Peer Reviewed
Journalistic interventions matter: Understanding how Americans perceive fact-checking labels
Article Metrics
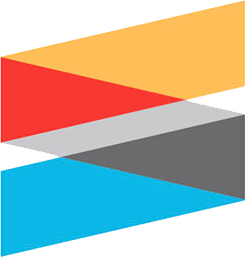
0
CrossRef Citations
Altmetric Score
PDF Downloads
Page Views
While algorithms and crowdsourcing have been increasingly used to debunk or label misinformation on social media, such tasks might be most effective when performed by professional fact checkers or journalists. Drawing on a national survey (N = 1,003), we found that U.S. adults evaluated fact-checking labels created by professional fact checkers as more effective than labels by algorithms and other users. News media labels were perceived as more effective than user labels but not statistically different from labels by fact checkers and algorithms. There was no significant difference between labels created by users and algorithms. These findings have implications for platforms and fact-checking practitioners, underscoring the importance of journalistic professionalism in fact-checking.
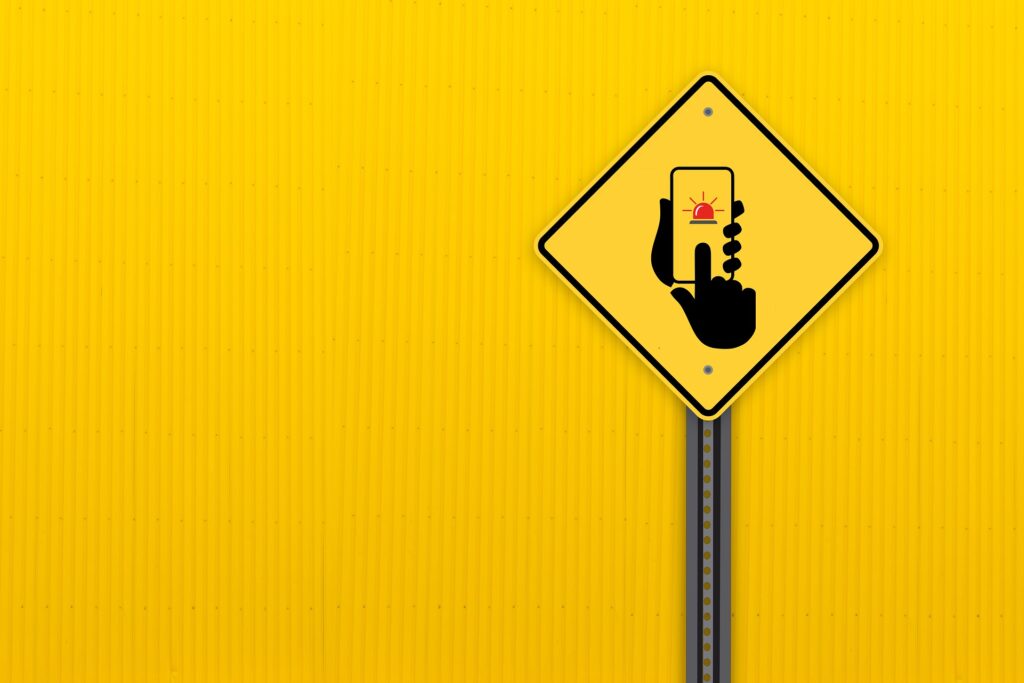
Research Questions
- How do people perceive the efficacy of fact-checking labels created by different sources (algorithms, social media users, third-party fact checkers, and news media)?
- Will partisanship, trust in news media, attitudes toward social media, reliance on algorithmic news, and prior exposure to fact-checking labels be associated with people’s perceived efficacy of different fact-checking labels?
- Will people’s prior exposure to fact-checking labels moderate the relationships between people’s trust in news media or attitudes toward social media platforms and label efficacy?
Essay Summary
- To examine how people perceive the efficacy of different types of fact-checking labels, we conducted a national survey of U.S. adults (N = 1,003) in March 2022. The sample demographics are comparable to the U.S. internet population in terms of gender, age, race/ethnicity, education, and income.
