Peer Reviewed
How different incentives reduce scientific misinformation online
Article Metrics
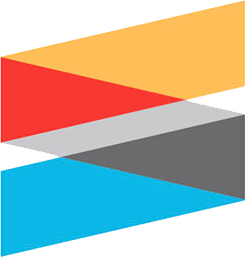
0
CrossRef Citations
Altmetric Score
PDF Downloads
Page Views
Several social media employ or consider user recruitment as defense against misinformation. Yet, it is unclear how to encourage users to make accurate evaluations. Our study shows that presenting the performance of previous participants increases discernment of science-related news. Making participants aware that their evaluations would be used by future participants had no effect on accuracy. Lastly, monetary rewards have the largest effect on accuracy. Our study provides support for the idea that a person’s motivation is an essential component of their propensity to be vigilant online and that it is possible to devise strategies to strengthen this motivation.
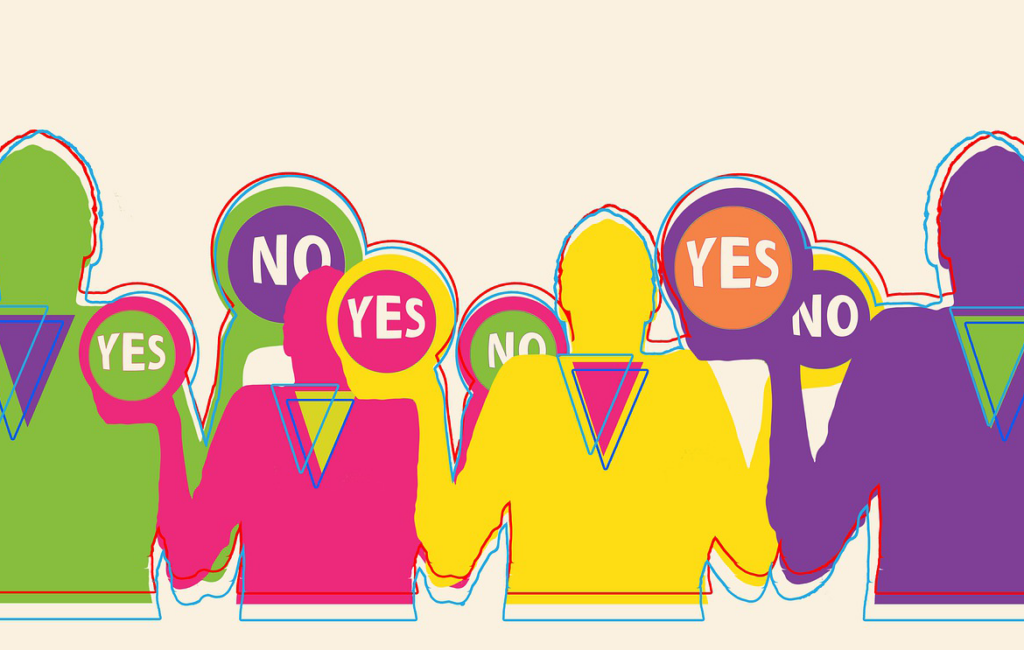
Research Questions
- Do non-monetary interventions (e.g., prompting a comparison with others’ performance or having one’s own evaluation inform others’ decisions) incentivize online users to evaluate scientific and pseudoscientific content more accurately?
- How do non-monetary incentives compare to remuneration?
- Do users employ different search strategies when under different types of incentives?
Essay Summary
- In a pre-registered online experiment (N = 3,999), we simulated a social media environment and measured participants’ ability to identify whether a Facebook-like post was scientifically valid or invalid.
- Our results show that presenting the success rate of previous raters works as an incentive to evaluate information more accurately, but that monetary bonuses provide the strongest increase in accuracy, and that only remuneration is associated with a greater use of external resources (e.g., search engines) that have been shown to meaningfully improve evaluation of new content.
