et al., 2022). Many of the accounts in the list I used have also historically been at risk of suspension (Brown, 2022). Finally, recent research shows that right-wing Twitter users are more likely to retweet content from ideologically congruent (in-group) users (Barberá & Rivero, 2015; Chang et al., 2023). It is this type of user, I hypothesize above, that will receive increased engagement in the aftermath of the Musk acquisition as a result of a newly re-energized user base that is politically aligned with their views.
The analysis compares outcomes for contentious and general user samples. The data comes from a seven-month period in 2022, and engagement was measured in terms of (logged) retweets and likes. I estimated tweet engagement with a user-fixed effects approach where the unit of analysis is the user-day. The regression estimates demonstrate that after Musk’s acquisition of Twitter, contentious actors on the platform see a sizeable boost in post engagement. I suggest these results are attributable to a simultaneous increase in users sympathetic to the views of these accounts, which resulted from Musk’s public signals of intent to overhaul existing moderation policies. Conversely, I did not find evidence that blue tick verification was associated with any additional increase in post engagement. This finding aligns with the more recent research suggesting that verification does not provide a heuristic for reliability (Edgerly & Vraga, 2019).
It is worth noting some key limitations to the research. Another explanation for the results I observed is that there were some unobserved changes to the Twitter algorithm, granting certain users more visibility. This would align with recent research that finds a modest right-wing bias in the Twitter amplification algorithm (Huszár et al., 2022). This would mean that any effect attributable to the Musk acquisition contains some additional algorithmic amplification. Unfortunately, without further experimental inquiry, it is not possible to disentangle observed effects from any algorithmic boost given to right-wing actors. One might have expected, nonetheless, the Twitter algorithm to enhance the visibility of users with verified status. That I find no evidence of this is surprising. One possible explanation for this is that paid verification did not receive any algorithmic boost during the initial rollout.2The subsequent public release of the Twitter ranking algorithm does suggest that users receive a visibility multiplier boost from blue-tick verification. See https://github.com/igorbrigadir/awesome-twitter-algo for a walkthrough. A further limitation is that the use of time dummies does not allow us to disentangle the effect of the Musk acquisition and blue-tick verification. This is because any signaling effect resulting from the Musk acquisition will also operate during and after the period users could purchase verification. Consistent across all estimates, however, is that the Musk acquisition and aftermath saw a sustained boost in engagement for content posted by contentious users—and this boost outstripped any concurrent increase for general users of the platform. Finally, the sample used is a significant limitation. I only have data on individuals who purchased blue tick verification and only that subset of right-wing or right-wing adjacent actors monitored by the data provider (detailed below). I do not have comparable information on left-wing actors and so findings should not be understood as implying that only right-wing actors gained a boost after the Musk acquisition. One possibility is that the Musk acquisition led to a polarization of political engagement generally, meaning one might see similar effects for left-wing users.
Findings
Finding 1: Engagement on posts by contentious users increased after the Musk acquisition.
Estimates are displayed in Appendix Table A1. The acquisition of Twitter by Elon Musk is associated with significant increase in engagement on posts by contentious user tweet engagement. This effect is net of any increased activity on the platform and is more pronounced for retweets than it is for likes (answers RQ1). Exponentiating these coefficients, the Musk acquisition saw an approximately 70% increase in retweets of contentious user tweets and a 14% increase in likes of contentious user tweets.
Panel A of Figure 1 displays coefficients for the three-day time period dummies over the month-long period following the Musk acquisition. Coefficients should be interpreted as within-user change relative to average engagement in the period before the Musk acquisition. I find a sustained increase in post engagement, which dips around the time of verification applications. The subsequent uptick returns to the same levels of engagement as before the opening of blue tick verification. In sum, there is no obvious evidence of any immediate additional effect of blue tick verification on post engagement (answers RQ2).
Finding 2: There is no additional boost conferred by blue-tick verification of contentious users.
Given there is no comparison group, one could argue that the effects we’re observing result from a general increase in Twitter traffic that coincided with the Musk acquisition and changed verification policies. To test this conjecture, I also collected a sample of users who purchased blue tick verification but who are not counted in the reference list of contentious right-wing accounts. For these accounts, I find that the period following the Musk acquisition also saw an increase in post engagement, but it was noticeably smaller: a 28% increase in retweets and a 4% increase in likes (answers RQ3). These results are displayed in Appendix Table A2, and three-day time dummy plots are displayed in Panel B of Figure 1.3For ease of interpretation, I estimate separate models for general and contentious users in the main text. In Table A1 of the Appendix, I also estimate a model with an interaction between the post-Musk acquisition variable and general versus contentious user status. This confirms a sizeable and significant difference in the effect of the Musk acquisition on general versus contentious users: the effect is considerably larger for contentious users than for general users. I also provide density plots of user-level retweet, like, and tweet counts before and after the Musk acquisition in Appendix Figures A7-A9.
