Peer Reviewed
Older Americans are more vulnerable to prior exposure effects in news evaluation
Article Metrics
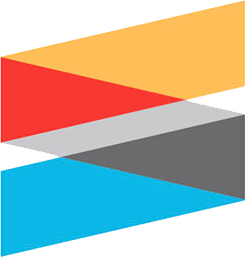
0
CrossRef Citations
Altmetric Score
PDF Downloads
Page Views
Older news users may be especially vulnerable to prior exposure effects, whereby news comes to be seen as more accurate over multiple viewings. I test this in re-analyses of three two-wave, nationally representative surveys in the United States (N = 8,730) in which respondents rated a series of mainstream, hyperpartisan, and false political headlines (139,082 observations). I find that prior exposure effects increase with age—being strongest for those in the oldest cohort (60+)—especially for false news. I discuss implications for the design of media literacy programs and policies regarding targeted political advertising aimed at this group.
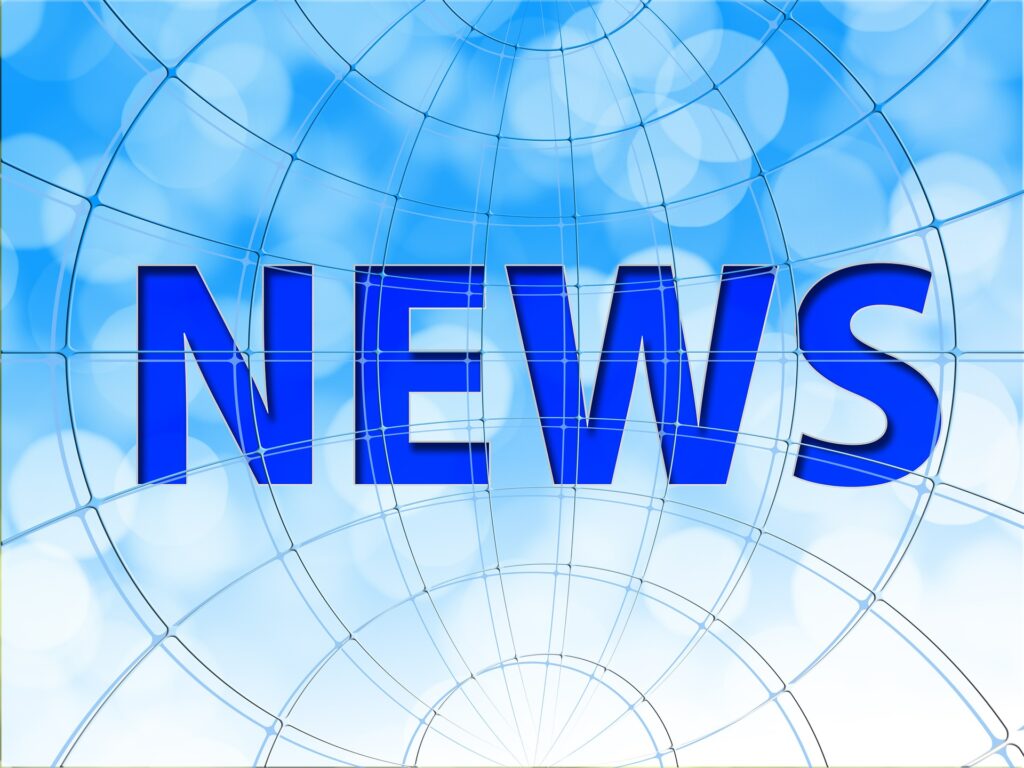
Research Questions
- Do the effects of prior exposure to news headlines increase with age?
- Does this vary by news type (false, hyperpartisan, mainstream)?
Essay Summary
- I used three two-wave, nationally representative surveys in the United States (N = 8,730) in which respondents rated a series of actual mainstream, hyperpartisan, or false political headlines. Respondents saw a sample of headlines in the first wave and all headlines in the second wave, allowing me to determine if prior exposure increases perceived accuracy differentially across age.
- I found that the effect of prior exposure to headlines on perceived accuracy increases with age. The effect increases linearly with age, with the strongest effect for those in the oldest age cohort (60+). These age differences were most pronounced for false news.
- These findings suggest that repeated exposure can help account for the positive relationship between age and sharing false information online. However, the size of this effect also underscores that other factors (e.g., greater motivation to derogate the out-party) may play a larger role.
Implications
Web-tracking and social media trace data paint a concerning portrait of older news users. Older American adults were much more likely to visit dubious news sites in 2016 and 2020 (Guess, Nyhan, et al., 2020; Moore et al., 2023), and were also more likely to be classified as false news “supersharers” on Twitter, a group who shares the vast majority of dubious news on the platform (Grinberg et al., 2019). Likewise, this age group shares about seven times more links to these domains on Facebook than younger news consumers (Guess et al., 2019; Guess et al., 2021).
Interestingly, however, older adults appear to be no worse, if not better, at identifying false news stories than younger cohorts when asked in surveys (Brashier & Schacter, 2020). Why might older adults identify false news in surveys but fall for it “in the wild?” There are likely multiple factors at play, ranging from social changes across the lifespan (Brashier & Schacter, 2020) to changing orientations to politics (Lyons et al., 2023) to cognitive declines (e.g., in memory) (Brashier & Schacter, 2020). In this paper, I focus on one potential contributor. Specifically, I tested the notion that differential effects of prior exposure to false news helps account for the disjuncture between older Americans’ performance in survey tasks and their behavior in the wild.
