Peer Reviewed
Pausing to consider why a headline is true or false can help reduce the sharing of false news
Article Metrics
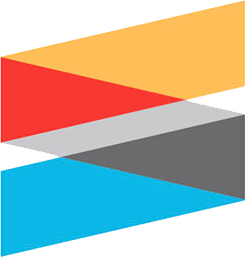
69
CrossRef Citations
Altmetric Score
PDF Downloads
Page Views
In an online experiment, participants who paused to explain why a headline was true or false indicated that they were less likely to share false information compared to control participants. Their intention to share accurate news stories was unchanged. These results indicate that adding “friction” (i.e., pausing to think) before sharing can improve the quality of information shared on social media.
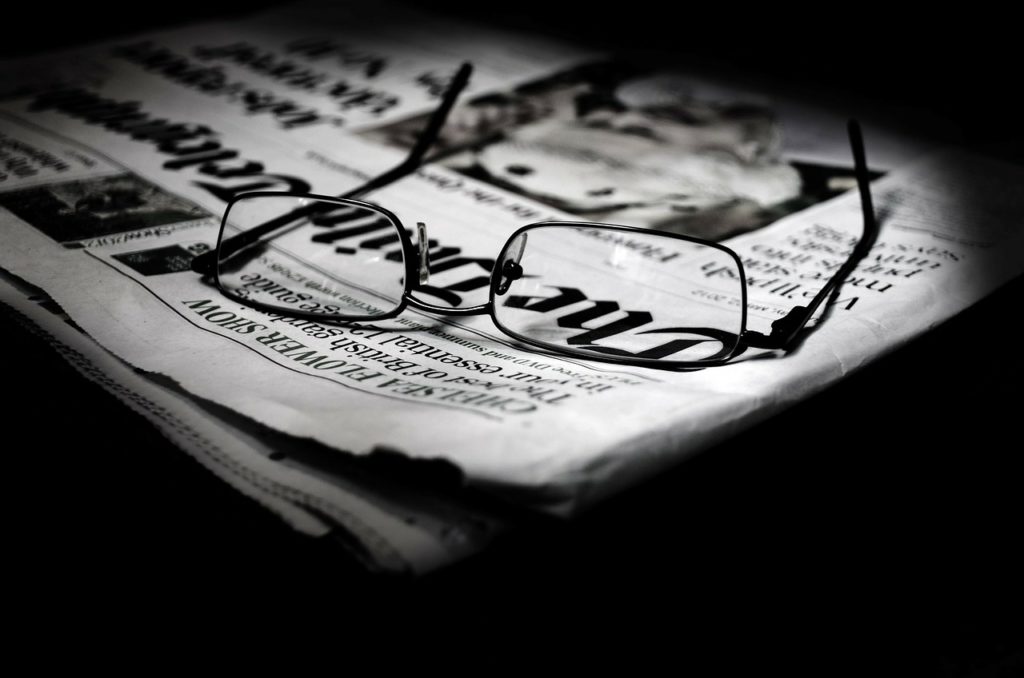
Research Questions
- Can asking people to explain why a headline is true or false decrease sharing of false political news headlines? Is this intervention effective for both novel headlines and ones that were seen previously?
Essay Summary
- In this experiment, 501 participants from Amazon’s mTurk platform were asked to rate how likely they would be to share true and false news headlines.
- Before rating how likely they would be to share the story, some participants were asked to “Please explain how you know that the headline is true or false.”
- Explaining why a headline was true or false reduced participants’ intention to share false headlines, but had no effect on true headlines.
- The effect of providing an explanation was larger when participants were seeing the headline for the first time. The intervention was less effective for headlines that had been seen previously in the experiment.
- This research suggests that forcing people to pause and think can reduce shares of false information.