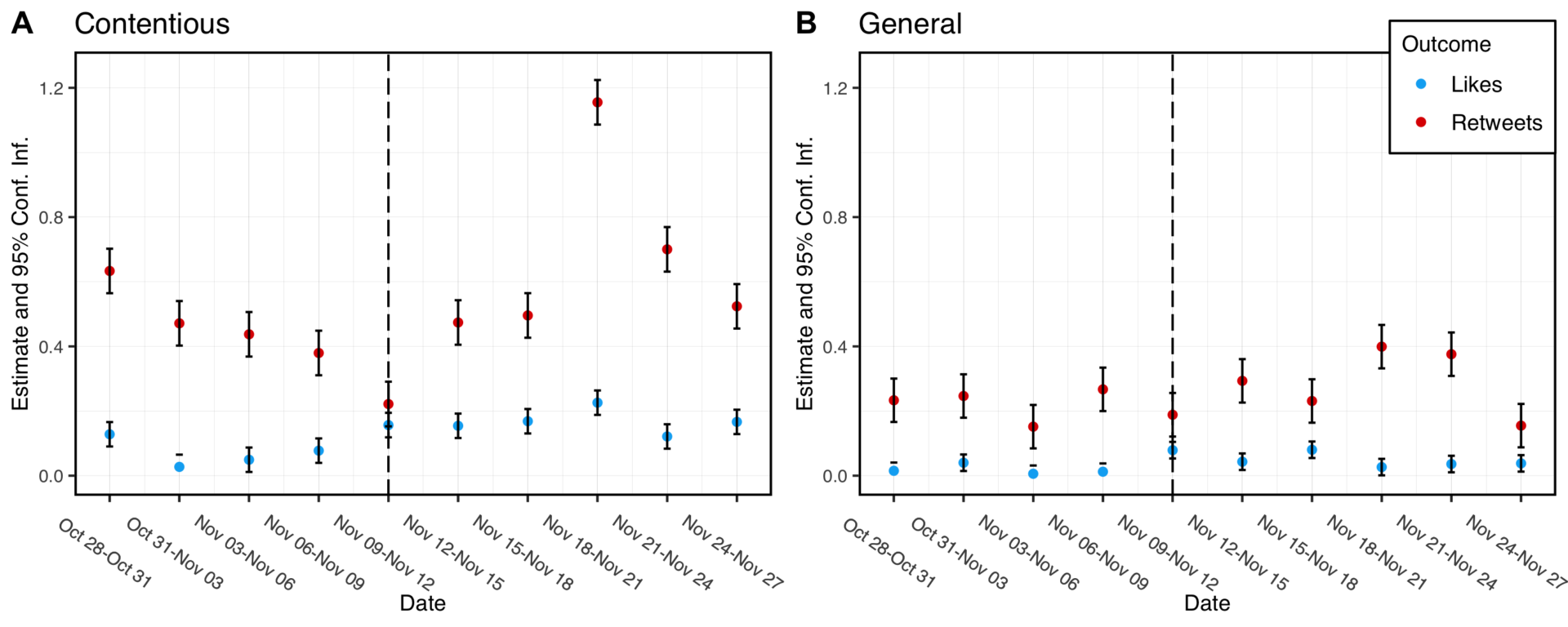
Finally, to determine the type of user-boosting content by contentious users, I took a random sample of tweets by contentious users that received between 100 and 1000 retweets. I then collected information on the users who retweeted these posts. From these accounts, I then took a random sample of 10k users and labeled their ideology using a technique initially developed by Barberá (2015) and made available through Mosleh & Rand (2022) on https://rapidapi.com/.4For a guide on using this (free) API, see https://github.com/mmosleh/minfo-exposure. I confirmed that these users were heavily skewed toward the right-hand-side (right-wing) pole of the ideology distribution. I also found that these users were more active in the aftermath of the Musk acquisition—and that increased activity predated the formal acquisition (see Appendix Figure A10). This suggests that the boost in tweet engagement for contentious users might be explained by a simultaneous increase in online activity among a network of ideologically aligned users.