A large body of literature has been dedicated to exploring the magnitude and potential boundary conditions of the illusory truth effect (Hassan & Barber, 2021; Henderson et al., 2021; Pillai & Fazio, 2021)—a phenomenon in which false statements or news headlines (De keersmaecker et al., 2020; Pennycook et al., 2018) come to be believed over multiple exposures. Might this effect increase with age? As detailed by Brashier and Schacter (2020), cognitive deficits are often blamed for older news users’ behaviors. This may be because cognitive abilities are strongest in young adulthood and slowly decline beyond that point (Salthouse, 2009), resulting in increasingly effortful cognition (Hess et al., 2016). As this process unfolds, older adults may be more likely to fall back on heuristics when judging the veracity of news items (Brashier & Marsh, 2020). Repetition, the source of the illusory truth effect, is one heuristic that may be relied upon in such a scenario. This is because repeated messages feel easier to process and thus are seen as truer than unfamiliar ones (Unkelbach et al., 2019).
Available evidence that can speak to this question is suggestive but inconclusive. Some past studies have suggested that older adults could be more susceptible to illusions of truth (Law et al., 1998; Mutter, 1995; but see Parks et al., 2006). However, it should be noted that these studies rely on quite different designs and stimuli (e.g., trivia statements or product claims) and small convenience samples. As others have noted, political headlines differ from trivia and product claims in relevance and other dimensions (Pennycook et al., 2018).
To determine whether age increases reliance on the heuristic provided by repetition, I conduct the analysis on a large dataset of three two-wave, nationally representative surveys in the United States in which respondents rated a series of actual political headlines, half of which were repeated in the second wave, about two weeks after the first exposure. I found that prior exposure effects increase with age and are strongest for those over 60 years old. This difference by age is present when pooling all headlines or looking at mainstream, hyperpartisan, or false news headlines in isolation, and appears to be strongest for false news headlines. Notably, though, prior exposure’s differential effects on perceived accuracy across age are quite small, substantively. This means that although there are clear differences in the prior exposure effect by age group, these are not classified as large effects. For context, the effect of prior exposure on belief in false news for those age 60+ is about a third the size of the effect of the headline’s agreement with the respondent’s partisan leanings (though partisan congeniality is regarded as one of the most powerful factors determining belief, see e.g., Gawronski et al., 2023). This effect size is in line with a great deal of findings about psychology and media effects when tested in high-powered pre-registered settings (see Schäfer & Schwarz, 2019); few social phenomena are monocausal, and as such concurrent explanations can also help explain the news engagement among older consumers. Indeed, it would be concerning if there were extremely large age differences in prior exposure effects, as this would sound the alarm regarding the plausibility of the findings (Hilgard, 2021). On the other hand, I tested only the effect of a single exposure that occurred about two weeks prior; it is reasonable to assume that a news consumer could repeatedly encounter viral misinformation, thereby enhancing the effect to some degree (Hassan & Barber, 2021).
It is imperative to be clear that I am not arguing for a clinical diagnosis of cognitive decline per se, and there are no direct indicators of this in the available data. Instead, I only provide initial evidence for differential effects of prior exposure across age. Psychologists have speculated this may occur due to natural declines that occur with age that might prompt greater reliance on fluency heuristics (e.g., Brashier & Schacter, 2020). However, other accounts, perhaps centering on how older adults differentially engage with political information based on shifting orientations to politics, may be valid. Given the nature of these data, I cannot speak directly to mechanism, and others should explore this topic.
In any case, one way these findings might be applied is in the design of media literacy interventions, especially those targeted to older news consumers (e.g., Guess, Lerner, et al., 2020; Moore & Hancock, 2022). If older people are more susceptible to prior exposure effects, this would be an overlooked component of addressing age-based media literacy (e.g., programs such as Poynter’s MediaWise for Seniors). In other words, current wisdom suggests I can best help older news users by focusing on skills for identifying misinformation online. However, because older users are well equipped to spot misinformation in the first place (Brashier & Schacter, 2020) and literacy training is not more effective among these users (which suggests they are not at a comparative deficit in such skills) (Lyons et al., 2023), explicitly communicating the effects of repetition might be one way to supplement training modules. In sum, interventions aimed at older news consumers could be modified to include information about the effects of repeated exposure, which might boost metacognitive awareness and lead to debiasing (for potential limitations of this approach, see Kristal & Stanos, 2021).
Additionally, if older users are uniquely susceptible to repeated targeting, as found here, there may be new ethical implications to consider when platforms set internal policy or are externally regulated regarding targeted advertising (King, 2022). Currently, for example, those under 18 are a protected class of Facebook and Instagram users as the platforms “agreed with youth advocates that young people might not be equipped to make decisions about targeting,” (Culliford, 2021) and have since been “given an option to ‘see less’ of a given topic, shaping which ads the platform will serve them” (Hatmaker, 2023). One potential proposition based on the findings presented here would be that users above a certain age threshold similarly be protected from repeated political targeting that could exploit an increased vulnerability to the illusory truth effect. Regardless of platforms’ internal policies, these findings may strengthen advocates’ arguments against microtargeted advertising more broadly, given the potential for harm identified here (e.g., Banning Microtargeted Political Ads Act of 2021; Cyphers & Schwartz, 2022).
Although I bring a large dataset drawn from multiple representative samples of Americans to bear on this question, some limitations should be considered. First, this is an analysis of previously collected data and therefore is exploratory. The study was also conducted in the United States and thus may not generalize elsewhere (see Henderson et al., 2021). Similarly, the headline stimuli are politically focused; although other studies of prior exposure effects of false news headlines take a similar approach, future work may test for these effects across a variety of news topics. For these reasons, the findings should be further replicated. Another direction for future work would be to over-sample older adults to probe whether important differences emerge within this subgroup of respondents. Finally, while I did find evidence that older Americans are more subject to prior exposure effects, it is important to emphasize that other factors, such as increasing political interest and entrenched partisan identity across the lifespan, likely also play a role in different patterns of news behaviors among older adults (Glenn & Grimes, 1968; Sears & Funk, 1999; Stoker & Jennings, 2008).
