Peer Reviewed
“Fact-checking” fact checkers: A data-driven approach
Article Metrics
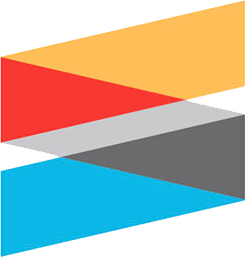
1
CrossRef Citations
Altmetric Score
PDF Downloads
Page Views
This study examined four fact checkers (Snopes, PolitiFact, Logically, and the Australian Associated Press FactCheck) using a data-driven approach. First, we scraped 22,349 fact-checking articles from Snopes and PolitiFact and compared their results and agreement on verdicts. Generally, the two fact checkers agreed with each other, with only one conflicting verdict among 749 matching claims after adjusting minor rating differences. Next, we assessed 1,820 fact-checking articles from Logically and the Australian Associated Press FactCheck and highlighted the differences in their fact-checking behaviors. Major events like the COVID-19 pandemic and the presidential election drove increased the frequency of fact-checking, with notable variations in ratings and authors across fact checkers.
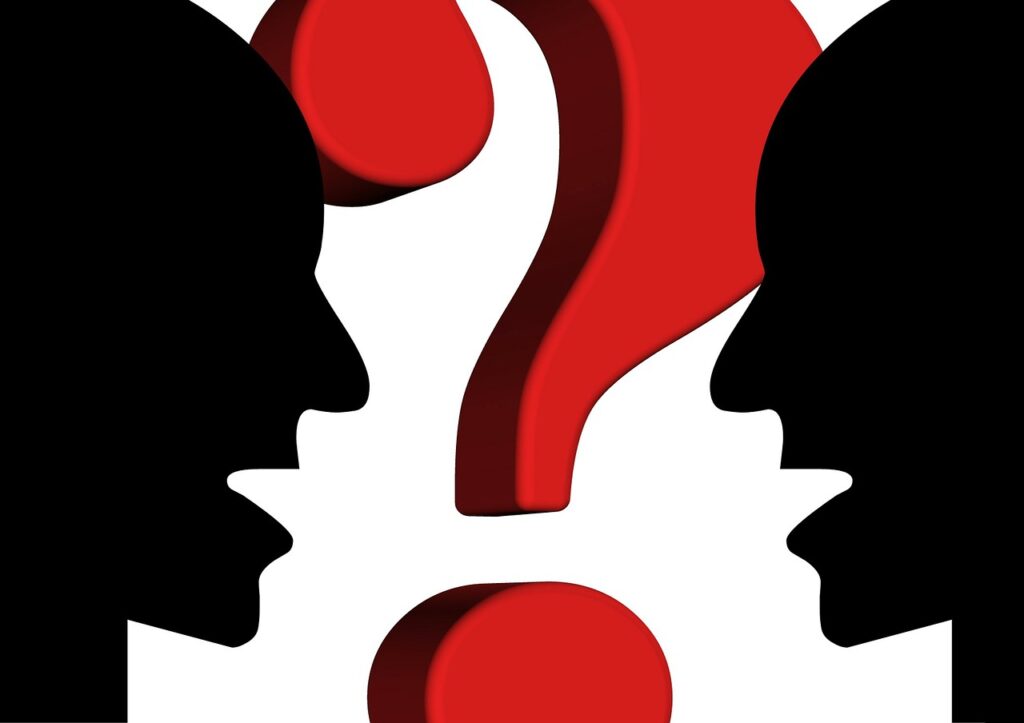
Research Questions
- Do different fact checkers exhibit similar or distinct behaviors with respect to the frequency of fact-checking, types of claims selected for fact-checking, and the individuals responsible for conducting fact checks?
- What percentage of statements debunked by fact checkers are overlapping (i.e., matching claims) across multiple fact checkers?
- Is there a reasonable level of agreement among fact checkers in their ratings of matching claims that have been debunked by multiple fact checkers?
Essay Summary
- This study examined four fact-checking organizations (so-called fact checkers)—Snopes, PolitiFact, Logically, and the Australian Associated Press FactCheck (AAP)—by analyzing their fact-checking articles from January 1, 2016, to August 31, 2022.
- Results showed an increased number of fact-checking articles during major events, such as the COVID-19 pandemic and the U.S. presidential election, suggesting their influence on fact-checking activities.
- Furthermore, variations were found in ratings and authors of fact-checking articles among fact checkers. While PolitiFact and AAP primarily focused on verifying suspicious claims, Snopes and Logically emphasized affirming truthful claims. The distribution of the number of fact-checking articles per author also differed across fact checkers, likely reflecting variations in their operational scope and scale.
- Critically, we assessed the degree of consensus between Snopes and PolitiFact’s verdicts on matching claims (i.e., the same [mis]information with the wording of the claim being slightly different). Out of 11,639 and 10,710 fact-checking articles from Snopes and PolitiFact, respectively, 6.5% (749) were matching claims, of which 521 (69.6%) received identical ratings, while the remaining 228 (30.4%) had diverging ratings.
- Rating discrepancies are attributed to various systematic factors: 1) differences in the granularity of verdict ratings, 2) differences in focus between two fact checkers, 3) similar claims but subtle differences in the key information to fact-check, and 4) different timing in fact-checking. After adjusting these systematic discrepancies, we found only one case out of 749 matching claims with conflicting verdict ratings.
- Consequently, our findings show high agreement between Snopes and PolitiFact regarding their fact-checking verdicts after adjusting minor rating differences.
Implications
Misinformation, in a broad sense, refers to information that is presented as factually accurate but includes false or misleading content, irrespective of the intentions of the presenter (van der Linden, 2022). The utilization of social media as a primary source of news consumption has, to some extent, contributed to the dissemination of misinformation (Wu et al., 2019). According to a Pew Research survey in 2021, about half of Americans get their news on social media (Walker & Matsa, 2021). Because online users can upload and share news without verifications, information, especially misinformation, diffuses quickly on social media (Vosoughi et al., 2018). The spread of misinformation can have severe negative impacts on individuals and society, such as COVID-19 vaccination hesitancy (Garett & Young, 2021) or election results manipulation (Allcott & Gentzkow, 2017). Furthermore, individuals characterized as ‘lazy’ are not the only ones susceptible to misinformation (Pennycook & Rand, 2019); specific types of misinformation, such as associatively inferred misinformation, can make individuals with higher cognitive ability levels even more susceptible (Lee et al., 2020, 2023; Xiong et al., 2023).
Fact-checking organizations, often known as fact checkers, are instrumental in identifying and debunking misinformation. Fact-checking has traditionally been performed by human professionals, either individuals or teams, who manually review and analyze claims using various resources and methods to affirm the information’s accuracy (Amazeen, 2015). Although these human fact checkers can apply considerable expertise and critical thinking, their work can be time-consuming and costly. In response, automated fact-checking techniques have emerged to debunk misinformation on a large scale (Cui et al., 2020; D’Ulizia et al., 2021; Shu et al., 2019; Wu et al., 2019; Zhang & Ghorbani, 2020; Zhou & Zafarani, 2020). These misinformation detection algorithms employ advanced techniques such as natural language processing, machine learning, and deep learning to detect patterns and correlations in large datasets. Despite substantial advancements, these automated techniques face challenges. The sheer volume of data and rapid spread of false claims make timely detection difficult, and the accuracy and effectiveness of these algorithms are limited by the need for high-quality training datasets and the potential for bias (Wu et al., 2019). Furthermore, new types of misinformation, such as deep fakes, remain challenging to detect (Rana et al., 2022), requiring additional human expertise and intervention.
