Commentary
Misinformation reloaded? Fears about the impact of generative AI on misinformation are overblown
Article Metrics
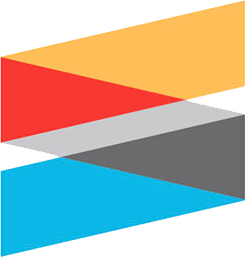
3
CrossRef Citations
Altmetric Score
PDF Downloads
Page Views
Many observers of the current explosion of generative AI worry about its impact on our information environment, with concerns being raised about the increased quantity, quality, and personalization of misinformation. We assess these arguments with evidence from communication studies, cognitive science, and political science. We argue that current concerns about the effects of generative AI on the misinformation landscape are overblown.
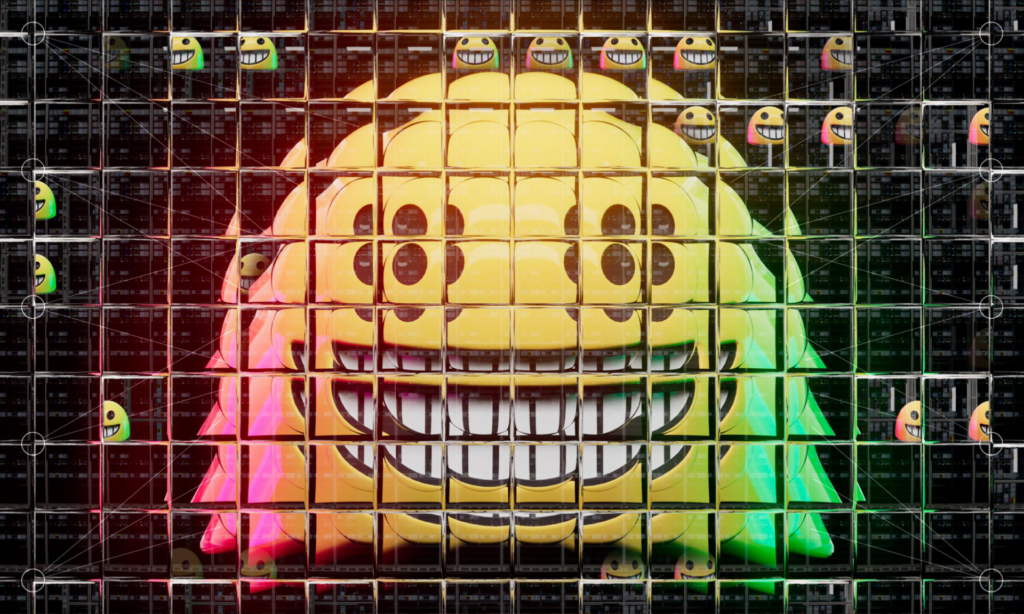
Introduction
Recent progress in generative AI has led to concerns that it will “trigger the next misinformation nightmare” (Gold & Fisher, 2023), that people “will not be able to know what is true anymore” (Metz, 2023), and that we are facing a “tech-enabled Armageddon” (Scott, 2023).
Generative AI systems are capable of generating new forms of data by applying machine learning to large quantities of training data. This new data can include text (such as Google’s Bard, Meta’s LLaMa, or OpenAI’s ChatGPT), visuals (such as Stable Diffusion or OpenAI’s DALL-E), or audio (such as Microsoft’s VALL-E). The output that can be produced with these systems at great speed and ease for a majority of users is, depending on the instructions, sufficiently sophisticated that humans can perceive it as indistinguishable from human-generated content (Groh et al., 2022).
According to various voices, including some leading AI researchers, generative AI will make it easier to create realistic but false or misleading content at scale, with potentially catastrophic outcomes for people’s beliefs and behaviors, the public arena of information,1We understand the public arena to be the common but contested mediated space in which different actors exchange information, discuss matters of common concern, and which mediates the relation between different parts of society (Jungherr & Schroeder, 2021a). and democracy. These concerns can be divided in four categories (Table 1).
