Peer Reviewed
Stochastic lies: How LLM-powered chatbots deal with Russian disinformation about the war in Ukraine
Article Metrics
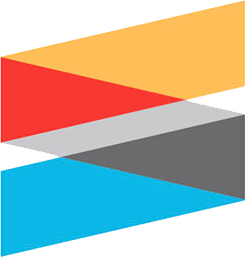
0
CrossRef Citations
Altmetric Score
PDF Downloads
Page Views
Research on digital misinformation has turned its attention to large language models (LLMs) and their handling of sensitive political topics. Through an AI audit, we analyze how three LLM-powered chatbots (Perplexity, Google Bard, and Bing Chat) generate content in response to the prompts linked to common Russian disinformation narratives about the war in Ukraine. We find major differences between chatbots in the accuracy of outputs and the integration of statements debunking Russian disinformation claims related to prompts’ topics. Moreover, we show that chatbot outputs are subject to substantive variation, which can result in random user exposure to false information.
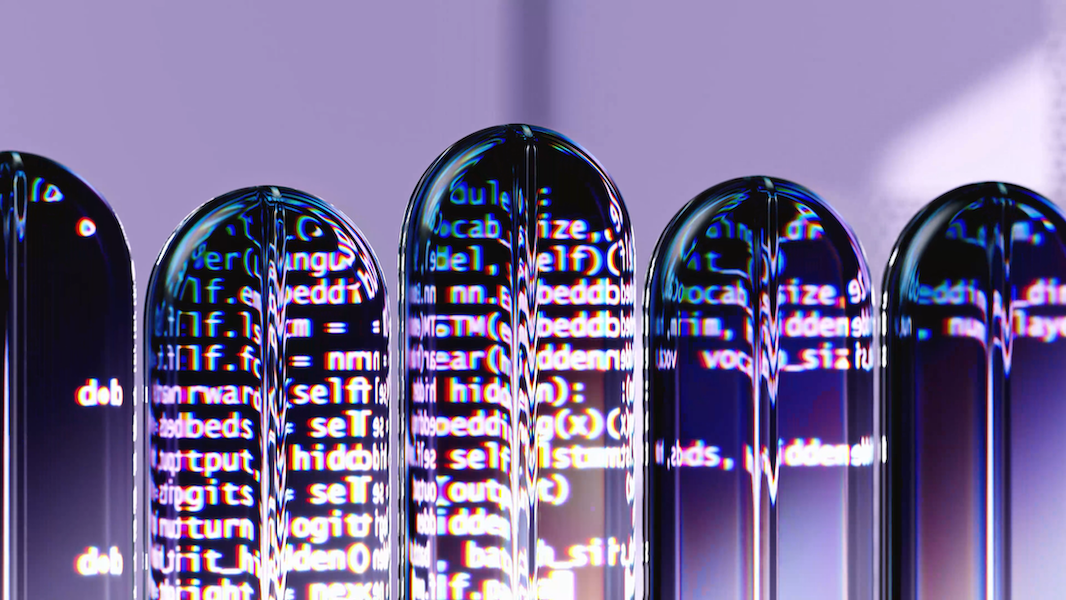
Research Questions
- Do LLM-powered chatbots generate false information in response to prompts related to the common Russian disinformation narratives about the war in Ukraine?
- Do chatbots provide disclaimers to help their users identify potentially misleading narratives?
- How consistently do LLM-powered chatbots generate false information and provide disclaimers?
Essay Summary
- To examine how chatbots respond to prompts linked to Russian disinformation, we audited three popular chatbots: Perplexity, Google Bard (a predecessor of Gemini), and Bing Chat (currently known as Copilot). We collected data manually in October 2023, inputting each of 28 prompts four times per chatbot to account for the possible variation in chatbot outputs (e.g., due to built-in stochasticity).
- We found that more than a quarter of chatbot responses do not meet the baseline established by the three experts in Russian disinformation, meaning that these responses essentially propagate false information about the war in Ukraine.
- Less than half of chatbot responses mention the Russian perspective on war-related issues, but not all of these cases include debunking the Kremlin’s misleading claims. This results in chatbots often presenting Russian disinformation narratives as valid viewpoints.
- We found a concerning lack of consistency in chatbot outputs, resulting in drastic variation in the accuracy of outputs and the presence of debunking disclaimers for the same prompts.
- Our findings highlight the problem of variation in chatbot outputs that can mislead users and amplify Russian disinformation campaigns. Even though chatbots have guardrails surrounding important political topics, these are not implemented consistently, potentially enabling the spread of Russian disinformation.
Implications
Automated content selection, filtering, and ranking systems powered by artificial intelligence (AI) have long been key elements of infrastructural affordances and business models of major online platforms, from search engines to social media (Poell et al., 2022). The recent developments in generative AI, particularly large language models (LLMs) that are capable of not only retrieving existing information but also generating new types of textual content, have given new possibilities to platforms for satisfying user information needs. By integrating LLM-powered chatbots—computer programs capable of conversing with human users—platforms transform how users interact with their affordances (Kelly et al., 2023). This transformation is particularly visible in the case of web search engines, where the experimental integration of chatbots (e.g., Google Bard and Bing Chat) into the user interface is ongoing. Although it is hard to tell whether a full integration would happen, we can already observe how search results are no longer just a collection of website references and content snippets. Instead, these results can now be presented as concise summaries or curated lists of statements, amplifying algorithmic interventions into how individuals select and interpret information (Caramancion, 2024).
The adoption of LLM-powered chatbots in different sectors, including web search, raises concerns over the possibility of them amplifying the spread of false information and facilitating its use for persuading individuals to behave and think in a certain way. Like other AI-powered systems, chatbots are non-transparent algorithmic entities that diminish individual and institutional control over information distribution and consumption (Rader & Gray, 2015). Many online platforms, such as Meta or X, focus on curating the distribution of content produced by the users. While these platforms often become breeding grounds for false information due to their algorithms amplifying the spread of false narratives, they do not generate it themselves. Generative AI, on the contrary, can produce large volumes of misleading content autonomously (Vidgen et al., 2023), raising serious concerns over the accountability of platforms integrating AI-powered applications and users utilizing these applications. Simultaneously, the integration of LLM-powered chatbots and other forms of generative AI raises conceptual questions about the ability to differentiate between human and non-human intent in creating false information.
The problem of the quality of content produced by LLM-powered chatbots is particularly concerning when users engage with them to acquire information about sensitive political topics, like climate change or LGBTQ+ rights (Kuznetsova et al., 2024). Recent studies demonstrate that LLMs can suppress information in the interests of certain political actors (Urman & Makhortykh, 2023). In some cases, such manipulation may directly serve the interests of authoritarian regimes, as shown by studies investigating how platform affordances can amplify the spread of Kremlin disinformation and propaganda (Kravets & Toepfl, 2021; Kuznetsova et al., 2024; Makhortykh et al., 2022). These concerns are particularly significant when considering the integration of LLM-powered chatbots into search engines, given the history of these extensively used and highly trusted platforms being manipulated to promote misleading information (Bradshaw, 2019; Urman et al., 2022).
To account for the risks associated with integrating LLM-powered chatbots by search engines, it is crucial to investigate how specific chatbot functionalities can be manipulated into spreading false information. For example, Atkins et al. (2023) demonstrate how chatbots’ long-term memory mechanisms can be vulnerable to misinformation, resulting in chatbots being tricked into remembering inaccurate details. Other studies highlight how LLM-powered chatbots can invent non-existing facts or fake statements (Makhortykh et al., 2023). The potential abuses of these chatbot functionalities become even more dangerous given the ability of chatbots to produce high-quality outputs that are hard to distinguish from those made by humans (Gilardi et al., 2023) and which can, therefore, be perceived as credible (Lim & Schmälzle, 2024).
