Peer Reviewed
Source alerts can reduce the harms of foreign disinformation
Article Metrics
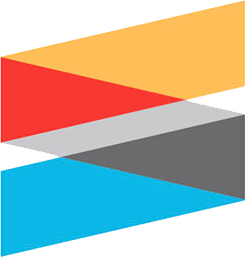
3
CrossRef Citations
Altmetric Score
PDF Downloads
Page Views
Social media companies have begun to use content-based alerts in their efforts to combat mis- and disinformation, including fact-check corrections and warnings of possible falsity, such as “This claim about election fraud is disputed.” Another harm reduction tool, source alerts, can be effective when a hidden foreign hand is known or suspected. This paper demonstrates that source alerts (e.g., “Determined by Twitter to be a Russian government account”) attached to pseudonymous posts can reduce the likelihood that users will believe and share political messages.
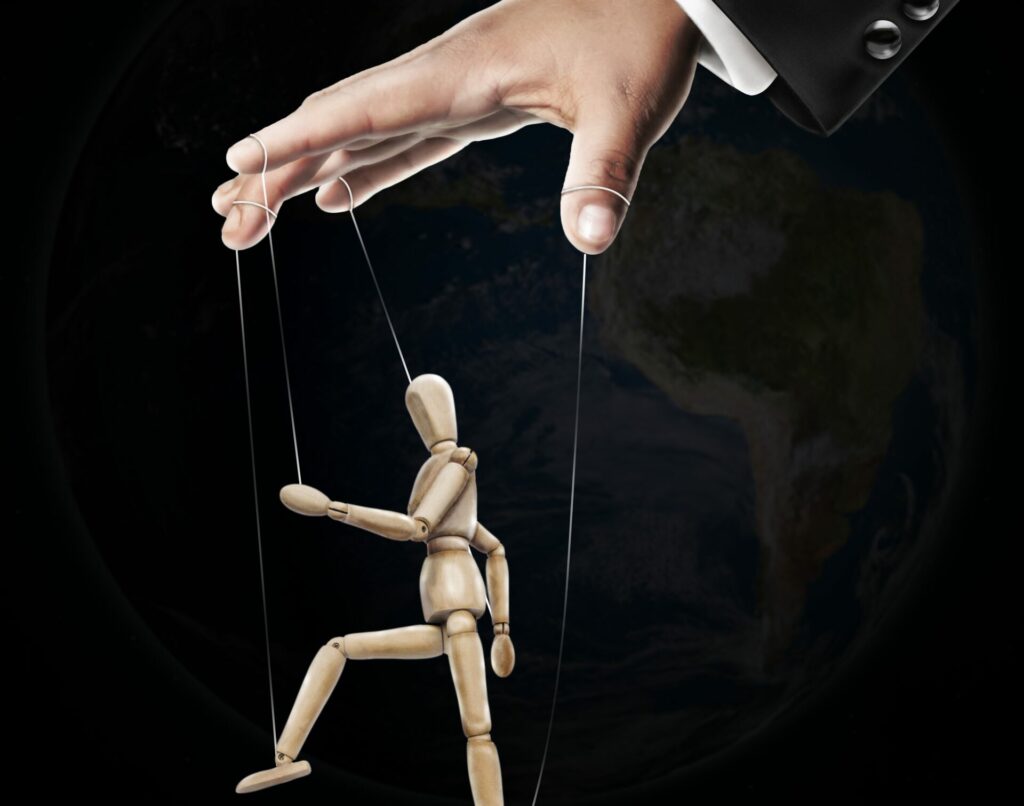
Research Questions
- To what extent do source alerts affect social media users’ tendency to believe and spread pseudonymous disinformation produced by foreign governments?
- Is there a difference between general (e.g., “foreign government”) and specific (e.g., “Russian government”) source alerts in mitigating the belief in and spread of disinformation?
- Are Democrats and Republicans equally likely to respond to source alerts that warn social media users about pseudonymous disinformation produced by foreign government sources?
Essay Summary
- We conducted an experiment via Mechanical Turk (MTurk) (October 22-23, 2020; N = 1,483) exposing subjects to Facebook and Twitter memes based on real disinformation in order to test the efficacy of using source alerts to reduce the tendency of social media users to believe, like, share, and converse offline about pseudonymous disinformation related to the 2020 presidential election.
- We find that source alerts can reduce belief in the meme’s claim and mitigate social media users’ tendency to spread the disinformation online and offline, but the effects vary by the partisanship of the user, the type of social media, and the specificity of the alert.
- The findings advance our understanding of the theoretical promises and limitations of source alerts as a means of combating the belief in and spread of disinformation online. Specifically, our findings suggest that tech companies should continue to invest in efforts to track and warn users of disinformation and to expand their current practices to include country-of-origin tags. Our findings come short of suggesting a one-size-fits all solution, however, as we highlight the importance of considering partisanship as a confounding factor, as well as the importance of considering the nuances of competing social media environments.
Implications
Research on technological solutions to combat mis- and disinformation on social media platforms tends to focus on content-based warnings attached to messages that correct false claims or increase awareness of possible falsity (Bode & Vraga, 2015; Clayton et al., 2019; Lewandowsky et al., 2017; Margolin et al., 2018; Vraga & Bode, 2017). As a result, we know much less about the efficacy of source-based warnings, especially with regard to posts created by covert actors working for hostile foreign powers. Existing studies in this area have examined alerts attached to foreign governments’ overt propaganda outlets, such as Russia’s RT and Iran’s PressTV platforms (Gleicher, 2020; Nassetta & Gross, 2020).