Methods
In order to determine any engagement boost that followed the Musk acquisition, I made use of a public list of accounts that paid for verification during the short window of its initial launch: November 9, 2022–November 11, 2022. This list was compiled and made public by two software developers, Travis Brown and Casey Ho. The data release was verified and reported on by major international newspapers and civil society organizations at the time.5See e.g., https://www.washingtonpost.com/technology/2022/11/16/musk-twitter-email-ultimatum-termination/, https://www.nytimes.com/2022/11/11/technology/twitter-blue-fake-accounts.html, https://www.splcenter.org/hatewatch/2022/11/16/twitter-blesses-extremists-paid-blue-checks.
These data on the timing of verification are both novel and useful. They are novel because information on the timing of any verification decision is not normally made public through the Twitter API. They are useful as they enable us to determine both the effect of the Musk acquisition and any additional effect of blue tick verification on post engagement by these users. In other words, in the absence of information on the timing of verification, one could mistakenly attribute an uptick in engagement to the Musk acquisition rather than the conferral of a blue tick. Given that both the Musk acquisition and blue tick verification are hypothesized to exert independent effects, it is important to attempt to distinguish between them.
The list includes ∼138k accounts and consists of the account user name at the time of collection and their unique account ID. One of the developers involved in the original data release then merged these accounts with an existing project monitoring the activity of a list of “far-right and far-right-adjacent accounts” on Twitter.6See https://github.com/travisbrown/twitter-watch. This allowed us to filter the 138k newly verified accounts by ranked centrality to these far-right networks. Rank centrality in these networks was measured using the PageRank algorithm on retweet networks within this sample of contentious users. Note that the level of hate or violent speech in tweets by these users is unknown. I know only that they are active in these networks.
With these data, I took the top 1000 accounts based on rank centrality to far-right communication networks—as measured by the data providers.7More information on the full seed accounts for the Twitter Watch project can be found here: https://github.com/travisbrown/unsuspensions. While there is no published sampling protocol, further correspondence with one of the data providers confirmed that the data were seeded with: “a subset of the VoterFraud2020 promoters + a combination of lists from activists and researchers I trust + manually approved new accounts that were suggested by the system.” The VoterFraud2020 dataset can be accessed here: https://voterfraud2020.io/; the full paper on which it is based is by Abilov et al. (2021). It contains information on Twitter accounts claiming election fraud in the United States between October and December 2020. With this information, I then used the R package academictwitteR (Barrie & Ho, 2021) to retrieve all tweets posted by these accounts over the period from May 17, 2022 to November 23, 2022. In total, it was possible to gather data for 961 contentious accounts, giving ∼4.8m tweets. I repeated the same procedure for a sample of general accounts—that is, accounts that purchased blue-tick verification but did not appear on the reference list of contentious right-wing users. In total, I gathered ~1.3m tweets from 943 unique users.8Given that I do not have exact information on the sampling protocol used for these accounts, I label ideological positions using the same technique described in Mosleh & Rand (2022). The distribution of labels for general and contentious users confirms that our general users are evenly distributed across this underlying latent dimension, while ideology labels for contentious accounts are skewed to the right-hand side (see Appendix Figure A2).
I do not know the exact date of each account’s acquisition of blue-tick status. As a result, I used November 11 as my cut-off in the analysis. I am interested in any engagement boost that followed 1) Musk’s acquisition of Twitter on October 27, and 2) paid blue tick verification on or after November 11.
I first used a panel setup where the unit of analysis is the user day. The panel runs from May 17, 2022 to November 29, 2022. I used ordinary least squares (OLS) regression with standard errors clustered on the user. The estimating equation then takes the following functional form:
Retweets (logged)𝑖,𝑡 = 𝛽Musk Acquisition𝑖,𝑡 + 𝛾X𝑖,𝑡 +𝛼𝑖 +𝜖𝑖,𝑡 (1)
where 𝑖 represents individual contentious Twitter users, 𝑡 indexes our unit of observation (here: user-days), X is a control variable representing the total number of tweets posted by user on day 𝑡. I use this control variable as I want to measure tweet engagement net of any boost associated with increased overall activity. The Musk acquisition is operationalized as a binary variable coded 1 if the date of the post is on or after October 27, and 0 otherwise. I estimate separate models for the general and contentious user samples. I plot all of the data for retweet and like dependent variables for contentious and general users in Appendix Figures A3–A6.
It is important to include the number of tweets posted by a given user on a certain day, as this means I am able to estimate daily tweet engagement independent of activity. By introducing user-level fixed effects, I am recovering within-user estimates. In other words, I am able to recover the variation relative to what one might expect for a given user.