Argument | Explanation of claim | Presumed effect | Source |
1. Increased quantity of misinformation | Due to the ease of access and use, generative AIs can be used to create mis-/disinformation at scale at little to no cost to individuals and organized actors | Increased quantity of misinformation allows ill-intentioned actors to “flood the zone” with incorrect or misleading information, thus drowning out factual content and/or sowing confusion | Bell (2023), Fried (2023), Hsu & Thompson (2023), Marcus (2023), Pasternack (2023), Ordonez et al. (2023), Tucker (2023), Zagni & Canetta (2023) |
2. Increased quality of misinformation | Due to their technical capabilities and ease of use, generative AIs can be used to create higher-quality misinformation | Increased quality of misinformation leads to increased persuasive potential, as it creates content that is more plausible and harder to debunk or verify. This would either allow for the spread of false information or contribute (with the increased quantity of misinformation) to an epistemic crisis, a general loss of trust in all types of news | Epstein & Hertzmann (2023), Fried (2023), Goldstein et al. (2023), Hsu & Thompson (2023), Pasternack (2023), Tiku (2022), Tucker (2023) |
3. Increased personalization of misinformation | Due to their technical capabilities and ease of use, generative AIs can be used to create high-quality misinformation personalized to a user’s tastes and preferences | Increased persuasion of consumers of misinformation, with the same outcomes as above | Benson (2023), Fried (2023), Hsu & Thompson (2023), Pasternack (2023) |
4. Involuntary generation of plausible but false information | Generative AIs can generate useful content (e.g., chatbots generating code). However, they can also generate plausible-looking information that is entirely inaccurate. Without intending to, users could thus generate misinformation, which could potentially spread | Misinforming users of generative AI and potentially those with whom they share the information | Fried (2023), Gold & Fischer (2023), Ordonez et al. (2023), Pasternack (2023), Shah & Bender (2023), Zagni & Canetta (2023) |
We review, in turn, the first three arguments—quantity, quality, and personalization—arguing that they are at the moment speculative, and that existing research suggests at best modest effects of generative AI on the misinformation landscape. Looking at each of these themes in turn, we argue that current concerns about the effects of generative AI are overblown.
We do not address the fourth argument in detail here, as it lies beyond the scope of this commentary and is too idiosyncratic to the constantly evolving versions of generative AI tools under discussion and the context of use (e.g., GPT-4 avoids many of the mistakes made by GPT-2 or -3). People with low online literacy will likely be misled by some of these tools, but we do not see a clear route for the mistakes that generative AI makes, in the hands of well-intentioned users, to spread and create a significant risk for society. Similarly, the risk of factually inaccurate information accidentally appearing in news content as news media increasingly make use of generative AI (Hanley & Durumeric, 2023) will plausibly be curtailed by publishers’ efforts to control the use of the technology in news production and distribution (Arguedas & Simon, 2023; Becker et al., 2023), although failure on the part of publishers to implement such measures or a fundamental lack of awareness regarding the issue remain a concern.
Increased quantity of misinformation
Generative AI makes it easier to create misinformation, which could increase the supply of misinformation. However, it is not because there is more misinformation that people will necessarily consume more of it. Instead, we argue here that the consumption of misinformation is mostly limited by demand and not by supply.
Increases in the supply of misinformation should only increase the diffusion of misinformation if there is currently an unmet demand and/or a limited supply of misinformation. Neither possibility is supported by evidence. Regarding limited supply, the already low costs of misinformation production and access, and the large number of misinformation posts that currently exist but go unnoticed, means that generative AI has very little room to operate. Regarding unmet demand, given the creativity humans have showcased throughout history to make up (false) stories and the freedom that humans already have to create and spread misinformation across the world, it is unlikely that a large part of the population is looking for misinformation they cannot find online or offline. Moreover, as we argue below, demand for misinformation is relatively easy to meet because the particular content of misinformation is less important than the broad narrative it supports.
In absolute terms, misinformation already abounds online and, unlike high-quality news or scientific articles, it is rarely behind paywalls. Yet, despite the quantity and accessibility of misinformation, the average internet user consumes very little of it (e.g., Allen et al., 2020; for review, see Acerbi et al., 2022). Instead, misinformation consumption is heavily concentrated in a small portion of very active and vocal users (Grinberg et al. 2019). What makes misinformation consumers special is not that they have privileged access to misinformation but traits that make them more likely to seek out misinformation (Broniatowski et al., 2023; Motta et al., 2023), such as having low trust in institutions or being strong partisans (Osmundsen et al., 2021). Experts on misinformation view partisanship and identity as key determinants of misinformation belief and sharing, while they believe lack of access to reliable information only plays a negligible role (Altay et al., 2023). The problem is not that people do not have access to high-quality information but instead that they reject high-quality information and favor misinformation. Similarly, conspiracy theories exist everywhere and are easily accessible online across the globe. Yet, despite similarities in supply, demand for conspiracy theories varies across countries, such that in more corrupt countries, conspiracy theories are more popular (Alper, 2023; Cordonier & Cafiero, 2023).
