Peer Reviewed
Where conspiracy theories flourish: A study of YouTube comments and Bill Gates conspiracy theories
Article Metrics
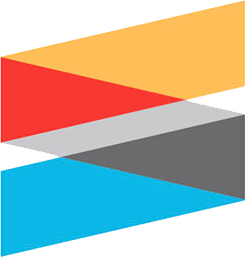
2
CrossRef Citations
Altmetric Score
PDF Downloads
Page Views
We studied YouTube comments posted to Covid-19 news videos featuring Bill Gates and found they were dominated by conspiracy theories. Our results suggest the platform’s comments feature operates as a relatively unmoderated social media space where conspiracy theories circulate unchecked. We outline steps that YouTube can take now to improve its approach to moderating misinformation.
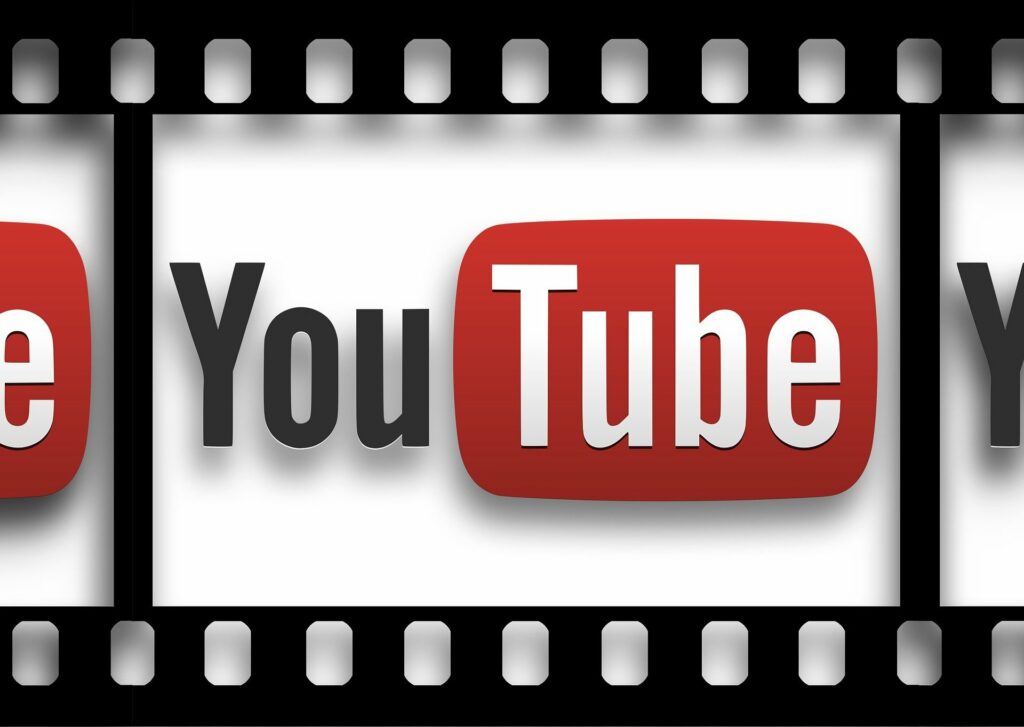
Research Questions
- RQ1a: What are the dominant conspiratorial themes discussed among YouTube commenters on news videos about Bill Gates and Covid-19?
- RQ1b: Which conspiratorial topics on these news videos attract the most user engagement?
- RQ2: What discursive strategies are YouTube commenters using to formulate and share conspiracy theories about Bill Gates and Covid-19?
Essay Summary
- During the Covid-19 pandemic, YouTube introduced new policies and guidelines aimed at limiting the spread of medical misinformation on the platform, but the comments feature remains relatively unmoderated and has low barriers to entry for posting publicly.
- We studied a dataset of 38,564 YouTube comments, drawn from three Covid-19-related videos posted by news media organisations Fox News, Vox, and China Global Television Network. Each video featured Bill Gates and, at the time of data extraction, had between 13,000–14,500 comments posted between April 5, 2020, and March 2, 2021.
- Through topic modelling and qualitative content analysis, we found the comments for each video to be heavily dominated by conspiratorial statements, covering topics such as Bill Gates’s hidden agenda, his role in vaccine development and distribution, his body language, his connection to Jeffrey Epstein, 5G network harms, and human microchipping.
- Results suggest that during the Covid-19 pandemic, YouTube’s comments feature may have played an underrated role in participatory cultures of conspiracy theory knowledge production and circulation. The platform should consider design and policy changes that respond to discursive strategies used by conspiracy theorists to prevent similar outcomes for future high-stakes public interest matters.
Implications
YouTube has been a popular source of information among diverse populations throughout the Covid-19 pandemic (Khatri et al., 2020). In 2020, in response to criticisms that it was amplifying misinformation about the virus (Bruns et al., 2020; Shahsavari et al., 2020), the platform introduced a range of policies and design changes aimed at limiting the spread of Covid-19 medical misinformation. These included a system for amplifying authoritative content in automated video recommendations (Matamoros-Fernandez et al., 2021) and amendments to the Community Guidelines to prohibit content “about COVID-19 that poses a serious risk of egregious harm” (YouTube Help, 2022a, para. 2). The revised guidelines specify that Covid-19 medical misinformation includes any content that contradicts health authorities’ guidance on Covid-19 treatments, prevention, diagnosis, physical distancing, and the existence of Covid-19. Claims about vaccines that “contradict expert consensus,” including claims that vaccines cause death or infertility, or contain devices used to track or identify individuals, and false claims about the effectiveness of vaccines are all explicitly in violation of YouTube’s Covid-19 medical misinformation guidelines (YouTube Help, 2022a, para. 3).