Foreign powers can have multiple persuasion goals related to covert psychological operations on social media, from changing users’ attitudes about specific candidates or policies to decreasing trust in government or established facts. Some, like Russia, have long sought to “deepen the splits” – that is, to exacerbate existing socio-political conflicts (Osnos et al., 2017). Persuasion theorists since Aristotle have emphasized both source and message factors (among others) as key causes of attitude change (Aristotle, Rhetoric). Empirical research has identified multiple source characteristics that influence citizen decision-making, including: expert status (Clark et al., 2012; Hovland & Weiss, 1951; Hovland et al., 1953; Kelman & Hovland, 1953), occupation (Johnson et al., 2011), perceived ideology (Iyengar & Han, 2009; Stroud, 2011; Zaller, 1992), insider status (Carmines & Kuklinski, 1990), likeability (Chaiken, 1980; Lau & Redlawsk, 2001; Sniderman et al., 1991), party position or affiliation (Lodge & Hamill, 1986; Rahn, 1993), public approval (Mondak, 1993; Page et al., 1987), and trustworthiness (Aronson & Golden, 1962; Darmofal, 2005; Hovland & Weiss, 1951; Miller & Krosnick, 2000; Popkin, 1991). Source factors probably operate differently across media types (e.g., traditional versus social media), but we still require work that clarifies those differences.
Social media users may be less inclined to believe or share posts that are written pseudonymously by covert operatives of foreign powers if those individuals were made aware of the true source of the messages. Some users might regard a foreign power, such as the Russian government, as a deceptive, hostile force, while others might have mixed or indifferent views. Yet even those who do not see Russia as a malicious actor would likely think twice about believing or sharing a post if an alert made them aware of the underlying deception – for example, that a Russian agent pretended to be an American from Texas. A source-related alert could activate users’ thoughts about the source’s trustworthiness, likeability, and political affiliation, among others, which could halt the virality of a post that would otherwise be promoted through social media algorithms based on users’ tendency to “like” or “share” the pseudonymous content. Overall, for multiple reasons, the identification of the foreign power behind a message will likely reduce its influence.
To determine whether, and to what extent, source alerts limit the influence of foreign disinformation, we conducted an experimental test of our arguments on a large national MTurk sample a week before the 2020 presidential election.
Our findings demonstrate the utility of an underused technological intervention that can reduce the belief in and spread of disinformation.1We use the term disinformation throughout this article because our experimental manipulations are presented as originating from foreign governments’ covert psychological operations. When a real-world disinformation post gains traction, it sometimes gets decoupled from the foreign power, at least directly – for instance, when an ordinary American citizen shares the message in a way that obscures the original poster. While that individual might not have the intentionality that is a key feature of disinformation campaigns, the message itself remains connected to the foreign campaign. Indeed, a goal of contemporary social media disinformation campaigns is to lead unsuspecting targets to spread the deceptive messages on their own. We follow Guess and Lyons (2020) in defining misinformation “as constituting a claim that contradicts or distorts common understandings of verifiable facts” (p. 10) and Tucker et al. (2018) in defining disinformation as a subset of misinformation that is “deliberately propagated false information” (p. 3, n. 1). As more people continue to receive political information, actively or passively, through social media channels, the threat of pseudonymous disinformation infiltrating our discourse around elections, policy making, and current events remains high. In response, consumer and political pressures mount for social media companies to rein in disinformation and slow its spread. While we observed differences across partisanship, social media platforms, and alert structure variables, future research could clarify the conditions under which source alerts work best. For example, while Facebook users in our sample tended to show greater resistance to source alerts than Twitter users, other Facebook alerts might be more effective if they identify countries that users are predisposed to distrust. Just as our Facebook Democrats were moved to disbelieve and not share disinformation when it was tagged with a specific (“Russian government”) source alert, which conforms to existing left-leaning concerns about Russian interference in general, Republican Facebook users might be more sensitive to alerts identifying China or Iran as the likely instigator. Future research should investigate whether Republican-leaning subjects are more responsive to source alerts that identify other countries. Future research might also clarify whether strength of partisanship is a confounding variable, especially as social media algorithms make it easier for disinformation to be targeted to strong partisans. Finally, future research should investigate the extent to which other political variables, such as strength of ideology, shape responses to alerts.
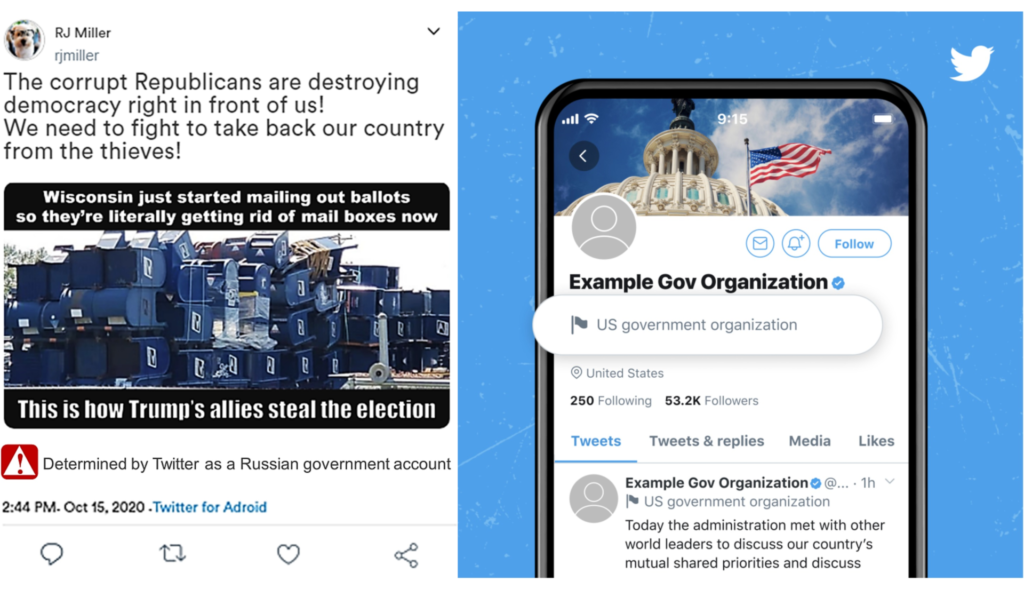
In light of our results, we recommend that Twitter, Facebook, and other social media companies experiment with a range of source alert formats for pseudonymous messages. The alerts shown to be effective in this study are similar to those introduced by Twitter in August 2020 “for government and state-affiliated media accounts.” Firms might also experiment with more probabilistic language for accounts that are not clearly foreign government-controlled and that fail to cross a given threshold for “inauthentic behavior” (e.g., Facebook’s Community Standards, Part IV, Section 20). Of course, because foreign powers try to conceal their efforts, tech companies will rarely be certain that an account is foreign-controlled. However, through human and AI-aided analyses, it is possible to estimate probabilities that accounts have links to foreign powers. In this study, we developed memes based on real disinformation that had been published on social media and had not been removed or otherwise targeted for violating community standards. Finally, we recommend that scholars continue to investigate the causes and consequences of the observed partisan, platform, and alert structure differences.