- We found that the perceived efficacy of third-party fact checker labels was the highest, which was higher than the perceived efficacy of algorithmic labels and other user labels. The effectiveness of news media labels was perceived as the second highest, but the statistically meaningful difference was detected only with user labels; the perceived efficacy of news media labels was not statistically different from labels by fact checkers and algorithms. There was no significant difference between the labels created by users and algorithms.
- We also found that political and media-related variables are associated with the perceptions of fact-checking labels. Republicans evaluated the effectiveness of all types of fact-checking labels lower than Democrats. News media trust and attitudes toward social media were positively associated with the perceived effectiveness of all types of labels. These findings hold true for Democrats and Republicans in most cases. For Republicans, the positive association between media trust and the perceived efficacy of user labels was not statistically significant, which was the only exception.
- Our findings highlight the importance of institutions enacting journalistic interventions, suggesting the need for closer collaboration between platforms and professional fact checkers, rather than relying too much on automated or crowdsourcing techniques in countering misinformation. To promote conservative users’ trust in fact-checking, professional fact checkers also need to be transparent and objective in their selection of claims to verify.
Implications
As the spread of misinformation on social media has become a deep societal concern in recent years, social media platforms such as Twitter1We did not use its current name (“X”) because our study was conducted when it was named Twitter. and Facebook have taken various interventions to curb such content (Yaqub et al., 2020). One of the interventions that have gained traction is putting a fact-checking label (Kozyreva et al., 2022; Oeldorf-Hirsch et al., 2020)—also known as a “credibility label” (Saltz et al., 2021) and a “veracity label” (Morrow et al., 2021)—on posts that contain false, inaccurate, or misleading information (Saltz et al., 2021). Research on the effects of fact-checking labels provides mixed results: Some found such labels effectively reducing perceived accuracy of false information (Pennycook et al., 2020) and willingness to share such content (Nekmat, 2020; Yaqub et al., 2020), but others found little effects of labels on perceived credibility, sharing intention, or engagement (Bradshaw et al., 2021; Oeldorf-Hirsch et al., 2020; Papakyriakopoulos & Goodman, 2022).
The current study focuses on how people perceive the effectiveness of fact-checking labels attributed to different sources. This line of inquiry is important because it might provide a possible explanation for the mixed findings concerning the effects of fact-checking labels in that people’s evaluation of labels could affect the accuracy evaluation of or engagement with posts containing misinformation. As fact-checking labels on social media are provided by various sources, ranging from institutions such as independent fact checkers (e.g., PolitiFact.com, Snopes.com) and news organizations2News organizations in this study refer to legacy/mainstream news outlets that produce reliable information through strict editorial norms and judgments. Some news organizations such as The Washington Post (https://www.washingtonpost.com/news/fact-checker) and the Associated Press (https://apnews.com/ap-fact-check) provide their own fact-checking instead of relying on third-party fact-checking platforms. to general social media users to algorithms (Lu et al., 2022; Seo et al., 2019; Yaqub et al., 2020), we examined people’s perception of the effectiveness of fact-checking labels based on four different sources: (a) third-party fact checkers, (b) news organizations, (c) algorithms, and (d) social media users (i.e., crowdsourcing or community labels). We asked participants to rate their perceived efficacy of each fact-checking label after showing them a visual example of how social media platforms label posts containing misleading or inaccurate information so that they could understand what we meant by fact-checking labels. Source identification and credibility have long been known to play a critical role in the evaluation of information like news content (Chaiken, 1980; Hovland & Weiss, 1951). Similarly, social media users might evaluate fact-checking labels based on the source issuing the labels (Oeldorf-Hirsch et al., 2020). Especially when the sources are different in terms of expertise (e.g., professional fact checkers/journalists vs. peer users) and decision-making agents (human vs. machines), people may perceive the effectiveness of labels differently. To be specific, individuals might perceive the labels by institutions (e.g., professionalfact checkers or journalists) to be more legitimate than crowdsourcing labels as research showed that correction by an expert fact checker successfully reduced misperceptions, whereas correction by a peer user failed to do so (Vraga & Bode, 2017). It is also possible that people may perceive algorithmic labels to be more effective compared to the labels by fact checkers or peer users given that people tend to perceive decisions by machines or algorithms as more objective, politically unbiased, and credible than those by humans (Dijkstra et al., 1998; Sundar, 2008; Sundar & Kim, 2019).