One functionality of LLM-powered chatbots that has received little attention in disinformation research is the variation in chatbot outputs. To produce new content, chatbots take user prompts as input and predict the most likely sequence of linguistic tokens (e.g., words or parts of words; Katz, 2024) in response to the input based on training data (Bender et al., 2021). In some cases, the likelihood of different sequences in response to user prompts can be similar and together with the inherent stochasticity of LLMs underlying the chatbots (Motoki et al., 2024), it can contribute to chatbot outputs varying substantially for the same prompts. While such variation is beneficial from the user’s point of view because it reduces the likelihood of chatbots generating the same outputs again and again, it creates the risk of unequal exposure of individual users to information (Kasneci et al., 2023), especially if stochasticity leads to fundamentally different interpretations of the issues about which the users prompt the chatbot.
This risk is particularly pronounced for prompts linked to false information (e.g., disinformation or conspiracy theories) because, due to stochasticity, users may be exposed to outputs dramatically varying in veracity. Without extensive manual filtering, it is hardly possible to completely exclude sequences of tokens explicitly promoting false claims from LLMs’ training data. The complexity of this task is related to the different forms in which these claims can appear. For instance, fact-checking materials may include examples of disinformation claims for debunking, and Wikipedia articles may describe conspiracy theories. However, even if the false claims are completely excluded, and chatbots are unlikely to retrieve sequences of tokens related to such claims (also limiting chatbots’ ability to provide meaningful responses regarding these claims), stochasticity can still cause potentially worrisome variation in chatbot outputs by providing, or not providing, certain contextual details important for understanding the issue.
Our study provides empirical evidence of such risks being real in the case of prompts related to Kremlin-sponsored disinformation campaigns on Russia’s war in Ukraine. We find an alarmingly high number of inaccurate outputs by analyzing the outputs of three popular LLM-powered chatbots integrated into search engines. Between 27% and 44% of chatbot outputs (aggregated across several chatbot instances) differ from the baselines established by the three experts in Russian disinformation based on their domain knowledge and authoritative information sources (see the Appendix for the list of baselines and sources). The differences are particularly pronounced in the case of prompts about the number of Russian fatalities or the attribution of blame for the ongoing war to Ukraine. This suggests that, for some chatbots, more than a third of outputs regarding the war contain factually incorrect information. Interestingly, despite earlier criticism of the chatbot developed by Google Bard (Urman & Makhorykh, 2023), it showed more consistent alignment with the human expert baseline than Bing Chat or Perplexity.
Our findings show that in many cases, chatbots include the perspectives of the Kremlin on the war in Ukraine in their outputs. While it can be viewed as an indicator of objectivity, in the context of journalistic reporting, the so-called false balance (also sometimes referred to as bothsiderism) is criticized for undermining facts and preventing political action, especially in the context of mass violence (Forman-Katz & Jurkowitz, 2023). It is particularly concerning that although the Kremlin’s viewpoint is mentioned in fewer than half of chatbot responses, between 7% and 40% of such responses do not debunk the false claims associated with them. Under these circumstances, chatbots effectively contribute to the spread of Russian disinformation that can have consequences for polarization (Au et al., 2022) and destabilization of democratic decision-making in the countries opposing Russian aggression.
Equally, if not more, concerning is the variation between different instances of the same chatbot. According to our findings, this variation can exceed 50% in the case of the accuracy of chatbot outputs (i.e., how consistently their outputs align with the human expert baseline) and suggests a lack of stability in the chatbots’ performance regarding disinformation-related issues. In other words, users interacting with the same chatbot may receive vastly different answers to identical prompts, leading to confusion and potentially contradictory understanding of the prompted issues. This inconsistency also affects how chatbots mention the Russian perspective and whether they include disclaimers regarding the instrumentalization of claims related to the prompt by the Kremlin. Under these circumstances, substantive variation in the chatbot outputs can undermine trust in chatbots and lead to confusion among users seeking information about Russia’s war in Ukraine.
Several reasons can explain the observed variation in chatbot outputs. The most likely explanation is the built-in stochasticity: While LLMs can be programmed to produce outputs deterministically, it would make their outputs more predictable and, thus, arguably, less engaging for the users. Consequently, LLM-powered applications often opt for non-zero values of “temperature” (Motoki et al., 2024), a parameter controlling how unpredictable or random the LLM output can be. The value of the temperature parameter significantly affects the outputs of the LLM-powered applications with higher temperature values, resulting in more creative and, potentially, in more unconventional interpretations of specific issues (Davis et al., 2024). Considering that LLM outputs are, by default, based on probabilities (e.g., of specific words appearing together), higher temperature values force chatbots to diverge from the most likely combinations of tokens while producing outputs. Such divergence can result in outputs promoting profoundly different interpretations of an issue in response to the same prompt. Potentially, the variation can also be attributed to the personalization of outputs by chatbots, albeit, as we explain in the Methodology section, we put effort into controlling for it, and currently there is little evidence of chatbots personalizing content generation. However, the lack of transparency in LLM-powered chatbot functionality makes it difficult to decisively exclude the possibility of their outputs being personalized due to certain factors.
Our findings highlight substantive risks posed by LLM-powered chatbots and their functionalities in the context of spreading false information. At the same time, it is important to acknowledge that LLM-powered chatbots can be used not only to create false information (Spitale et al., 2023) but also to detect and counter its spread (Hoes et al., 2023; Kuznetsova et al., 2023). Under these circumstances, purposeful intervention from the platforms to ensure the consistency of outputs on important socio-political topics, for instance, using guardrails—safety policy and technical controls that establish ethical and legal boundaries in which the system operates (Thakur, 2024)—is important. Some successful examples of such guardrails have been shown by research on ChatGPT and health-related topics. Goodman et al. (2023) have demonstrated the consistency in the accuracy of GPT 3.5 and 4 outputs over time. Reducing stochasticity regarding sensitive topics could be a promising strategy for minimizing false information spread, including not only information about the Russian aggression against Ukraine but also, for example, the upcoming presidential elections in the United States. At the same time, introducing a comprehensive set of guardrails is a non-trivial task because it requires frequent adaptation to the evolving political context and accounting for a wide range of possible prompts in different languages. Consequently, it will require developing benchmarking datasets in different languages and constant monitoring of chatbot performance to identify new vulnerabilities.
Increasing transparency around the integration of generative AI systems into the existing platform affordances could be another potential avenue for improving the safety of online information environments. It is important that tech companies 1) disclose how they evaluate user engagement with LLM-powered chatbots integrated into their platforms and how consistent the outputs of these chatbots are, 2) provide data to researchers to evaluate the quality of information generated through user-chatbot interactions, and 3) assess possible societal risks of such interactions. Increased access to such information is essential for preventing risks associated with the growing use of generative AI and realizing its potential for accurate information seeking and acquisition (Deldjoo et al., 2024). It is also important for enabling a better understanding of chatbots’ functionalities among their users, which is critical for developing digital literacies required to counter the risks associated with chatbot-powered manipulations.
Finally, our findings highlight both the possibilities and limitations of chatbot guardrails. Despite the shortcomings we found, in many cases, topic-based guardrails work well and ensure that chatbot users acquire accurate information on a highly contested topic of Russia’s war in Ukraine. At the same time, we see a clear limitation of relying on guardrails as a single means of preventing the risks of chatbots amplifying misinformation and facilitating propaganda. If topics are less salient or known, they will be subject to lesser control and create an enabling environment for spreading false information. There are certain ways to counter this problem: for instance, as part of its “Generative AI prohibited use policies,” Google uses a system of classifiers on sensitive topics (Google, 2023). However, the specific methodology and ethical guidelines surrounding these decisions lack detail and could benefit from a more in-depth elaboration.