Regardless, the analysis offers robust evidence that prior exposure to news headlines increases perceptions of accuracy more strongly for older adults. This holds across mainstream, hyperpartisan, and especially false headlines. This finding addresses an open question in ongoing debates of direct public interest and helps untangle the complicated relationship between age and news engagement.
Findings
Finding 1: Older Americans are especially affected by repeated exposure to news headlines in general.
First, it is worth noting that both prior exposure and age have main effects on perceived accuracy of news headlines, as shown in Table B1. I used OLS regression models with perceived headline accuracy measured in wave 2 as the outcome variable. I used wave 1 exposure to a given headline, as well as age as predictors, and controlled for headline congeniality (whether the headline is favorable to the respondent’s political party affiliation) as a covariate. As the analyses were conducted at the headline level, I included fixed effects for each headline and clustered at the respondent level to account for correlations between their ratings across headlines. Prior exposure increases perceived accuracy of headlines in general, as well as for each news type individually. On the other hand, age is associated with lower perceived accuracy in the pooled headline analysis, and for false headlines especially (as seen in prior studies, e.g., Brashier & Schacter, 2020).
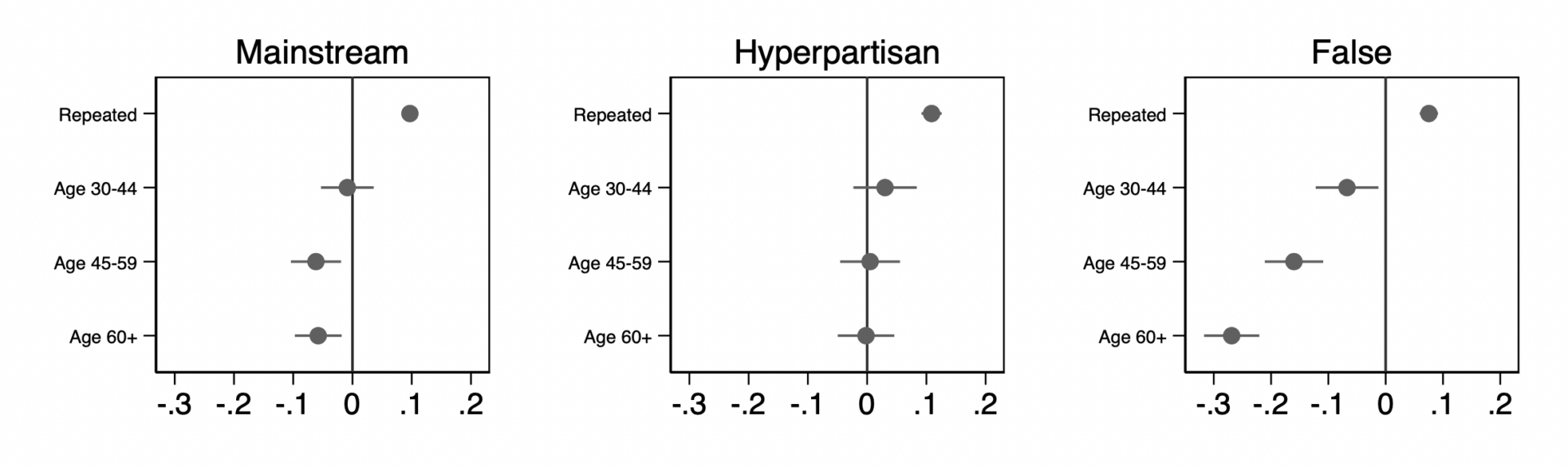
However, the key test of the research question is the interaction of age and prior exposure. Here, I looked at effects across age groups: 18-29, 30-44, 45-59, and 60+. Table B2 depicts the results of this initial model, which examines prior exposure effects pooling across all headline types, resulting in 139,082 observations. I found that, compared to Americans aged 18-29, older Americans (45-59: b = .04, SE = .01, p < .005; 60+: b = .07, SE = .01, p < .005) are especially affected by repeated exposure to news headlines in general.
Finding 2: Older American’s increased sensitivity holds across mainstream, hyperpartisan, and false headlines.