Finally, (mis)information, on its own, has no causal effect on the world. (Mis)information only gains causal powers when humans see it. Yet, the number of things that go viral on the internet and get seen is finite because our attention is finite (Jungherr & Schroeder, 2021a; Taylor, 2014). And since generative AI is unlikely to increase demand for misinformation and will not increase the number of things humans can pay attention to, the increase in misinformation supply will likely have limited influence on the diffusion of misinformation.
Increased quality of misinformation
Another argument suggesting that generative AI raises a significant threat to the public arena is that generative AI can help create misinformation that is more persuasive than that created by current means. For instance, in the same way as generative AI can create a text in the style of a limerick or of a particular author, generative AIs could create content that looks more reliable, professional, scientific, and accurate (by using sophisticated words, the appropriate tone, scientific looking references, etc.). Experimental studies have shown that the credibility of online sources can be affected by such features (e.g., Metzger, 2007), lending support to the argument. However, there are at least three reasons why this may not be a significant cause for concern.
First, it seems that it would already be relatively easy for producers of misinformation to increase the perceived reliability of their content. In the realm of visuals, Photoshop has long afforded bad actors the ability to make an artificially created image look real (Kapoor & Narayanan, 2023). If they opt not to do so, it might be because making a text or image look more reliable or real might conflict with other goals, such as making the same more accessible, appealing, or seemingly authentic. It’s not clear that generative AIs could increase content quality on multiple dimensions, as there might be some unavoidable tradeoffs.
Second, even if generative AI managed to increase the overall appeal of misinformation, most people are simply not exposed, or only very marginally exposed, to misinformation (see above and Acerbi et al., 2022). Instead, most people overwhelmingly consume content from mainstream sources, typically the same handful of popular media outlets (Altay et al., 2022; Guess et al., 2021). As a result, any increase in the quality of misleading content would be largely invisible to most of the public.
Third, generative AI could also help increase the quality of reliable news sources—for instance, by facilitating the work of journalists in some areas. Given the rarity of misleading content compared to reliable content, the increase in the appeal of misinformation would have to be 20 to 100 times larger than the increase in the appeal of reliable content for the effects of generative AI to tip the scales in favor of misinformation (Acerbi et al., 2022). We are not aware of an argument that would suggest such a massive imbalance in the effects of generative AI on misinformation and on reliable information.
Fourth, it has been argued that generative AI provides actors with a new argument for plausible deniability in what has been called “the liar’s dividend”—where the availability of a technology creating high-quality content can be used to dismiss incriminating evidence as fake (Christopher, 2023). However, while there is limited evidence that such a strategy can have some effect, for example for politicians (Schiff et al., 2023), this possibility does not hinge on the technology itself. As mentioned above, technology that enables creating plausible fake content has been available for (at least) decades, and it has already been used in attempts to discredit evidence.2See for instance Donald Trump’s questioning of the authenticity of the so-called “Access Hollywood” tape: https://www.nytimes.com/2017/11/28/us/politics/trump-access-hollywood-tape.html. Arguably, the major factor deciding the effectiveness of such attempts is not the plausibility of a given technology being able to generate some content but other factors, such as partisanship or people’s preexisting trust in the individual attempting to discredit the evidence (Ecker et al., 2022).
Increased personalization of misinformation
The final argument is that generative AI will make it easier to create and micro-target users with personalized misinformation that plays to their beliefs and preferences, thus making it easier to persuade or mislead them. Looking at the abilities of generative AI to mimic a variety of styles and personalities, this certainly seems plausible. However, there are also problems with this argument.