In practice, a few initiatives employing manual fact-checking have been launched and are playing a vital role in combating misinformation. However, these can invite criticism due to the subjective choice of claims to verify and the inconsistency in the evaluation process (Nieminen & Rapeli, 2019). Specifically, concerns have been raised about the potential uncertainty that may arise among individuals if different fact checkers provide conflicting assessments for the same claim (Marietta et al., 2015). Previous studies evaluated the performance of fact checkers and showed conflicting results. Amazeen’s (2015, 2016) study demonstrated consistency in the verdicts of various fact checkers using manually gathered samples of political ads from the 2008 and 2012 U.S. presidential elections. In contrast, Marietta et al. (2015) found significant discrepancies among three fact checkers—PolitiFact, The Fact Checker,1The Fact Checker of The Washington Post: https://www.washingtonpost.com/news/fact-checker/ and FactCheck.org2https://www.factcheck.org/—in their assessments of the statements and conclusions on the existence of climate change, the influence of racism, and the consequences of the national debt. Notably, it reported that the fact checkers agreed regarding the existence of climate change, while they disagreed on the issue of the national debt. Additionally, only PolitiFact assessed the influence of racism. The findings indicate that individuals seeking to discern the veracity of disputed claims may not perceive fact-checking to be particularly efficacious, especially in the context of polarized political topics. Lim (2018) also manually collected samples of 2016 U.S. presidential candidates’ statements from two different fact checkers (i.e., The Fact Checker and PolitiFact) and evaluated their performance. This study found that only 10% of statements were fact-checked by both fact checkers, and the fact checkers agreed on obvious truths and falsehoods but had lower agreement rates for statements in the ambiguous rating range. The findings indicate that fact-checking is challenging, and disagreements are common, particularly when politicians use ambiguous language.
It is important to acknowledge that previous studies have used different samples from fact checkers and different methods, which could explain their conflicting conclusions (Nieminen & Rapeli, 2019). The findings were based on a small number of manually collected claims on specific topics (e.g., presidential candidates, climate change, debt) during specific periods (e.g., election periods), using a limited set of keywords. Furthermore, they hand-coded to find statements that were fact-checked by multiple fact checkers. Because such a manual process is time-consuming and error-prone, previous works have not evaluated the fact checkers’ performance comprehensively, which also could have led to the conflicting results across the literature.
To address the aforementioned limitations, in this work, we propose an automatic method to collect fact checkers’ data across topics and periods, and automatic techniques to find matching claims across fact checkers. During our analysis, we selected four fact checkers (see Appendix A): Snopes, PolitiFact, Logically, and the Australian Associated Press FactCheck (AAP), each of which provided a summarized claim about the (mis)information being evaluated. While examining the same (mis)information, these fact checkers may use distinct phrasing to depict the claim. Therefore, we have devised an automated technique to identify corresponding claims that pertain to the same (mis)information that is being fact-checked (even though their wording could be slightly different) and named them as matching claims. Furthermore, some fact checkers use a different rating system for their conclusions, and the agreement rate among matching claims of fact checkers depends on how the rating system was converted for the agreement comparison (Lim, 2018; Marietta et al., 2015). We only chose fact checkers who had comparable rating systems with only minor conversions.
The low percentage (i.e., around 6.5%) of matching claims between two major fact checkers, Snopes and PolitiFact, from 2016 to 2022 is an intriguing finding. This could suggest that fact-checking is a complex and multifaceted process that involves numerous variables, including the nature of the claims being fact-checked and the fact checkers’ methods and priorities. In contrast to traditional news articles that typically prioritize exclusive reports, fact-checking articles gain value from the confirmation of (in)accurate information through multiple fact checks by different organizations. Thus, it is crucial for fact checkers to collaborate and cross-check their findings to provide the most reliable information to the public. The low percentage of matching claims may also indicate that the fact-checking landscape is diverse, and that fact checkers have unique ways of selecting and verifying claims, which can impact agenda-setting. Future research could investigate these variations and their potential impact on public trust in fact checkers.
The high level of agreement, with only one contradicting case, between Snopes and PolitiFact in their fact-checking conclusions is critical. This suggests that the two fact checkers have established consistent and reliable fact-checking practices. Such consistency is important for several reasons. First, it enhances the credibility of fact checkers in the eyes of the public. When multiple fact-checking organizations consistently agree on the accuracy of a statement, the public is more likely to trust their assessments. Furthermore, the consistency of fact-checking among major organizations is crucial for mitigating misinformation online, especially as the evaluations of these organizations are increasingly being used by social media outlets such as Facebook and X (Allcott et al., 2019; Ananny, 2018).
Previous literature has conducted meta-analyses to investigate the effectiveness of fact-checking in correcting misinformation (Walter & Murphy, 2018; Walter & Tukachinsky, 2020). These studies have consistently identified the timing of corrections as a significant factor influencing the effectiveness of fact-checking. However, the exact timing that yields optimal results remains somewhat controversial in prior research (Ecker et al., 2022). For instance, research conducted by Brashier et al. (2021) and Walter & Murphy (2018) suggests that debunking, which involves fact-checking after the exposure of misinformation, tends to be more effective than forewarning or prebunking. In contrast, Jolley and Douglas (2017) found that prebunking, which involves addressing misinformation prior to exposure, was more successful in correcting anti-vaccine conspiracy theories compared to debunking. Additionally, the effectiveness of corrections tends to diminish over time when there is a significant delay between the exposure to misinformation and the subsequent correction (Walter & Murphy, 2018; Walter & Tukachinsky, 2020). Our findings revealed a higher number of fact-checking articles during major events, such as the COVID-19 pandemic and the U.S. presidential election, where misinformation tends to spread widely (Cinelli et al., 2020; Grinberg et al., 2019; Sharma et al., 2022). Combined with previous research, this suggests that actively countering misinformation during these critical events through the incorporation of fact-checking articles can be beneficial in correcting misinformation on social media. Moreover, when multiple fact checkers consistently convey the same message to debunk the same misinformation, it enhances their credibility among the public (Amazeen, 2015; 2016). For example, social media platforms could consider showing such fact-checking consistency across different fact checkers. Therefore, the findings of this study can inform and improve the fact-checking practices of social media platforms, ultimately contributing to the promotion of truth and the prevention of the spread of misinformation on social media.
Findings
Finding 1: Major events like the COVID-19 pandemic declaration and the 2020 U.S. election surge fact-checking activities.