Findings
Finding 1: Source alerts do reduce social media users’ tendency to believe pseudonymous disinformation in most social media environments; however, no significant effect is found for Republican or Democratic Facebook users exposed to the general (“foreign government”) source alert.
The effects of Table 1 are depicted as predicted probabilities in Figure 1. Looking first at the Facebook conditions, exposure to the Russia alert reduced the probability that a Democratic respondent said the claims were truthful by 18.6%, compared to the no alert condition. Among Democrats in the Twitter conditions, exposure to the general foreign alert reduced the probability that a respondent said the claims were truthful by 26.4%, and exposure to the Russia alert reduced the probability that a respondent said the claims were truthful by 23.2%, compared to the no alert condition. Finally, among Republicans in the Twitter conditions, exposure to the foreign alert reduced the probability that a respondent said the claims were truthful by 16.8%, and exposure to the Russia alert reduced the probability that a respondent said the claims were truthful by 8.3%, compared to the no alert condition. Results were not statistically significant for Republicans in the Facebook conditions.
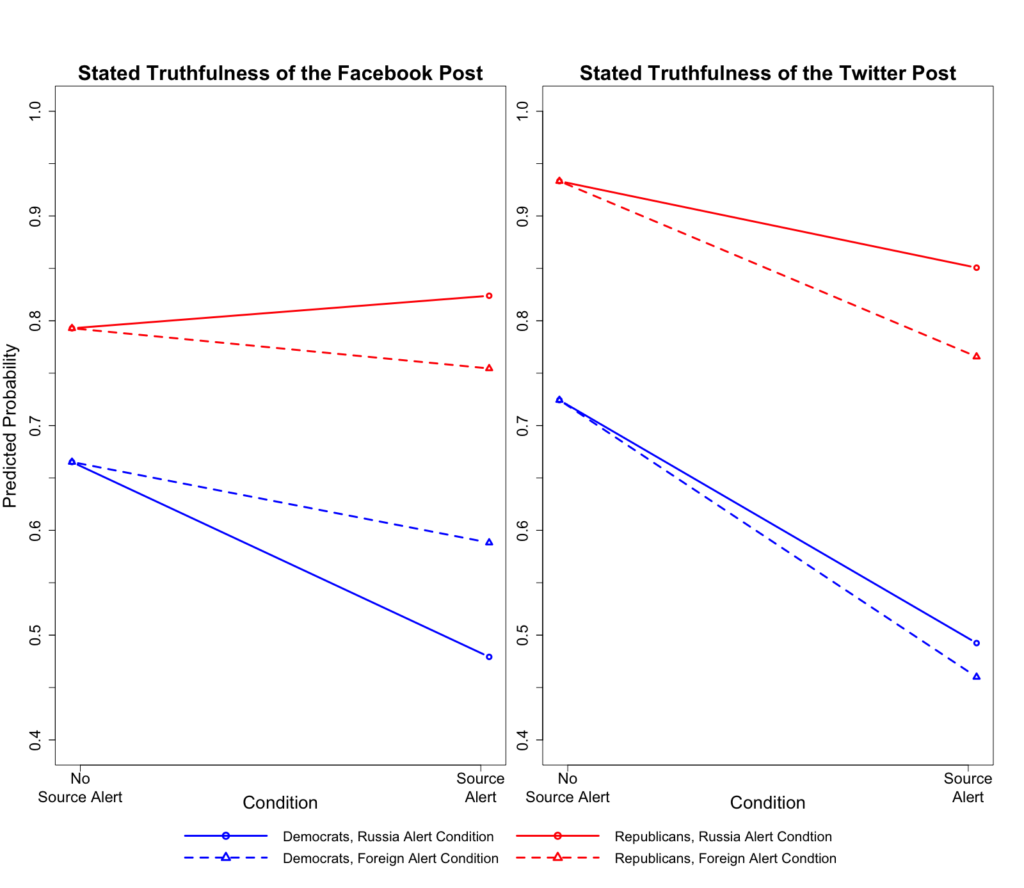
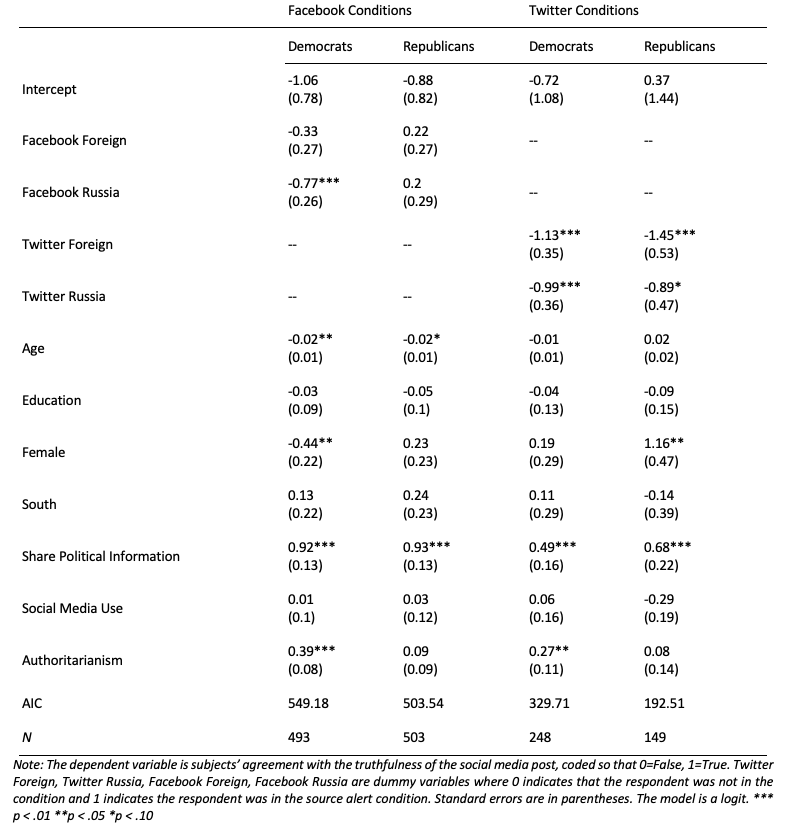
Finding 2: Source alerts do reduce the spread of disinformation on Twitter regardless of the users’ partisanship; however, source alerts do not reduce the spread of disinformation online on Facebook.