YouTube states that its Covid-19 medical misinformation rules apply to all content posted to the platform, including video comments. In our study, however, we found comments made against three news videos to be dominated by conspiratorial statements about Covid-19 vaccines in violation of these rules. A significant portion of comments appeared in obvious violation of YouTube’s rules, for example, comments that proposed vaccines are used for mass sterilisation or to insert microchips into recipients. Other comments could be considered “borderline content,” which YouTube defines as content that “brushes up against” but does not cross the lines set by its rules (The YouTube Team, 2019, para. 7). In our data, examples of borderline content include comments that raise doubts about Bill Gates’s motives in vaccine development and distribution and those that suggest that he is seeking to take control in a “new world order.” These comments implied or linked to theories about using vaccines as a means for controlling or tracking large populations of people. Overall, the prevalence of conspiratorial statements about Covid-19 vaccines in our dataset indicates that YouTube’s rules were not well-enforced in the platform’s comments feature at the time of data collection.
Following Marwick and Partin (2022), we suggest that conspiracy theory discussions in YouTube comments have all the characteristic traits of participatory culture, meaning that it is a site of intense collective sense-making and knowledge production. Our results suggest that YouTube’s comments feature can, like anonymous message boards such as 4chan and 8kun, function as an under-regulated epistemic space in which conspiracy theories flourish. Research on Reddit has established the important role of user comments to social processes of knowledge production on platforms, particularly for judgments on information value and credibility (Graham & Rodriguez, 2021). Sundar’s (2008) study of technology effects on credibility found that “when users were attributed as the source of online news, study participants liked the stories more and perceived them to be of higher quality than when news editors or receivers themselves were identified as sources” (p. 83). Sundar and others (see Liao & Mak, 2019; Xu, 2013) have argued this can be explained by the “bandwagon heuristic” in which users’ collective endorsement of content has “powerful influences on publics’ credibility judgments” (Liao & Mak, 2019, p. 3).
The affordances of YouTube’s comments section likely play a significant role in activating the bandwagon heuristic. On YouTube, comments are ranked and displayed according to levels of engagement—that is, according to the number of likes—thus, the comment with the most total likes ranks first. Users can opt to sort and display comments according to date, with the most recent comments at the top; however, the default setting is to rank according to engagement, thereby creating a “voting” function that can signal value and credibility to other users. As Rosenblum and Muirhead (2020) have argued, this may mean that a given YouTube comment appears credible simply because “a lot of people are saying” (p. ix) that it is. This raises important questions about the role of YouTube’s comment liking feature and other algorithmic procedures for sorting content, as they may be exacerbating the kind of “new conspiracism” set out by Rosenblum and Muirhead (2020).
YouTube has acknowledged that the “quality of comments” is frequently raised as a problem by YouTube creators (Wright, 2020). In 2020, the platform introduced a comments filter aimed at automatically identifying and preventing the publication of “inappropriate or hurtful” comments (Wright, 2020). The machine learning classifier that underpins this feature is designed to learn iteratively over time so that it becomes more effective at identifying undesirable comments. Creators can also add specific terms to the list of blocked words (YouTube, 2022b). Our study suggests that it is not working for conspiratorial commentary about Covid-19. To limit the spread of Covid-19 conspiracy theories, this classifier could be trained to identify and exclude from publication comments engaging in Covid-19 medical misinformation.
Automated systems can only go so far, however. Because we found a high prevalence of conspiratorial themes in videos about Bill Gates and Covid-19 (RQ1a), we suggest future policy responses to YouTube’s rampant problem of misinformation in comment sections need to consider how conspiracy theorising functions within the discursive space. We found conspiratorial commenting fits into three top-level functional groups: strengthening a conspiracy theory, discrediting an authority, and defending a conspiracy theory. This is not a wholly novel observation—the same could be said for other platforms that afford conversational interaction through reply threads, notably Reddit. What is different for YouTube is that it almost completely lacks the community-led moderation features that help to mitigate and curb the production and spread of conspiracy theorising and misinformation on Reddit.
Because we found that YouTube commenters use discursive strategies that automated systems are likely to miss (RQ2), our findings suggest that algorithmic approaches to Covid-19 misinformation, such as automated filtering, will not be sufficient to capture the nuance of conspiratorial discourse and the discursive tactics used by conspiracy theorists. For instance, a comment from a new YouTube user that undermines the authority of the U.S. Government National Institute of Allergy and Infectious Diseases is unlikely to be automatically flagged as breaching YouTube’s community guidelines on Covid-19 misinformation, even though it might be clearly part of a conversation thread about Covid denialism. Similarly, we found short conspiratorial statements receive the most engagement (RQ1b), and machine learning text classifiers typically perform poorly on short, ambiguous text inputs (Huang et al., 2013). On Reddit, such a comment might be recognised for the discursive tactic that it is and thereby moderated in a variety of ways, for example: downvoted by other users until it is hidden automatically from view (Graham & Rodriguez, 2021); flagged as “controversial” and de-ranked in terms of visibility; removed by volunteer subreddit moderators; or simply not allowed because the user does not have the minimum credibility (“karma” score) to post comments in that particular subreddit. In severe cases where community-led moderation fails, Reddit may “quarantine” or even ban entire subreddits that become overrun with misinformation.