Our findings show that third-party fact checker labels were perceived as the most effective, although their efficacy was not significantly different from that of news media labels. The news media in this study refers to legacy/mainstream news organizations that produce reliable information through strict editorial norms and judgments. These results suggest that people put more faith in institutions especially equipped with journalistic professionalism and expertise than algorithms or peer users in terms of verifying facts.3These findings should be interpreted with caution as people, especially partisans, could have had different understandings of news media and fact checkers when evaluating the label sources. As Graves (2016) pointed out, fact-checking is a novel genre of journalism, enacting the journalistic practice of objectivity norms. In recent years, researchers and platforms have attempted various interventions including algorithmic misinformation detection (Jia et al., 2020; Seo et al., 2019; Yaqub et al., 2020) and crowdsourcing labels (Epstein et al., 2020; Godel et al., 2021) because professional fact checkers and journalists cannot intervene in every piece of misinformation. Arguably, the emergence of large language models such as ChatGPT achieves a decent accuracy rate in discerning false information (Bang et al., 2023; Lee & Jia, 2023), but automated and crowdsourcing techniques may not take the lead over “journalistic interventions” (Amazeen, 2020) as our findings suggest. In this light, relying too much on automated and crowdsourcing techniques could be less effective in curbing misinformation, which could also erode people’s trust in fact-checking practice itself.
We also investigated various individual-level factors that might influence the evaluation of fact-checking labels. Past studies have focused primarily on individual characteristics that make people fall prey to misinformation, but little is known about individual-level differences related to people’s perception of fact-checking labels. One of the notable findings in this regard is partisan asymmetry: Republicans exhibited higher skepticism toward all types of fact-checking labels compared to Democrats. This aligns with previous findings that Republicans oppose fact-checking labels in general (Saltz et al., 2021) and that accuracy nudge interventions are less effective for Republicans than Democrats (Pennycook et al., 2022). It is also known that Republicans tend to accuse fact checkers (Jennings & Stroud, 2021), news media outlets (Hemmer, 2016), and social media platforms (Vogels et al., 2020) of being liberally biased, and such sentiment could translate into their perceptions of fact-checking label efficacy.
Another noteworthy finding is the positive relationship between the perceived effectiveness of all types of labels and news media trust. One possible explanation is that people who have higher trust in news media are likely to care more about facts and truth and have more faith in the verification process, and thus, may show more support for fact-checking labels in general (Saltz et al., 2021). It is also worth noting that the positive relationships between news media trust and the efficacy of labels by both news media and fact checkers became stronger for those who were exposed to such labels more frequently. These findings suggest that raising the visibility of fact-checking labels can help increase their effectiveness, especially among those who trust news media.
In addition, we found positive relationships between people’s attitudes toward social media and the perceived effectiveness of all types of labels. For algorithmic and user fact-checking labels, in particular, the positive relationships became stronger among those more familiar with such labels. This is partly because people with favorable attitudes toward social media platforms are more likely to be gratified with algorithms (Kim & Kim, 2019) and crowdsourcing (Bozarth et al., 2023), one of the main features of these platforms. Conversely, however, those with negative attitudes toward social media could distrust fact-checking labels altogether, regardless of their sources.
These results have practical implications for social media platforms and fact-checking practitioners. As people trust institutional fact checkers more than algorithms or peer users, platforms need to keep collaborating with fact-checking organizations and news outlets, along with developing and implementing misinformation detection algorithms and crowdsourcing techniques (e.g., Twitter’s Community Notes). Platforms might also consider making fact-checking labels by professional fact checkers more visible by changing their content recommendation algorithms. However, those who use social media and rely on algorithms for news more frequently are more likely to trust labels by algorithms and other users, which suggests that platforms should strive to boost the accuracy of algorithmic and crowdsourcing labels because such users could blindly believe these labels.