Implications
While propagandists, profiteers, and trolls are responsible for the creation and initial sharing of much of the misinformation found on social media, this false information spreads due to actions of the general public (Vosoughi, Roy, & Aral, 2018). Thus, one way to reduce the spread of misinformation is to reduce the likelihood that individuals will share false information that they find online. Social media exists to allow people to share information with others, so our goal was not to reduce shares in general. Instead, we sought a solution that would reduce shares of incorrect information while not affecting accurate information.
In a large online survey experiment, we found that asking participants to explain how they knew that a political headline was true or false decreased their intention to share false headlines. This is good news for social media companies who may be able to improve the quality of information on their site by asking people to pause and think before sharing information, especially since the intervention did not reduce sharing of true information (the effects were limited to false headlines).
We suggest that social media companies should implement these pauses and encourage people to consider the accuracy and quality of what they are posting. For example, Instagram is now asking users “Are you sure you want to post this?” before they are able to post bullying comments (Lee, 2019). By making people pause and think about their action before posting, the intervention is aimed at decreasing the number of bullying comments on the platform. We believe that a similar strategy may also decrease shares of false information on other social media. Individuals can also implement this intervention on their own by committing to always pause and think about the truth of a story before sharing it with others.
One of the troubling aspects of social media is that people may see false content multiple times. That repetition can increase people’s belief that the false information is true (Fazio, Brashier, Payne, & Marsh, 2015; Pennycook, Cannon, & Rand, 2018), reduce beliefs that it is unethical to publish or share the false information (Effron & Raj, 2019), and increase shares of the false information (Effron & Raj, 2019). Thus, in our study, we examined how providing explanations affected shares of both new and repeated headlines.
Unlike prior research (Effron & Raj, 2019), we found that repetition did not increase participants intention to share false headlines. Both studies used very similar materials, so the difference is likely due to the number of repetitions. The repeated headlines in Effron and Raj (2019) were viewed five times during the experiment, while in our study they were only viewed twice. It may be that repetition does affect sharing, but only after multiple repetitions.
However, repetition did affect the efficacy of the intervention. Providing an explanation of why the headline was true or false reduced sharing intentions for both repeated and novel headlines, but the decrease was larger for headlines that were being seen for the first time. One possible explanation is that because the repeated headlines were more likely to be thought of as true, providing an explanation was less effective in reducing participants’ belief in the headline and decreasing their intentions to share. This finding suggests that it is important to alter how people process a social media post the first time that they see it.
There are multiple reasons why our intervention may have been effective. Providing an explanation helps people realize gaps between their perceived knowledge and actual knowledge (Rozenblit & Keil, 2002) and improves learning in classroom settings (Dunlosky, Rawson, Marsh, Nathan, & Willingham, 2013). Providing the explanation helps people connect their current task with their prior knowledge (Lombrozo, 2006). In a similar way, providing an explanation of why the headline is true or false may have helped participants consult their prior knowledge and realize that the false headlines were incorrect. The prompt may have also slowed people down and encouraged them to think more deeply about their actions rather than simply relying on their gut instinct. That is, people may initially be willing to share false information, but with a pause, they are able to resist that tendency (as in Bago, Rand, & Pennycook, 2020). Finally, the explanation prompt may have also encouraged a norm of accuracy and made participants more reluctant to share false information. People can have many motivations to share information on social media – e.g., to inform, to entertain, or to signal their group membership (Brady, Wills, Jost, Tucker, & Van Bavel, 2017; Metaxas et al., 2015). Thinking about the veracity of the headline may have shifted participants’ motivations for sharing and caused them to value accuracy more than entertainment.
To be clear, we do not think that our explanation task is the only task that would reduce shares of false information. Other tasks that emphasize a norm of accuracy or that force people to pause before sharing or consult their prior knowledge, may also be effective. Future research should disentangle if each of these three factors can reduce sharing of false information on their own, or if all three are necessary.
Findings
Finding 1: Explaining why a headline was true or false reduced participants’ intention to share false headlines, but did not affect sharing intentions for true headlines.