Our findings support previous studies that argue for understanding disinformation as a collective, socially produced phenomenon. The conspiratorial YouTube comments in our study evoke the notion of “evidence collages” (Krafft & Donovan, 2020), where positive “evidence” filters algorithmically to the top of the thread and is presented to users in a way that affords visibility and participation directly beneath the video. This participatory commenting trades “up the chain” (Marwick & Lewis, 2017, p. 38) through voting practices that increase the visibility of false and misleading narratives to directly under the news publisher’s video. For example, comments that draw attention to Bill Gates’s body language form a multimodal evidence collage beneath the video, inviting others to theorise particular time points in the video and add their own interpretation directly beneath the video in the top comment thread. As Marwick and Clyde (2022) argue, we need to take seriously these tools and interpretive practices of conspiracy theorising. YouTube comment threads afford a participatory mode of knowledge production that actively cultivates an epistemic authority grounded in populist expertise (Marwick & Partin, 2022) and is arguably an important part of the platform’s layer of “disinformation infrastructure” (Pasquetto et al., 2022, Table 3, p. 22).
YouTube critically lacks the social moderation infrastructure that has helped other platforms to deal with misinformation and conspiracy theories that would otherwise spread largely unchecked within the millions of conversations occurring each day. Other platforms have had more success in part because they recognise that the misinformation problem is coextensive with participatory culture, and therefore the design features have evolved to afford community-led moderation that is geared towards the cultures and identity groups that co-exist within the platform. In short, for YouTube to adequately address this problem, it must attend to both the discursive strategies that evade automated detection systems and to redesigning the space to provide users with the tools they need to self-moderate effectively.
For news publishers on YouTube, the platform might look to develop best practice content moderation guidelines for news organisations engaged in publishing content relating to matters of public interest. These guidelines should explain the need for and how to properly moderate comments to ensure their videos do not have the effect of exacerbating conspiracy theory production and circulation. Critically, best practices should be attentive to common discursive strategies conspiracy theorists use and how to look out for them when moderating comment sections. In addition to automated filtering, when posting videos that cover high-stakes public interest matters, news organisations should consider disabling the comments feature unless they are able to commit substantial resources towards manually moderating comments. Ideally, YouTube would provide news content creators with functionality to assess which topics and/or keywords tend to receive high engagement in the comments section. For example, our study shows a common strategy whereby commenters strengthen conspiracy theories by connecting disparate information (“connecting the dots”). This discursive strategy, whilst largely invisible to automated detection systems, would likely stand out to content creators who pore over engagement metrics for the most upvoted (and therefore visible) comments on their videos. However, we also warn against placing too much onus on content creators to bear the responsibility of increasing the “quality” of commentary. To be sure, while empowering creators is useful, it should not displace responsibility solely onto creators. A major implication of our study is that YouTube needs much more effort to redesign the space to provide social moderation infrastructure. Otherwise, the discursive strategies of conspiracy theorists will continue to evade detection systems, pose insurmountable challenges for content creators, and play into the hands of content producers who benefit from and/or encourage such activity.