First, the technological infrastructures that enable micro-targeting of users with content are not directly impacted by improvements in generative AI (although they might improve through advances in AI more broadly).3A matter for conjecture is whether generative AI could be used to form relationships with individuals at scale (for example through chatbots messaging people on social media pretending to be real people), and then once having formed a relationship begin feeding them misinformation. However, such a possibility remains entirely speculative at the moment, and we do not discuss it here. As a result, generative AI should not affect the efficiency of the infrastructure by which content reaches individuals. The cost of reaching people with misinformation, rather than the cost of creating it, remains a bottleneck (see also Kapoor & Narayanan, 2023). In addition, the evidence suggests that micro-targeting by, for example, political actors has mostly limited persuasive effects on the majority of recipients (Jungherr et al., 2020; Simon, 2019), not least because many people do not pay attention to these messages in the first place (Kahloon & Ramani, 2023).
Still, generative AI might be able to improve on the content of already targeted misinformation, making it more suited to its target. However, the evidence on the effectiveness of political advertising personalized to target, for instance, people with different personalities is mixed, with at best small and context-dependent effects (Zarouali et al., 2022). In addition, the assumption that generative AI will be able to create more personalized and thus more persuasive content is so far unproven. Current generative AIs are trained in intervals on a large general corpus of data, aided by approaches such as reinforcement learning with human feedback (RLHF) or retrieval augmented generation (RAG), where an information retrieval system provides more up-to-date data when an LLM produces output. However, LLMs are currently unable to represent the full range of users’ preferences and values (Kirk et al., 2023), and do not hold direct information about users themselves, which severely limits their ability to create truly personalized content that would match an individual’s preferences (Newport, 2023). Even with advances on these fronts, current evidence suggests that the persuasive effects of microtargeting—including with LLMs—are often limited and highly context-dependent (Hackenburg & Margetts, 2023; Tappin et al., 2023). In general, the effects of political advertising are small and will likely remain so, regardless of whether they are (micro)targeted or not, because persuasion is difficult (Coppock, 2023; Mercier, 2020).
Conclusion
We have argued that concerns over the effects of generative AI on the information landscape—and, in particular, the spread of misinformation—are overblown. These concerns are part of an old and broad family of moral panics surrounding new technologies (Jungherr & Schroeder, 2021b; Orben, 2020; Simon & Camargo, 2021). When it comes to new information technologies, such panics might be based on the mistaken assumption that people are gullible, driven in part by the third-person effect (Altay & Acerbi, 2023; Mercier, 2020).
These concerns also tend to overlook the fact that we already owe our current information environment to a complex web of institutions that has allowed the media to provide broadly accurate information, and for the public, in turn, to trust much of the information communicated by the media. These institutions have already evolved to accommodate new media, such as film and photography (Habgood-Cote, 2023; Jurgenson, 2019), even though it has always been possible to manipulate these media. Moreover, it’s far from clear that the more technologically complex forms of manipulation are the most efficient: nowadays, journalists and fact checkers struggle not so much with deepfakes but with visuals taken out of context or with crude manipulations, such as cropping of images or so-called “cheapfakes” (Brennen et al., 2020; Kapoor & Narayanan, 2023; Paris & Donovan, 2019; Weikmann & Lecheler, 2023).
A limitation of our argument is that we mostly rely on evidence about the media environment of wealthy, democratic countries with rich and competitive media ecosystems. Less data is available in other countries, and we cannot rule out that generative AI might have a larger negative effect there (although, arguably, generative AI could also have a larger positive effect in these countries).
We are not arguing that nothing needs to be done to regulate or address generative AI. If misinformation is so rare in the information environment of wealthy, democratic countries, it is thanks to the hard work of professionals—journalists, fact checkers, experts, etc.—and to the norms and know-how that have developed over time in these professions (e.g., Paris and Donovan, 2019; Silverman, 2014). Strengthening these institutions and trust in reliable news overall (Acerbi et al., 2022) will likely be pivotal. Journalists, fact checkers, authorities, and human rights advocates will also face new challenges, and they will have to develop new norms and practices to cope with generative AI.4For a discussion, see Gregory (2023). This includes, for instance, norms and know-how related to disclosure, “fingerprinting” of content, and the establishment of provenance mechanisms.5See, e.g., the Responsible Practices for Synthetic Media proposed by Partnership on AI. Digital and media literacy education could also help abate issues arising with AI-generated misinformation (e.g., Doss et al., 2023).