For each headline, participants rated the likelihood that they would share it online on a scale from 1 = not at all likely to 6 = extremely likely. For true headlines, participants’ intention to share the stories did not differ between the control condition (M = 2.17) and the explanation condition (M = 2.20), t(499) = 0.27, p = .789. Explaining why the headline was true or false did not change participants’ hypothetical sharing behavior for factual news stories (Figure 1A).
For false headlines, participants who first explained why the headline was true or false indicated that they would be less likely to share the story (M= 1.79) than participants in the control condition (M = 2.11), t(499) = 3.026, p = .003, Cohen’s d = 0.27 (Figure 1B). In the control condition, over half of the participants (57%) indicated that they would be “likely”, “somewhat likely” or “extremely likely” to share at least one false headline. However, in the explanation condition, only 39% indicated that they would be at least “likely” to share one or more false headlines. A similar decrease occurred in the number of people who indicated that they would be “extremely likely” to share at least one false headline (24% in control condition; 17% in explanation condition).
These patterns were reflected statistically in the results of a 2 (repetition: new, repeated) x 2 (truth status: true, false) x 2 (task: control, explain) ANOVA. This preregistered analysis indicated that there was a main effect of headline truth, F(1,499) = 60.39, p < .001, = .108, with participants being more likely to share true headlines than false headlines. There was also an interaction between the truth of the headline and the effect of providing an explanation, F(1,499) = 34.44, p < .001, = .065. Within the control condition, participants indicated that they were equally likely to share true (M = 2.17) and false headlines (M = 2.11), t(259) = 1.52, p= .129, Cohen’s d = 0.09. In the explain condition, however, participants indicated that they would be less likely to share false headlines (M = 1.79) as compared to true headlines (M = 2.20), t(240) = 8.63, p < .001, Cohen’s d = 0.56.
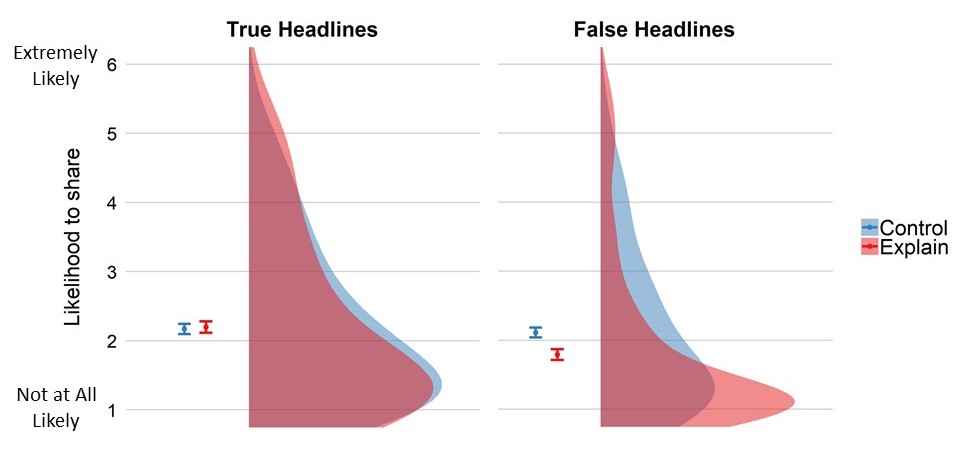
Finding 2: The intervention was not as effective for repeated headlines.
Overall, participants indicated that they were equally likely to share new headlines (M= 2.07) and repeated headlines (M = 2.06). However, as shown below (Figure 2), providing an explanation reduced participants’ likelihood to share new headlines more than repeated headlines. Within the false headlines, providing an explanation reduced participants’ intent to share for both new (control M = 2.16, explain M = 1.74, t(298) = 3.87, p < .001, Cohen’s d = 0.35) and repeated headlines (control M = 2.07, explain M = 1.85, t(298) = 2.04, p = .042, Cohen’s d = 0.18). This decrease in sharing intentions was much larger when the headline was being viewed for the first time.