To build trust in misinformation interventions among Republicans skeptical about fact-checking labels altogether, platforms should increase transparency around their intervention decisions and be more open to oversight and regulations from the outside (Saltz et al., 2021). Given that Republicans often blame fact checkers’ partisan bias for choosing statements favorable to Democrats, fact checkers also need to select claims to verify based on clear criteria to foster Republicans’ trust in fact-checking.
Considering the positive relationship between news media trust and the effectiveness of all types of labels as well as the role of label exposure in strengthening such relationships, it is necessary to regulate untrustworthy sources masquerading as legitimate news outlets on social media and increase users’ familiarity with fact-checking labels verified by credible journalistic institutions. These strategies will ultimately help foster positive attitudes toward social media platforms among the public, which could also translate into their perceptions of fact-checking labels as the results suggested.
Findings
Finding 1: Third-party fact checker labels were perceived as more effective than algorithmic labels and other user labels.
Our first research question explores how people perceive different fact-checking labels. A one-way ANOVA was conducted to test the difference in perceived effectiveness across four types of labels. As shown in Figure 1, there were significant differences across four types of labels, F (3, 4008) = 12.10, p < .001, partial η2 = .01. A series of post hoc comparisons using the Bonferroni test showed that labels provided by third-party fact checkers (M = 4.24, SD = 1.36) were perceived to be more effective