In all but one experimental condition, Democratic and Republican Twitter users alike reported that they would be less likely to “like” and “retweet” the disinformation contained in our meme after being exposed to either the general (“foreign government”) or specific (“Russian government”) source alert treatment. As Table 2 shows, for Democrats on Twitter, both the general (p = .039) and specific (p = .008) treatments produced a reported reduction in the likelihood that users would “like” the disinformation. Those in the specific treatment also reported a reduction in the likelihood of sharing the disinformation by retweeting it (p = .016). For Republicans on Twitter, the general alert was effective in reducing the tendency of liking (p = .000) and retweeting (p = .011) the disinformation, and the specific alert was effective in reducing the tendency of liking (p = .004) and retweeting (p = .013) the disinformation.
The findings for Twitter users are notably different from the Facebook users. As Table 2 shows, the tendency of both Democratic and Republican Facebook users to “like” and “share” our experimental memes remained unmoved by our general and specific source alert treatments. The difference in the efficacy of source alerts between the two platforms might be due to the different ways users choose to engage with and exchange information within those social media environments, based on the nuances of those platforms and their networks. This is an area for future scholarly research.
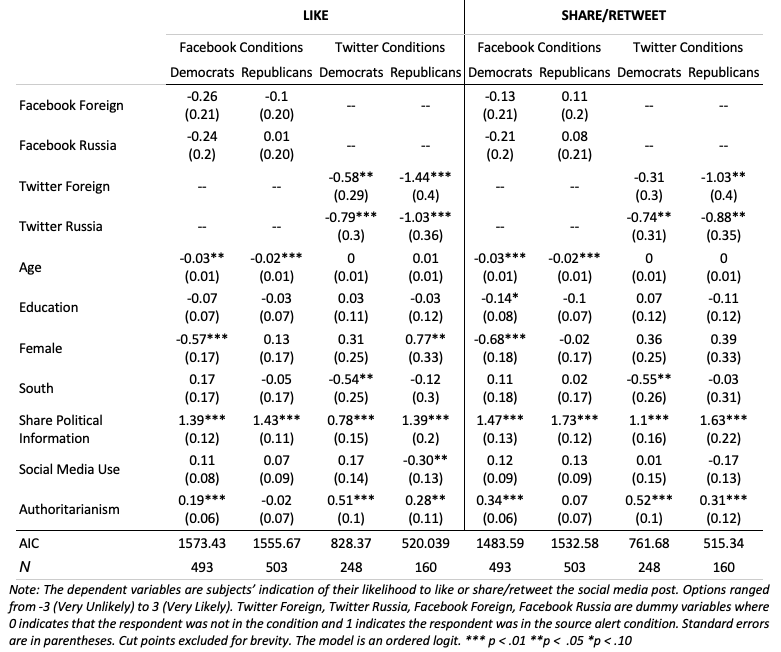
Finding 3: Source alerts reduce the spread of disinformation offline among Democrats in the Facebook conditions; however, the effect for Republicans is more limited and the observed effect is less generalizable to Twitter.
Source alerts reduced the spread of offline disinformation by Democratic Facebook users. We observed no such effects in the Republican Facebook conditions. Democratic Facebook users in the specific (“Russian government”) treatment reported a reduced likelihood of both initiating conversation about the disinformation presented in the meme (p = .046) and of engaging in conversation about the disinformation with friends, family members, acquaintances, etc. (p = .042) (see Table 3). Democratic Facebook users also reported a significantly reduced likelihood of initiating conversation when exposed to the general (“foreign government”) treatment (p = .013). Thus, Democratic Facebook users were relatively consistent in responding to source alerts, which reduced the likelihood that they would spread disinformation offline. In contrast, only the general source alert had a statistically significant effect in the Democratic Twitter condition (p = .040) on the tendency to initiate conversations. Only the general source alert had a statistically significant effect in the Republican Twitter condition (p = .020) on the tendency to engage in conversation about the information in the meme. Our inconsistent Twitter results limit our ability to draw general conclusions about that platform. However, we did find statistically significant results for Democratic and Republican Twitter users related to the dependent variable about initiating offline conversations. That alone provides justification for continuing to explore the efficacy of source cues on Twitter.
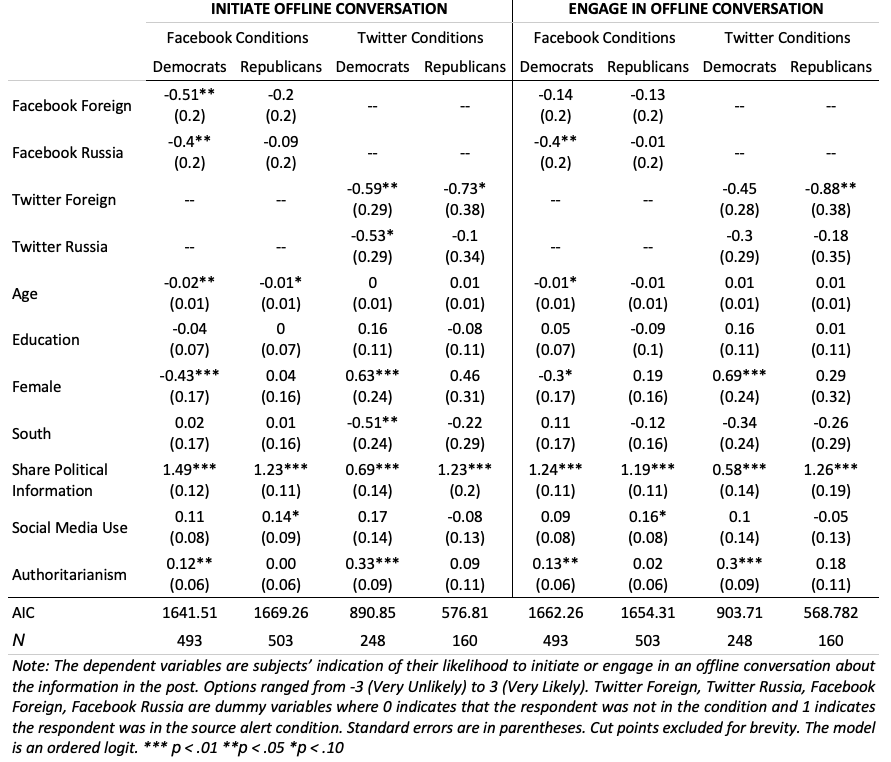
Finding 4: Democratic and Republican partisans respond differently to source alerts, but the difference is conditioned by users’ preferred social media platform.