- While many avenues have been explored in recent years to try to stem the spread of misinformation online, our study provides further support to the idea that individuals’ motivation is an essential component of their propensity to be vigilant on the internet and that it is possible to design strategies aimed at strengthening this motivation.
Implications
The circulation of poor-quality information on the internet is a well-known problem with huge costs for our societies in the short and long term (Bruns et al., 2021; Jarvis et al., 2022). Social scientists, regulators, and social media platforms are all trying to improve the quality of online information. Some strategies for reducing the spread of disinformation target the sources, imposing filters on what can pass as information and sometimes even banning some sources (Bak-Coleman, 2022), like social media accounts targeted by so-called anti-fake-news laws (Alemanno, 2018). For instance, Google and Facebook policies ban websites spreading fake news from using their online advertising services (Wingfield et al., 2016). In 2020, Twitter decided to flag tweets with harmful or inaccurate information about COVID-19 (Roth & Pickles, 2020), and Facebook placed related articles immediately beneath controversial stories (Su, 2017). Clearly, these strategies are limited in scope and are seen by some political factions as both potentially abusive and infringing on people’s freedom of expression (Kozyreva et al., 2022). Moreover, there is the conceptual problem of defining what constitutes an accurate (i.e., correct) response, which requires an objective reference point that eventually will need to be confirmed by experts.
This implies that end-user strategies are to be favored, as they boost people’s critical thinking ability while at the same time preserving political freedom of expression. Research has shown that people are indeed able to properly evaluate online information when given the appropriate tools or good reasons to do so (Pennycook & Rand, 2021; Roozenbeek et al., 2022).
One effective approach is to incentivize individuals to provide unbiased assessments. Incentives, unlike the various forms of debunking, have the main advantage that they are not tailored specifically to the content of each false claim. The use of incentives has been proven to increase task accuracy in various computerized tasks (Rogstadius et al., 2011; Shaw et al., 2011; Tang et al., 2019). Monetary incentives, in particular, have been shown to significantly improve the ability to spot false information even when content is technical and goes beyond users’ expertise (Panizza et al., 2022). However, monetary incentives have very limited applicability and are less scalable than non-monetary incentives because their costs increase linearly as the number of users increases. Beyond the obvious practical issue of adapting such interventions to serve a large population, there is again the problem of defining what constitutes an accurate response.
However, extending a pay-for-accuracy scheme would require categorizing most information as either accurate or inaccurate, which is clearly impossible from an epistemological perspective. From a practical perspective, a comprehensive cost-benefit analysis would be necessary to determine whether a pay-for-accuracy scheme is enforceable by social media platforms, but with the element at our disposal, we believe it unlikely that social media platforms would be able to establish and maintain such a scheme. Compared to non-monetary incentives that rely on minimal message interventions, interventions based on monetary incentives cannot be easily scaled or fully automated (Grüning et al., 2023). All in all, experimental investigations on monetary incentives are valuable, but other types of incentives should also be properly investigated.
Many interventions that aim to shape human behavior leverage people’s social tendencies to cooperate and compare with others (Baumeister & Leary, 2017; Goldstein et al., 2008). In fact, decision-makers are influenced by other people’s behavior as well as by the consequences of their own behavior (Chapman, 2019). Panizza et al. (2023) show that online users’ evaluations are influenced by prior judgments of other users, even when they report not having done so, and regardless of whether prior judgments were accurate or misleading. Non-monetary incentives can take a variety of forms, from eliciting one’s competitive attitudes against other players to appealing to one’s sense of responsibility when their judgment could affect others. Reputation and the desire to fit in and conform to the expectations of one’s peer group is a powerful motivator. This phenomenon is particularly pronounced in the realm of social media, where achievement is often gauged by the extent of one’s network and the validation received from others.