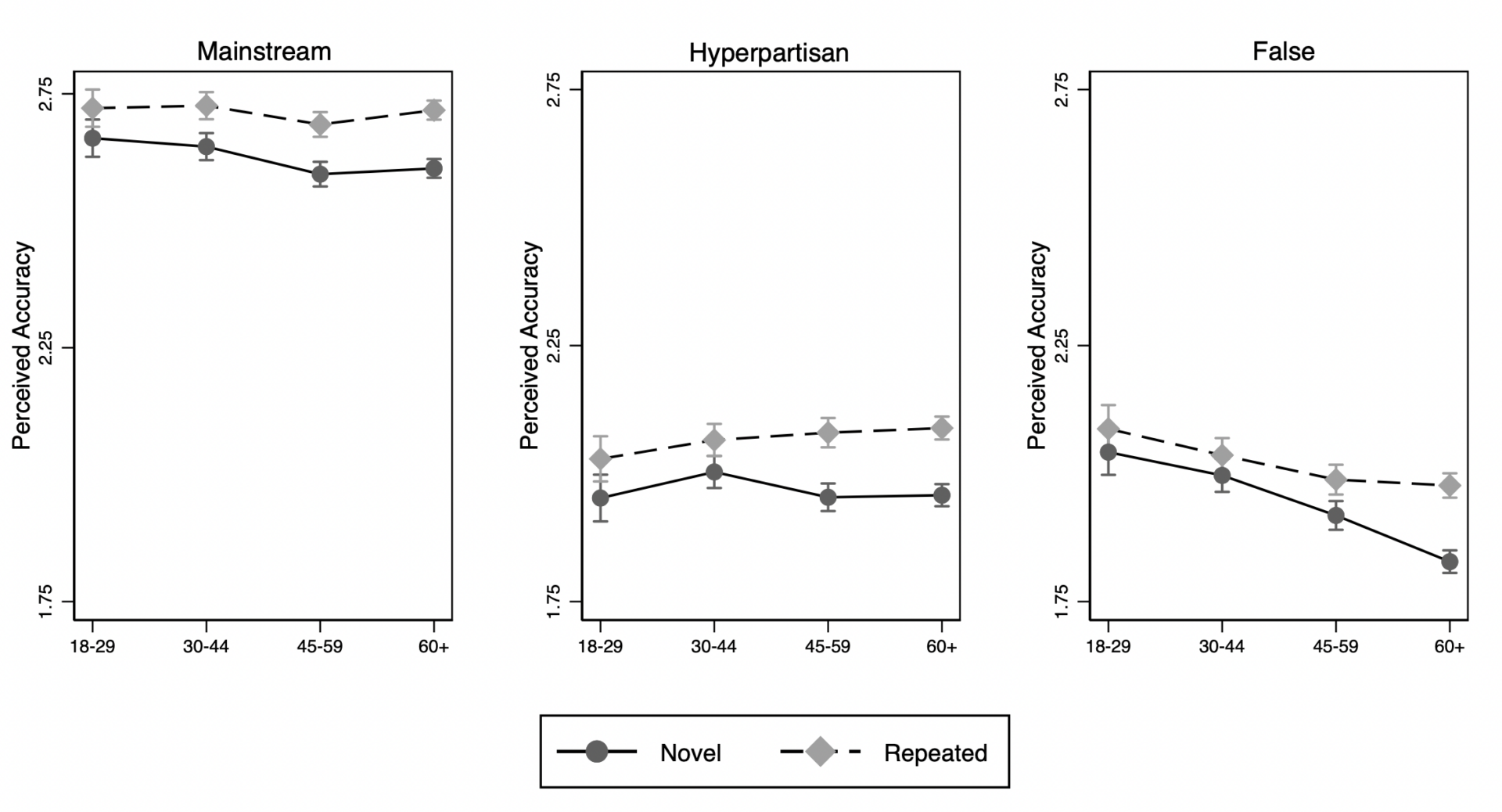
Table B3 depicts the results broken down by news type, with the number of observations ranging from 34,770 to 69,537. Older Americans exhibited greater effects of prior exposure for mainstream (b = .06, SE = .02, p < .005), hyperpartisan (b = .05, SE = .03, p < .005), and false headlines (b = .10, SE = .02, p < .005). The effect is again strongest among those in the oldest subgroup (60+). This increased sensitivity is especially pronounced for false headlines.
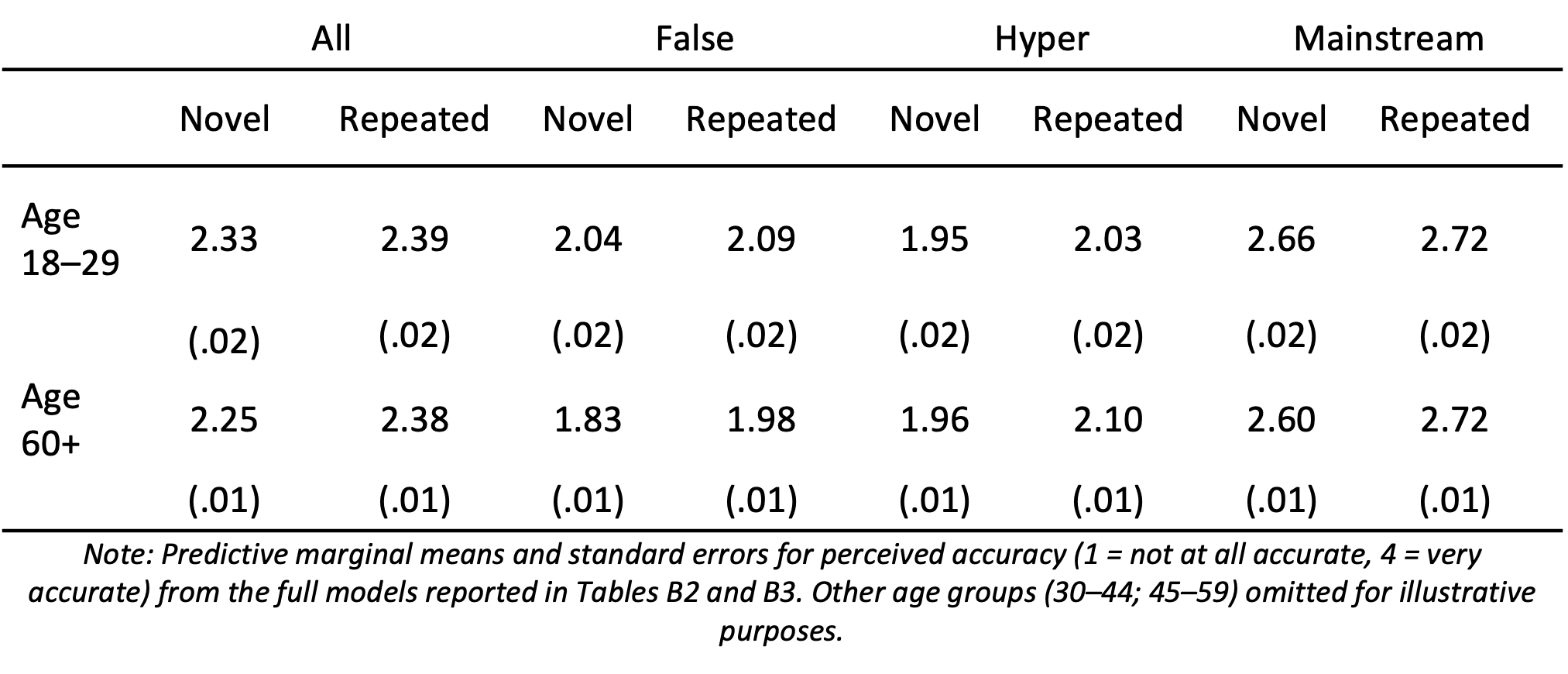
We can get a better sense for the size of this effect by examining the predictive margins of prior exposure by age category (Table 1). For mainstream news, among 18–29-year-olds, prior exposure only increases perceived accuracy from 2.66 to 2.72 on the 4-pt scale. Among the 60+ group, prior exposure increases perceived accuracy from 2.60 to 2.72. The difference is largest for false news: among 18–29-year-olds, prior exposure only increases perceived accuracy from 2.04 to 2.09, about a 1% increase on the 4-pt. scale. Among the 60+ group, prior exposure increases perceived accuracy from 1.83 to 1.98, about a 4% increase in believability, on average, for false news headlines from a brief, single exposure about two weeks prior. To put the size of this effect in context, partisan congeniality, which is recognized as one of the most powerful factors in believability of political news (e.g., Gawronski et al., 2023; Osmundsen et al., 2021; Pereira et al., 2023), increases perceived accuracy by about 14% for false headlines.
Methods
I used data from three two-wave survey panels conducted by the survey company YouGov leading up to and following the 2018 U.S. midterm elections. Specifically, I drew on:
- A two-wave panel study fielded June 25–July 3, 2018 (wave 1; N = 1,718) and July 9–17, 2018 (wave 2; N = 1,499)
- A two-wave panel study fielded October 19–26 (wave 1; N = 3,378) and October 30–November 6, 2018 (wave 2; N = 2,948)
- A two-wave panel study fielded November 20–December 27, 2018 (wave 1; N = 4,907) and December 14, 2018–January 3, 2019 (wave 2; N = 4,283).
Respondents were selected by YouGov’s matching and weighting algorithm to approximate the demographic and political attributes of the U.S. population (see Appendix A for demographic breakdowns). Participants were not able to take part in more than one study. Note that the data, analysis script, and materials can be found here: https://osf.io/rsbvm/.
Procedure
In the first wave of each survey, respondents completed demographics and sociopolitical measures pre-treatment. They then evaluated a randomized subset of eight headlines, from 16 total possible headlines. Exposure to a headline in wave 1 served as the independent variable in the analysis. After a delay between waves that averaged several weeks, respondents took part in wave 2 of the survey, where they rated all 16 headlines. The rating of a headline in wave 2 serves as the dependent variable. Respondents were then debriefed about the study, informed which headlines were false, and provided links to fact-checks for each.