Our analysis reveals that fact-checking activity notably surged in response to major events from May 2019 to August 2022. Figure 1 presents the monthly article count of the four fact checkers, showing a peak around the 2020 U.S. election for the three U.S.-based fact checkers (i.e., Snopes, PolitiFact, and Logically), but not Australia-based (i.e., AAP). Following the election, the rampant spread of unverified 2020 election fraud claims significantly undermined public trust, culminating in the U.S. Capitol breach (Abilov et al., 2021). Consequently, increased fact-checking is evident. Another local peak in fact-checking coincided with the World Health Organization’s (WHO) declaration of the COVID-19 pandemic in March 2020 (see Figure 1). Given Australia’s lower COVID-19 contraction rates compared to the United States (World Health Organization, 2020), it suggests that major health events could also amplify fact-checking efforts for U.S.-based fact checkers.
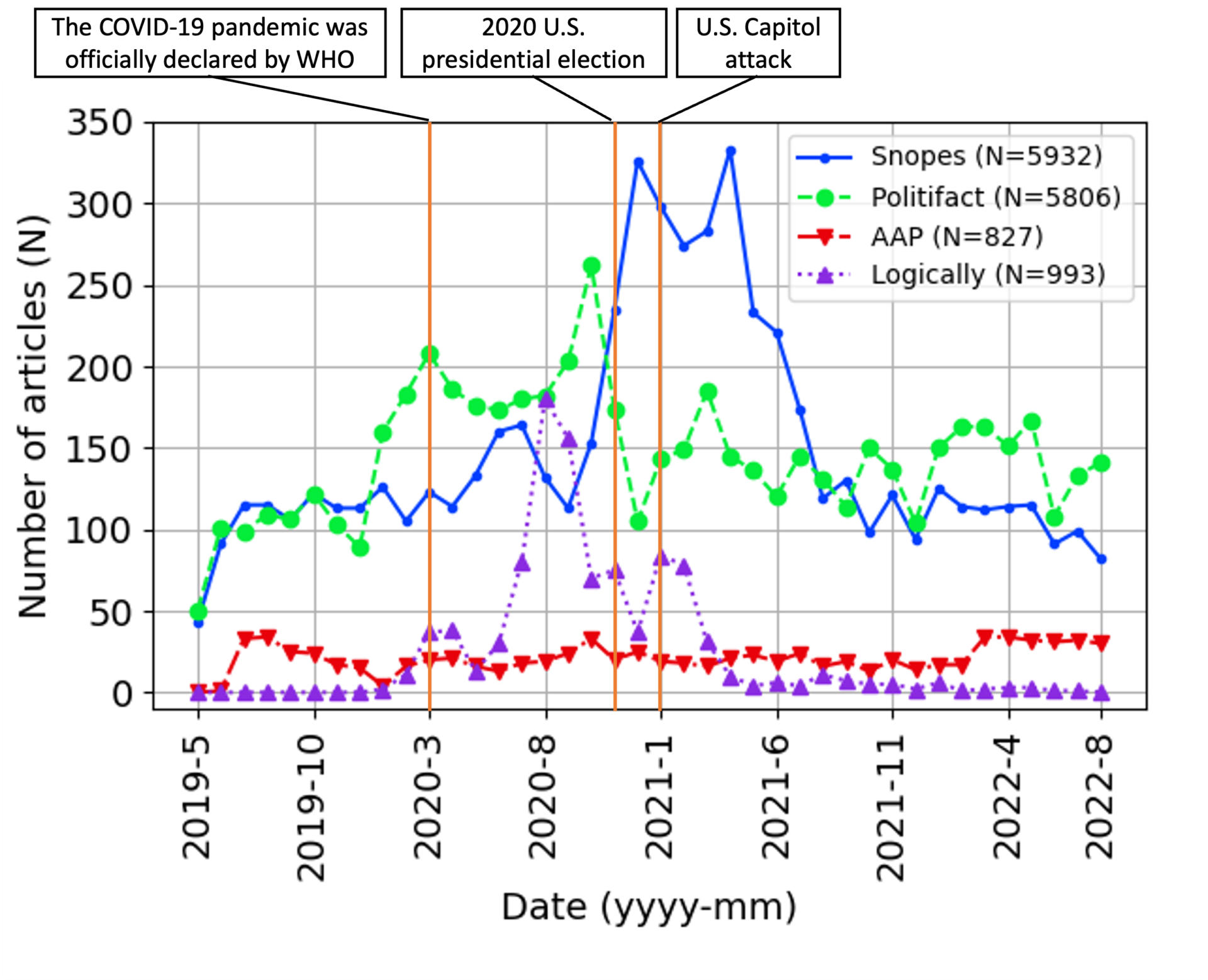
Finding 2: While fact checkers understandably prioritize the verification of suspicious claims, some also prioritize the affirmation of truth claims.
In Figure 2, the total number of fact-checking articles is displayed for each rating. As indicated, most of the fact checks resulted in fake (i.e., False or Mostly False). This suggests that fact checkers have primarily concentrated on scrutinizing dubious claims, leading to an abundance of fake claims being fact-checked. The distribution of real claims (i.e., Mostly True and True) varied among the four fact checkers, revealing an intriguing observation. Specifically, Snopes exhibited a higher proportion of real claims, with 28.65% of its fact-checking articles falling under this category. In contrast, only 10.95% of PolitiFact’s fact-checking articles were classified as real claims. The existence of such a gap suggests that various fact checkers may place varying degrees of emphasis on verifying truth claims.
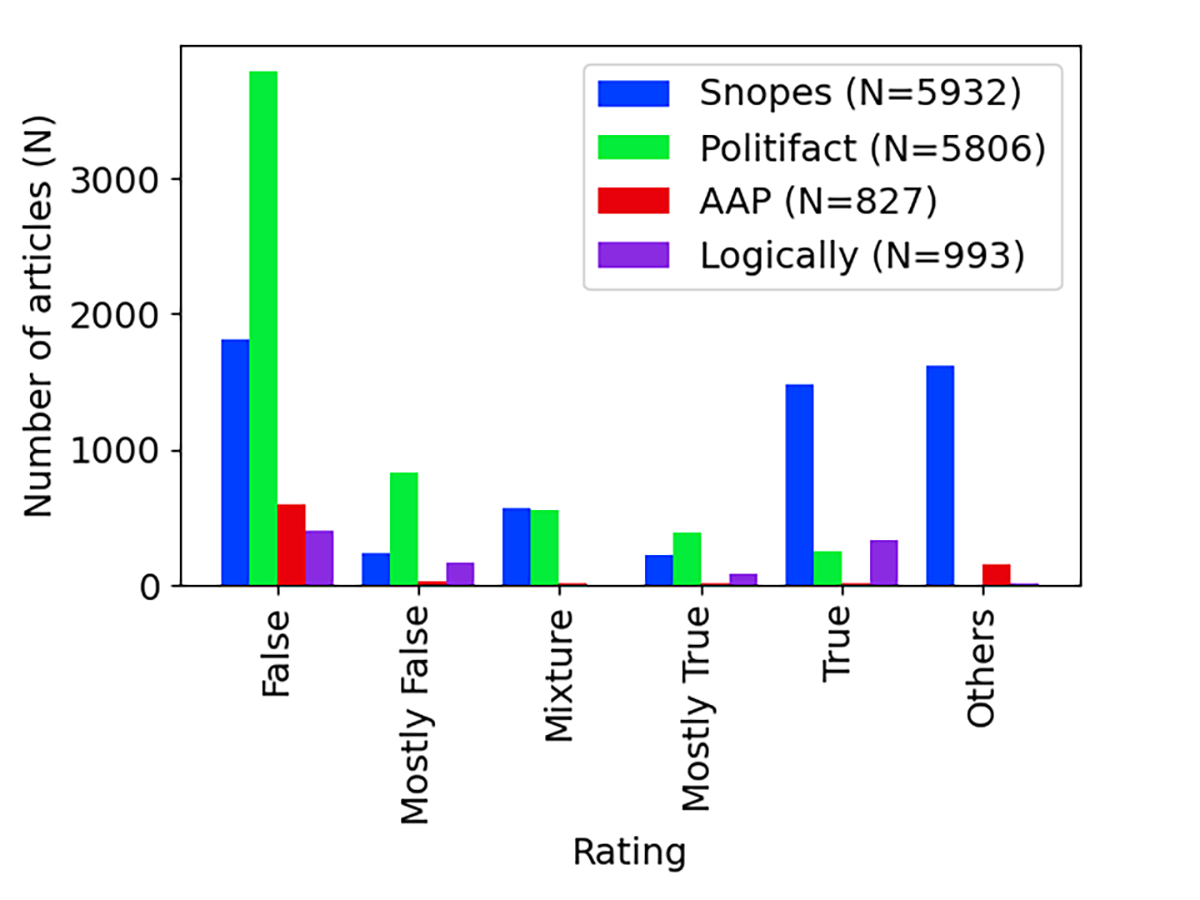
Finding 3: Variations in the distribution of fact-checking articles among authors across different platforms were noted.