Given these considerations, we chose to explore the effectiveness of non-monetary incentives in two different ways, both of which depended on social factors: 1) comparing oneself against a success benchmark of previous raters, and 2) making participants aware that their evaluations would be used by future participants. The first approach stems from the observation that some of the most popular content on social media are posts inviting users to engage, for instance, by solving puzzles or sharing personal thoughts (Clark, 2021). The second approach is inspired by prosocial initiatives such as citizen science or crowdsensing (Scholtz & Mloza-Banda, 2019) and tries to engage participants by making salient the social consequences of their evaluations.
We found that intervention 1 is effective: Presenting the performance of previous raters worked as an incentive to evaluate information more accurately, probably because people in this experimental condition were encouraged to be more vigilant towards disinformation. Humans have special cognitive skills—what Sperber et al. (2010) call “epistemic vigilance”—dedicated to monitoring information coming from others. Such information is evaluated against our own prior knowledge, but the amount of cognitive effort involved in deciding whether or not to believe a certain fact also depends on the relevance of that information to the recipient, which in turn depends on her own goals and priorities at the time she processes it.
In that context, the first implication of this experiment is that it is, in principle, possible to incentivize accurate crowdsourced fact-checking using non-monetary incentives that are both highly scalable and do not restrict the users’ freedom of choice. Although prior knowledge is obviously important, if a high level of accuracy makes a difference, users will be more vigilant and attentive, even to their own cognitive limitations. Several results in the literature support the idea that individuals do mobilize their epistemic vigilance depending on the stakes associated with knowing the truth on a given topic. For instance, in the health domain, citizens are generally more vigilant against misinformation and more trusting of the medical consensus when they have to make an important decision about treatment choices (Motta & Callaghan, 2020). The challenge is to make the stakes of this epistemic vigilance more salient when, most of the time, false information has only a limited negative impact on the daily life of individuals.
We also find that monetary bonuses provide an even stronger increase in accuracy. On the one hand, this finding might speak for the robustness and effectiveness of monetary rewards. In addition, compensation is a minimum requirement for completing distressing tasks such as content moderation, and platforms should not underestimate the ethical considerations of enlisting users without providing some sort of remuneration. On the other hand, this finding also implies that policymakers should try to design stronger scalable strategies that aim at making individuals more accurate. For instance, the present investigations tested two socially relevant strategies (i.e., presenting participants with others’ performance vs. making them aware that their judgments will inform others) as alternatives. However, it might be the case that increased accuracy benefits from the joint force of these two interventions. For instance, individuals’ accuracy might increase with interventions that simultaneously present the performance of peers and make participants’ responses available to other participants. In other words, we have emerging evidence for the fact that non-monetary incentives can be effective, but it is important to understand (i) how they work (e.g., social comparison, peer pressure, etc.) and (ii) how their effectiveness can be boosted if we want them to be effective in increasing accuracy.
Finally, we found that only monetary bonuses are associated with a greater use of successful search strategies. Despite the fact that intervention increases accuracy, it does not do so because people are using search strategies like lateral reading or source checking more often than non-incentivized subjects. This difference in behavior suggests that users do not necessarily need to search for additional information to evaluate content more accurately despite additional information producing clear benefits. The separation between evaluation outcomes and strategy supports the line of research suggesting that mere attention is sufficient to increase the discernment between false and true information (Fazio, 2020; Pennycook & Rand, 2021). It is unclear what might underlie this difference in behavior: whether different incentives impact different types of psychological mechanisms or whether simply monetary incentives have a quantitatively stronger impact on attention and motivation. In either case, providing these strategies could produce a further increase in performance in non-monetary incentives and could perhaps close the gap with monetary incentives.