In early 2022, YouTube announced that it was testing features that would allow YouTube creators to set channel-specific guidelines for comments so that creators can “better shape the tone of conversations on their channel” (Mohan, 2022). At the same time, however, YouTube stated it was also trialing a feature that allows users to sort and view comments timed to relevant points in the video to give “valuable context to comments as they read them” (YouTube, 2020a). If this feature is applied to comments that are not moderated to enforce YouTube’s Covid-19 medical misinformation policies, it could increase the chance of triggering the bandwagon heuristic among YouTube users, further amplifying Covid-19 medical misinformation. Any changes to the comments feature that increase the visibility of or engagement with YouTube comments should be carefully calibrated against both YouTube creator and public interest objectives, and transparently outlined to policymakers and the public.
Findings
Finding 1a: Conspiratorial statements dominate in video comments, with three closely related but distinct thematic clusters.
Topic modelling analysis of the full dataset of comments showed 22 main topics. A manual analysis of a random sample of 50 comments from each of the 22 topics (n = 1,100) revealed that conspiracy theories or conspiratorial statements were present in all 22 topics (Figure 1). These included a range of conspiratorial ideas and narratives about Bill Gates specifically, as well as elements from conspiracy theories not directly related to Bill Gates and information about real-world events.
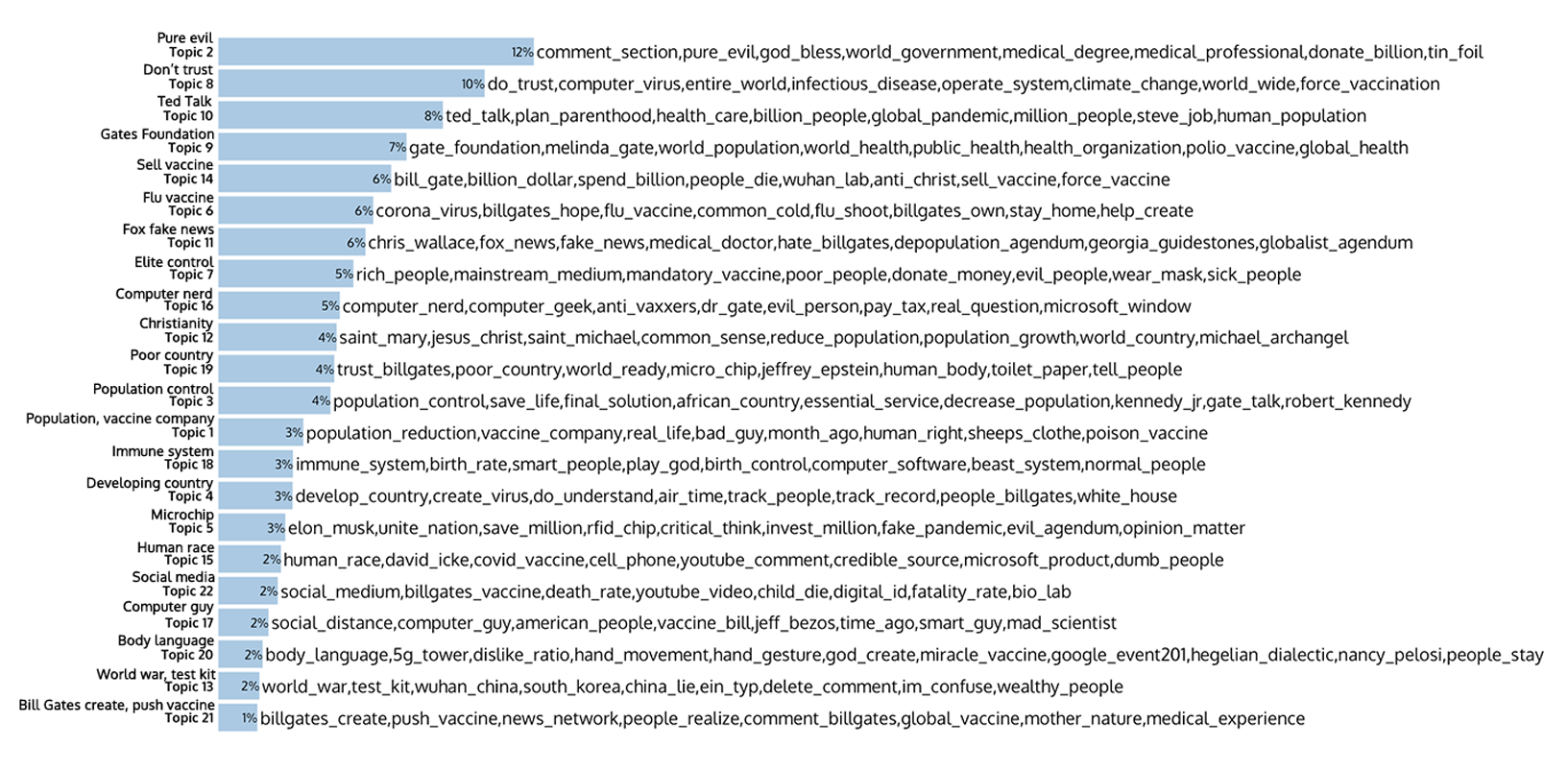
An analysis of the topics through an intertopic distance map, which represents the spread of topics according to the number of comments in each topic and connections between topics, showed three distinct topic clusters (Figure 2). Each cluster is related to conspiracy theories but with slightly divergent themes: Bill Gates as an individual, his role in vaccine development and distribution, and the Bill and Melinda Gates Foundation.
A thematic analysis of the first cluster found it to be dominated by comments alleging that Bill Gates is an evil person with a hidden agenda. Comments proposed that Gates’s body language indicates his malevolent intent (Topic 20) and shared well-known conspiracy sources such as videos about Gates from the Corbett Report (Topic 2). Comments also connected Gates to seemingly unrelated conspiracy theories, such as the theory that Covid-19 is caused by the rollout of 5G networks (Topic 20). Within this cluster, comments also posited a connection between Gates and convicted sex offender Jeffrey Epstein (Topic 19) and proposed Gates is seeking to microchip people through vaccinations (Topic 19). Cryptocurrency mining patent W02020060606 (Topic 2), ID20201See https://id2020.org/overview (Topic 2), and a pandemic simulation exercise2See https://www.centerforhealthsecurity.org/event201/scenario.html (Topic 21) were all connected to the microchipping theory.
The second cluster was dominated by comments about Bill Gates’s motives for vaccine promotion. A common theme was that Gates is motivated by a “depopulation agenda” and comments referred to the United Nations’ 2030 Agenda3See https://sdgs.un.org/2030agenda (Topic 5), Gates’s TED Talk about pandemic preparedness (Topic 10), ID2020 (Topic 8), and sources by Dr. Rashit A. Buttar and Judy Mikovits (Topic 7). The population control theory was interwoven with ideas about Gates’s controlling individuals through microchipping and the “Mark of the Beast” (a physical mark left by the smallpox vaccines) (Topics 1, 15, and 18). Distrust of mainstream media was a sub-theme within this cluster. Comments displayed an anti-institution and anti-elite sentiment in discussions about the effectiveness of Covid-19 vaccines (Topic 6) and in relation to conspiracies about Jeffrey Epstein, Planned Parenthood founder Margaret Sanger (Topic 17), and Chief Medical Advisor to the President of the United States, Anthony Fauci (Topic 7).
Theories about the Bill and Melinda Gates Foundation dominated the third cluster. For example, commenters hypothesised that Gates created the Zika virus for population control in developing countries (Topic 4) and that the foundation seeks to sterilise African women (Topic 14) and paralyse Indian children (Topic 9).