Fact-checking article distributions varied among authors across different fact checkers. The most prolific authors of Snopes and PolitiFact wrote over 20% of the articles, whereas Logically’s top contributor wrote only 9%, displaying more balanced authorship (see Figure 3). The number of authors per year has remained steady for Snopes but has dwindled since 2020 for PolitiFact and Logically (see Figure 4). During the study period, Snopes had 13 authors, while PolitiFact had 177, reflecting variations in their fact-checking operations’ scope and scale. Our further analysis of rating preference (or bias) suggested varied expertise among Snopes authors (see Appendix B).
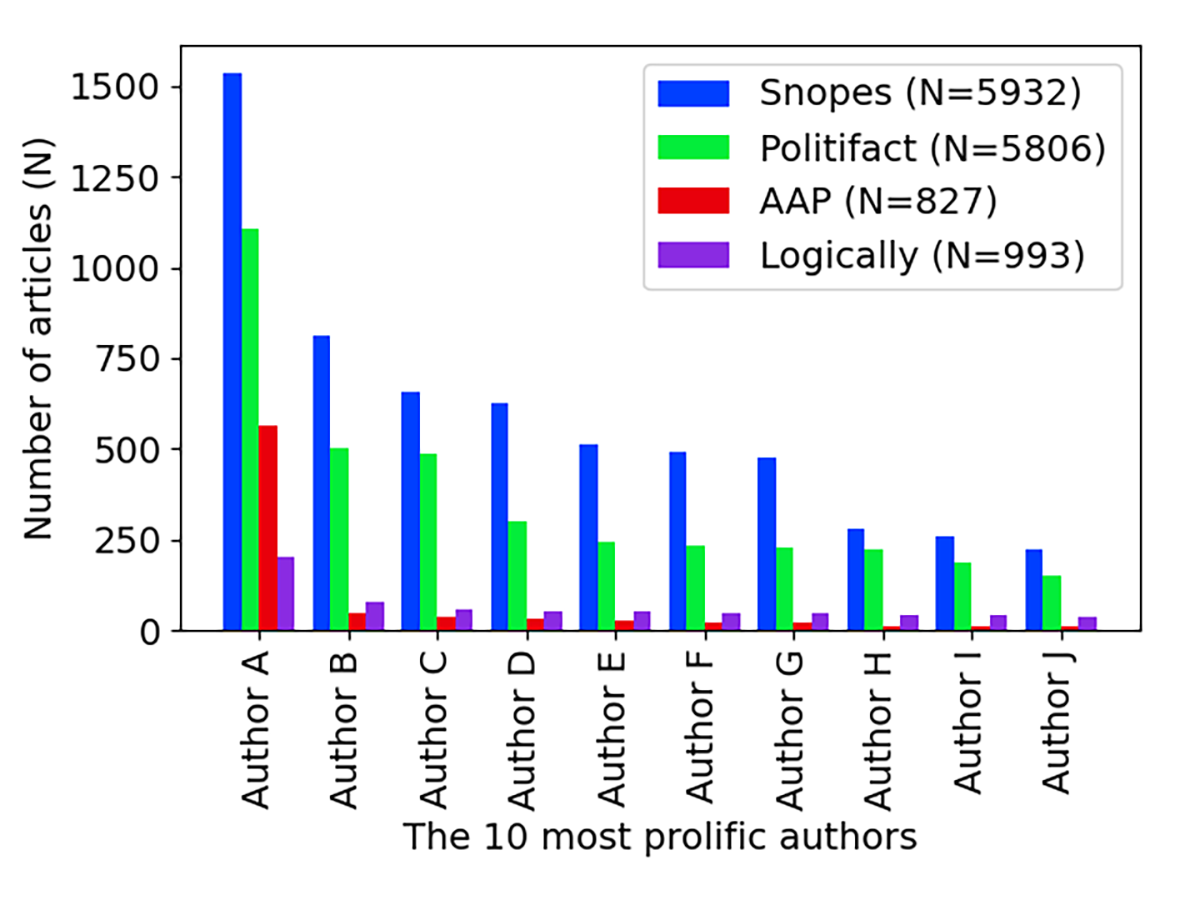
Finding 4: We found only one case of conflicting fact-check verdicts between Snopes and PolitiFact when minor rating differences were adjusted. Therefore, our finding suggests a high level of agreement between Snopes and PolitiFact in their fact-checking verdicts during the studied period.
We examined the consistency of fact-check ratings for matching claims (see Appendix C). Since Snopes and PolitiFact have a similar number of fact-checking articles, while AAP and Logically have substantially fewer articles, we focused on analyzing the matching claims between Snopes and PolitiFact (see Appendix D for the matching claims results for the four fact checkers). To compare the ratings, we redefined the comparison period to cover the matching claims published between January 1, 2016, and August 31, 2022. During this period, Snopes and PolitiFact published 11,639 and 10,710 fact-checking articles, respectively. Note that the original rating level (e.g., False, Mostly False, Mixture, Mostly True, True, etc.) was used for the rating level comparison, whereas False and Mostly False were combined as Fake, and True and Mostly True were combined as Real for the veracity level comparison.
Among Snopes’ 11,639 claims, 749 (6.4%) claims had at least one matching claim in PolitiFact (see Table 1). Among these, 521 (69.6%) had consistent ratings, and 556 (74.2%) had consistent veracity between Snopes and PolitiFact. Similarly, among PolitiFact’s 10,710 claims, 722 (6.7%) claims had at least one matching claim in Snopes. Among these, 502 (69.5%) had consistent ratings, and 538 (74.5%) had consistent veracity with Snopes.