One potential limitation of our experimental design is the possibility that users, instead of revealing their personal evaluations, may simply try to respond to what they think the experimenters think about the validity of the content. While it’s challenging to eliminate this issue, our approach to significantly mitigate it involved the careful selection and pre-testing of multiple scientific content posts that minimized reliance on widely circulated scientific claims and hence did not spur huge polarization in the public debate. Where this was not possible—concerning denialist claims about climate change—we included a post that went in the opposite direction (i.e., a post predicting an imminent human extinction within the next decade). In addition to using scientifically invalid posts pushing in opposite directions, instructions were worded to avoid any possible expectations about the researchers’ beliefs on each topic. This problem has been recently raised and discussed in the literature, with encouraging evidence assuaging concerns about potential demand effects (Rathje et al., 2023). Moreover, although it is impossible to completely rule out this problem, we sought to address this concern by selecting and pre-testing posts with content that does not polarize the public debate or by including posts that extremize widely held beliefs (e.g., about the imminent extinction of humans due to catastrophic climate change) to balance climate change denial posts.
Another potential limitation to the applicability of the interventions is that prior exposure to false claims might counteract the use of incentives, if not backfire (Lewandowsky et al., 2012; Swire-Thompson et al., 2022). As with other interventions acting on users’ motivation, the effectiveness and helpfulness of incentives depend on their timing relative to initial exposure. However, given the ease with which non-monetary incentives could be introduced on an online platform, it is reasonable to expect that such interventions could act before users are exposed online.
There are other examples in the literature of non-content-specific interventions that have shown promising results. Fazio (2020) showed that introducing a mandatory pause during users´ evaluation of a headline reduces the likelihood of fake news being shared. Similarly, Pennycook et al. (2021) showed how working on shifting users’ attention can reduce the spread of false claims. In contrast to these works, our intervention offers an explicit incentive for users to be accurate that takes the form of a reward or the possibility to avoid a potential loss (e.g., public shame). This type of explicit incentive can be used on top of nudges that modify the choice architecture without the users being aware of it.
Non-monetary incentives can be useful in combating misinformation because they are not content-specific and do not restrict users’ freedom. Our study provides evidence on their effectiveness. Governments and large online platforms can use them to promote the accuracy of online users’ judgments without having to determine and impose what is and is not accurate. However, the mechanisms by which non-monetary incentives operate are still a bit of a puzzle. While monetary incentives encourage more frequent use of fact-checking techniques, non-monetary incentives appear to operate at a different level, mobilizing users’ epistemic vigilance.
Findings
Finding 1: Merely presenting the performance of previous raters provides an incentive to evaluate information more accurately.
Finding 2: Participants who knew that their responses would be used by future participants did not significantly increase accuracy compared to the control condition.
We asked participants to rate the scientific validity of a Facebook post, and we recorded their responses on a scale from 1 (least accurate) to 6 (fully accurate).
Participants rated the scientific validity of a Facebook post, and their responses were recorded on a scale from 1 (least accurate) to 6 (fully accurate). Displaying the performance of previous subjects increased accuracy (M = 4.01, β= 0.20 [0.00,0.40], z = 2.54, p = .016). By contrast, informing participants that their responses would be used by future participants did not significantly increase accuracy compared to the control condition (M = 3.95 versus M = 3.87, β = 0.11 [-0.09,0.32], z = 1.45, p = .175).
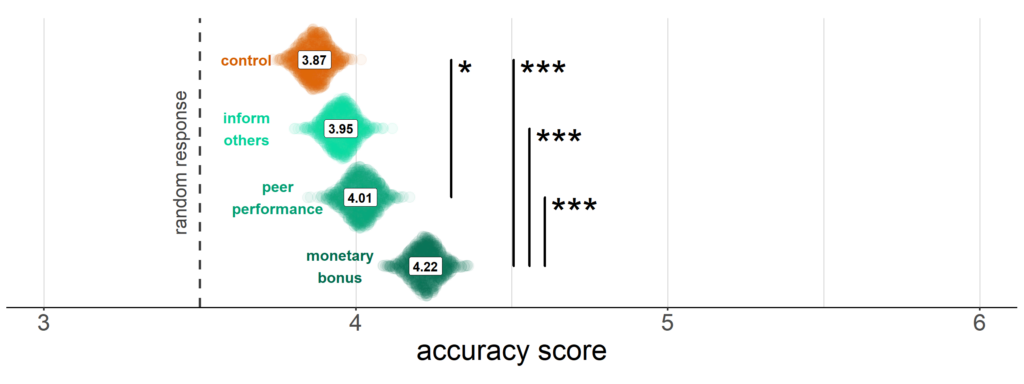
Finding 3: Monetary bonuses provide an even stronger increase in accuracy.