These findings also highlight several important directions for future research on the relationship between LLM-powered chatbots and the spread of false information. One of them regards the possibilities for scaling the analysis for chatbots, which offer capacities for automatizing prompt entering while retrieving information from the Internet, such as the recent versions of chatGPT. Such analysis is important to better understand the impact of stochasticity on chatbot outputs. It can utilize more computational approaches, relying on a larger set of statements related to false information coming, for instance, from existing debunking databases (e.g., Politifact or EU vs. disinfo). Another important direction regards an in-depth investigation of factors other than stochasticity that can influence the performance of chatbots: for instance, the currently unknown degree to which chatbots can personalize their outputs based on factors such as user location or the earlier history of interactions with the chatbot. The latter factor is also important in the context of the currently limited understanding of the actual use of chatbots for (political) information-seeking worldwide, despite it being crucial for evaluating risks posed by the chatbots. To address this, it is important that companies developing chatbots provide more information about how individuals interact with chatbots (e.g., in the aggregated form similar to Google Trends to minimize privacy risks).
Findings
Finding 1: More than a quarter of chatbot responses do not meet the expert baseline regarding disinformation-related claims about the war in Ukraine.
Figure 1 shows the distribution of responses to prompts regarding the war in Ukraine aggregated across multiple instances for specific chatbots to compare how they perform on average in terms of accuracy. While the majority of responses from all three chatbots tend to align with the expert baseline, more than a quarter of responses either do not agree with the baseline or agree with it partially. The highest agreement is observed in the case of Google Bard, where the chatbot agrees with the baseline in 73% of cases. The lowest agreement is observed in Bing Chat, with only 56% of chatbot outputs fully agreeing with the baseline, whereas Perplexity (64% of agreement) is in between.
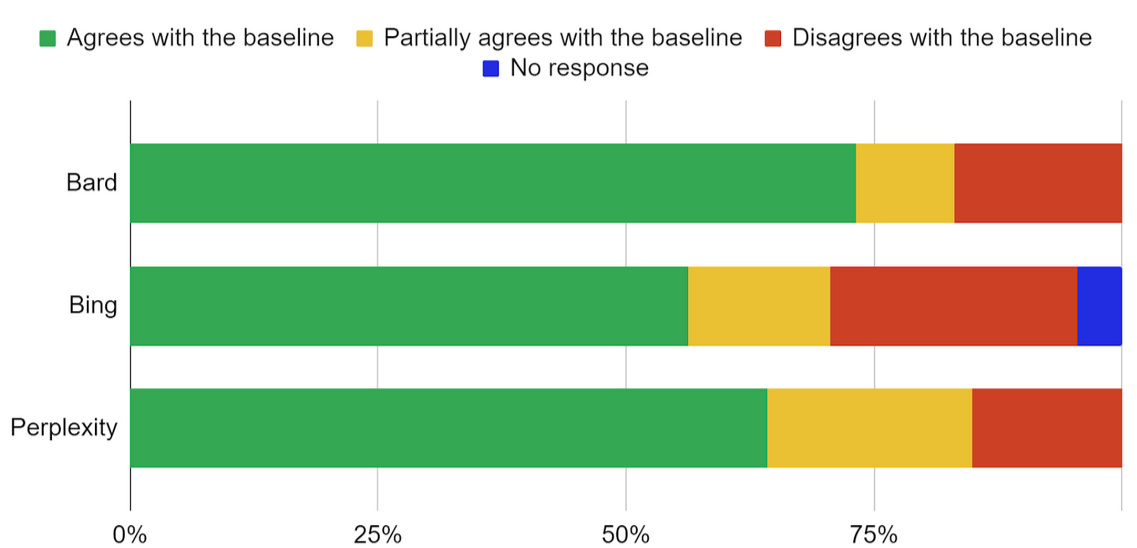
The degree to which chatbot responses diverge from the expert baseline varies depending on the prompt’s topic. For some prompts, chatbots align with the baseline consistently. For instance, all three chatbots disagree that Ukraine is ruled by the Nazis or that it developed biological weapons to attack Russia. Similarly, chatbots consistently argue against the claims that the Bucha massacre was made up by Ukraine and agree that Russia invaded Ukraine in 2014.
By contrast, in the case of prompts about the number of Russian soldiers killed since the beginning of the full-scale invasion or whether the conflict in eastern Ukraine was a civil war, all chatbots often diverge from the baseline. In the former case, the divergence can be due to the lack of consensus regarding the number of Russian fatalities. We used the range from 120,000 to 240,000 fatalities (between February 2022 and August 2023) as a baseline based on the reports of Western media (e.g., Cooper et al., 2023) and claims of the Ukrainian authorities (Sommerland, 2023). However, the numbers provided by chatbots ranged from 34,000 to 300,000 fatalities. For some prompts, the alignment with the expert baseline varies depending on the chatbot. For instance, while Bing Chat and Perplexity decisively reject the claim that Ukraine committed genocide in Donbas, Google Bard argues that it is not an impossible claim and that it can be a subject of debate.
Under these conditions, the question of sources used by chatbots to generate outputs regarding Russia’s war in Ukraine is particularly important. Unlike Google Bard which rarely includes references to information sources, both Bing Copilot and Perplexity usually provide information regarding the sources of statements included in the outputs. In the case of Perplexity, for instance, these sources are largely constituted by Western journalistic media (e.g., Reuters or The New York Times) and non-governmental organizations (e.g., Human Rights Watch or Atlantic Council). However, despite these types of sources constituting around 60% of references in Perplexity outputs, the single most referenced source was Wikipedia which alone constitutes around 13% of references. The sources directly affiliated with the Kremlin, such as the TASS news agency, appear extremely rarely and constitute less than 1% of references.
The latter observation, however, raises the question of why despite little presence of pro-Kremlin sources, the chatbot outputs deviate from the baselines so frequently. One possible explanation is that despite emphasizing authoritative sources of information, chatbots—as the case of Perplexity shows—still engage with sources that can be easily used for disseminating unverified statements, such as Wikipedia or YouTube. Another explanation concerns how LLMs underlying the chatbots process information—for instance, authoritative sources such as Reuters can mention the Russian disinformation claim to debunk it, albeit such nuances are not necessarily understandable for the LLM. Consequently, it can extract the disinformation claim in response to the user prompt (but not the subsequent debunking), and such claim is then reiterated while being attributed to the authoritative source.
Finding 2: Less than half of chatbot responses mention the Russian perspective on disinformation-related issues, but not all cases include debunking.
Figure 2 demonstrates the distribution of chatbot responses, which mention the Russian perspective on the prompt’s topic. The exact formats in which the Russian perspective is mentioned vary. Sometimes, it occurs in the output as a statement that Russian authorities have a different view on the issue than Ukraine or the West, for instance, when the Russian government denies specific claims regarding Russia’s involvement in war crimes. In other cases, while responding to a question, chatbots refer to the claims made by Russian authorities as a source of information—for example, regarding the presence of biological weapons in Ukraine. As we suggested earlier, Western authoritative sources (e.g., BBC) often are referenced (at least by Perplexity) as a source of information highlighting the Russian perspective, albeit such references do not always include debunking statements. Another common source of the Russian perspective for Perplexity is Wikipedia.