Although some differences do emerge between Democratic and Republican responses to source alerts, the effects of our treatments on Democratic and Republican Twitter users generally mirrored one another. Furthermore, Democrats on Facebook acted more like Democrats and Republicans on Twitter, when we analyzed the effectiveness of source alerts on the tendency to believe disinformation and to spread disinformation online. However, they responded similarly to Republicans on Facebook, when we analyzed source alerts’ ineffectiveness to curb their tendency to spread disinformation online using Facebook’s “like” and “share” features, perhaps due to subtle differences in how Facebook users of both parties engage with information on the platform. Most notably, the Republican Facebook users appeared resistant to source alerts, a pattern that distinguishes them not only from Democratic Facebook users, but Twitter users of both parties. There appears to be something distinct about Republicans who prefer Facebook as a platform for their social communications or how they engage with that specific social media environment. This distinction could cause them to continue to perpetuate disinformation online and offline regardless of the company’s attempts to put source alerts in place to warn of pseudonymous foreign disinformation attempts. Future research should focus on this problem.
Methods
To determine whether, and to what extent, source alerts limit the influence of foreign disinformation, we conducted an experimental test of the following hypotheses on a large national MTurk sample a week before the 2020 presidential election:
Hypothesis1: Exposure to source alerts will reduce social media users’ tendency to believe pseudonymous disinformation.
Hypothesis2: Exposure to source alerts will mitigate social media users’ tendency to spread pseudonymous disinformation online.
H2a: Exposure to source alerts will mitigate social media users’ tendency to “like” pseudonymous disinformation.
H2b: Exposure to source alerts will mitigate social media users’ tendency to “share/retweet” pseudonymous disinformation.
Hypothesis3: Exposure to source alerts will mitigate social media users’ tendency to spread pseudonymous disinformation offline.
H3a: Exposure to source alerts will mitigate social media users’ tendency to initiate conversations about pseudonymous disinformation.
H3b: Exposure to source alerts will mitigate social media users’ tendency to engage in conversations about pseudonymous disinformation.
Data collection
In order to test our hypotheses, we conducted an experiment utilizing disinformation directly related to the 2020 U.S. presidential election. The experiment took place on October 22-23, 2020 (N = 1,483) and is a two (social media platforms) by two (party consistent message) by two (source cue) design, producing eight experimental conditions and four control conditions. Subjects were recruited via MTurk. All subjects were required to be at least 18 years of age and U.S. citizens. The survey was built and distributed via SurveyMonkey and randomization was used.3We conducted randomization tests involving partisanship and social media types. Results of the randomization checks are available from the authors. Thirteen subjects dropped out of the experiment. The experiment was estimated to take approximately seven minutes to complete.
Prior to exposure to the treatments, we asked subjects about their social media usage and party identification. These questions were used to branch subjects into the appropriate stimuli groups. That is, subjects who indicated a preference for Twitter were branched into the Twitter treatment group; while subjects who indicated a preference for Facebook received a treatment that appeared to be from Facebook. The Twitter and the Facebook posts use the same images, wording, and user profile details, but the meme was altered to appear as a Facebook or Twitter post. Additionally, Democrats and Republicans were exposed to treatment memes that were relevant to their stated partisanship. Democrats were shown a message about alleged voter suppression efforts by Republicans, while Republicans were shown a message about voter fraud efforts allegedly perpetrated by Democrats.4In response to the partisanship question, subjects who initially indicated that they were independent or something else were then prompted to indicate whether they think of themselves as being closer to the Republican or Democratic Party and branched into an experimental condition accordingly. Self-proclaimed Independents and non-partisans often act in the same fashion as their partisan counterparts, marking partisan independence as a matter of self-presentation rather than actual beliefs and behaviors (Petrocik, 2009). Additionally, negative partisanship has been identified as a primary motivator in the way Americans respond to political parties and candidates (Abramowitz & Webster, 2016); our treatments capture appeals to negative partisanship more than they capture loyalty to any one particular party. After the filter questions, all subjects were randomly assigned to the source cues (control, Russian Government Account, Foreign Government Account). Source alerts were presented adjacent to the posts with a highly visible cautionary symbol to draw subjects’ attention to the alert. Finally, subjects were asked a number of questions about the social media post they viewed and how they would engage with the post if it appeared on their Facebook or Twitter feed.
Overall, our MTurk sample is more male, educated, and younger than the U.S. population: 42.2% are female, 69.7% have post-secondary degrees, and 24.8% are 18-29 years old, 59.87% are 30-49, and 15.3% are 50 or older.5There is an emerging consensus among political methodologists about the efficacy of MTurk sampling procedures in experimental research. Berinsky et al. (2012), for example, find MTurk samples to be more representative than “the modal sample in published experimental political science” (p. 351) even if it is less representative than national probability samples or internet-based panels. In addition, 77.6% are white, while 39% reside in the south. Moreover, 52.1% side with the Democratic Party, with 47.9% noting a closer link with the Republican Party.