How do non-monetary incentives compare to remuneration of correct answers? Of the three interventions, monetary bonus was the one that had the strongest impact (M = 4.22, β = 0.50 [0.29,0.70], z = 6.21, p < .001), and was significantly more effective than both making the evaluation public (β = 0.38 [0.18,0.59], z = 4.79, p < .001) and providing peer performance (β = 0.29 [0.09,0.50], z = 3.66, p < .001). If incentives of social nature may motivate people to make more careful evaluations of news, promises of compensation are between 2.5 and 4.3 times more effective.
Finding 4: Only monetary bonuses are associated with a greater use of successful search strategies.
Do incentives lead participants to search for information differently? We measured self-report assessments of two search strategies: lateral reading (comparing claims on other websites through the use of a search engine) and click restraint (assessing multiple sources regardless of their position among the search results). Consulting external resources by means of a search engine has been shown to be a successful strategy to improve evaluation of new content. Monetary bonuses increased the share of participants using these strategies compared to the control (+4%, β= 0.61 [0.15,1.07], z = 3.423, p = .004), in line with previous results (Panizza et al., 2022).
By contrast, non-monetary incentives did not significantly increase the use of these strategies (public evaluation of ratings: +1%, β = 0.13 [−0.26,0.53], z = 0.866, p = .580; providing peer performance: +0%, β = 0.06 [−0.34,0.46], z = 0.384, p = .701). The link between strategy use and performance is confirmed in our analyses (+0.54 [+0.32, +0.76] on the 1 to 6 scale, z = 4.78, p < .001).
More frequent external searches could partly explain the increase in performance in the monetary bonus condition; despite being a strong predictor of performance, however, even 85% of participants who were offered the prospect of a bonus still reported not using any of these strategies.
Methods
Data, pre-registered hypotheses, and materials are available at https://osf.io/9vc7p/.
Participants
Three-thousand and nine hundred ninety-nine U.K. residents who met the eligibility criteria in our pre-registration completed the study via Prolific. The average age was 37 (SD = 13, 6 not specified), 63% of participants were female (9 not specified), and 58.7% held a bachelor’s degree or higher.
Materials
Participants observed one out of 10 possible science-themed Facebook posts. Five posts contained scientifically valid claims, and the other five contained invalid claims. All posts came from relatively unknown sources (as pre-screened in Panizza et al., 2022), some of which were factually accurate, such as United Press International, while others had a record of fabricated news, such as sciencevibe.com. Lack of familiarity with the source allowed us to study scientific claims as they would appear through sponsored content, a widely used form of advertising on many social media platforms. Posts were selected out of a larger dataset through a pre-test survey (N = 99). This selection served to balance a number of features between valid and invalid posts: perceived bias in reporting, text comprehensibility, overall interestingness, plausibility of the claims, and familiarity with the news. This step was necessary to ensure that participants in our experiment were confronted with unfamiliar content and that, on average, they did not have a strong prior on the scientific validity of the post.
Design and procedure
Participants’ task in the experiment was to rate the scientific validity of the statements reported in the title, subtitle, and caption of the post (“How scientifically valid would you rate the information contained in the post?;” 6-point Likert scale from 1 [definitely invalid] to 6 [definitely valid]). After the rating, participants filled out a series of control questions, including self-reported search style and perception of the Facebook post.