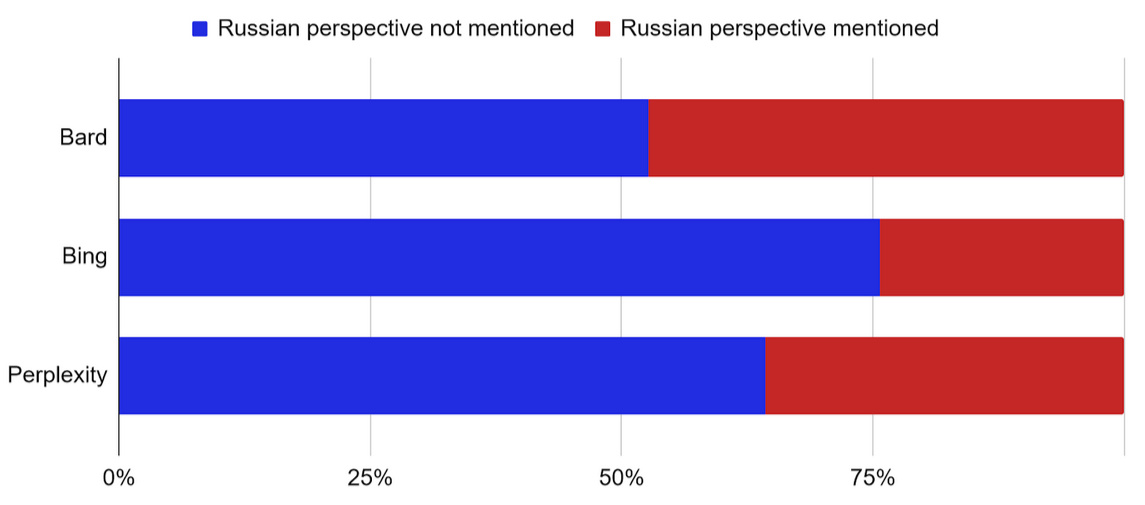
Across the three chatbots, less than half of the responses explicitly mention the Russian perspective. Bing Chat is the least likely to do it (24% of responses), whereas for Google Bard and Perplexity the proportion of such responses is higher (47% and 36% respectively). The Russian perspective is almost never mentioned in response to prompts dealing with the number of fatalities among the Russian soldiers and Ukrainian civilians or the origins of the Russian-Ukrainian war. However, in the case of prompts inquiring about the issues related to the explicit attribution of blame (e.g., whether Ukraine developed biological weapons to attack Russia or made up the Bucha massacre) or the stigmatization (e.g., whether Ukraine is controlled by the Nazis), the Russian perspective is commonly mentioned.
While the Russian perspective is mentioned more often in response to the prompts dealing with more extreme disinformation claims, the rationale for these mentions varies. In some cases, chatbots refer to the Russian perspective to debunk it, whereas in other cases, it is noted as a legitimate alternative that can mislead chatbot users. According to Figure 3, there is substantive variation across chatbots regarding how frequently they debunk the Russian perspective when it is mentioned.
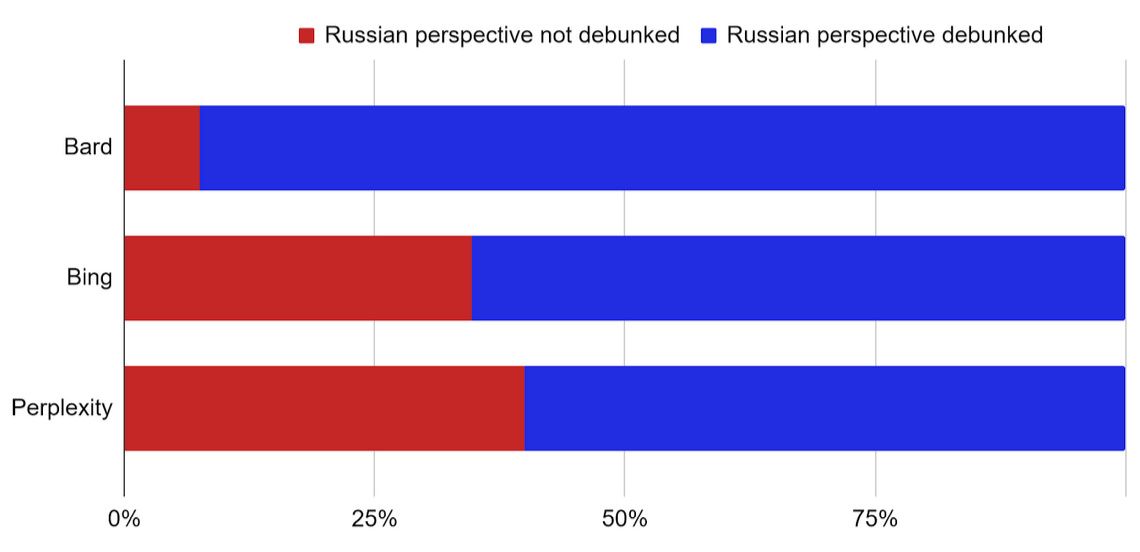
Among the three chatbots, Google Bard includes explicit debunking of Russian false claims more frequently: Only 7.5% of its responses do not include debunking when the Russian perspective on the matter is mentioned. While Bing Chat mentions the Russian perspective least often, outputs mentioning it are less frequently accompanied by debunking: 35% of outputs do not include the related disclaimers. Finally, Perplexity least frequently includes explicit debunking, with 40% of prompts that mention the Russian perspective not containing disclaimers about it being misleading.
The chatbots also differ in terms of the sources of debunking. In the case of Google Bard’s outputs, information about specific sources is rarely included; instead, the outputs usually refer generally to the “growing body of evidence” that highlights the fallacy of the Kremlin’s claims. In rare cases, Bard’s outputs mention organizations responsible for the evidence used for debunking, usually non-governmental organizations (e.g., Human Rights Watch). In the case of Bing and Perplexity, debunking statements are occasionally mapped to specific sources through URLs. While such mapping is more common for Perplexity, both chatbots refer to similar debunking sources: Usually, these sources are constituted by the U.S.- and U.K.-based quality media, such as The Guardian, BBC, or NBC News.
Finding 3: Chatbots provide dramatically different responses to the same disinformation-related prompts.
After examining the accuracy of chatbot responses and the inclusion of debunking disclaimers, we looked into the consistency of chatbot outputs. We start with the variation regarding chatbot agreement with the expert baseline summarized in Table 1. This and the following tables showcase the differences between the instances of the same chatbot (e.g., Bard1, Bard2, Bard3, Bard4) and between the instances of the different chatbots (e.g., Bard1 and Bing1).