Time will tell whether alarmist headlines about generative AI were warranted or not, but regardless of the outcome, the discussion of the impact of generative AI on misinformation would benefit from being more nuanced and evidence-based, especially against the backdrop of ongoing regulatory efforts.
The Council of Europe (2019), for example, stresses that the exercise and enjoyment of individual human rights and “the dignity of all humans as independent moral agents” should be protected from underexplored forms of algorithmic (aided) persuasion which “may have significant effects on the cognitive autonomy of individuals and their right to form opinions and take independent decisions” (paragraph 9). Elsewhere, the upcoming EU AI Act will likely include stipulations regarding how the issues discussed here should be best addressed, while the United States has released a blueprint for an “AI Bill of Rights” and is trying to regulate the use of AI in a fraught political environment. In addition, there are efforts by non-governmental organizations such as Reporters Without Borders to draft guidelines that “safeguard information integrity” amid the growing use of AI for information production, dissemination, retrieval, and consumption.
Yet, while such efforts are laudable and required, they should be based on the best available evidence—especially if this evidence questions received wisdom. Excessive and speculative warnings about the ill effects of AI on the public arena and democracy, even if well-intentioned, can also have negative externalities, such as reducing trust in factually accurate news and the institutions that produce it (Hameleers, 2023) or overshadowing other problems posed by generative AI, like nonconsensual pornography disproportionately harming women even if they do not scale up (Kapoor & Narayanan, 2023), or the potential for identity thefts and scams.
Our aim is not to settle or close the discussion around the possible effects of generative AI on our information environment. We also do not wish to simply dismiss concerns around the technology. Instead, in the spirit of Robert Merton’s observation that “the goal of science is the extension of certified knowledge” on the basis of “organized skepticism” (Merton, 1973, pp. 267–278) we hope to contribute to the former by injecting some of the latter into current debates on the possible effects of generative AI.
Topics
Bibliography
Acerbi, A., Altay, S., & Mercier, H. (2022). Research note: Fighting misinformation or fighting for information? Harvard Kennedy School (HKS) Misinformation Review, 3(1). https://doi.org/10.37016/mr-2020-87
Alper, S. (2023). There are higher levels of conspiracy beliefs in more corrupt countries. European Journal of Social Psychology, 53(3), 503–517. https://doi.org/10.1002/ejsp.2919
Altay, S., & Acerbi, A. (2023). People believe misinformation is a threat because they assume others are gullible. New Media & Society. https://doi.org/10.1177/14614448231153379
Altay, S., Nielsen, R. K., & Fletcher, R. (2022). Quantifying the “infodemic”: People turned to trustworthy news outlets during the 2020 coronavirus pandemic. Journal of Quantitative Description: Digital Media, 2. https://journalqd.org/article/view/3617/2703
Altay, S., Berriche, M., Heuer, H., Farkas, J., & Rathje, S. (2023). A survey of expert views on misinformation: Definitions, determinants, solutions, and future of the field. Harvard Kennedy School (HKS) Misinformation Review, 4(4). https://doi.org/10.37016/mr-2020-119
Allen, J., Howland, B., Mobius, M., Rothschild, D., & Watts, D. J. (2020). Evaluating the fake news problem at the scale of the information ecosystem. Science Advances, 6(14). https://doi.org/10.1126/sciadv.aay3539
Arguedas, A. R., & Simon, F. M. (2023). Automating democracy: Generative AI, journalism, and the future of democracy. Balliol Interdisciplinary Institute, University of Oxford. http://dx.doi.org/10.5287/ora-e262xv7no
Becker, K. B., Simon, F. M., & Crum, C. (2023). Policies in parallel? A comparative study of journalistic AI policies in 52 global news organisations. SocArXiv. https://doi.org/10.31235/osf.io/c4af9
Bell, E. (2023, March 3). Fake news, ChatGPT, truth, journalism, disinformation. The Guardian. https://www.theguardian.com/commentisfree/2023/mar/03/fake-news-chatgpt-truth-journalism-disinformation
Benson, T. (2023, August 1). This disinformation is just for you. Wired. https://www.wired.com/story/generative-ai-custom-disinformation/
Brennen, J. S., Simon, F. M., & Nielsen, R. K. (2020). Beyond (mis)representation: Visuals in COVID-19 misinformation. The International Journal of Press/Politics, 26(1), 277–299. https://doi.org/10.1177/1940161220964780
Broniatowski, D. A., Simons, J. R., Gu, J., Jamison, A. M., & Abroms, L. C. (2023). The efficacy of Facebook’s vaccine misinformation policies and architecture during the COVID-19 pandemic. Science Advances, 9(37). https://doi.org/10.1126/sciadv.adh2132
Christopher, N. (2023, July 5). An Indian politician says scandalous audio clips are AI deepfakes. We had them tested. Rest of World. https://restofworld.org/2023/indian-politician-leaked-audio-ai-deepfake/
Coppock, A. (2023). Persuasion in parallel: How information changes minds about politics. University of Chicago Press.