Participants were randomly assigned to either a control group or one out of three incentive interventions. In the peer performance condition, participants read the following message prior to the task: “In a previous study, around 7 out of 10 participants were able to answer correctly. What about you?” The statistic was calculated from a previous study using the same evaluation setup. In the inform others condition, participants were informed that their evaluation “will help other participants recognize whether the post is scientifically valid or not. We will show your evaluation to other prolific participants in a subsequent study, and your response will inform their decision.” Finally, in the monetary bonus condition, participants’ participation fee was doubled if they correctly evaluated the scientific validity of the post.
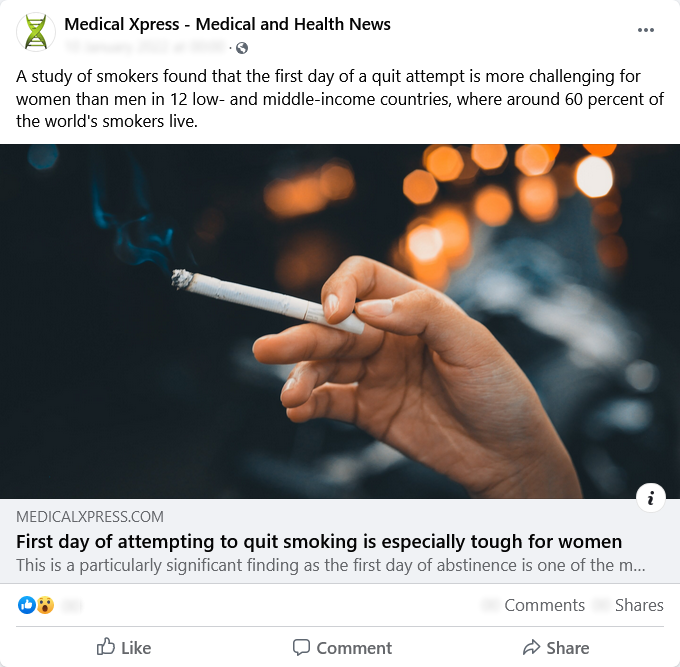
Analyses
Validity ratings were re-coded in terms of accuracy of evaluations. We measured difference in accuracy through an ordinal logistic regression and adoption of search techniques using simple logistic regressions. All p-values and confidence intervals were corrected for multiple comparisons (false discovery rate correction). Pre-registered and exploratory analyses were not reported in the main text; these, as well as any minor deviations from the original protocol, are reported in the supplementary materials.
Topics
Bibliography
Alemanno, A. (2018). How to counter fake news? A taxonomy of anti-fake-news approaches. European Journal of Risk Regulation, 9(1), 1–5. https://doi.org/10.1017/err.2018.12
Bak-Coleman, J. B., Kennedy, I., Wack, M., Beers, A., Schafer, J. S., Spiro, E. S., Starbird, K., & West, J. D. (2022). Combining interventions to reduce the spread of viral misinformation. Nature Human Behaviour, 6(10), 1372–1380. https://doi.org/10.1038/s41562-022-01388-6
Baumeister, R. F., & Leary, M. R. (2017). The need to belong: Desire for interpersonal attachments as a fundamental human motivation. In B. Laursen & R. Žukauseine (Eds.), Interpersonal Development (pp. 57–89). Routledge.