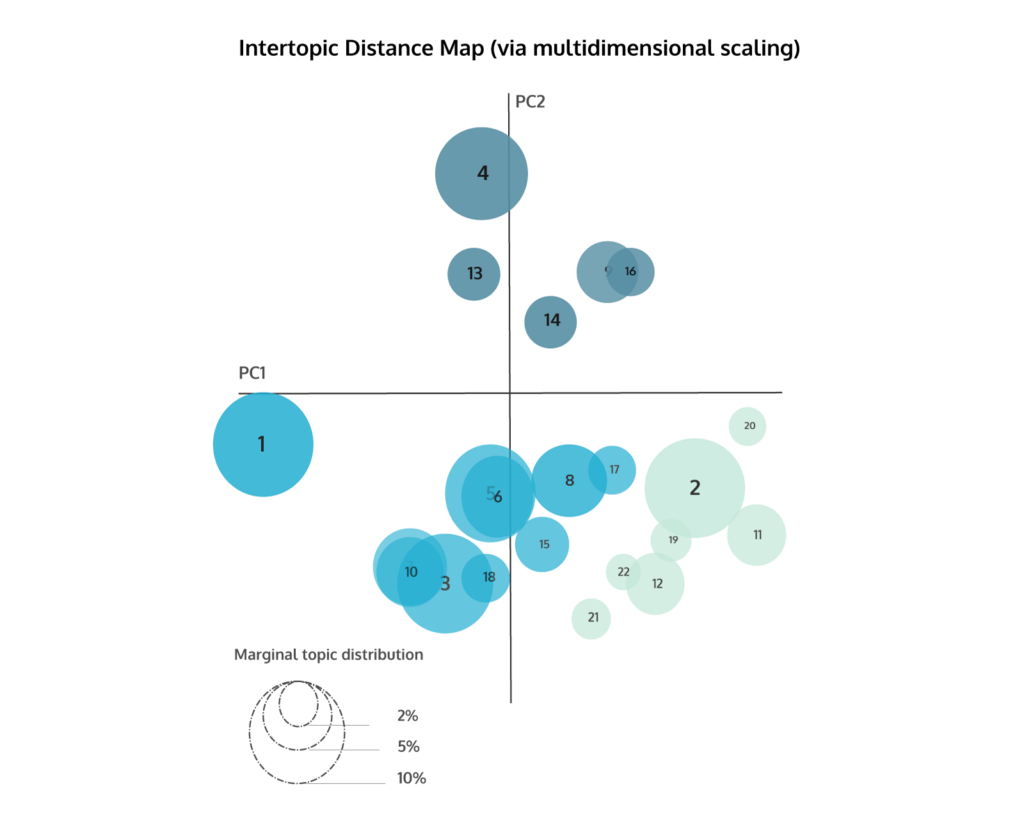
Finding 1b: Comments with the most user engagement tended to be short, general conspiratorial statements.
The number of likes and replies to comments was used to measure public engagement with the ideas put forth in comments. Comments with the highest engagement tended to be general in their assertions and short in length (Figure 3). There was the highest engagement with comments that expressed a general distrust of Bill Gates (Topic 21) and pointed to his body language (Topic 20), and>> as well as previous remarks about pandemic preparation (Topic 19).
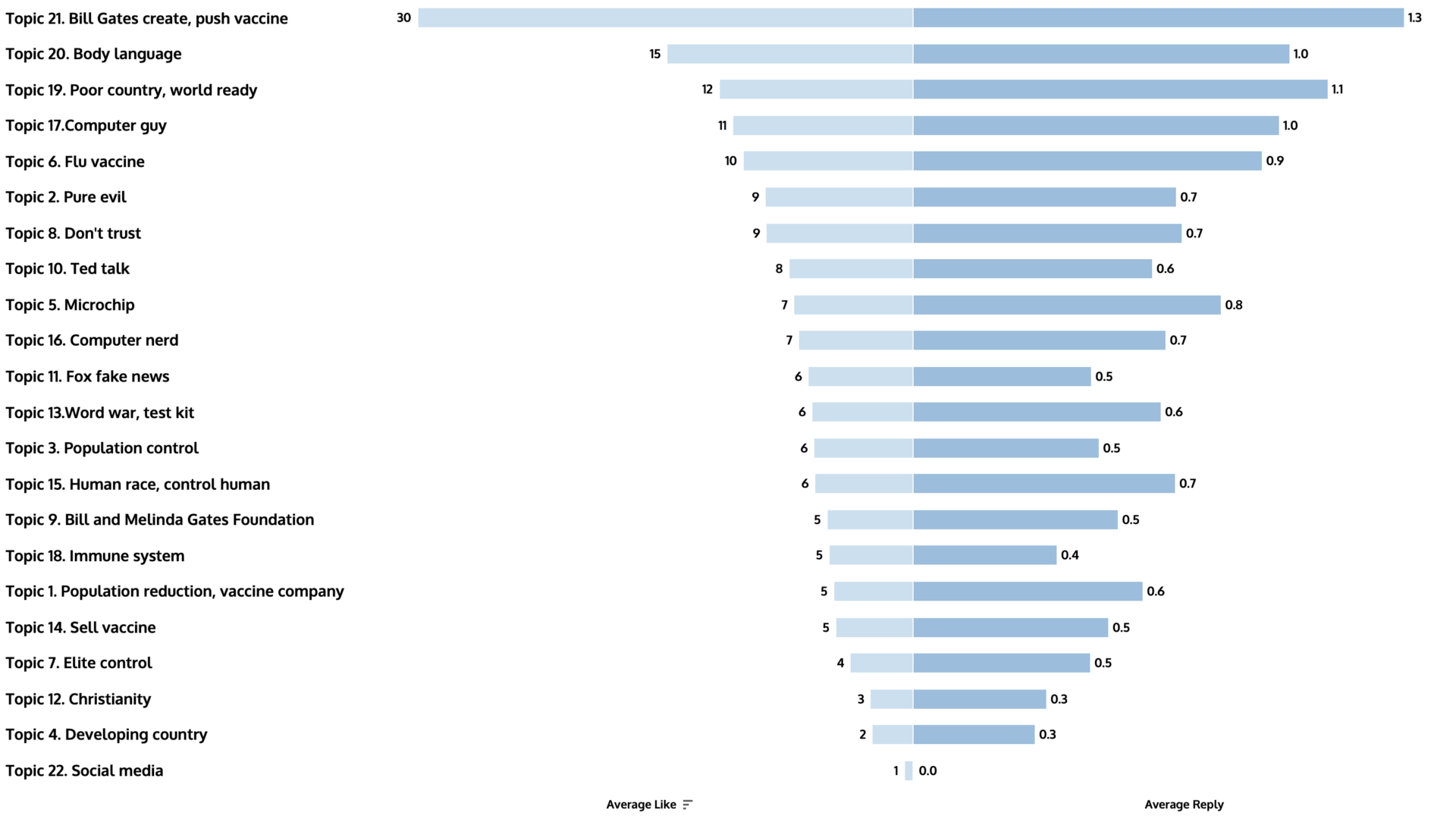
Finding 2: Commenters engaged in discursive practices common to conspiracy theory knowledge production and circulation.