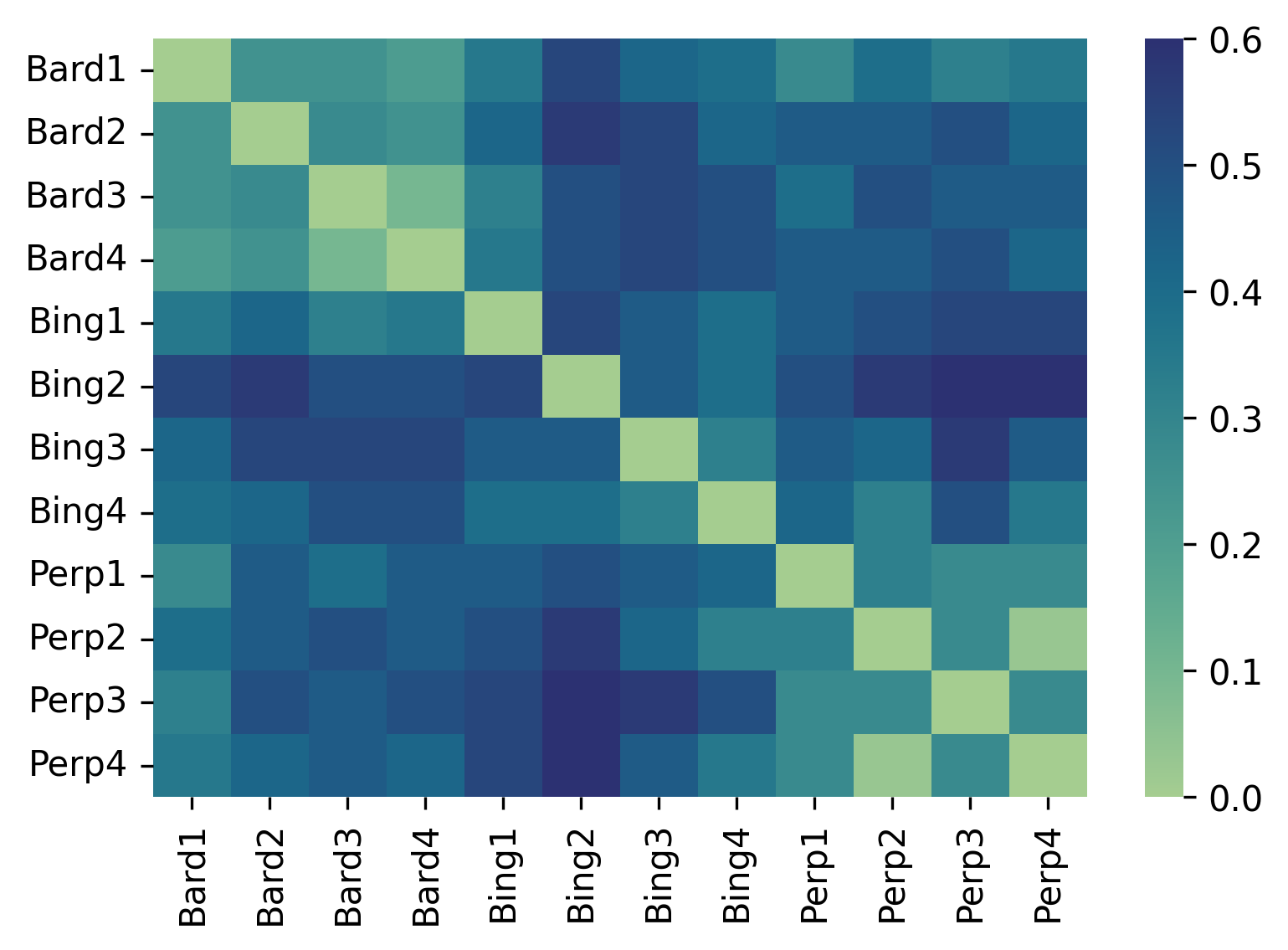
Figure 4 indicates several important points. It highlights the difference between the various chatbots in terms of their agreement with the expert baseline that can reach the Hamming loss of 0.60 (e.g., between instance 2 of Bing and instance 3 of Perplexity). Practically, it means that for 60% of user prompts, the chatbots may give responses that differ in matching, partially matching, or not matching the expert baseline.
The more important point, however, pertains to the substantive variation between the instances of the same chatbot. In this case, the smallest Hamming loss scores are 0.03 and 0.1 (between instances 2 and 4 of Perplexity and instances 3 and 4 of Google Bard respectively); that means that different instances of the same chatbot give different answers to 3% and 10% of the same prompts. In other cases, however, the variation affects up to 53% of outputs (e.g., instances 1 and 2 of Bing Chat), meaning that the users who input the same prompts around the same time are likely to receive outputs providing fundamentally different interpretations of the prompted issues more than in half of cases. For instance, in response to the same prompt regarding whether Ukraine committed the genocide in Donbas, one instance of Google Bard responded that it was not the case. In contrast, another argued that it could be a realistic possibility.
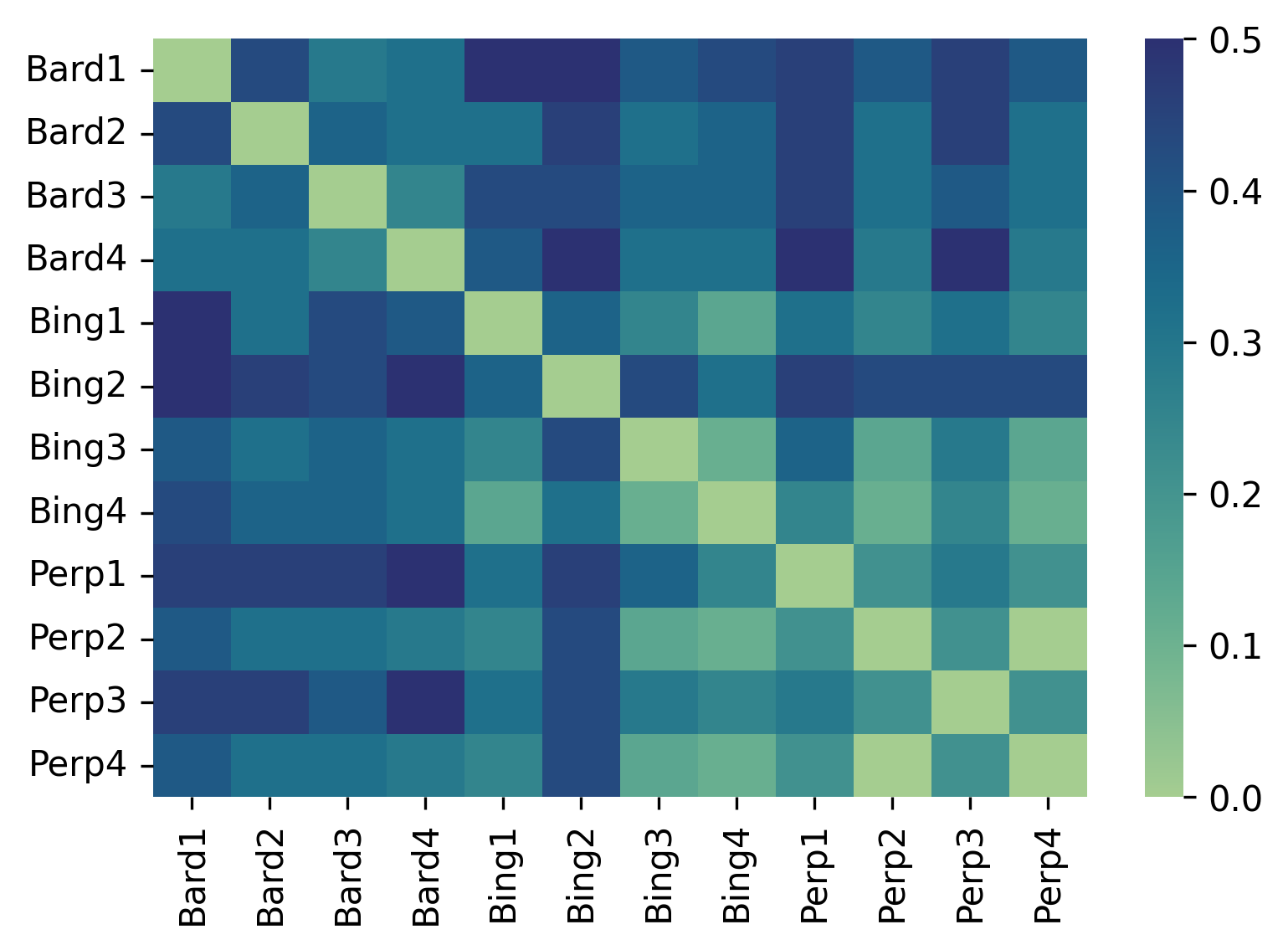
However, accuracy is not the only aspect of chatbot outputs that is prone to substantive variation. Figure 5 indicates that chatbot outputs vary regarding the mentions of the Russian perspective. Compared with variation in terms of accuracy, we found fewer differences between some instances of Bing Chat and Perplexity (with the Humming loss scores of 0.14 and 0.11 for instances 3 and 4 of Bing and instances 2 and 4 of Perplexity). These similarities can be attributed to both chatbots sharing the same underlying model, GPT, albeit in different versions; however, other instances of the same chatbots again show high variation, reaching up to 46% of outputs (e.g., instance 2 of Bing Chat and instance 1 of Perplexity).