To determine the persistence of any effect, I alter the estimating equation to include a set of dummy variables for the sequence of three-day periods following the acquisition. In this model, the Musk acquisition is coded 1 on October 27, and 0 otherwise. This model can be conceptualized as a type of interrupted time-series design where the Musk acquisition binary represents the time of first treatment and the three-day time dummies a set of variables measuring time since treatment. In other words, the equation takes the following form:
Retweets (logged)𝑖,𝑡 = 𝛽Musk Acquisition Day𝑖,𝑡 +𝛽Three-day Dummy𝑖,𝑡 +𝛾X𝑖,𝑡 +𝛼𝑖+𝜖𝑖,𝑡 (2)
Topics
Bibliography
Abilov, A., Hua, Y., Matatov, H., Amir, O., & Naaman, M. (2021). VoterFraud2020: A multi-modal dataset of election fraud claims on Twitter. arXiv. https://doi.org/10.48550/arXiv.2101.08210
Anyanwu, R. R., & Anyanwu, J. (2022, November 23). Why is Elon Musk’s Twitter takeover increasing hate speech? Brookings. https://www.brookings.edu/blog/how-we-rise/2022/11/23/why-is-elon-musks-twitter-takeover-increasing-hate-speech/
Appel, R. E., Pan, J., & Roberts, M. E. (2023). Partisan conflict over content moderation is more than disagreement about facts. SSRN. https://doi.org/10.2139/ssrn.4331868
Barberá, P. (2015). Birds of the same feather tweet together: Bayesian ideal point estimation using Twitter data. Political Analysis, 23(1), 76–91. https://doi.org/10.1093/pan/mpu011
Barberá, P., & Rivero, G. (2015). Understanding the political representativeness of Twitter users. Social Science Computer Review, 33(6), 712–729. https://doi.org/10.1177/0894439314558836
Barrie, C., & Ho, J. C. (2021). ‘AcademictwitteR: An R package to access the Twitter Academic Research Product Track v2 API endpoint’. Journal of Open Source Software 6(62), 3272. https://doi.org/10.21105/joss.03272
Brown, T. (2022). Twitter reports. Github. https://github.com/travisbrown/twitter-watch
Chang, H.-C. H., Druckman, J., Ferrara, E., & Willer, R. (2023). Liberals engage with more diverse policy topics and toxic content than conservatives on social media. OSF. https://doi.org/10.31219/osf.io/x59qt
Cinelli, M., Etta, G., Avalle, M., Quattrociocchi, A., Di Marco, N., Valensise, C., Galeazzi, A., & Quattrociocchi, W. (2022). Conspiracy theories and social media platforms. Current Opinion in Psychology, 47, 101407. https://doi.org/10.1016/j.copsyc.2022.101407
Edgerly, S., & Vraga, E. (2019). The blue check of credibility: Does account verification matter when evaluating news on Twitter? Cyberpsychology, Behavior, and Social Networking, 22(4), 283–287. https://doi.org/10.1089/cyber.2018.0475
Flanagin, A. J., & Metzger, M. J. (2007). The role of site features, user attributes, and information verification behaviors on the perceived credibility of web-based information. New Media & Society, 9(2), 319–342. https://doi.org/10.1177/1461444807075015
Ganesh, B., & Bright, J. (2020). Countering extremists on social media: Challenges for strategic communication and content moderation. Policy & Internet, 12(1), 6–19. https://doi.org/10.1002/poi3.236
González-Bailón, S., & De Domenico, M. (2021). Bots are less central than verified accounts during contentious political events. Proceedings of the National Academy of Sciences, 118(11), e2013443118. https://doi.org/10.1073/pnas.2013443118
Hickey, D., Schmitz, M., Fessler, D., Smaldino, P., Muric, G., & Burghardt, K. (2023). Auditing Elon Musk’s impact on hate speech and bots. arXiv. https://doi.org/10.48550/arXiv.2304.04129
Huszár, F., Ktena, S. I., O’Brien, C., Belli, L., Schlaikjer, A., & Hardt, M. (2022). Algorithmic amplification of politics on Twitter. Proceedings of the National Academy of Sciences, 119(1), e2025334119. https://doi.org/10.1073/pnas.2025334119
Jhaver, S., Boylston, C., Yang, D., & Bruckman, A. (2021). Evaluating the effectiveness of deplatforming as a moderation strategy on Twitter. Proceedings of the ACM on Human-Computer Interaction, 5(CSCW2), 1–30. https://doi.org/10.1145/3479525
Koenig, M. (2022, May 10). Elon Musk declares “Twitter has a strong left wing bias.”Daily Mail. https://www.dailymail.co.uk/news/article-10799561/Elon-Musk-declares-Twitter-strong-left-wing-bias.html