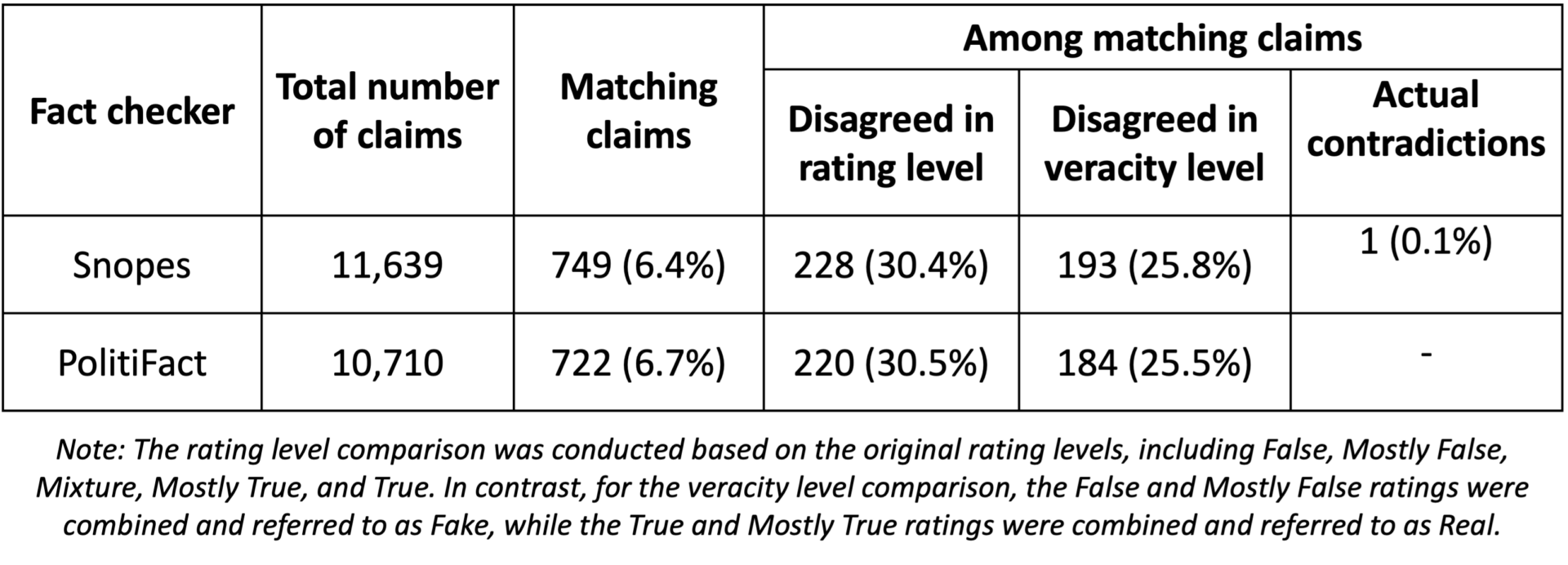
Our analysis revealed about 220 claims that were debunked by both fact checkers but had disagreeing ratings. To gain further insight into the reasons behind this disagreement, we conducted a manual analysis of these discrepant cases. We conducted an analysis of 228 (30.4%) fact-checked claims by Snopes that are matched with fact-checked claims by PolitiFact but have different ratings. Table 2 presents the reasons for the rating discrepancies.
First, we found that 98 of the cases that disagreed were caused by the difference in rating systems between Snopes and PolitiFact. PolitiFact uses six rating scales, while Snopes has more fine-grained ratings, such as Miscaptioned, Scam, Satire, etc., in addition to the five-point rating scales from True to False.
Second, 59 of the cases that disagreed showed subtle discrepancies in ratings due to differences in focus. For example, Snopes rated the statement “In 2022, members of Congress collectively voted to award themselves a 21 percent pay raise” as False, while PolitiFact rated the statement “Members of Congress gave themselves a 21% pay raise” as Mostly False. Despite both fact checkers agreeing that the statement claiming a 21% pay raise for members of Congress in 2022 was false due to salaries remaining unchanged since 2009, PolitiFact labeled it as Mostly False based on the increase in lawmakers’ office budgets in the 2022 spending bill.
Third, 57 cases involved similar but not identical claims, where the key information of the suspicious claim differed. For instance, Snopes rated the claim “Five people died during the Jan. 6, 2021, U.S. Capitol riot” as True, while PolitiFact rated the claim “Only one person died on that day during the Jan. 6 U.S. Capitol riot” as False. As the topics of the claims were almost the same, the algorithm identified these two as matching claims. However, the detailed numbers (five vs. only one) were different, resulting in disagreement between the fact checkers’ conclusions.
Fourth, in 13 cases, Snopes could not debunk the claims while PolitiFact debunked them. For instance, Snopes debunked the claim that “DMX took the COVID-19 vaccine days before he suffered a heart attack” on April 9, 2021, and labeled it as Unproven. However, PolitiFact debunked the same claim three days later (i.e., April 12, 2021) and labeled it as False. In their article, PolitiFact cited Snopes’ fact check and mentioned that it was unproven at that time. This suggests that the timing of fact-checking can sometimes be a source of rating discrepancy between fact checkers.
Finally, there was only one case in which fact checkers arrived at conflicting conclusions for matching claims, suggesting a high level of agreement between Snopes and PolitiFact in their fact-checking verdicts during the studied period. This contradicting case is listed in Table 3. The primary source of contradiction stems from divergent contextual interpretations by Snopes and PolitiFact. To elaborate, both fact checkers examined a statement from Carson’s 2014 column, which stated, “Anyone caught involved in voter fraud should be immediately deported and have his citizenship revoked.” Snopes interpreted “Anyone” to pertain specifically to (illegal) immigrants, rating the claim as Mostly True. Conversely, PolitiFact argued that “Anyone” could encompass any American, leading to a rating of Mostly False.