A manual content analysis of the top 3 comments (based on posterior topic probability) from 20 topics (n = 60) was undertaken to identify the discursive strategies used by commenters when sharing conspiratorial statements. We adopted Kou et al.’s (2017) categories of discursive practices to code the sample. These include three top-level functional groups: strengthening a conspiracy theory, discrediting an authority, and defending a conspiracy theory. In our data, as Figure 4 shows, 23% of comments sampled sought to strengthen conspiracy theories by connecting disparate information (“connecting the dots”), 25% made comments that discredited Bill Gates (“casting doubts”), 19% referenced authoritative information selectively, and 35% consisted of a range of strategies that each occurred infrequently (between 2% and 8% of total comments).
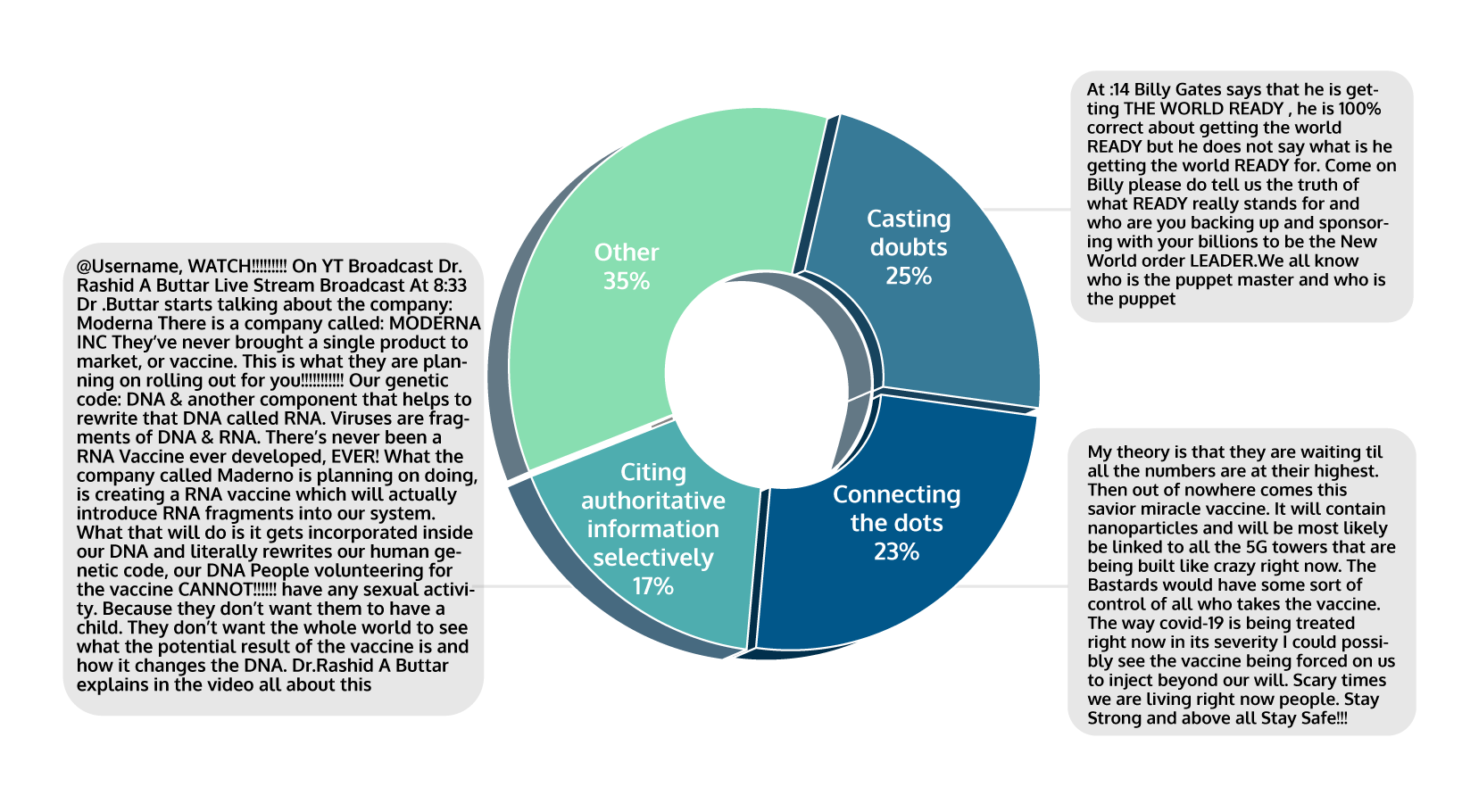
Methods
As outlined at the outset of this paper, our research was driven by two questions:
- RQ1a: What are the dominant conspiratorial themes discussed among YouTube commenters for news videos about Bill Gates and Covid-19?
- RQ1b: Which conspiratorial topics for these news videos attract the most user engagement?
- RQ2: What discursive strategies are YouTube commenters using to formulate and share conspiracy theories about Bill Gates and Covid-19?
To collect the data required to answer all the research questions, YouTube videos were chosen from Fox News, China Global Television Network (CGTN), and Vox to ensure comments were captured from diverse news media outlets. Each video featured Bill Gates prominently, highlighting his views on vaccines and pandemic preparedness. We purposively sampled for videos featuring Bill Gates, given that he is a mainstay of online conspiracy movements (Smith & Graham, 2017; Thomas & Zhang, 2020) and, therefore, of special analytical interest to the field. To extract the comments from the three videos, we employed the open-source tool VosonSML and made use of YouTube’s API. It should be noted that due to the specifications of the API, VosonSML collects a maximum of 100 reply comments for each top-level comment.