than those provided by algorithms (M = 4.02, SD = 1.34, p = .003) and other users (M = 3.88, SD = 1.39, p < .001), but fact checker labels were not significantly different from those provided by news media (M = 4.12, SD = 1.37, p = .32). Labels provided by news media were perceived to be more effective than those provided by other users (p < .001) but had no significant difference with algorithmic labels (p = .70). Although user fact-checking labels were perceived as the least effective among four labels, there was no significant difference between algorithmic labels and user labels (p = .13).
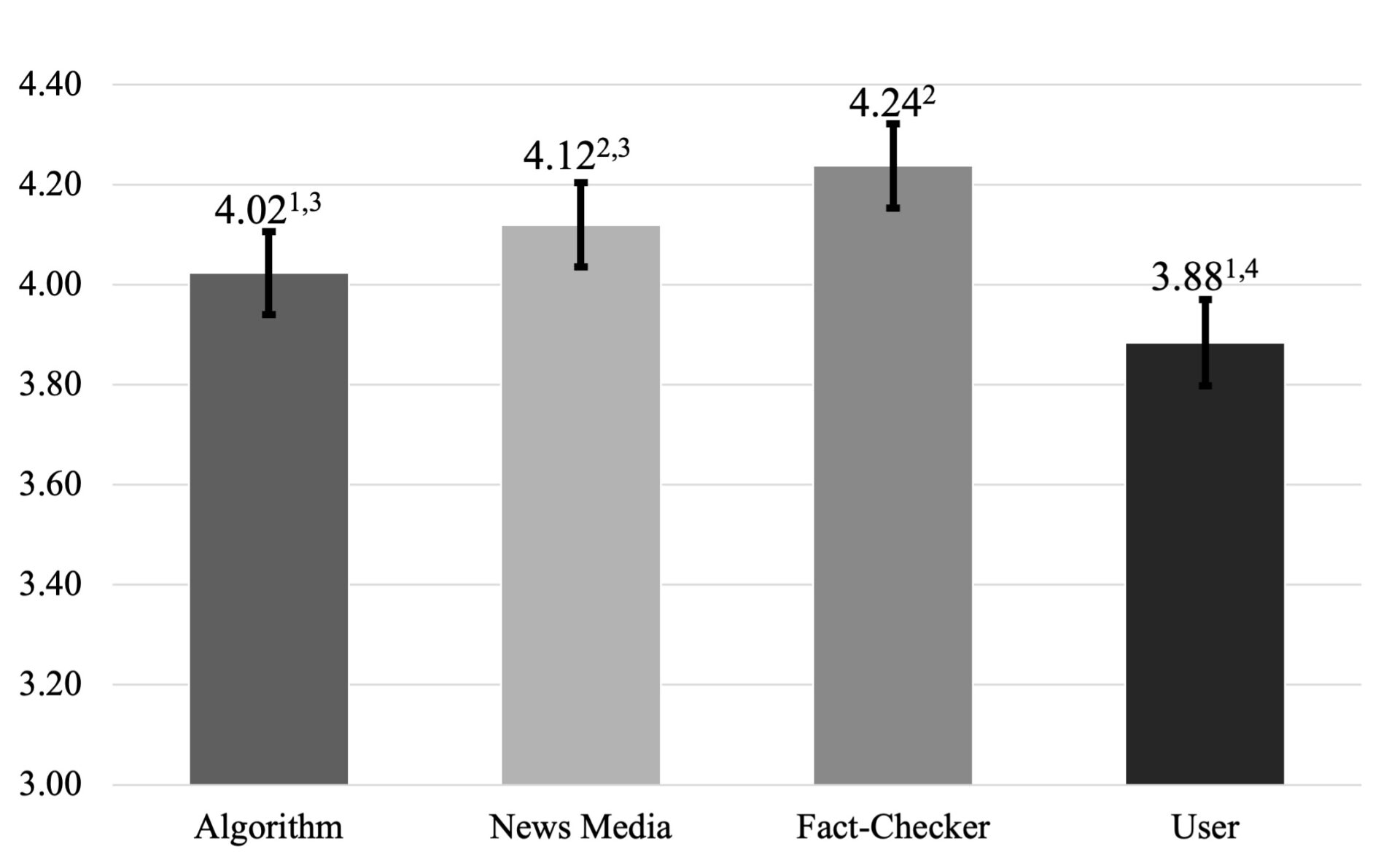
Finding 2: Republicans rated the effectiveness of all types of fact-checking labels lower than Democrats.
To answer RQ2, we explored factors that can predict people’s different perceptions of fact-checking labels. A two-way ANOVA showed that Republicans evaluated the effectiveness of all types of fact-checking labels lower than Democrats. The main effects of party self-identification [F (2, 4000) = 123.51, p < .001, partial η2 = .06] and label type [F (3, 4000) = 11.75, p < .001, partial η2 = .01] on label efficacy were significant for both parties. Several post hoccomparisons using the Bonferroni test indicated that Republicans rated the effectiveness of all types of fact-checking labels significantly lower than Democrats (p < .001). Specific means and SDs are listed in Table 2 in Appendix A.
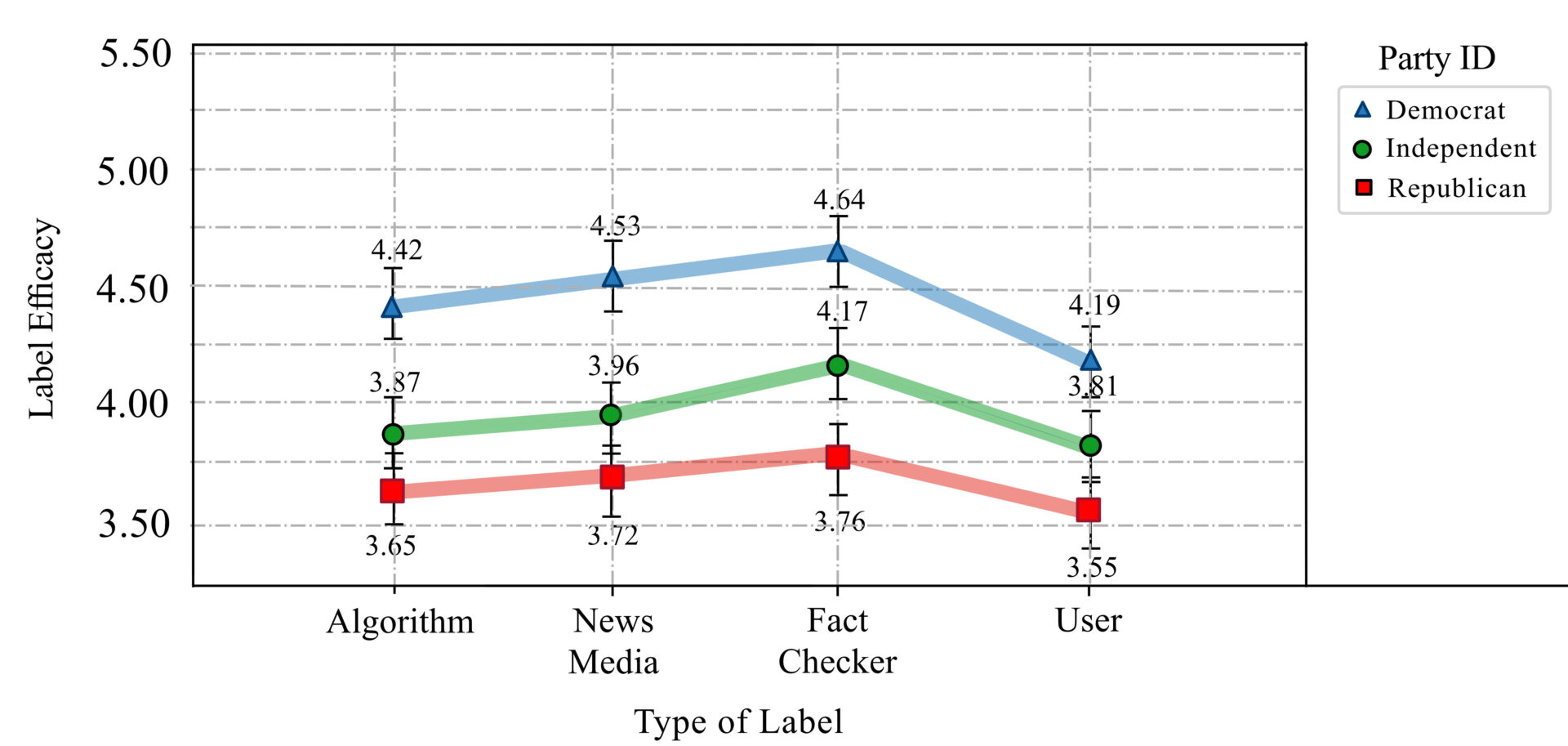
Finding 3: People’s trust in news media and attitudes toward social media platforms were positively associated with the perceived effectiveness of all types of fact-checking labels, but social media use and reliance on algorithms to get news were only positively associated with two types of labels.