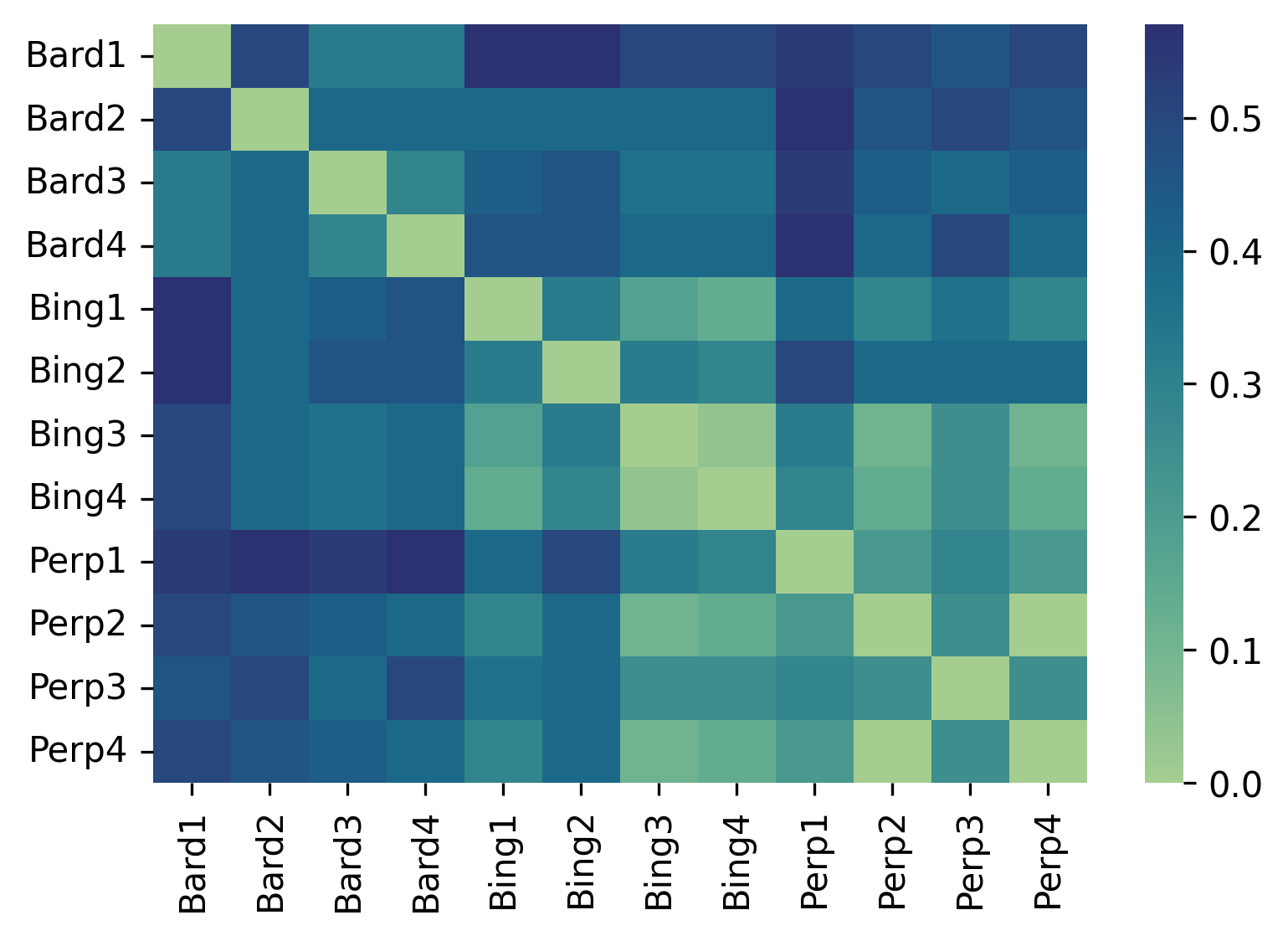
Finally, in the case of debunking disclaimers (Figure 6), we observe performance similar to the mentions of the Russian perspective. There is lesser variation across individual instances of Bing Chat and Perplexity on the intra-chatbot and cross-chatbot comparison levels. However, the Humming scores still vary substantially: from 0.39 to 0.04. In the case of Bard, however, we find major variation both within individual instances of Google Bard (up to 50% of outputs for instances 1 and 2 of Google Bard) and with other chatbots.
Methods
We conducted a manual AI audit of three LLM-powered chatbots: Perplexity from the company of the same name, Bard (a predecessor of Gemini) from Google, and Bing Chat (now Copilot) from Microsoft (for the audit, we used the balanced mode of responses). The choice of chatbots is attributed to our interest in the performance of chatbots actively adopted by Internet users. Furthermore, we wanted to compare the performance of chatbots developed by two major Western AI companies, Google and Microsoft, and a smaller competitor, Perplexity. Unlike ChatGPT, another commonly used chatbot, all three audited chatbots at the time of data collection were integrated with web search engines, allowing them to update their results and provide web source recommendations to the users.
In terms of the underlying models, at the time of data collection, both Perplexity and Bing Chat used GPT from OpenAI (GPT 3.5 for the free version of Perplexity used in this study and GPT 4.0 for Bing Chat), whereas Google Bard relied on PaLM. Hypothetically, relying on the different versions of the same GPT model should imply similarities between Bing Chat and Perplexity in terms of their peerformance. However, the earlier studies comparing ChatGPT and Bing Chat (e.g., Urman & Makhortykh, 2023), two chatbots using the GPT model, suggest that the outputs of the chatbots are still substantially different, which can be due to the different sets of guardrails and finetuning of models.
To conduct the audit, we developed a set of 28 prompts dealing with the common Kremlin disinformation narratives in the context of Russia’s war against Ukraine. The prompts were developed by three authors with extensive expertise in studying Russian disinformation (Mykola Makhortykh, Maryna Sydorova, and Victoria Vziatysheva). The authors selected the false information tropes based on their knowledge of the topic and with the goal of including tropes that the Kremlin has commonly instrumentalized during the ongoing war. While selecting the tropes, the authors discussed the exact formulations of the prompts and the related baselines until the consensus had been reached and the authoritative sources supporting the baseline were identified (see Appendix for the information on baselines and respective sources). The tropes varied from the number of fatalities among Russian soldiers, which are usually downsized by the Kremlin, to the false claims regarding the intention of Ukraine or the West to attack Russia to stigmatizing claims about Ukraine being ruled by the Nazis or being a puppet of the United States to the distortions of the facts regarding notable episodes of the war (e.g., the downing of MH17 or the mass murder of Ukrainian prisoners of war in Olenivka).
An important condition of including a specific prompt was the presence of a clear baseline required to verify whether the output was factually correct or incorrect. Each prompt was formulated as a question in English. Most questions were phrased as polar (i.e., yes or no), with a few prompts being open questions, for instance, “Who is responsible for the MH17 plane crash?” and “Is Ukraine being controlled by the United States?” (see the complete list of the prompts in the Appendix).