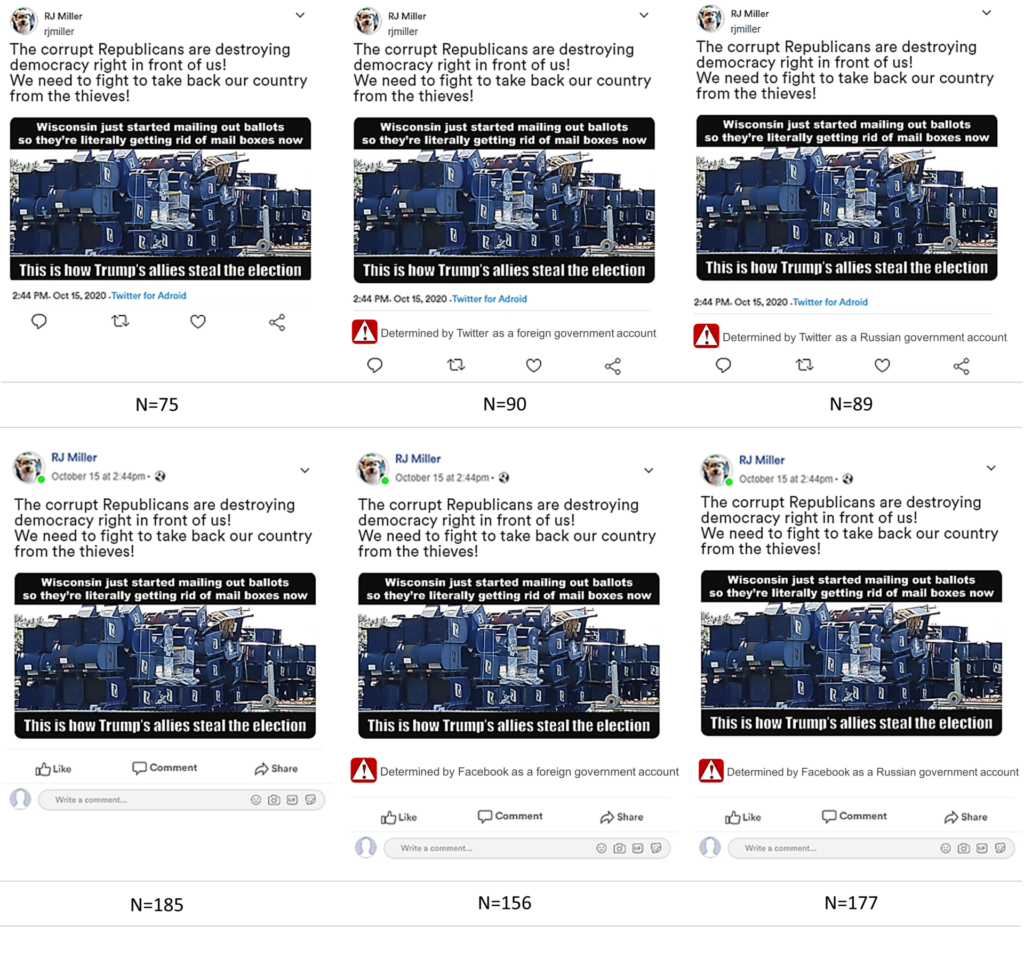
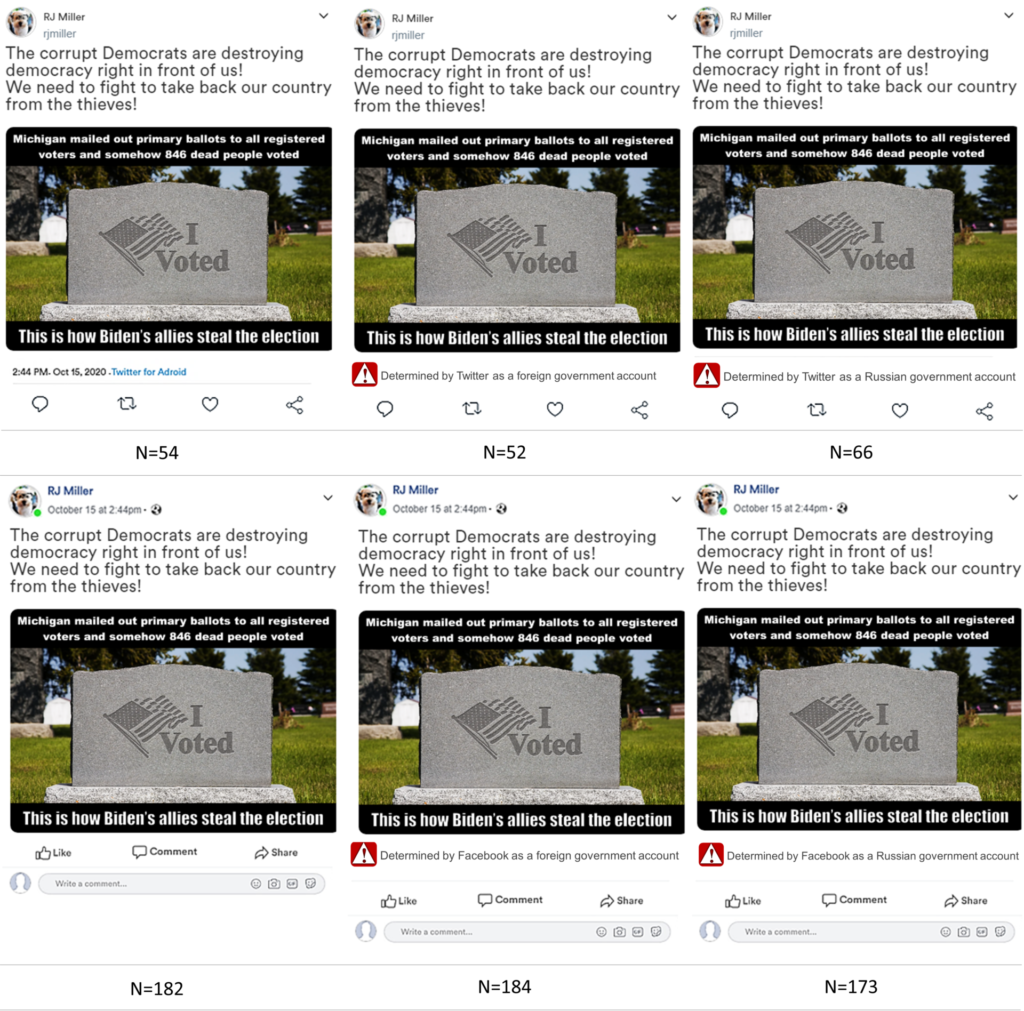
Dependent variables
Subjects answered a number of post-treatment response items to examine their perceptions and behavioral intentions. Specifically, we asked respondents to report how truthful the information in the post was (0 = false and 1 = true), how likely they would be to “like” and “share”/“retweet” the post, and how likely they would be to initiate conversation and engage in conversation about the post offline (each coded -3 to 3, ranging from least to most likely). All of those items, along with others measuring respondents’ partisanship and social media habits, are included in the appendix.