These patterns were reflected statistically in the same 2 (repetition: new, repeated) x 2 (truth status: true, false) x 2 (task: control, explain) ANOVA partially reported above. The main effect of repetition was not significant, F(1,499) = 0.44, p = .510, = .001, but there was an interaction between the effect of repetition and providing an explanation, F(1,499) = 7.23, p = .007, = .014. In addition, there was a 3-way interaction between repetition, task and truth, F(1,499) = 6.19, p = .013, = .012. No other main effects or interactions were significant.
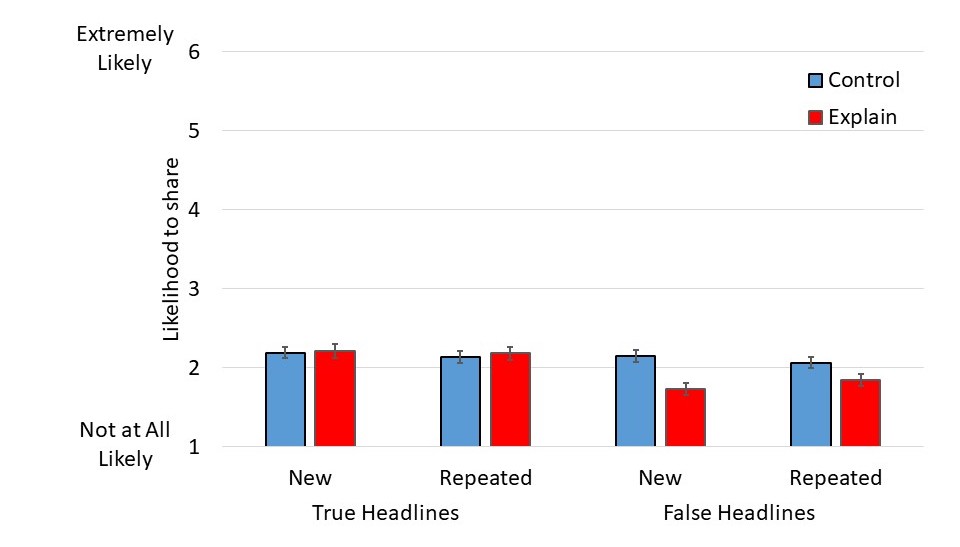
Limitations and future directions
One key question is how well participants’ intent to share judgments match their actual sharing behavior on social media platforms. Recent research, using the same set of true and false political news headlines as the current study, suggests that participants’ survey responses are correlated with real-world shares (Mosleh, Pennycook, & Rand, 2019). Headlines that participants indicated that they would be more likely to share in mTurk surveys were also more likely to be shared on Twitter. Thus, it appears that participants’ survey responses are predictive of actual sharing behavior.
In addition, both practitioners and researchers should be aware that we tested a limited set of true and false political headlines. While we believe that the headlines are typical of the types of true and false political stories that circulate on social media, they are not a representative sample. In particular, the results may differ when it is less obvious which stories are likely true or likely suspect.
A final limitation of the study was that the decrease in sharing intentions for the false headlines was relatively small (0.32 points on a 6-point scale). However, this small decrease could still have a large effect in social networks where shares affect how many people see a post. In addition, our participants were relatively unlikely to share these political news headlines – the average rating was just above “slightly likely”. Since participants were already unlikely to share the posts, the possible effect of the intervention was limited. Future research should examine how providing an explanation affects sharing of true and false posts that people are more likely to share.
Methods
All data are available online, along with a preregistration of our hypotheses, primary analyses and sample size (https://osf.io/mu7n8/).
Participants. Five hundred and one participants (Mage = 40.99, SD = 12.88) completed the full study online via Amazon’s Mechanical Turk (260 in the control condition, 241 in the explain condition). An additional 17 participants started but did not finish the study (5 in the control condition, 12 in the explain condition). Using TurkPrime (Litman, Robinson, & Abberbock, 2017), we restricted the sample to participants in the United States and blocked duplicate IP addresses.