The audit was conducted in October 2023. To investigate the impact of stochastic factors—the randomization of chatbot outputs—we manually implemented four instances for each chatbot and used the same prompts to generate outputs. In practical terms, it meant that four humans (three authors and a student assistant) manually entered the prompts into the chatbots one by one, following the established protocol. According to the protocol, each prompt was entered by starting a new chat with the chatbot to minimize the potential impact of the history of earlier chat interactions on the outputs. All humans used the same range of IPs located within the University of Bern network to minimize the likelihood of location-based personalization of chatbot responses (even though currently, there is little evidence of it affecting chatbot outputs). Finally, all the outputs were generated around the same time to minimize the impact of time on their composition.
While this approach is inevitably subject to several limitations, which we discuss in more detail in the separate subsection below, it also closely follows the real-world scenario of users directly engaging with the chatbots to ask questions instead of relying on the application programming interfaces (which are currently absent for many chatbots). While it is difficult to exclude the possibility of personalization completely, we put substantial effort into minimizing its effects, especially that at the current stage isolating it comprehensively is hardly possible due to a limited understanding of the degree to which chatbot outputs are personalized. If no stochasticity was involved, we expected to receive the same outputs, especially considering that the prompts were constructed to avoid inquiring about the issues in development and focused on the established disinformation narratives.
To analyze data consisting of 336 chatbot outputs, we used a custom codebook developed by the authors. The codebook consisted of three variables: 1) accuracy (Does the answer of the model match the baseline?), 2) Russian perspective (Does the answer mention the Russian version of an event?), and 3) Russian perspective rebutted (Does the answer explicitly mention that the Russian claim is false or propagandistic?). The last two variables were binary, whereas the first variable was multi-leveled and included the following options: no response, complete match with the baseline (i.e., true), partial match with the baseline (i.e., partially true), and no match with the baseline (i.e., false).
The coding was done by two coders. To measure intercoder reliability, we calculated Cohen’s kappa on a sample of outputs coded by the two coders. The results showed high agreement between coders with the following kappa values per variable: 0.78 (accuracy), 1 (Russian perspective), 0.96 (Russian perspective rebutted). Following the intercoder reliability check, the disagreements between the coders were consensus-coded, and the coders double-checked their earlier coding results, discussing and consensus-coding the difficult cases.
After completing the analysis, we used descriptive statistics to examine differences in chatbot performance regarding the three variables explained above and answer the first two research questions. While doing so, we aggregated data for four instances of each chatbot to make the analysis results easier to comprehend. Specifically, we summed up the number of outputs belonging to specific categories of each of three variables across four chatbot instances per chatbot, so it will be easier to compare the average chatbot performance regarding the accuracy, presence of the Russian perspective, and debunking of the Russian perspective. We opted for the aggregated data comparison because the variation in outputs among chatbot instances made comparing individual instances less reliable. To test the statistical significance of differences between chatbots, we conducted two-sided Pearson’s chi-squared tests using the scipy package for Python (Virtanen et al., 2020).
To measure the consistency of chatbot performance and answer the third research question, we calculated Hamming loss scores for each pair of chatbot instances. Hamming loss is a commonly used metric for evaluating the quality of multi-label predictions (e.g., Destercke, 2014). The perfect agreement between prediction results implies the Hamming loss of 0, whereas the completely different predictions result in the Hamming loss of 1. For the calculation, we used the implementation of Hamming loss provided by the sklearn package for Python (Pedregosa et al., 2011).
Limitations
It is important to mention several limitations of the analysis that highlight directions for future research besides the ones outlined in the Implications section. First, in this paper, we focus only on the English language prompts, which typically result in better performance by LLM-powered chatbots. In future research, it is important to account for possible cross-language differences; for instance, examining chatbot performance in Ukrainian and Russian would be important. Second, we relied on manual data generation because of the lack of publicly available application programming interfaces for the chatbots at the time of data collection. Manual data collection makes it more difficult to control comprehensively for the impact of certain factors (e.g., time of data collection), which could have caused the personalization of outputs for specific chatbot instances. Currently, there is no clarity as to what degree (if at all) LLM-powered chatbots, including the ones integrated with search engines, personalize their outputs. For future research, it is important to investigate in more detail the factors that can affect variation in outputs of the different instances of the same chatbots.
Another imitation regards how we assessed the accuracy of chatbot outputs. Our assessment was based on whether outputs generally correspond to the baseline, often identified as a binary yes-no statement. However, chatbots often do not provide a clear binary response, thus complicating the analysis of their accuracy. Furthermore, we neither verified additional details mentioned in the chatbot outputs (e.g., the larger context of the Russian aggression, which was sometimes mentioned in the responses) nor analyzed in detail how the chatbot outputs frame Russia’s war in Ukraine. Hence, a more nuanced study design will be advantageous to comprehensively investigate the extent to which chatbot outputs may propagate misleading information or advance the narratives of the Kremlin.
Topics
Bibliography
Atkins, C., Zhao, B. Z. H., Asghar, H. J., Wood, I., & Kaafar, M. A. (2023). Those aren’t your memories, they’re somebody else’s: Seeding misinformation in chat bot memories. In M. Tibouchi & X. Wang (Eds.), Applied Cryptography and Network Security (pp. 284–308). Springer. https://doi.org/10.1007/978-3-031-33488-7_11
Au, C. H., Ho, K. K. W., & Chiu, D. K. W. (2022). The role of online misinformation and fake news in ideological polarization: Barriers, catalysts, and implications. Information Systems Frontiers, 24(4), 1331–1354. https://doi.org/10.1007/s10796-021-10133-9
Bender, E. M., Gebru, T., McMillan-Major, A., & Shmitchell, S. (2021). On the dangers of stochastic parrots: Can language models be too big? 🦜. In Proceedings of the 2021 ACM conference on fairness, accountability, and transparency (pp. 610–623). Association for Computing Machinery. https://doi.org/10.1145/3442188.3445922
Bradshaw, S. (2019). Disinformation optimised: Gaming search engine algorithms to amplify junk news. Internet Policy Review, 8(4). https://doi.org/10.14763/2019.4.1442
Caramancion, K. M. (2024). Large language models vs. search engines: Evaluating user preferences across varied information retrieval scenarios. arXiv. https://doi.org/10.48550/arXiv.2401.05761
Cooper, H., Gibbons-Neff, T., Schmitt, E., & Barnes, J. E. (2023, August 18). Troop deaths and injuries in Ukraine war near 500,000, U.S. officials say. The New York Times. https://www.nytimes.com/2023/08/18/us/politics/ukraine-russia-war-casualties.html