News headline rating and prior exposure manipulation
Each survey asked respondents to rate the accuracy of a set of headlines on a four-point scale. All articles circulated during the 2018 midterm elections (i.e., were actually published) and were balanced within each group in terms of partisan sympathy. I employed four mainstream news stories sympathetic to Democrats and four sympathetic to Republicans (divided into low-prominence and high-prominence sources in each case). Note that I used the label “mainstream” rather than, e.g., “true,” as these headlines have not been verified as true by third parties, because selecting only verified-true news presents validity issues (Pennycook et al., 2021). As noted, mainstream headlines came from both high-prominence and low-prominence outlets. I define high-prominence mainstream sources as those that more than 4 in 10 Americans reported recognizing in polling by Pew (Mitchell et al., 2014). I similarly selected two pro-Democrat false news articles and two pro-Republican false news articles. False news was confirmed to be false by at least one independent fact-checking organization. Respondents also evaluated four hyperpartisan news headlines. Although such headlines are technically factual, they present facts in a misleading or distorted fashion. These headlines were chosen from outlets listed as hyperpartisan in prior work (e.g., Pennycook & Rand, 2019). When presented to the respondents, the headlines were formatted exactly as they appeared in the Facebook news feed circa 2018. Due to Facebook’s formatting at the time, the preview appearance of some false article headlines was slightly different from that of other articles. This format mimics the decision-making environment faced by everyday users, who often evaluate the accuracy of news articles based solely on the content they see in their news feed. All headlines are listed in Appendix A.
As mentioned, the 16 headlines in the study can be broken down by news type (high-prominence mainstream, low-prominence mainstream, hyperpartisan, and false) and slant (pro-Democrat, pro-Republican), for a total of eight type-slant categories. In the first wave of each survey, respondents saw a randomly drawn headline from each subcategory and rated each on a scale ranging from “Not at all accurate” (1) to “Very accurate” (4). In the follow-up wave of each survey, respondents rated all 16 headlines. The order of all headline presentation was randomized within each wave. The first survey, conducted in June and July 2018, used a distinct set of headlines from the latter two surveys, which used a common set.
Congeniality of these headlines is coded for partisans indicating whether the news item is favorable to their political leaning (e.g., a Republican viewing a headline that is favorable to a Republican). This binary measure uses the standard two-question party identification battery (which includes leaners) to classify respondents as Democrats or Republicans.