Materials. We used 24 true and false political headlines from Pennycook, Cannon, and Rand (2018) Experiment 3. Half of the headlines were true and came from reliable sources such as washingtonpost.com, nytimes.com and npr.org. The other half were false and came from disreputable sources such as dailyheadlines.net, freedomdaily.com and politicono.com. In addition, within each set, half of the headlines were pro-republican and the other half pro-democrat. (We did not measure participants’ political beliefs in this study; therefore, we did not examine differences in sharing between pro-republican and pro-democrat headlines).
As in the Pennycook study, the headlines were presented in a format similar to a Facebook post (a photograph with a headline and byline below it). See Figure 3 for examples. The full set of headlines is available from Pennycook and colleagues at https://osf.io/txf46/.
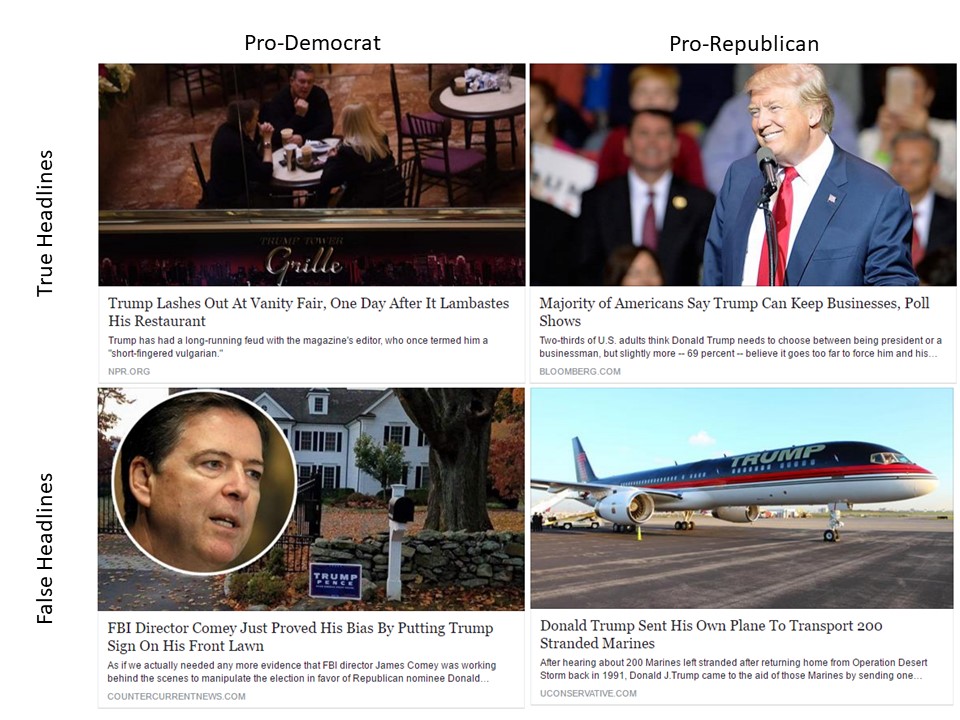
Design and counterbalancing. The experiment had a 2 (repetition: repeated, new) X 2 (task: control, explain) mixed design. Repetition was manipulated within-subjects, while the participants’ task during the share phase was manipulated between-subjects. The 24 headlines were split into two sets of 12, and both sets contained an equal number of true/false and pro-democrat/pro-republican headlines. Across participants, we counterbalanced which set of 12 was repeated (presented during the exposure and share phase) and new (presented only during the share phase).
Procedure. The experiment began with the exposure phase. Participants viewed 12 headlines and were asked to judge “How interested are you in reading the rest of the story?” Response options included Very Uninterested, Uninterested, Slightly Uninterested, Slightly Interested, Interested, and Very Interested. Each headline was presented individually, and participants moved through the study at their own pace. Participants were correctly informed that some of the headlines were true and others were false.
After rating the 12 headlines, participants proceeded immediately to the sharing phase. The full set of 24 headlines was presented one at a time and participants were asked “How likely would you be to share this story online?” The response options included Not at all likely, A little bit likely, Slightly likely, Pretty likely, Very likely, and Extremely likely. Participants in the control condition simply viewed each headline and then rated how likely they would be to share it online.