The sample size of comments across all three videos (Table 1) far exceeded our ability to manually code each comment, so we used the topic model approach to assist with summarising and thematically organising the large-scale text corpus used to answer the research questions. We applied a latent Dirichlet allocation (LDA) topic model to the corpus of YouTube comments using the STM package in R. Topic modelling helps to summarise large text collections by categorising the documents into a small set of themes or topics. Documents are a mix of topics defined by a probability distribution (e.g., document 1 is generated by topic k with probability p). Likewise, topics are a mix of words defined by a probability distribution (e.g., topic 1 generates the word “apple” with probability p). Topics need to be qualitatively interpreted and labelled by the researcher and topic models require several text processing decisions to be made, depending on the research design and dataset. We processed the YouTube comments data by removing non-English words, special characters, numbers, emojis, and duplicates before converting it into lowercase and representing the data using a bag-of-words model (a matrix with documents as rows, columns as words, and cell values representing the frequency of a given word in each document). We derived the number of topics statistically: the number of topics (the k parameter) was examined stepwise with different values k ∈{8,10,12,14,16,18,20,22,24} and validated through “searchK” function in the STM package.
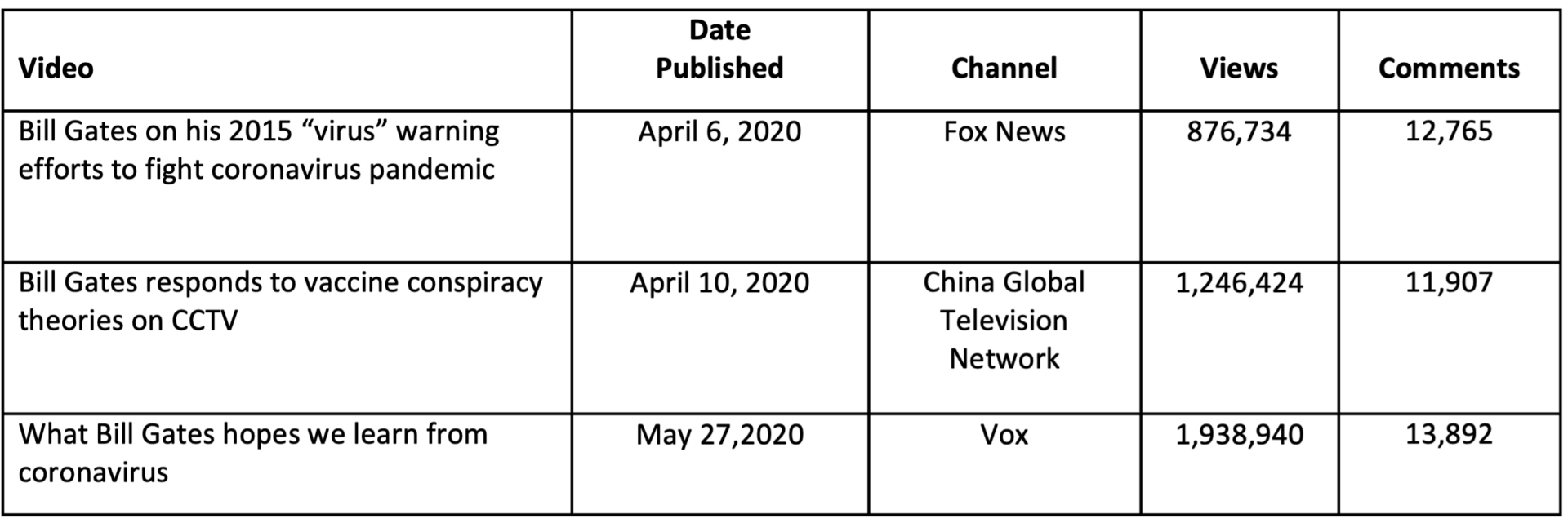
We selected 22 topics based on the exclusivity of words to topics and semantic coherence representing the co-occurrence of most probable words in a given topic. Topics 12 and 22 were excluded from the analysis as they included spam comments and had low affiliation probability (i.e., these are “junk” topics). We identified the two junk topics through our analysis for RQ1 and subsequently omitted these from analysis in RQ2. For RQ1, the first author applied a qualitative coding procedure to label the topics, and the research team discussed the coding schema in regular meetings to ensure reliability. Comments were grouped into topics based on the highest posterior topic probability output by the model for engagement analysis. For RQ2, two coders conducted a manual analysis of n = 60 comments, and inter-rater reliability was calculated at κ = 0.815.
Finally, a limitation of LDA topic modelling is that it is a probabilistic method and, therefore, comments might be misclassified into topics if there is not an “ideal” thematic fit (e.g., comments are an equal mix of topics). While there is invariably an error term in the classifications of documents into topics, we aimed to address validity and reliability issues for RQ2 by qualitatively coding topics via two independent coders and calculating inter-rater reliability. While the method is by no means perfect, we invite other researchers to apply similar approaches in order to test and expand the generalisability of our findings, as well as examine related case studies.