Independent variables
In addition to the condition dummy variables, we include a number of control variables in our models. Age is included because the mean age of subjects who chose the Twitter conditions was lower than the mean age of subjects who chose Facebook. We include a measure of gender because the exclusion of 13 incomplete responses resulted in a gender imbalance in two of our Twitter conditions. Education is included because belief in conspiracy thinking is more common among less educated individuals (Goertzel, 1994). We include a variable for the South because regional differences are common in political behavioral research. Subjects’ tendency to share political information and their rate of social media use are used to control for social media habits. Finally, we included an authoritarian personality measure because authoritarianism has been shown to be a key variable in influencing political behaviors in multiple domains (Feldman, 2003). Specifically, more authoritarian individuals have been found to staunchly defend prior attitudes and beliefs. Low authoritarian individuals, on the other hand, have demonstrated a greater need for cognition (Hetherington & Weiler, 2009; Lavine et al., 2005; Wintersieck, forthcoming). As a result, high authoritarians may be less moved by source alerts on information they are likely to believe, while low authoritarians may be more likely to take up these cues when making assessments about the memes. Information about coding these variables is presented in the appendix.
External validity
A number of steps were taken to increase the external validity of the experiment. First, we designed memes based on real disinformation about election fraud. Each meme was similar in imagery, language, and length. Furthermore, each meme presented disinformation that sows discord in U.S. electoral politics, a goal that would further the interests of foreign governments, like Russia, and that could, therefore, conceivably be spread by those pseudonymous sources. Second, we used two filter questions prior to exposure to the treatment to mimic actual social media engagement for the subjects. Specifically, we used a party ID filter that allowed us to create a typical social media echo chamber by filtering Democrats into conditions with misinformation that a Democrat would likely encounter on social media and Republicans into conditions with misinformation that a Republican would likely encounter on social media. Additionally, the social media filter allowed us to put subjects into social media treatment conditions with social media platforms that they were more likely to be familiar with. That is, if a subject does not use Twitter, or understand the Twitter platform, it is highly unlikely that they would indicate a willingness to share the tweet, etc. This becomes a confounding variable because we are unable to parse out whether lack of willingness to share is because of a lack of understanding of the platform or because of the source cue.
Topics
- Disinformation
- / Platforms
- / Russia
- / Social Media
- / Sources
- / Twitter/X
Bibliography
Abramowitz, A. I., & Webster, S. (2016). The rise of negative partisanship and the nationalization of U.S. elections in the 21st century. Electoral Studies, 41, 12–22. https://doi.org/10.1016/j.electstud.2015.11.001
Aronson, E., & Golden, B. W. (1962). The effect of relevant and irrelevant aspects of communicator credibility on opinion change. Journal of Personality, 30(2). https://doi.org/10.1111/j.1467-6494.1962.tb01680.x
Berinsky, A. J., Huber, G. A., & Lenz, G. S. (2012). Evaluating online labor markets for experimental research: Amazon.com’s Mechanical Turk. Political Analysis, 20(3), 351–368. https://doi.org/10.1093/pan/mpr057
Bode, L., & Vraga, E. K. (2015). In related news, that was wrong: The correction of misinformation through related stories functionality in social media. Journal of Communication, 65(4), 619–638. https://doi.org/10.1111/jcom.12166
Carmines, E. G., & Kuklinski, J. H. (1990). Incentives, opportunities, and the logic of public opinion in American political representation. In J. A. Ferejohn & J. H. Kuklinski (Eds.), Information and democratic processes (pp. 240-68). University of Illinois Press.
Chaiken, R. L. (1977). The use of source versus message cues in persuasion: an information processing analysis (Publication No. 1656) [Doctoral dissertation, University of Massachusetts Amherst]. https://scholarworks.umass.edu/dissertations_1/1656.
Clark, J. K., Wegener, D. T., Habashi, M. M., & Evans, A. T. (2012). Source expertise and persuasion: The effects of perceived opposition or support on message scrutiny. Personality and Social Psychology Bulletin, 38(1), 90–100. https://doi.org/10.1177/0146167211420733
Clayton, K., Blair, S., Busam, J. A., Forstner, S., Glance, J., Green, G., Kawata, A., Kovvuri, A., Martin, J., Morgan, E., Sandhu, M., Sang, R. Scholz-Bright, R., Welch, A., Wolff, A. G., Zhou, A. & Nyhan, B. (2020). Real solutions for fake news? Measuring the effectiveness of general warnings and fact-check tags in reducing belief in false stories on social media. Political Behavior, 42(4), 1073–1095. https://doi.org/10.1007/s11109-019-09533-0
Darmofal, D. (2005). Elite cues and citizen disagreement with expert opinion. Political Research Quarterly, 58(3), 381–395. https://doi.org/10.2307/3595609
Feldman, S. (2003). Enforcing social conformity: A theory of authoritarianism. Political Psychology, 24(1), 41–74. https://doi.org/10.1111/0162-895x.00316
Gleicher, N. (2020, June 4). Labeling state-controlled media on Facebook. Facebook Newsroom. https://about.fb.com/news/2020/06/labeling-state-controlled-media/
Guess, A. M., & Lyons, B. A. (2020) Misinformation, disinformation, and online propaganda. In N. Persily & J. Tucker (Eds.), Social media and democracy: the state of the field, prospects for reform (pp. 10–33). Cambridge University Press.
Hetherington, M. J., & Weiler, J. D. (2009). Authoritarianism and polarization in American politics. Cambridge University Press. https://doi.org/10.1017/cbo9780511802331
Hovland, C. I., & Weiss, W. (1951). The influence of source credibility on communication effectiveness. Public Opinion Quarterly, 15(4), 635–650. https://doi.org/10.1086/266350
Hovland, C. I., Janis, I. L., & Kelley, H. H. (1953). Communication and persuasion. Yale University Press.