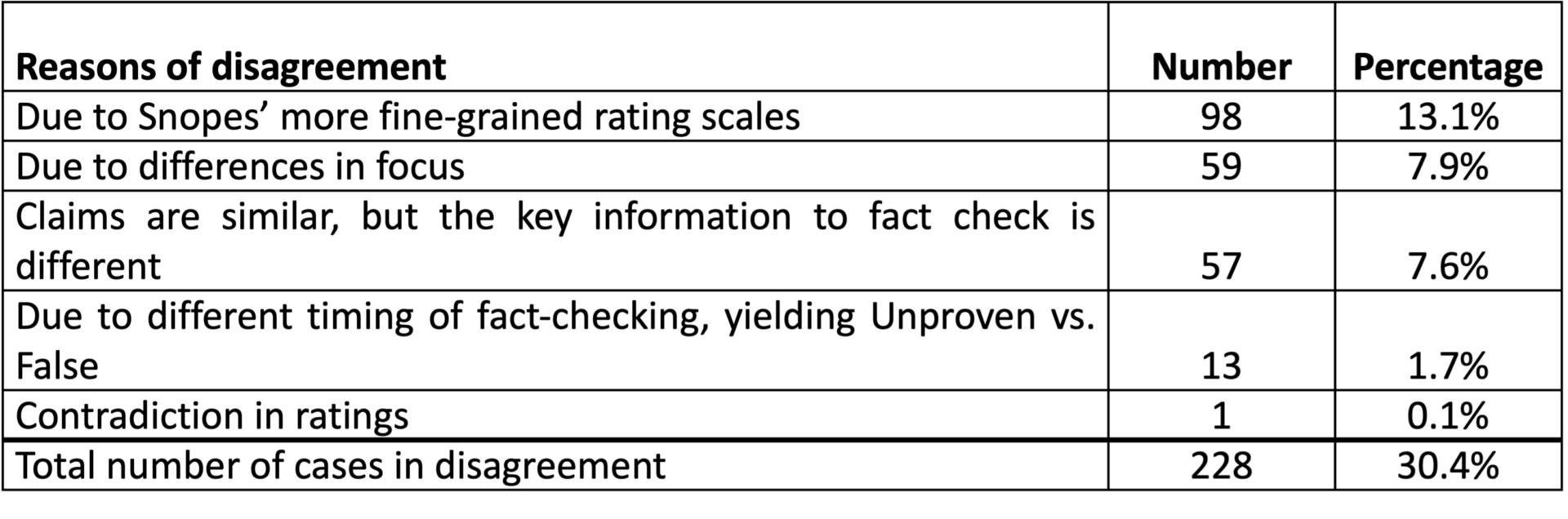
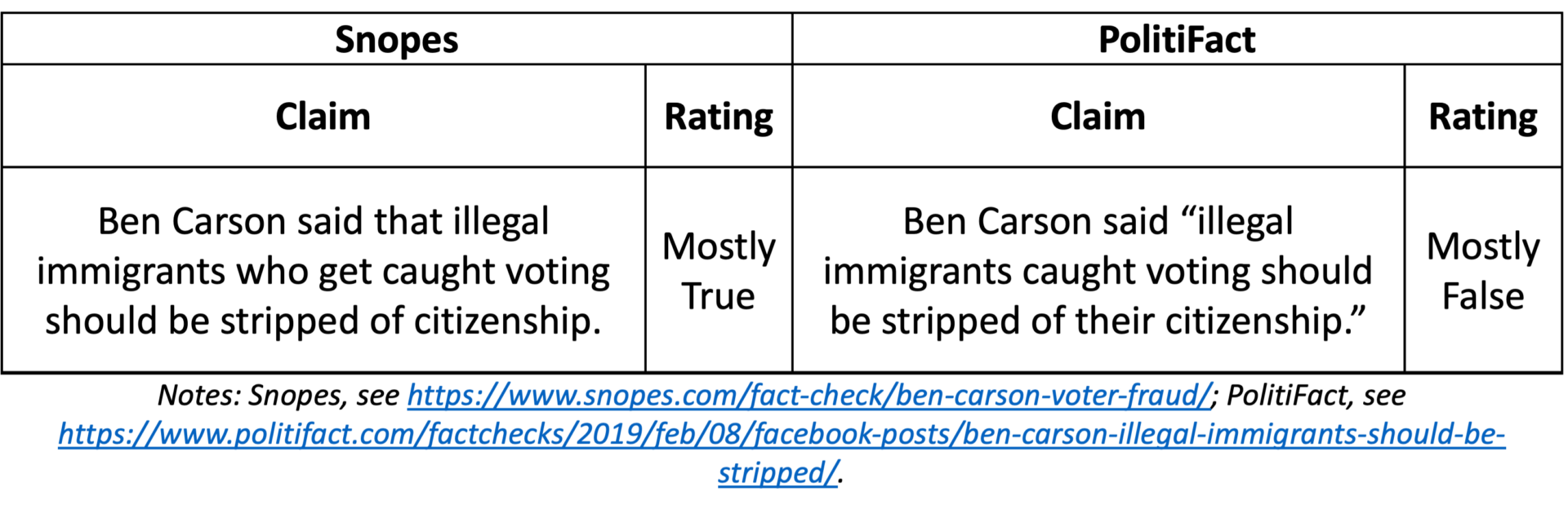
Methods
To create the dataset, we developed web crawlers in Python and gathered fact-checking articles from the inception of each fact checker until August 31, 2022. The four major fact-checking organizations are Snopes, PolitiFact, Logically, and AAP Fact Check. The selection was guided by specific criteria, including similar fact-checking domains and rating structures. These fact checkers represent fact-checking methods from human-based analysis to AI-driven approaches and fact-checking regions from the U.S.-based to Australia-based. Table 4 summarizes the key variables in the dataset. Appendix A includes further details about the four fact checkers, selection criteria, and the authorship and volumes of the fact-check articles.
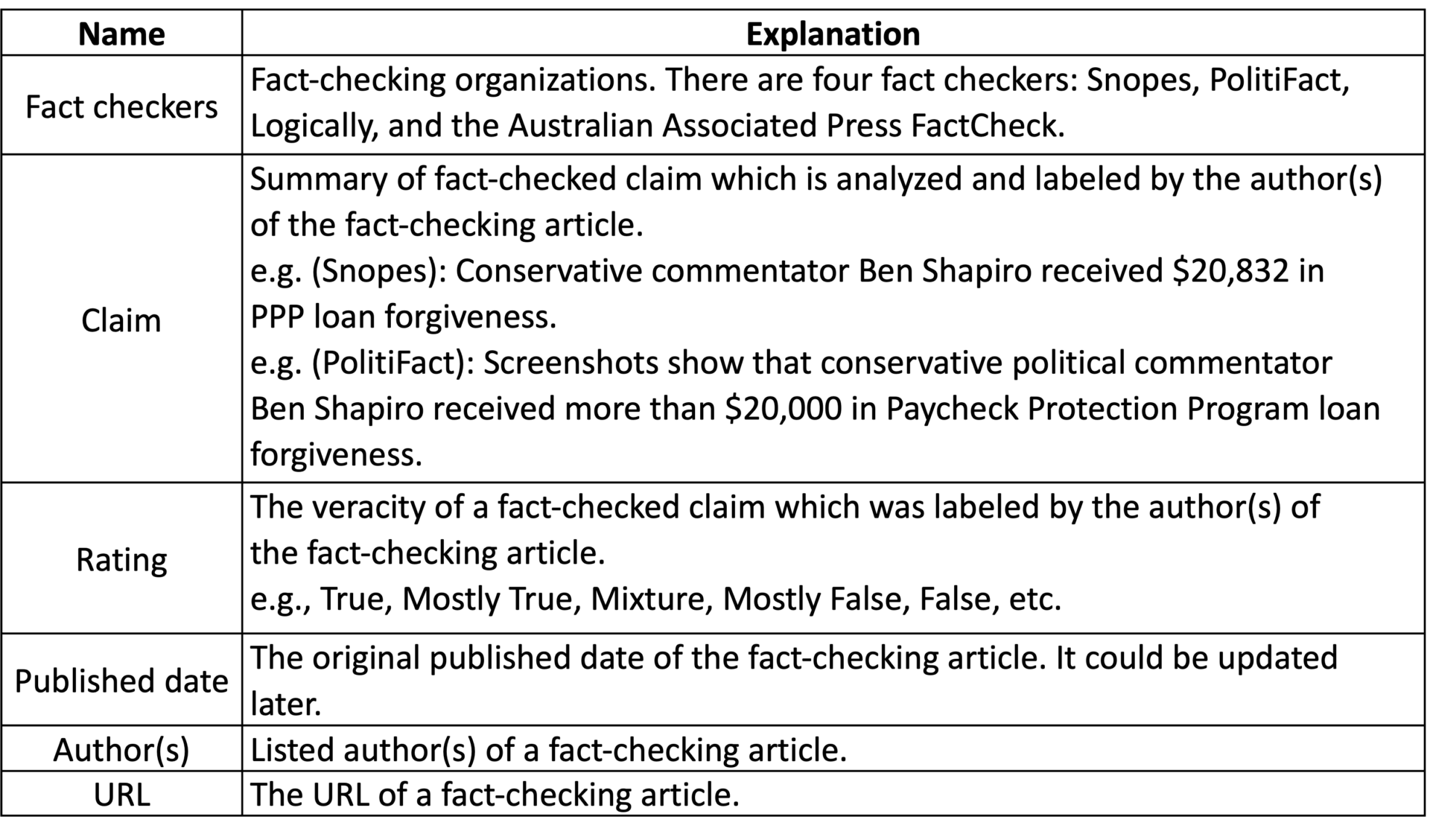
To evaluate the accuracy of suspicious claims, Snopes employs a five-point scale ranging from True to False. Recognizing the complexity of some claims, Snopes also uses additional categories of ratings such as outdated, miscaptioned, satire, among others. The complete list of Snopes fact-check ratings with their definitions is available on their website.4See, https://www.snopes.com/fact-check-ratings/ PolitiFact uses the Truth-O-Meter to assess the accuracy of a statement, which employs a rating system consisting of six levels ranging from True to False to Pants on Fire, to indicate the degree of truthfulness of the claim. To facilitate comparison with Snopes, we combined False and Pants on Fire ratings into a single category, as done by prior research (Lim, 2018; Marietta et al., 2015). The AAP employs a rating system that includes True, Mostly True, Mixture, Mostly False, False, Misleading, and Unproven, while the Logically uses True, Partly True, Misleading, and False.
Each fact checker provides a summary of the claim that was debunked in their fact-checking articles. For instance, Snopes includes a distinct “claim” section that outlines the exact sentence or statement that was refuted in the article. PolitiFact, on the other hand, incorporates the debunked statement in the title of each fact-checking article. We considered these summaries as the suspicious claims that were refuted by the fact-checking articles and employed them to evaluate the similarity of fact-checked claims across different fact checkers. When two fact-checked claims essentially conveyed the same idea, despite differences in wording, we recognized these as matching claims.
To identify matching claims across different fact checkers (see Appendix C for a comprehensive description of the method), we built on prior work by Lim (2018), which manually compared statements and ratings from PolitiFact and the Washington Post Fact Checker. To automatically identify matching claims, we used word embeddings and pre-trained models on the manually labeled data from Lim (2018). We utilized different sentence embedding techniques, such as the Count vectorizer (i.e., Bag-of-Words; Qader et al., 2019), Term Frequency-Inverse Document Frequency (TF-IDF) vectorizer (Kaur et al., 2020), and sentence BERT (Reimers & Gurevych, 2019), and varied the thresholds (in 0.05 intervals, ranging from 0 to 1) to identify the optimal approach for determining matching claims. For the distance metric, we used cosine similarity. The TF-IDF method provided the best performance with a 0.5 threshold (see Figure A2). Therefore, we applied this approach to identify matching claims between different fact checkers.
Lastly, we assessed the consistency of ratings for matching claims, focusing on Snopes and PolitiFact due to their larger dataset. We defined the comparison period for matching claims published between January 1, 2016, and August 31, 2022, during which Snopes and PolitiFact published 11,639 and 10,710 fact-checking articles, respectively. We used two different rating levels for this analysis. Firstly, we preserved the original rating system of each fact checker (e.g., False, Mostly False, Mixture, Mostly True, True, etc.) for the rating level comparison. Secondly, we combined True and Mostly True as Real and False and Mostly False as Fake to compare whether the rating for the matching claims agreed when making the real vs. fake decision (i.e., veracity level). After the automatic analysis of rating consensus, we manually examined the disagreed cases to identify the reasons for the different ratings assigned to matched claims (see Appendix E for the detailed procedure of the manual examination).