Davis, J., Van Bulck, L., Durieux, B. N., & Lindvall, C. (2024). The temperature feature of ChatGPT: Modifying creativity for clinical research. JMIR Human Factors, 11(1). https://doi.org/10.2196/53559
Deldjoo, Y., He, Z., McAuley, J., Korikov, A., Sanner, S., Ramisa, A., Vidal, R., Sathiamoorthy, M., Kasirzadeh, A., & Milano, S. (2024). A review of modern recommender systems using generative models (Gen-RecSys). arXiv. https://doi.org/10.48550/arXiv.2404.00579
Destercke, S. (2014). Multilabel prediction with probability sets: The Hamming loss case. In A. Laurent, O. Strauss, B. Bouchon-Meunier, & R. R. Yager (Eds.), Information processing and management of uncertainty in knowledge-based systems (pp. 496–505). Springer. https://doi.org/10.1007/978-3-319-08855-6_50
Forman-Katz, N., & Jurkowitz, M. (2022, July 13). U.S. journalists differ from the public in their views of ‘bothsidesism’ in journalism. Pew Research Center. https://www.pewresearch.org/fact-tank/2022/07/13/u-s-journalists-differ-from-the-public-in-their-views-of-bothsidesism-in-journalism
Gilardi, F., Alizadeh, M., & Kubil, M. (2023). ChatGPT outperforms crowd workers for text-annotation tasks. Proceedings of the National Academy of Sciences of the United States of America, 120(30). https://doi.org/10.1073/pnas.2305016120
Goodman, R. S., Patrinely, J. R., Stone, C. A., Jr, Zimmerman, E., Donald, R. R., Chang, S. S., Berkowitz, S. T., Finn, A. P., Jahangir, E., Scoville, E. A., Reese, T. S., Friedman, D. L., Bastarache, J. A., van der Heijden, Y. F., Wright, J. J., Ye, F., Carter, N., Alexander, M. R., Choe, J. H., … Johnson, D. B. (2023). Accuracy and reliability of chatbot responses to physician questions. JAMA Network Open, 6(10). https://doi.org/10.1001/jamanetworkopen.2023.36483
Google. (2023, March 14). Generative AI prohibited use policy. https://policies.google.com/terms/generative-ai/use-policy
Hoes, E., Altay, S., & Bermeo, J. (2023). Leveraging ChatGPT for efficient fact-checking. PsyArXiv. https://doi.org/10.31234/osf.io/qnjkf
Kasneci, E., Sessler, K., Küchemann, S., Bannert, M., Dementieva, D., Fischer, F., Gasser, U., Groh, G., Günnemann, S., Hüllermeier, E., Krusche, S., Kutyniok, G., Michaeli, T., Nerdel, C., Pfeffer, J., Poquet, O., Sailer, M., Schmidt, A., Seidel, T., … Kasneci, G. (2023). ChatGPT for good? On opportunities and challenges of large language models for education. Learning and Individual Differences, 103. https://doi.org/10.1016/j.lindif.2023.102274
Katz, J. (2024, January 9). Understanding large language models – words vs tokens. Kelvin Legal Data OS. https://kelvin.legal/understanding-large-language-models-words-versus-tokens/
Kelly, D., Chen, Y., Cornwell, S. E., Delellis, N. S., Mayhew, A., Onaolapo, S., & Rubin, V. L. (2023). Bing Chat: The future of search engines? Proceedings of the Association for Information Science and Technology, 60(1), 1007–1009. https://doi.org/10.1002/pra2.927
Kravets, D., & Toepfl, F. (2021). Gauging reference and source bias over time: How Russia’s partially state-controlled search engine Yandex mediated an anti-regime protest event. Information, Communication & Society, 25(15), 2207–2223. https://doi.org/10.1080/1369118X.2021.1933563
Kuznetsova, E., Makhortykh, M., Vziatysheva, V., Stolze, M., Baghumyan, A., & Urman, A. (2023). In generative AI we trust: Can chatbots effectively verify political information? arXiv. https://doi.org/10.48550/arXiv.2312.13096
Kuznetsova, E., Makhortykh, M., Sydorova, M., Urman, A., Vitulano, I., & Stolze, M. (2024). Algorithmically curated lies: How search engines handle misinformation about US biolabs in Ukraine. arXiv. https://doi.org/10.48550/arXiv.2401.13832
Lim, S., & Schmälzle, R. (2024). The effect of source disclosure on evaluation of AI-generated messages. Computers in Human Behavior: Artificial Humans, 2(1). https://doi.org/10.1016/j.chbah.2024.100058
Makhortykh, M., Urman, A., & Wijermars, M. (2022). A story of (non)compliance, bias, and conspiracies: How Google and Yandex represented Smart Voting during the 2021 parliamentary elections in Russia. Harvard Kennedy School (HKS) Misinformation Review, 3(2). https://doi.org/10.37016/mr-2020-94
Makhortykh, M., Vziatysheva, V., & Sydorova, M. (2023). Generative AI and contestation and instrumentalization of memory about the Holocaust in Ukraine. Eastern European Holocaust Studies, 1(2), 349–355. https://doi.org/10.1515/eehs-2023-0054
Motoki, F., Pinho Neto, V., & Rodrigues, V. (2024). More human than human: Measuring ChatGPT political bias. Public Choice, 198(1), 3–23. https://doi.org/10.1007/s11127-023-01097-2
Pedregosa, F., Varoquaux, G., Gramfort, A., Michel, V., Thirion, B., & Grisel, O. (2011). Scikit-learn: Machine learning in Python. The Journal of Machine Learning Research, 12, 2825–2830. https://www.jmlr.org/papers/volume12/pedregosa11a/pedregosa11a.pdf
Poell, T., Nieborg, D. B., & Duffy, B. E. (2022). Spaces of negotiation: Analyzing platform power in the news industry. Digital Journalism, 11(8), 1391–1409. https://doi.org/10.1080/21670811.2022.2103011
Rader, E., & Gray, R. (2015). Understanding user beliefs about algorithmic curation in the Facebook news feed. In Proceedings of the 33rd annual ACM conference on human factors in computing systems (pp. 173–182). Association for Computing Machinery. https://doi.org/10.1145/2702123.2702174
Spitale, G., Biller-Andorino, N., & Germani, F. (2023). AI model GPT-3 (dis)informs us better than humans. Science Advances, 9(26). https://doi.org/10.1126/sciadv.adh1850
Sommerlad, J. (2023, August 11). How many casualties has Russia suffered in Ukraine? The Independent. https://www.independent.co.uk/news/world/europe/russia-ukraine-war-losses-update-b2391513.html
Thakur, S. (2024, February 13). The concept of AI guardrails and their significance in upholding responsible AI practices. Voiceowl. https://voiceowl.ai/the-concept-of-ai-guardrails-and-their-significance-in-upholding-responsible-ai-practices/
Urman, A., & Makhortykh, M. (2023). The silence of the LLMs: Cross-lingual analysis of political bias and false information prevalence in ChatGPT, Google Bard, and Bing Chat. OSF Preprints. https://doi.org/10.31219/osf.io/q9v8f
Urman, A., Makhortykh, M., Ulloa, R., & Kulshrestha, J. (2022). Where the earth is flat and 9/11 is an inside job: A comparative algorithm audit of conspiratorial information in web search results. Telematics and Informatics, 72. https://doi.org/10.1016/j.tele.2022.101860
Vidgen, B., Scherrer, N., Kirk, H. R., Qian, R., Kannappan, A., Hale, S. A., & Röttger, P. (2023). SimpleSafetyTests: A test suite for identifying critical safety risks in large language models. arXiv. https://doi.org/10.48550/ARXIV.2311.08370
Virtanen, P., Gommers, R., Oliphant, T. E., Haberland, M., Reddy, T., Cournapeau, D., Burovski, E., Peterson, P., Weckesser, W., Bright, J., van der Walt, S., Brett, M., Wilson, J., Millman, J., Mayorov, N., Nelson, A., Jones, E., Kern, R., Larson, E., … SciPy 1.0 Contributors. (2020). SciPy 1.0: Fundamental algorithms for scientific computing in Python. Nature Methods, 17(3), 261–272. https://doi.org/10.1038/s41592-019-0686-2
Funding
This work has been financially supported by the Federal Ministry of Education and Research of Germany (BMBF) (grant no.: 16DII131 – “Weizenbaum-Institut”).
Competing Interests
The authors declare no competing interests.
Ethics
Because our research did not involve data collection from human users or any interaction with human users, it was exempt from the ethical review.
Copyright
This is an open access article distributed under the terms of the Creative Commons Attribution License, which permits unrestricted use, distribution, and reproduction in any medium, provided that the original author and source are properly credited.
Data Availability
All materials needed to replicate this study are available via the Harvard Dataverse: https://doi.org/10.7910/DVN/ZEDNXH
Acknowledgements
We would like to thank the anonymous reviewers and the editors of the Harvard Kennedy School (HKS) Misinformation Review for the excellent feedback, which helped us to improve the manuscript substantially. We also would like to thank Dr. Tobias Rohrbach for his valuable methodological feedback.