Topics
Bibliography
Bruns, A., Harrington, S., & Hurcombe, E. (2020). ‘Corona? 5G? Or both?’: The dynamics of COVID-19/5G conspiracy theories on Facebook. Media International Australia, 177(1), 12–29. https://doi.org/10.1177/1329878X20946113
Graham, T., & Rodriguez, A. (2021). The sociomateriality of rating and ranking devices on social media: A case study of Reddit’s voting practices. Social Media + Society, 7(3), 20563051211047668. https://doi.org/10.1177/20563051211047667
Huang, S., Peng, W., Li, J., & Lee, D. (2013). Sentiment and topic analysis on social media: A multi-task multi-label classification approach. In Proceedings of the 5th annual ACM web science conference (pp. 172–181). Association for Computing Machinery. https://doi.org/10.1145/2464464.2464512
Khatri, P., Singh, S. R., Belani, N. K., Yeong, Y. L., Lohan, R., Lim, Y. W., & Teo, W. Z. (2020). YouTube as source of information on 2019 novel coronavirus outbreak: A cross sectional study of English and Mandarin content. Travel Medicine and Infectious Disease, 35, 1–6. https://doi.org/10.1016/j.tmaid.2020.101636
Kou, Y., Gui, X., Chen, Y., & Pine, K. (2017). Conspiracy talk on social media: Collective sensemaking during a public health crisis. Proceedings of the ACM on Human-Computer Interaction 61, 1–21. https://doi.org/10.1145/3134696
Krafft, P. M., & Donovan, J. (2020). Disinformation by design: The use of evidence collages and platform filtering in a media manipulation campaign. Political Communication, 37(2), 194–214. https://doi.org/10.1080/10584609.2019.1686094
Liao, M., & Mak, A. (2019). “Comments are disabled for this video”: A technological affordances approach to understanding source credibility assessment of CSR information on YouTube. Public Relations Review, 45(5), 101840. https://doi.org/10.1016/j.pubrev.2019.101840
Marwick, A. E., & Lewis, R. (2017). Media manipulation and disinformation online. Data & Society.
Marwick, A. E., & Partin, W. C. (2022). Constructing alternative facts: Populist expertise and the QAnon conspiracy. New Media & Society. OnlineFirst. https://doi.org/10.1177/14614448221090201
Matamoros-Fernandez, A., Gray, J. E., Bartolo, L., Burgess, J., & Suzor, N. (2021). What’s “up next”? Investigating algorithmic recommendations on YouTube across issues and over time. Media and Communication, 9(4), 234–249. https://doi.org/10.17645/mac.v9i4.4184
Pasquetto, I. V., Olivieri, A. F., Tacchetti, L., Riotta, G., & Spada, A. (2022). Disinformation as Infrastructure: Making and maintaining the QAnon conspiracy on Italian digital media. Proceedings of the ACM on Human-Computer Interaction, 6(CSCW1), 1–31. https://doi.org/10.1145/3512931
Rosenblum, N. L., & Muirhead, R. (2020). A lot of people are saying: The new conspiracism and the assault on democracy. Princeton University Press.
Shahsavari, S., Holur, P., Wang, T., Tangherlini, T. R., & Roychowdhury, V. (2020). Conspiracy in the time of corona: Automatic detection of emerging COVID-19 conspiracy theories in social media and the news. Journal of Computational Social Science, 3(2), 279–317. https://doi.org/10.1007/s42001-020-00086-5
Smith, N., & Graham, T. (2019). Mapping the anti-vaccination movement on Facebook. Information, Communication & Society, 22(9), 1310–1327. https://doi.org/10.1080/1369118X.2017.1418406
Sundar, S. (2008). The MAIN model: A heuristic approach to understanding technology effects on credibility. In M. J. Metzger & A. J. Flanagin (Eds.), Digital media, youth, and credibility (pp.73–100). MIT Press.
The YouTube Team. (December 3, 2019). The four Rs of responsibility, part 2: Raising authoritative content and reducing borderline content and harmful misinformation. YouTube. https://blog.youtube/inside-youtube/the-four-rs-of-responsibility-raise-and-reduce/
Thomas, E., & Zhang, A. (2020). ID2020, Bill Gates and the mark of the beast: How Covid-19 catalyses existing online conspiracy movements. Australian Strategic Policy Institute. http://www.jstor.org/stable/resrep25082
Wright, J. (December 3, 2020). Updates on our efforts to make YouTube a more inclusive platform. YouTube. https://blog.youtube/news-and-events/make-youtube-more-inclusive-platform/
Xu, Q. (2013). Social recommendation, source credibility, and recency: Effects of news cues in a social bookmarking website. Journalism & Mass Communication Quarterly, 90(4), 757–775. https://doi.org/10.1177/1077699013503158
Mohan, N. (February 10, 2022). A look at 2022: Community, collaboration, and commerce. https://blog.youtube/inside-youtube/innovations-for-2022-at-youtube/
YouTube Help. (2022a). COVID-19 medical misinformation policy. YouTube. https://support.google.com/youtube/answer/9891785?hl=en&ref_topic=10833358
YouTube Help. (2022b). Learn about comment settings. YouTube. https://support.google.com/youtube/answer/9483359?hl=en
Funding
The authors disclose receipt of the following financial support: Graham is the recipient of an Australian Research Council DECRA Fellowship (project number DE220101435). YouTube data provided courtesy of Google’s YouTube Data API.
Competing Interests
The authors declare no potential conflicts of interest with respect to the research, authorship, and/or publication of this article.
Ethics
The research protocol employed in this research project was approved by the Queensland University of Technology’s ethics committee for human or animal experiments (approval number 200000475). Human subjects did not provide informed consent.
Copyright
This is an open access article distributed under the terms of the Creative Commons Attribution License, which permits unrestricted use, distribution, and reproduction in any medium, provided that the original author and source are properly credited.
Data Availability
All materials needed to replicate this study are available via the Harvard Dataverse: https://doi.org/10.7910/DVN/WF2BFB
Acknowledgements
All authors acknowledge continued support from the Queensland University of Technology (QUT) through the Digital Media Research Centre.