Bode, L., & Vraga, E. K. (2018). See something, say something: Correction of global health misinformation on social media. Health Communication, 33(9), 1131–1140. https://doi.org/10.1080/10410236.2017.1331312
Bruns, R., Hosangadi, D., Trotochaud, M., & Sell, K. (2021). COVID-19 vaccine misinformation and disinformation costs an estimated $50 to $300 million each day. Johns Hopkins Center for Health Security. https://centerforhealthsecurity.org/sites/default/files/2023-02/20211020-misinformation-disinformation-cost.pdf
Chapman, G. B. (2019). A decision-science approach to health-behavior change. Current Directions in Psychological Science, 28(5), 469–474. https://doi.org/10.1177/096372141985
Clark, M. (2021, August 18). Facebook releases a report on the most-viewed content in news feed. The Verge. https://www.theverge.com/2021/8/18/22630813/facebook-report-most-viewed-content-links-news-feed-transparancy
Fazio, L. (2020). Pausing to consider why a headline is true or false can help reduce the sharing of false news. Harvard Kennedy School (HKS) Misinformation Review, 1(2). https://doi.org/10.37016/mr-2020-009
Gibbons, F. X., & Buunk, B. P. (1999). Individual differences in social comparison: Development of a scale of social comparison orientation. Journal of Personality and Social Psychology, 76(1), 129–142. https://doi.org/10.1037/0022-3514.76.1.129
Goldstein, N. J., Cialdini, R. B., & Griskevicius, V. (2008). A room with a viewpoint: Using social norms to motivate environmental conservation in hotels. Journal of Consumer Research, 35(3), 472–482. https://doi.org/10.1086/586910
Grüning, D. J., Kamin, J., Panizza, F., Katsaros, M., Lorenz-Spreen, P. (2023). A framework of digital interventions for online prosocial behavior. PsyArXiv. https://doi.org/10.31234/osf.io/ysfm8
Guess, A. M., Lerner, M., Lyons, B., Montgomery, J. M., Nyhan, B., Reifler, J., & Sircar, N. (2020). A digital media literacy intervention increases discernment between mainstream and false news in the United States and India. Proceedings of the National Academy of Sciences, 117(27), 15536–15545. https://doi.org/10.1073/pnas.1920498117
Jarvis, S., Deschenes, O., & Jha, A. (2022). The private and external costs of Germany’s nuclear phase-out. Journal of the European Economic Association, 20(3), 1311–1346. https://doi.org/10.1093/jeea/jvac007
Kozyreva, A., Herzog, S., Lewandowsky, S., Hertwig, R., Lorenz-Spreen, P., Leiser, M., & Reifler, J. (2022). Free speech vs. harmful misinformation: Moral dilemmas in online content moderation. PsyArXiv. https://doi.org/10.31234/osf.io/2pc3a
Lewandowsky, S., Ecker, U. K., Seifert, C. M., Schwarz, N., & Cook, J. (2012). Misinformation and its correction: Continued influence and successful debiasing. Psychological Science in the Public Interest, 13(3), 106–131. https://doi.org/10.1177/1529100612451018
Motta, M., & Callaghan, T. (2020). The pervasiveness and policy consequences of medical folk wisdom in the U.S. Scientific Reports, 10, 10722. https://doi.org/10.1038/s41598-020-67744-6
Panizza, F., Ronzani, P., Mattavelli, S., Morisseau, T., and Martini, C. (2023). How do online users respond to crowdsourced fact-checking? Humanities and Social Sciences Communications, 10, 867. https://doi.org/10.1057/s41599-023-02329-y
Panizza, F., Ronzani, P., Martini, C., Mattavelli, S., Morisseau, T., and Motterlini, M. (2022). Lateral reading and monetary incentives to spot disinformation about science. Scientific Reports, 12, 5678. https://doi.org/10.1038/s41598-022-09168-y
Pennycook, G., & Rand, D. G. (2021). The psychology of fake news. Trends in Cognitive Sciences, 25(5), 388–402. https://doi.org/10.1016/j.tics.2021.02.007
Pennycook, G., Epstein, Z., Mosleh, M., Arechar, A. A., Eckles, D., & Rand, D. G. (2021). Shifting attention to accuracy can reduce misinformation online. Nature, 592(7855), 590–595. https://doi.org/10.1038/s41586-021-03344-2