I computed a measure of prior exposure at the headline level based on whether the respondent was randomly assigned to rate a given headline in wave 1, wherein each respondent rated a subset of headlines from each slant-and-veracity subcategory (as noted, in the second wave of each survey, respondents subsequently evaluated all 16 headlines, thus allowing a random subset by respondent to carry potential prior exposure effects).
Demographics and sociopolitical measures
I measured a number of personal characteristics that includes objective political knowledge, political interest, dichotomous indicators of Democrat and Republican affiliation (including leaners), college education, gender, and nonwhite racial background. For the analysis of age effects, I used a set of common dichotomous indicators of age (18–29, 30–44, 45–59, and 60+; 18–29 is used as the omitted category) as well as the linear term (full question list available in Appendix A).
Analytical approach
I used OLS regression models with perceived headline accuracy measured in wave 2 as the outcome variable. I used wave 1 exposure to a given headline, age, and their interaction term as the predictors, and controlled for headline congeniality as a covariate. As the analyses were conducted at the headline level, I included fixed effects for each headline to account for their differing baseline levels of plausibility and clustered at the respondent level to account for correlations between their ratings across headlines. In sum, following recent illusory truth effect studies (Fazio et al., 2022; Henderson et al., 2021; Vellani et al., 2023), this design improves on prior analyses (Dechêne et al., 2010) by conducting the analysis at the level of the individual rating, rather than averaging across headline ratings (Barr et al., 2013; Judd et al., 2012). To do so, I used OLS regressions with standard errors clustered on subject and fixed effects for headlines (Pennycook et al., 2021). In these tests of treatment effects, I do not include survey weights (Franco et al., 2017; Miratrix et al., 2018). I first looked at the effect by pooling all headlines, then analyzed prior exposure effects across mainstream, false, and hyperpartisan headlines separately. Analysis for the main effects of prior exposure was pre-registered (https://osf.io/94x5b), but the focal age moderation is exploratory.
The primary analysis used dichotomous indicators for a standard set of age groups, as fitting linear interaction models can mask nonlinearities in interaction effects (Hainmueller et al., 2019). Further, studies employing trace data summarized above suggest that it is the oldest subgroup of users, in particular, who are most likely to consume dubious news, specifically those 60+ (Guess et al., 2020; Moore et al., 2022). As such, I looked at the size of prior exposure effects across the same age categories as used in these studies. However, I included the linear interaction models in subsequent analyses. As in the previous analyses, Table B4 shows a significant interaction of prior exposure and a linear age term when pooling all headlines or examining headlines of any news type.
Additionally, I replicated the analysis while controlling for a set of standard covariates: Democrat, Republican, college education, political knowledge, political interest, gender, and nonwhite racial background. The primary models do not include other covariates because inclusion of background controls is not necessary for experimental designs due to benefits of random assignment, and in fact, flexible inclusion of covariates in an analysis can lead to Type I error inflation (e.g., Simmons et al., 2011; Simonsohn et al., 2014) or otherwise add noise to the model in some cases (Mutz & Pemantle, 2012). However, in other cases, covariate adjustment can increase precision around the estimated treatment effect. With this in mind, this supplemental analysis, available in Table B5, shows all outcomes are robust to the inclusion of these covariates.
Finally, it is worth examining the role of headline congeniality, which is controlled for in the analyses above. In further supplemental analyses available in the OSF repository, I first document a positive effect of headline congeniality on perceived accuracy, which notably increases with age (see also Lyons et al., 2023). The effects of prior exposure are also stronger for congenial headlines. However, as seen in Table B6, the data also show that the interaction of age and prior exposure is significant for both congenial and non-congenial headlines across all news types, which suggests congeniality itself is not driving this central finding.
Topics
Bibliography
Banning Microtargeted Political Ads Act of 2021, H.R.4955, 117th Cong. (2021). https://www.congress.gov/bill/117th-congress/house-bill/4955
Barr, D. J., Levy, R., Scheepers, C., & Tily, H. J. (2013). Random effects structure for confirmatory hypothesis testing: Keep it maximal. Journal of Memory and Language, 68(3), 255–278. https://doi.org/10.1016/j.jml.2012.11.001
Brashier, N. M., & Marsh, E. J. (2020). Judging truth. Annual Review of Psychology, 71, 99–515. https://doi.org/10.1146/annurev-psych-010419-050807
Brashier, N. M., & Schacter, D. L. (2020). Aging in an era of fake news. Current Directions in Psychological Science, 29(3), 316–323. https://doi.org/10.1177/0963721420915872
Calvillo, D. P., & Smelter, T. J. (2020). An initial accuracy focus reduces the effect of prior exposure on perceivedaccuracy of news headlines. Cognitive Research: Principles and Implications, 5(1). https://doi.org/10.1186/s41235-020-00257-y