Iyengar, S., & Hahn, K. S. (2009). Red media, blue media: Evidence of ideological selectivity in media use. Journal of Communication, 59(1), 19–39. https://doi.org/10.1111/j.1460-2466.2008.01402.x
Johnson, T., Dunaway, J., & Weber, C. R. (2011). Consider the source: Variations in the effects of negative campaign messages. Journal of Integrated Social Sciences, 2(1), 98–127. https://www.jiss.org/documents/volume_2/issue_1/JISS_2011_Consider_the_Source.pdf
Kelman, H. C., & Hovland, C. I. (1953). “Reinstatement” of the communicator in delayed measurement of opinion change. The Journal of Abnormal and Social Psychology, 48(3), 327. https://doi.org/10.1037/h0061861
Lau, R. R., & Redlawsk, D. P. (2001). Advantages and disadvantages of cognitive heuristics in political decision making. American Journal of Political Science 45(4), 951–971. https://doi.org/10.2307/2669334
Lavine, H., Lodge, M., & Freitas, K. (2005). Threat, authoritarianism, and selective exposure to information. Political Psychology, 26(2), 219–244. https://doi.org/10.1111/j.1467-9221.2005.00416.x
Lewandowsky, S., Ecker, U. K., & Cook, J. (2017). Beyond misinformation: Understanding and coping with the “post-truth” era. Journal of Applied Research in Memory and Cognition, 6(4), 353–369. https://doi.org/10.1016/j.jarmac.2017.07.008
Lodge, M., & Hamill, R. (1986). A partisan schema for political information processing. The American Political Science Review,80(2), 505–520. https://doi.org/10.2307/1958271
Margolin, D. B., Hannak, A., & Weber, I. (2018). Political fact-checking on Twitter: When do corrections have an effect? Political Communication, 35(2), 196–219. https://doi.org/10.1080/10584609.2017.1334018
Miller, J. M., & Krosnick, J. A. (2000). News media impact on the ingredients of presidential evaluations: Politically knowledgeable citizens are guided by a trusted source. American Journal of Political Science, 44(2), 301–315. https://doi.org/10.2307/2669312
Mondak, J. J. (1993). Source cues and policy approval: The cognitive dynamics of public support for the Reagan agenda. American Journal of Political Science, 37(1), 186–212. https://doi.org/10.2307/2111529
Nassetta, J., & Gross, K. (2020). State media warning labels can counteract the effects of foreign misinformation. Harvard Kennedy School (HKS) Misinformation Review, 1(7). https://doi.org/10.37016/mr-2020-45
Osnos, E., Remnick, D., & Yaffa, J. (2017, March 6). Trump, Putin, and the new cold war. The New Yorker. https://www.newyorker.com/magazine/2017/03/06/trump-putin-and-the-new-cold-war
Page, B. I., Shapiro, R. Y., & Dempsey, G. R. (1987). What moves public opinion? The American Political Science Review, 81(1),23–43. https://doi.org/10.2307/1960777
Petrocik, J. R. (2009). Measuring party support: Leaners are not independents. Electoral Studies, 28(4), 562–572. https://doi.org/10.1016/j.electstud.2009.05.022
Popkin, S. L. (1991). The reasoning voter: Communication and persuasion in presidential campaigns. University of Chicago Press.
Rahn, W. M. (1993). The role of partisan stereotypes in information processing about political candidates. American Journal of Political Science, 37(2), 472–496. https://doi.org/10.2307/2111381
Sniderman, P. M., Brody, R. A., & Tetlock, P. E. (1991). Reasoning and choice: Explorations in political psychology. Cambridge University Press.
Stroud, N. J. (2011). Niche news: The politics of news choice. Oxford University Press.
Tucker, J. A., Guess, A., Barberá, P., Vaccari, C., Siegel, A., Sanovich, S., Stukal, D., & Nyhan, B. (2018, March 29). Social media, political polarization, and political disinformation: A review of the scientific literature. Hewlett Foundation. https://www.hewlett.org/wp-content/uploads/2018/03/Social-Media-Political-Polarization-and-Political-Disinformation-Literature-Review.pdf
Vraga, E. K., & Bode, L. (2017). Using expert sources to correct health misinformation in social media. Science Communication, 39(5), 621–645. https://doi.org/10.1177/1075547017731776
Wintersieck, A. (forthcoming). Authoritarianism, fact-checking, and citizens’ response to presidential election information. In Barker, D., & Suhay, E. Elizabet (Eds.), The politics of truth in polarized America. Oxford University Press
Zaller, J. R. (1992). The nature and origins of mass opinion. Cambridge University Press.
Funding
Funding for this study was provided by the Department of Political Science and the College of Humanities and Sciences at Virginia Commonwealth University.
Competing Interests
The authors have no competing interests.
Ethics
This study was approved on October 22, 2020, by the Institutional Review Board at Virginia Commonwealth University (HM20017649_Ame1). All participants provided informed consent prior to participating in these studies.
The authors determined gender categories in the survey with a set of three questions. The categories included: “other,” “male,” “female,” “transgender,” “do not identify as male, female, or transgender,” and “prefer not to say.” Respondents who chose “other” were then given an open-ended question. However, all subjects but one selected “male” or “female.” Moreover, because of the need to drop 13 cases who did not complete the experiment, there was a gender imbalance in the Democrat Facebook condition. As a result, the authors decided to control for gender in the statistical models, using a binary measure. The authors included gender in the study because it is a key cause of political behavior, as are race and ethnic identities. Although the authors did not use race and ethnicity in the statistical models, they did ask respondents about their racial and ethnic identities, using questions from NORC’s AmeriSpeak battery.
Copyright
This is an open access article distributed under the terms of the Creative Commons Attribution License, which permits unrestricted use, distribution, and reproduction in any medium, provided that the original author and source are properly credited.
Data Availability
All materials needed to replicate this study are available via the Harvard Dataverse: https://doi.org/10.7910/DVN/9IYSBS