Cordonier, L., & Cafiero, F. (2023). Public sector corruption is fertile ground for conspiracy beliefs: A comparison between 26 Western and non-Western countries. OSF. https://doi.org/10.31219/osf.io/b24gk
Council of Europe Committee of Ministers. (2019, February 13). Declaration by the Committee of Ministers on the manipulative capabilities of algorithmic processes (Adopted by the Committee of Ministers on 13 February 2019 at the 1337th meeting of the Ministers’ Deputies). Council of Europe. https://search.coe.int/cm/pages/result_details.aspx?ObjectId=090000168092dd4b#globalcontainer
Doss, C., Mondschein, J., Shu, D., Wolfson, T., Kopecky, D., Fitton-Kane, V. A., Bush, L., & Tucker, C. (2023). Deepfakes and scientific knowledge dissemination. Scientific Reports, 13(1), 13429. https://doi.org/10.1038/s41598-023-39944-3
Ecker, U. K. H., Lewandowsky, S., Cook, J., Schmid, P., Fazio, L. K., Brashier, N., Kendeou, P., Vraga, E. K., & Amazeen, M. A. (2022). The psychological drivers of misinformation belief and its resistance to correction. Nature Reviews Psychology, 1(1), 13–29. https://doi.org/10.1038/s44159-021-00006-y
Epstein, Z., & Hertmann, A. (2023). Art and the science of generative AI. Science, 380(6650), 1110–1111. https://doi.org/10.1126/science.adh4451
Fried, I. (2023, July 10). How AI will turbocharge misinformation—And what we can do about it. Axios. https://www.axios.com/2023/07/10/ai-misinformation-response-measures
Goldstein, J. A, Chao, J., & Grossman, S., Stamos, A. & Tomz, M. (2023). Can AI write persuasive propaganda? SocArXiv. https://doi.org/10.31235/osf.io/fp87b
Gold, A. & Fischer, S. (2023, February 21). Chatbots trigger next misinformation nightmare. Axios. https://www.axios.com/2023/02/21/chatbots-misinformation-nightmare-chatgpt-ai
Gregory, S. (2023). Fortify the truth: How to defend human rights in an age of deepfakes and generative AI. Journal of Human Rights Practice, huad035. https://doi.org/10.1093/jhuman/huad035
Grinberg, N., Joseph, K., Friedland, L., Swire-Thompson, B., & Lazer, D. (2019). Fake news on Twitter during the 2016 U.S. presidential election. Science, 363(6425), 374–378. https://doi.org/10.1126/science.aau2706
Groh, M., Sankaranarayanan, A., Lippman, A., & Picard, R. (2022). Human detection of political deepfakes across transcripts, audio, and video. arXiv. https://doi.org/10.48550/arXiv.2202.12883
Guess, A. M. (2021). (Almost) everything in moderation: New evidence on Americans’ online media diets. American Journal of Political Science, 65(4), 1007–1022. https://doi.org/10.1111/ajps.12589
Habgood-Coote, J. (2023). Deepfakes and the epistemic apocalypse. Synthese, 201(103). https://doi.org/10.1007/s11229-023-04097-3
Hackenburg, K., & Margetts, H. (2023). Evaluating the persuasive influence of political microtargeting with large language models. OSF. https://doi.org/10.31219/osf.io/wnt8b
Hameleers, M. (2023). The (un)intended consequences of emphasizing the threats of mis- and disinformation. Media and Communication, 11(2), 5–14. https://doi.org/10.17645/mac.v11i2.6301
Hanley, H. W., & Durumeric, Z. (2023). Machine-made media: Monitoring the mobilization of machine-generated articles on misinformation and mainstream news websites. arXiv. https://doi.org/10.48550/arXiv.2305.09820
Hsu, T., & Thompson, S. A. (2023, February 8). AI chatbots could spread disinformation, experts warn. The New York Times. https://www.nytimes.com/2023/02/08/technology/ai-chatbots-disinformation.html
Jungherr, A., & Schroeder, R. (2021a). Digital transformations of the public arena. Cambridge University Press.