Culliford, E. (2021, July 27). Facebook will restrict ad targeting of under-18s. Reuters. https://www.reuters.com/technology/facebook-will-restrict-ad-targeting-under-18s-2021-07-27/
Cyphers, B., & Schwartz, A. (2022, November 17). Ban online behavioral advertising. Electronic Frontier Foundation. https://www.eff.org/deeplinks/2022/03/ban-online-behavioral-advertising
Dechêne, A., Stahl, C., Hansen, J., & Wänke, M. (2010). The truth about the truth: A meta-analytic review of the truth effect. Personality and Social Psychology Review, 14(2), 238–257. https://doi.org/10.1177/1088868309352251
De keersmaecker, J., Dunning, D., Pennycook, G., Rand, D. G., Sanchez, C., Unkelbach, C., & Roets, A. (2020). Investigating the robustness of the illusory truth effect across individual differences in cognitive ability, need for cognitive closure, and cognitive style. Personality and Social Psychology Bulletin, 46(2), 204–215. https://doi.org/10.1177/0146167219853844
Fazio, L. K., Pillai, R. M., & Patel, D. (2022). The effects of repetition on belief in naturalistic settings. Journal of Experimental Psychology: General, 151(10), 2604–2613. https://doi.org/10.1037/xge0001211
Franco, A., Malhotra, N., Simonovits, G., & Zigerell, L. (2017). Developing standards for post-hoc weighting in population-based survey experiments. Journal of Experimental Political Science, 4(2), 161–172. https://doi.org/10.1017/XPS.2017.2
Gawronski, B., Ng, N. L., & Luke, D. M. (2023). Truth sensitivity and partisan bias in responses to misinformation. Journal of Experimental Psychology: General. Advance online publication. https://psycnet.apa.org/doi/10.1037/xge0001381
Glenn, N. D., & Grimes, M. (1968). Aging, voting, and political interest. American Sociological Review, 33(4), 563–575. https://doi.org/10.2307/2092441
Grinberg, N., Joseph, K., Friedland, L., Swire-Thompson, B., & Lazer, D. (2019). Fake news on Twitter during the 2016 US presidential election. Science, 363(6425), 374–378. https://doi.org/10.1126/science.aau2706
Guess, A., Aslett, K., Tucker, J., Bonneau, R., & Nagler, J. (2021). Cracking open the news feed: Exploring what US Facebook users see and share with large-scale platform data. Journal of Quantitative Description: Digital Media, 1. https://doi.org/10.51685/jqd.2021.006
Guess, A., Lerner, M., Lyons, B., Montgomery, J. M., Nyhan, B., Reifler, J., & Sircar, N. (2020). A digital media literacy intervention increases discernment between mainstream and false news in the United States and India. Proceedings of the National Academy of Sciences, 117(27), 15536–15545. https://doi.org/10.1073/pnas.1920498117
Guess, A., Nagler, J., & Tucker, J. (2019). Less than you think: Prevalence and predictors of fake news dissemination on Facebook. Science Advances, 5(1). https://doi.org/10.1126/sciadv.aau4586
Guess, A., Nyhan, B., & Reifler, J. (2020). Exposure to untrustworthy websites in the 2016 us election. Nature Human Behaviour, 4(5), 472–480. https://doi.org/10.1038/s41562-020-0833-x
Hainmueller, J., Mummolo, J., & Xu, Y. (2019). How much should I trust estimates from multiplicative interactionmodels? simple tools to improve empirical practice. Political Analysis, 27(2), 163–192. https://doi.org/10.1017/pan.2018.46
Hassan, A., & Barber, S. J. (2021). The effects of repetition frequency on the illusory truth effect. CognitiveResearch: Principles and Implications, 6(1). https://doi.org/10.1186/s41235-021-00301-5
Hatmaker, T. (2023, January 13). Instagram and Facebook introduce more limits on targeting teens with ads. TechCrunch. https://techcrunch.com/2023/01/10/instagram-and-facebook-introduce-more-limits-on-targeting-teens-with-ads/
Henderson, E. L., Simons, D. J., & Barr, D. J. (2021). The trajectory of truth: A longitudinal study of the illusory truth effect. Journal of Cognition, 4(1). https://doi.org/10.5334/joc.161
Hess, T. M., Smith, B. T., & Sharifian, N. (2016). Aging and effort expenditure: The impact of subjective perceptions of task demands. Psychology and Aging, 31(7), 653–660. https://doi.org/10.1037/pag0000127
Hilgard, J. (2021). Maximal positive controls: A method for estimating the largest plausible effect size. Journal of Experimental Social Psychology, 93, 104082. https://doi.org/10.1016/j.jesp.2020.104082
Judd, C. M., Westfall, J., & Kenny, D. A. (2012). Treating stimuli as a random factor in social psychology: A new and comprehensive solution to a pervasive but largely ignored problem. Journal of Personality and Social Psychology, 103(1), 54–69. https://doi.org/10.1037/a0028347
King, J. M. (2022). Microtargeted political ads: An intractable problem. Boston University Law Review, 102, 1129. https://www.bu.edu/bulawreview/files/2022/04/KING.pdf
Kristal, A. S., & Santos, L. R. (2021). G.I. Joe phenomena: Understanding the limits of metacognitive awareness on debiasing (No. 21-084). Harvard Business School Working Paper. https://www.hbs.edu/faculty/Pages/item.aspx?num=59722
Law, S., Hawkins, S. A., & Craik, F. I. (1998). Repetition-induced belief in the elderly: Rehabilitating age-related memory deficits. Journal of Consumer Research, 25(2), 91–107. https://doi.org/10.1086/209529
Lyons, B., Montgomery, J., & Reifler, J. (2023). Partisanship and older Americans’ engagement with dubious political news. OSF Preprints. https://doi.org/10.31219/osf.io/etb89
Miratrix, L. W., Sekhon, J. S., Theodoridis, A. G., & Campos, L. F. (2018). Worth weighting? How to think about and use weights in survey experiments. Political Analysis, 26(3), 275–291. Https://doi.org/10.1017/pan.2018.1
Mitchell, A., Gottfried, J., Kiley, J., Matsa, K. E. (2014). Political polarization & media habits. Pew Research Center. https://www.pewresearch.org/wp-content/uploads/sites/8/2014/10/Political-Polarization-and-Media-Habits-FINAL-REPORT-7-27-15.pdf