Topics
Bibliography
Abilov, A., Hua, Y., Matatov, H., Amir, O., & Naaman, M. (2021). VoterFraud2020: a Multi-modal dataset of election fraud claims on Twitter. Proceedings of the International AAAI Conference on Web and Social Media, 15, 901–912. https://doi.org/10.1609/icwsm.v15i1.18113
Allcott, H., & Gentzkow, M. (2017). Social media and fake news in the 2016 election. Journal of Economic Perspectives, 31(2), 211–236. https://doi.org/10.1257/jep.31.2.211
Allcott, H., Gentzkow, M., & Yu, C. (2019). Trends in the diffusion of misinformation on social media. Research & Politics, 6(2). https://doi.org/10.1177/2053168019848554
Amazeen, M. A. (2015). Revisiting the epistemology of fact-checking. Critical Review, 27(1), 1–22. https://doi.org/10.1080/08913811.2014.993890
Amazeen, M. A. (2016). Checking the fact-checkers in 2008: Predicting political ad scrutiny and assessing consistency. Journal of Political Marketing, 15(4), 433–464. https://doi.org/10.1080/15377857.2014.959691
Ananny, M. (2018). The partnership press: Lessons for platform-publisher collaborations as Facebook and news outlets team to fight misinformation. Tow Center for Digital Journalism, Columbia University. https://doi.org/10.7916/D85B1JG9
Brashier, N. M., Pennycook, G., Berinsky, A. J., & Rand, D. G. (2021). Timing matters when correcting fake news. Proceedings of the National Academy of Sciences, 118(5), e2020043118. https://doi.org/10.1073/pnas.2020043118
Cinelli, M., Quattrociocchi, W., Galeazzi, A., Valensise, C. M., Brungnoli, E., Schmidt, A. L., Zola, P., Zollo, F., & Scala, A. (2020). The COVID-19 social media infodemic. Scientific Reports, 10. https://doi.org/10.1038/s41598-020-73510-5
Cui, L., Seo, H., Tabar, M., Ma, F., Wang, S., & Lee, D. (2020). DETERRENT: Knowledge guided graph attention network for detecting healthcare misinformation. In KDD ’20: Proceedings of the 26th ACM SIGKDD international conference on knowledge discovery & data mining (pp. 492–502). Association for Computing Machinery. https://doi.org/10.1145/3394486.3403092
D’Ulizia, A., Caschera, M. C., Ferri, F., & Grifoni, P. (2021). Fake news detection: A survey of evaluation datasets. PeerJ Computer Science, 7. https://doi.org/10.7717/PEERJ-CS.518/SUPP-2
Ecker, U. K., Lewandowsky, S., Cook, J., Schmid, P., Fazio, L. K., Brashier, N., Kendeou, P., Vraga, E. K., & Amazeen, M. A. (2022). The psychological drivers of misinformation belief and its resistance to correction. Nature Reviews Psychology, 1(1), 13–29. https://doi.org/10.1038/s44159-021-00006-y
Garett, R., & Young, S. D. (2021). Online misinformation and vaccine hesitancy. Translational Behavioral Medicine, 11(12), 2194–2199. https://doi.org/10.1093/tbm/ibab128
Grinberg, N., Joseph, K., Friedland, L., Swire-Thompson, B., & Lazer, D. (2019). Fake news on Twitter during the 2016 U.S. presidential election. Science, 363(6425), 374–378. https://doi.org/10.1126/science.aau2706
Jolley, D., & Douglas, K. M. (2017). Prevention is better than cure: Addressing anti‐vaccine conspiracy theories. Journal of Applied Social Psychology, 47(8), 4594–69. https://doi.org/10.1111/jasp.12453
Kaur, S., Kumar, P., & Kumaraguru, P. (2020). Automating fake news detection system using multi-level voting model. Soft Computing, 24(12), 9049–9069. https://doi.org/10.1007/s00500-019-04436-y
Lee, S., Forrest, J. P., Strait, J., Seo, H., Lee, D., & Xiong, A. (2020). Beyond cognitive ability: Susceptibility to fake news is also explained by associative inference. In CHI EA ’20: Extended abstracts of the 2020 CHI conference on human factors in computing systems (pp. 1–8). Association for Computing Machinery. https://doi.org/10.1145/3334480.3383077
Lee, S., Seo, H., Lee, D., & Xiong, A. (2023). Associative inference can increase people’s susceptibility to misinformation. Proceedings of the International AAAI Conference on Web and Social Media, 17(1), 530–541. https://doi.org/10.1609/icwsm.v17i1.22166
Lim, C. (2018). Checking how fact-checkers check. Research & Politics, 5(3). https://doi.org/10.1177/2053168018786848
Marietta, M., Barker, D. C., & Bowser, T. (2015). Fact-checking polarized politics: Does the fact-check industry provide consistent guidance on disputed realities? The Forum, 13(4), 577–596. https://doi.org/10.1515/for-2015-0040
Nieminen, S., & Rapeli, L. (2019). Fighting misperceptions and doubting journalists’ objectivity: A review of fact-checking literature. Political Studies Review, 17(3), 296–309. https://doi.org/10.1177/1478929918786852