Participants in the explain condition saw the headline and were first asked to “Please explain how you know that the headline is true or false” before being asked how likely they would be to share the story. All participants were told that some of the headlines would be ones they saw earlier, and that others would be new. They were also again told that some headlines would be true and others not true.
Topics
Bibliography
Bago, B., Rand, D. G., & Pennycook, G. (2020). Fake news, fast and slow: Deliberation reduces belief in false (but not true) news headlines. Journal of Experimental Psychology: General. doi:10.1037/xge0000729
Brady, W. J., Wills, J. A., Jost, J. T., Tucker, J. A., & Van Bavel, J. J. (2017). Emotion shapes the diffusion of moralized content in social networks. Proceedings of the National Academy of Sciences, 114(28), 7313-7318. doi:10.1073/pnas.1618923114
Dunlosky, J., Rawson, K. A., Marsh, E. J., Nathan, M. J., & Willingham, D. T. (2013). Improving students’ learning with effective learning techniques promising directions from cognitive and educational psychology. Psychological Science in the Public Interest, 14(1), 4-58. doi:10.1177/1529 i 00612453266
Effron, D. A., & Raj, M. (2019). Misinformation and morality: encountering fake-news headlines makes them seem less unethical to publish and share. Psychological Science, 31, 75-87. doi:10.1177/0956797619887896
Fazio, L. K., Brashier, N. M., Payne, B. K., & Marsh, E. J. (2015). Knowledge does not protect against illusory truth. Journal of Experimental Psychology: General, 144(5), 993-1002. doi:10.1037/xge0000098
Lee, D. (2019). Instagram now asks bullies: ‘Are you sure?’. Retrieved from https://www.bbc.com/news/technology-48916828?
Litman, L., Robinson, J., & Abberbock, T. (2017). TurkPrime.com: A versatile crowdsourcing data acquisition platform for the behavioral sciences. Behavior Research Methods(49), 433-442. doi:10.3758/s13428-016-0727-z
Lombrozo, T. (2006). The structure and function of explanations. Trends in Cognitive Sciences, 10(10), 464-470. doi:10.1016/j.tics.2006.08.004
Metaxas, P., Mustafaraj, E., Wong, K., Zeng, L., O’Keefe, M., & Finn, S. (2015). What do retweets indicate? Results from user survey and meta-review of research.Paper presented at the Ninth International AAAI Conference on Web and Social Media.
Mosleh, M., Pennycook, G., & Rand, D. G. (2019). Self-reported willingness to share political news articles in online surveys correlates with actual sharing on Twitter.PsyArXiv Working Paper. doi:10.31234/osf.io/zebp9
Pennycook, G., Cannon, T. D., & Rand, D. G. (2018). Prior exposure increases perceived accuracy of fake news. . Journal of Experimental Psychology: General, 147(12), 1865-1880. doi:10.1037/xge0000465
Rozenblit, L., & Keil, F. (2002). The misunderstood limits of folk science: An illusion of explanatory depth. Cognitive science, 26(5), 521-562. doi:10.1207/s15516709cog2605_1
Vosoughi, S., Roy, D., & Aral, S. (2018). The spread of true and false news online. Science, 359(6380), 1146-1151. doi:10.1126/science.aap9559
Funding
This research was funded by a gift from Facebook Research.
Competing Interests
The author(s) declared no potential conflicts of interest with respect to the research, authorship, and/or publication of this article.
Ethics
Approval for this study was provided by the Vanderbilt University Institutional Review Board and all participants provided informed consent.
Copyright
This is an open access article distributed under the terms of the Creative Commons Attribution License, which permits unrestricted use, distribution, and reproduction in any medium, provided that the original author and source are properly credited.
Data Availability
All materials needed to replicate this study are available via the Center for Open Science:https://osf.io/mu7n8/.