Moore, R. C., Dahlke, R., & Hancock, J. T. (2023). Exposure to untrustworthy websites in the 2020 US election. Nature Human Behaviour. https://doi.org/10.1038/s41562-023-01564-2
Moore, R. C., & Hancock, J. T. (2022). A digital media literacy intervention for older adults improves resilience to fake news. Scientific Reports, 12, 6008. https://doi.org/10.1038/s41598-022-08437-0
Mutter, S. A., Lindsey, S. E., & Pliske, R. M. (1995). Aging and credibility judgment. Aging, Neuropsychology, and Cognition, 2(2), 89–107. https://doi.org/10.1080/13825589508256590
Mutz, D., & Pemantle, R. (2012). The perils of randomization checks in the analysis of experiments [Unpublished manuscript]. University of Pennsylvania. https://repository.upenn.edu/asc_papers/742
Osmundsen, M., Bor, A., Vahlstrup, P. B., Bechmann, A., & Petersen, M. B. (2021). Partisan polarization is the primary psychological motivation behind political fake news sharing on Twitter. American Political Science Review, 115(3), 999–1015. https://doi.org/10.1017/S0003055421000290
Parks, C. M., & Toth, J. P. (2006). Fluency, familiarity, aging, and the illusion of truth. Aging, Neuropsychology, and Cognition, 13(2), 225–253. https://doi.org/10.1080/138255890968691
Pennycook, G., Binnendyk, J., Newton, C., & Rand, D. G. (2021). A practical guide to doing behavioral research on fake news and misinformation. Collabra: Psychology, 7(1), 25293. https://doi.org/10.1525/collabra.25293
Pennycook, G., & Rand, D. G. (2019). Fighting misinformation on social media using crowdsourced judgments of news source quality. Proceedings of the National Academy of Sciences, 116(7), 2521–2526. https://doi.org/10.1073/pnas.1806781116
Pennycook, G., Cannon, T. D., & Rand, D. G. (2018). Prior exposure increases perceived accuracy of fake news.Journal of Experimental Psychology: General, 147(12), 1865–1880. https://doi.org/10.1037/xge0000465
Pereira, A., Harris, E., & Van Bavel, J. J. (2023). Identity concerns drive belief: The impact of partisan identity on the belief and dissemination of true and false news. Group Processes & Intergroup Relations, 26(1), 24–47. https://doi.org/10.1177/13684302211030004
Pillai, R. M., & Fazio, L. K. (2021). The effects of repeating false and misleading information on belief. WIREs Cognitive Science, 12(6), e1573. https://doi.org/10.1002/wcs.1573
Salthouse, T. A. (2009). When does age-related cognitive decline begin? Neurobiology of Aging, 30(4), 507–514. https://doi.org/10.1016/j.neurobiolaging.2008.09.023
Schäfer, T., & Schwarz, M. A. (2019). The meaningfulness of effect sizes in psychological research: Differences between sub-disciplines and the impact of potential biases. Frontiers in Psychology, 10. https://doi.org/10.3389/fpsyg.2019.00813
Sears, D. O., & Funk, C. L. (1999). Evidence of the long-term persistence of adults’ political predispositions. The Journal of Politics, 61(1). https://doi.org/10.2307/2647773
Simmons, J. P., Nelson, L. D., & Simonsohn, U. (2011). False-positive psychology: Undisclosed flexibility in data collection and analysis allows presenting anything as significant. Psychological Science, 22, 1359–1366. https://doi.org/10.1177/0956797611417632
Simonsohn, U., Nelson, L. D., & Simmons, J. P. (2014). P-curve: A key to the file-drawer. Journal of Experimental Psychology: General, 143(2), 534–547. https://doi.org/10.1037/a0033242
Smelter, T. J., & Calvillo, D. P. (2020). Pictures and repeated exposure increase perceived accuracy of news headlines. Applied Cognitive Psychology, 34(5), 1061–1071. https://doi.org/10.1002/acp.3684
Stoker, L., & Jennings, M. K. (2008). Of time and the development of partisan polarization. American Journal of Political Science, 52(3), 619–635. https://doi.org/10.1111/j.1540-5907.2008.00333.x
Unkelbach, C. (2007). Reversing the truth effect: Learning the interpretation of processing fluency in judgments of truth. Journal of Experimental Psychology: Learning, Memory, and Cognition, 33(1), 219–230.https://doi.org/10.1037/0278-7393.33.1.219
Unkelbach, C., Koch, A., Silva, R. R., & Garcia-Marques, T. (2019). Truth by repetition: Explanations and implications. Current Directions in Psychological Science, 28(3), 247–253. https://doi.org/10.1177/0963721419827854
Vellani, V., Zheng, S., Ercelik, D., & Sharot, T. (2023). The illusory truth effect leads to the spread of misinformation. Cognition, 236, 105421. https://doi.org/10.1016/j.cognition.2023.105421
Funding
This study was funded by the European Research Council (ERC) under the European Union’s Horizon 2020 research and innovation programme (grant agreement no. 682758) as well as the Democracy Fund.
Competing Interests
The author reports no competing interests.
Ethics
This research was approved by the institutional review boards of the University of Exeter, the University of Michigan, Princeton University, and Washington University in St. Louis.
Copyright
This is an open access article distributed under the terms of the Creative Commons Attribution License, which permits unrestricted use, distribution, and reproduction in any medium, provided that the original author and source are properly credited.
Data Availability
All materials needed to replicate this study are available via the Harvard Dataverse: https://doi.org/10.7910/DVN/MGBREI and OSF: https://osf.io/rsbvm/
Acknowledgements
Thanks to Sam Luks and Marissa Shih at YouGov for survey assistance. Special thanks to Andy Guess, Jacob Montgomery, Brendan Nyhan, and Jason Reifler as collaborators on the broader project’s survey design and data collection and for additional feedback. All conclusions and any errors are my own.