A series of OLS regression analyses showed that news media trust was positively associated with the perceived effectiveness of fact-checking labels regardless of the sources (algorithm: b = .25, SE = .03, p < .001; news media: b= .35, SE = .03, p < .001; fact checker: b = .24, SE = .03, p < .001; user: b = .16, SE = .03, p < .001). Attitudes toward social media platforms were also positively associated with the perceived effectiveness of fact-checking labels across all four sources (algorithm: b = .21, SE = .03, p < .001; news media: b = .20, SE = .03, p < .001; fact checker: b = .20, SE = .03, p < .001; user: b = .23, SE = .03, p < .001). Additional analyses showed that such results hold true for both Democrats and Republicans. The only exception was that the positive association between media trust and perceived efficacy of user labels was not statistically significant for Republicans (b = .11, SE = .07, p = .13; see Table 3 in Appendix A for details). The frequency of social media use was positively associated with perceived effectiveness of fact-checking labels made by (a) algorithms (b = .08, SE = .04, p = .08) and (b) other social media users (b = .10, SE = .03, p = .03) but not significantly associated with the other labels (news media: b = .07, SE = .03, p = .10; fact-checker: b = .04, SE = .03, p =.42). People’s reliance on algorithms to find news was positively associated with both fact-checking labels made by algorithms (b = .08, SE = .03, p = .03) and other users (b = .16, SE = .03, p < .001).
Finding 4: People’s prior exposure to fact-checking labels strengthened the relationships between people’s trust in news media or attitudes toward social media platforms and label efficacy.