Pennycook, G., & Rand, D. G. (2019). Lazy, not biased: Susceptibility to partisan fake news is better explained by lack of reasoning than by motivated reasoning. Cognition, 188, 39–50. https://doi.org/10.1016/j.cognition.2018.06.011
Qader, W. A., Ameen, M. M., & Ahmed, B. I. (2019). An overview of bag of words; Importance, implementation, applications, and challenges. In 2019 International Engineering Conference (IEC) (pp. 200–204). IEEE. https://doi.org/10.1109/IEC47844.2019.8950616
Rana, M. S., Nobi, M. N., Murali, B., & Sung, A. H. (2022). Deepfake detection: A systematic literature review. IEEE Access, 10, 25494–25513. https://doi.org/10.1109/ACCESS.2022.3154404
Reimers, N., & Gurevych, I. (2019). Sentence-BERT: Sentence embeddings using Siamese BERT-networks. arXiv. http://arxiv.org/abs/1908.10084
Sharma, K., Ferrara, E., & Liu, Y. (2022). Characterizing online engagement with disinformation and conspiracies in the 2020 U.S. presidential election. Proceedings of the International AAAI Conference on Web and Social Media, 16(1), 908–919. https://doi.org/10.1609/icwsm.v16i1.19345
Shu, K., Cui, L., Wang, S., Lee, D., & Liu, H. (2019). dEFEND: Explainable fake news detection. In KDD ’19:Proceedings of the 25th ACM SIGKDD international conference on knowledge discovery & data mining (pp. 395–405). Association for Computing Machinery. https://doi.org/10.1145/3292500.3330935
Thomas, D. R. (2006). A general inductive approach for analyzing qualitative evaluation data. American Journal of Evaluation, 27(2), 237–246. https://doi.org/10.1177/1098214005283748
van der Linden, S. (2022). Misinformation: Susceptibility, spread, and interventions to immunize the public. Nature Medicine, 28(3), 460–467. https://doi.org/10.1038/s41591-022-01713-6
Vosoughi, S., Roy, D., & Aral, S. (2018). The spread of true and false news online. Science, 359(6380), 1146–1151. https://doi.org/10.1126/SCIENCE.AAP9559
Walker, M., & Matsa, K. E. (2021). News consumption across social media in 2021. Pew Research Center. https://www.pewresearch.org/journalism/2021/09/20/news-consumption-across-social-media-in-2021/
Walter, N., & Murphy, S. T. (2018). How to unring the bell: A meta-analytic approach to correction of misinformation. Communication Monographs, 85(3), 423–441. https://doi.org/10.1080/03637751.2018.1467564
Walter, N., & Tukachinsky, R. (2020). A meta-analytic examination of the continued influence of misinformation in the face of correction: How powerful is it, why does it happen, and how to stop it? Communication Research, 47(2), 155–177. https://doi.org/10.1177/0093650219854
World Health Organization (2020). Coronavirus disease 2019 (COVID-19) situation report – 95. https://www.who.int/docs/default-source/coronaviruse/situation-reports/20200424-sitrep-95-covid-19.pdf?sfvrsn=e8065831_4
Wu, L., Morstatter, F., Carley, K. M., & Liu, H. (2019). Misinformation in social media. ACM SIGKDD Explorations Newsletter, 21(2), 80–90. https://doi.org/10.1145/3373464.3373475
Xiong, A., Lee, S., Seo, H., & Lee, D. (2023). Effects of associative inference on individuals’ susceptibility to misinformation. Journal of Experimental Psychology: Applied, 29(1), 1–17. https://doi.org/10.1037/xap0000418
Zhang, X., & Ghorbani, A. A. (2020). An overview of online fake news: Characterization, detection, and discussion. Information Processing and Management, 57(2). https://doi.org/10.1016/J.IPM.2019.03.004
Zhou, X., & Zafarani, R. (2020). A survey of fake news. ACM Computing Surveys (CSUR), 53(5), 1–40. https://doi.org/10.1145/3395046
Funding
This research was supported in part by Penn State under PSU SSRI Seed Grant and the National Science Foundation under grants 1820609, 1915801, and 2121097.
Competing Interests
The authors declare no competing interests.
Ethics
Institutional review board approval was not required for this study.
Copyright
This is an open access article distributed under the terms of the Creative Commons Attribution License, which permits unrestricted use, distribution, and reproduction in any medium, provided that the original author and source are properly credited.
Data Availability
All materials needed to replicate this study are available via the Harvard Dataverse: https://doi.org/10.7910/DVN/FXYZDT
Acknowledgements
Special thanks to Jie Zhu for assisting as one of the coders in manually examining matching claims in disagreements.