Kozyreva, A., Herzog, S. M., Lewandowsky, S., Hertwig, R., Lorenz-Spreen, P., Leiser, M., & Reifler, J. (2023). Resolving content moderation dilemmas between free speech and harmful misinformation. Proceedings of the National Academy of Sciences, 120(7), e2210666120. https://doi.org/10.1073/pnas.2210666120
Metzger, M. J., Flanagin, A. J., & Medders, R. B. (2010). Social and heuristic approaches to credibility evaluation online. Journal of Communication, 60(3), 413–439. https://doi.org/10.1111/j.1460-2466.2010.01488.x
Milmo, D. (2022, April 14). How ‘free speech absolutist’ Elon Musk would transform Twitter. The Guardian. https://www.theguardian.com/technology/2022/apr/14/how-free-speech-absolutist-elon-musk-would-transform-twitter
Morris, M. R., Counts, S., Roseway, A., Hoff, A., & Schwarz, J. (2012). Tweeting is believing?: Understanding microblog credibility perceptions. In S. Poltrock, C. Simone, J. Grudin, G. Mark, & J. Riedl (Eds.), CSCW ’12: Proceedings of the AMC 2012 conference on Computer Supported Cooperative Work (pp. 441–450). Association for Computing Machinery. https://doi.org/10.1145/2145204.2145274
Mosleh, M., & Rand, D. G. (2022). Measuring exposure to misinformation from political elites on Twitter. Nature Communications, 13, 7144. https://doi.org/10.1038/s41467-022-34769-6
Mosleh, M., Yang, Q., Zaman, T., Pennycook, G., & Rand, D. (2022). Trade-offs between reducing misinformation and politically-balanced enforcement on social media. PsyArXiv. https://doi.org/10.31234/osf.io/ay9q5
Network Contagion Research Institute [@ncri_io]. (2022, October 28). Evidence suggests that bad actors are trying to test the limits on @Twitter. Several posts on 4chan encourage users to amplify derogatory slurs. For example, over the last 12 hours, the use of the n-word has increased nearly 500% from the previous average [Tweet]. Twitter. https://twitter.com/ncri_io/status/1586007698910646272
Otala, J. M., Kurtic, G., Grasso, I., Liu, Y., Matthews, J., & Madraki, G. (2021). Political polarization and platform migration: A study of Parler and Twitter usage by United States of America Congress members. In J. Leskovec, M. Grobelnik, & M. Najork (Eds.), WW ’21: Companion proceedings of the Web Conference 2021 (pp. 224–231). Association for Computing Machinery. https://doi.org/10.1145/3442442.3452305
Papakyriakopoulos, O., Medina Serrano, J. C., & Hegelich, S. (2020). The spread of COVID-19 conspiracy theories on social media and the effect of content moderation. Harvard Kennedy School (HKS) Misinformation Review, 1(3). https://doi.org/10.37016/mr-2020-034
Rauchfleisch, A., Artho, X., Metag, J., Post, S., & Schäfer, M. S. (2017). How journalists verify user-generated content during terrorist crises. Analyzing Twitter communication during the Brussels attacks. Social Media + Society, 3(3), 205630511771788. https://doi.org/10.1177/2056305117717888
Sharevski, F., Jachim, P., Pieroni, E., & Devine, A. (2022). “Gettr-ing” deep insights from the social network Gettr. arXiv. https://doi.org/10.48550/arXiv.2204.04066
Taylor, S. J., Muchnik, L., Kumar, M., & Aral, S. (2023). Identity effects in social media. Nature Human Behaviour, 7(1), 27–37. https://doi.org/10.1038/s41562-022-01459-8
Funding
This research did not benefit from a particular grant.
Competing Interests
None.
Ethics
This research made use of a public list of user names and the Twitter Academic API, which remained open at the time of data collection. No individually identifying information will be made public in replication materials. No ethical review was required.
Copyright
This is an open access article distributed under the terms of the Creative Commons Attribution License, which permits unrestricted use, distribution, and reproduction in any medium, provided that the original author and source are properly credited.
Data Availability
All materials needed to replicate this study are available via the Harvard Dataverse: https://doi.org/10.7910/DVN/ZENMDG
Acknowledgements
I am grateful to Travis Brown for providing the original data used in this paper and for answering my queries.