Jungherr, A., & Schroeder, R. (2021b). Disinformation and the structural transformations of the public arena: Addressing the actual challenges to democracy. Social Media + Society, 7(1). https://doi.org/10.1177/2056305121988928
Jungherr, A., Rivero, G., & Gayo-Avello, D. (2020). Retooling politics: How digital media are shaping democracy. Cambridge University Press.
Jurgenson, N. (2019). The social photo: On photography and social media. Verso.
Kahloon, I., & Ramani, A. (2023, August 31). AI will change American elections, but not in the obvious way. The Economist. https://www.economist.com/united-states/2023/08/31/ai-will-change-american-elections-but-not-in-the-obvious-way
Kapoor, S., & Narayanan, A. (2023). How to prepare for the deluge of generative AI on social media. Knight First Amendment Institute, Columbia University. https://knightcolumbia.org/content/how-to-prepare-for-the-deluge-of-generative-ai-on-social-media
Kirk, H. R., Vidgen, B., Röttger, P., & Hale, S. A. (2023). Personalisation within bounds: A risk taxonomy and policy framework for the alignment of large language models with personalised feedback. arXiv. https://doi.org/10.48550/arXiv.2303.05453
Marcus, G. (2023, February 8). AI’s Jurassic Park moment. Communications of the ACM. https://cacm.acm.org/blogs/blog-cacm/267674-ais-jurassic-park-moment/fulltext
Mercier, H. (2020). Not born yesterday: The science of who we trust and what we believe. Princeton University Press.
Merton, R. K. (1973). The sociology of science: Theoretical and empirical investigations. University of Chicago Press.
Metz, C. (2023, May 1). ‘The Godfather of A.I.’ leaves Google and warns of danger ahead. The New York Times. https://www.nytimes.com/2023/05/01/technology/ai-google-chatbot-engineer-quits-hinton
Metzger, M. J. (2007). Making sense of credibility on the web: Models for evaluating online information and recommendations for future research. Journal of the American Society for Information Science and Technology, 58(13), 2078–2091. https://doi.org/10.1002/asi.20672
Motta, M., Hwang, J., & Stecula, D. (2023). What goes down must come up? Pandemic-related misinformation search behavior during an unplanned Facebook outage. Health Communication. https://doi.org/10.1080/10410236.2023.2254583
Newport, C. (2023, April 13). What kind of mind does ChatGPT have? The New Yorker. https://www.newyorker.com/science/annals-of-artificial-intelligence/what-kind-of-mind-does-chatgpt-have
Ordonez, V., Dunn, T., & Noll, E. (2023, May 19). OpenAI CEO Sam Altman says AI will reshape society, acknowledges risks: ‘A little bit scared of this’. ABC News. https://abcnews.go.com/Technology/openai-ceo-sam-altman-ai-reshape-society-acknowledges/story?id=97897122
Orben, A. (2020). The Sisyphean cycle of technology panics. Perspectives on Psychological Science, 15(5), 1143–1157. https://doi.org/10.1177/1745691620919372
Osmundsen, M., Bor, A., Vahlstrup, P. B., Bechmann, A., & Petersen, M. B. (2021). Partisan polarization is the primary psychological motivation behind political fake news sharing on Twitter. American Political Science Review, 115(3), 999–1015. https://doi.org/10.1017/S0003055421000290
Paris, B., & Donovan, J. (2019). Deepfakes and cheapfakes. The manipulation of audio and visual evidence. Data & Society Research Institute. https://datasociety.net/library/deepfakes-and-cheap-fakes/
Pasternack, A. (2023, March 17). Deepfakes getting smarter thanks to GPT. FastCompany. https://www.fastcompany.com/90853542/deepfakes-getting-smarter-thanks-to-gpt
Scott, L. (2023, September 5). World faces ‘tech-enabled armageddon,’ Maria Ressa says. Voice of America. https://www.voanews.com/a/world-faces-tech-enabled-armageddon-maria-ressa-says-/7256196.html