R Core Team (2018). R: A language and environment for statistical computing. R Foundation for Statistical Computing. http://www.R-project.org/
Rathje, S., Roozenbeek, J., Van Bavel, J. J. & van der Linden, S. (2023). Accuracy and social motivations shape judgements of (mis)information. Nature Human Behavior, 7, 892–903. https://doi.org/10.1038/s41562-023-01540-w
Rogstadius, J., Kostakos, V., Kittur, A., Smus, B., Laredo, J., & Vukovic, M. (2011). An assessment of intrinsic and extrinsic motivation on task performance in crowdsourcing markets. Proceedings of the International AAAI Conference on Web and Social Media, 5(1), 321–328. https://doi.org/10.1609/icwsm.v5i1.14105
Roozenbeek, J., Suiter, J., & Culloty, E. (2022). Countering misinformation: Evidence, knowledge gaps, and implications of current interventions. PsyArXiv. https://doi.org/10.31234/osf.io/b52um
Roth, Y., & Pickles, N. (2020, May 11). Updating our approach to misleading information. Twitter Blog. https://blog.twitter.com/en_us/topics/product/2020/updating-our-approach-to-misleading-information
Rushton, J. P., Chrisjohn, R. D., & Fekken, G. C. (1981). The altruistic personality and the self-report altruism scale. Personality and Individual Differences, 2(4), 293–302. https://doi.org/10.1016/0191-8869(81)90084-2
Shaw, A. D., Horton, J. J., & Chen, D. L. (2011). Designing incentives for inexpert human raters. In CSCW ’11: Proceedings of the ACM 2011 conference on computer supported cooperative work (pp. 275–284). Association for Computing Machinery. https://doi.org/10.1145/1958824.1958865
Scholtz, B., & Mloza-Banda, C. (2019). Applying theories for using non-monetary incentives for citizens to participate in crowdsensing projects. South African Computer Journal, 31(2), 99–116. https://hdl.handle.net/10520/EJC-1d75c3b2b5
Sperber, D., Clément, F., Heintz, C., Mascaro, O., Mercier, H., Origgi, G., & Wilson, D. (2010). Epistemic vigilance. Mind & Language, 25(4), 359–393. https://doi.org/10.1111/j.1468-0017.2010.01394.x
Su, S. (2017, April 25). New test with related articles. Facebook Newsroom. https://www.newsroom.fb.com/news/2017/04/news-feed-fyi-new-test-with-related-articles
Swire-Thompson, B., Miklaucic, N., Wihbey, J. P., Lazer, D., & DeGutis, J. (2022). The backfire effect after correcting misinformation is strongly associated with reliability. Journal of Experimental Psychology: General, 151(7), 1655–1665. https://doi.org/10.1037/xge0001131
Tang, W., Yin, M., & Ho, C. J. (2019). Leveraging peer communication to enhance crowdsourcing. In WWW ’19: The world wide web conference (pp. 1794–1805). Association for Computing Machinery. https://doi.org/10.1145/3308558.3313554
Wingfield, N., Isaac, M., & Benner, K. (2016, November 16). Google and Facebook take aim at fake news sites. The New York Times. http://nyti.ms/2ezMPpS
Funding
This project has received funding from the European Union’s Horizon 2020 research and innovation programme under grant agreement No. 870883–PEriTiA. The information and opinions are those of the authors and do not necessarily reflect the opinion of the European Commission.
Competing Interests
The authors have no conflicts of interest to declare.
Ethics
All participants gave their written informed consent for participating in the experiment. The experimental protocols were approved by the Research Ethics Committee (CER) at the University Paris Cité (IRB No. 00012021-05), and all research was performed in accordance with the relevant guidelines and regulations.
Copyright
This is an open access article distributed under the terms of the Creative Commons Attribution License, which permits unrestricted use, distribution, and reproduction in any medium, provided that the original author and source are properly credited.
Data Availability
All materials needed to replicate this study are available via the Harvard Dataverse: https://doi.org/10.7910/DVN/M5EBXL