Shah, C., & Bender, E. (2023). Envisioning information access systems: What makes for good tools and a healthy web? Unpublished manuscript. https://faculty.washington.edu/ebender/papers/Envisioning_IAS_preprint.pdf
Schiff, K. J., Schiff, D. S., & Bueno, N. (2022, May 11). The liar’s dividend: Can politicians use deepfakes and fake news to evade accountability? SocArXiv. https://doi.org/10.31235/osf.io/q6mwn
Silverman, C. (Ed.). (2014). Verification handbook: An ultimate guideline on digital age sourcing for emergency coverage. European Journalism Centre. https://datajournalism.com/read/handbook/verification-3
Simon, F. M. (2019). “We power democracy”: Exploring the promises of the political data analytics industry. The Information Society, 53(3), 158–169. https://doi.org/10.1080/01972243.2019.1582570
Simon, F. M., & Camargo, C. Q. (2021). Autopsy of a metaphor: The origins, use and blind spots of the ‘infodemic’. New Media & Society, 25(8), 2219–2240. https://doi.org/10.1177/14614448211031908
Tappin, B. M., Wittenberg, C., Hewitt, L. B., Berinsky, A. J., & Rand, D. G. (2023). Quantifying the potential persuasive returns to political microtargeting. Proceedings of the National Academy of Sciences, 120(25), e2216261120. https://doi.org/10.1073/pnas.2216261120
Taylor, G. (2014). Scarcity of attention for a medium of abundance. An economic perspective. In M. Graham & W. H. Dutton (Eds.), Society & the internet (pp. 257–271). Oxford University Press.
Tiku, S. (2022, June 21). Artificial intelligence images that look like real photos are here. The Washington Post. https://www.washingtonpost.com/technology/interactive/2022/artificial-intelligence-images-dall-e/
Tucker, J. (2023, July 14). AI could create a disinformation nightmare in the 2023 election. The Hill. https://thehill.com/opinion/4096006-ai-could-create-a-disinformation-nightmare-in-the-2024-election/
Weikmann, T., & Lecheler, S. (2023). Cutting through the hype: Understanding the implications of deepfakes for the fact-checking actor-network. Digital Journalism. https://doi.org/10.1080/21670811.2023.2194665
Zagni, G., & Canetta, T. (2023, April 5). Generative AI marks the beginning of a new era for disinformation. European Digital Media Observatory. https://edmo.eu/2023/04/05/generative-ai-marks-the-beginning-of-a-new-era-for-disinformation/
Zarouali, B., Dobber, T., De Pauw, G., & de Vreese, C. (2022). Using a personality-profiling algorithm to investigate political microtargeting: Assessing the persuasion effects of personality-tailored ads on social media. Communication Research, 49(8), 1066–1091. https://doi.org/10.1177/0093650220961965
Funding
Felix M. Simon gratefully acknowledges support from the OII-Dieter Schwarz Scholarship at the University of Oxford. Sacha Altay received funding from the European Research Council (ERC) under the European Union’s Horizon 2020 research and innovation program (grant agreement no. 883121). Hugo Mercier received funding from the Agence Nationale De La Recherche (ANR) (ANR-21-CE28-0016-01, as well as ANR-17-EURE-0017 to FrontCog, and ANR-10-IDEX-0001-02 to PSL).
Competing Interests
The authors have no conflicts of interest to disclose.
Copyright
This is an open access article distributed under the terms of the Creative Commons Attribution License, which permits unrestricted use, distribution, and reproduction in any medium, provided that the original author and source are properly credited.
Authorship
All authors contributed equally to this article.
Acknowledgements
Felix M. Simon would like to thank Hannah Kirk, Hal Hodson, Ralph Schroeder, Michelle Disser, and Emily Bell for insightful discussions on the topic. He is also grateful for the insights of participants at various off-the-record roundtable discussions on the topic.