Lastly, to answer RQ3, we tested two interaction effects—trust in news media x prior exposure to fact-checking labels and attitudes toward social media platforms x prior exposure—on the perceived efficacy of the different labels. Following Saltz et al. (2021), we expected that people who have encountered labels more frequently would be more familiar with and potentially have more positive attitudes towards labels, thereby strengthening the relationships between either social media attitudes or news media trust and the perceived effectiveness of different fact-checking labels. We found significant interaction effects between people’s news media trust and prior exposure to fact-checking labels on their evaluation of labels by (a) fact checkers (b = .35, SE = .01, p < .001) and (b) news media (b = .38, SE = .01, p < .001). Specifically, the positive relationships between news media trust and the perceived effectiveness of labels by both news media and fact checkers became stronger for those who reported high in prior exposure to such labels, as shown in Figure 3.
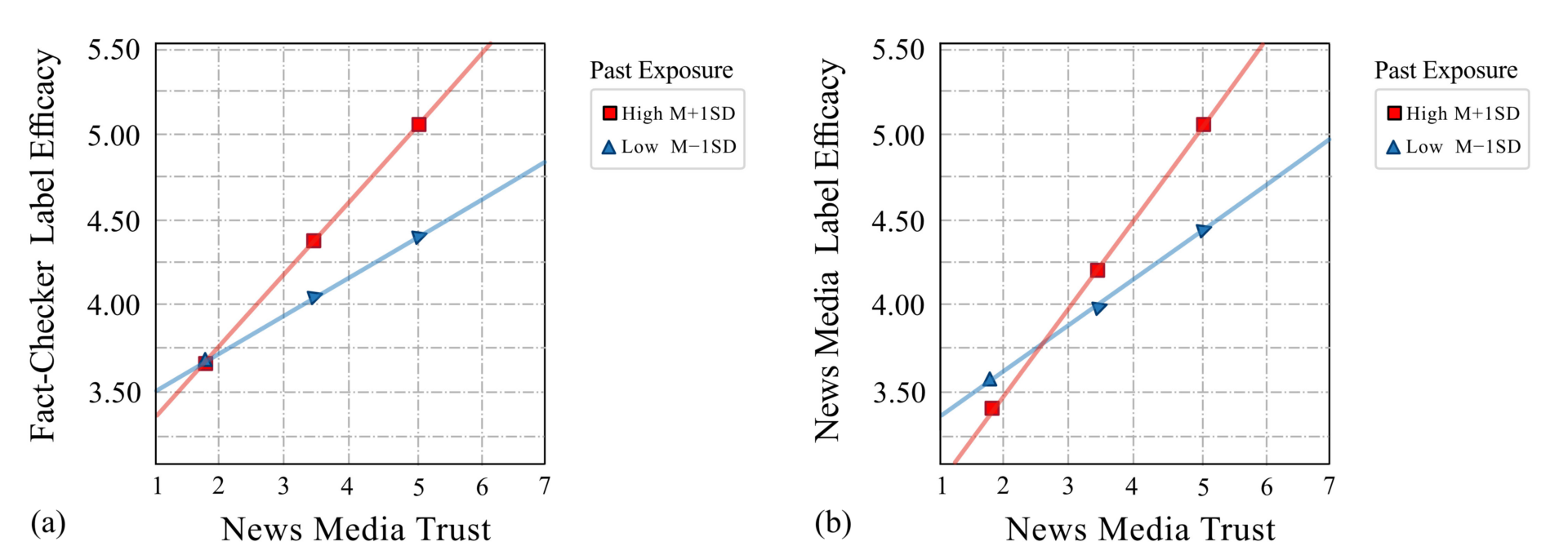
Results also showed significant interaction effects of people’s attitudes toward social media platforms and prior exposure to fact-checking labels on their evaluation of (a) algorithmic labels (b = .40, SE = .01, p < .001) and (b) user labels (b = .25, SE = .01, p = .01). Specifically, the positive relationships between people’s attitudes toward social media platforms and the perceived effectiveness of (a) algorithmic and (b) user fact-checking labels became stronger for those who reported high in prior exposure to such labels (Figure 4).
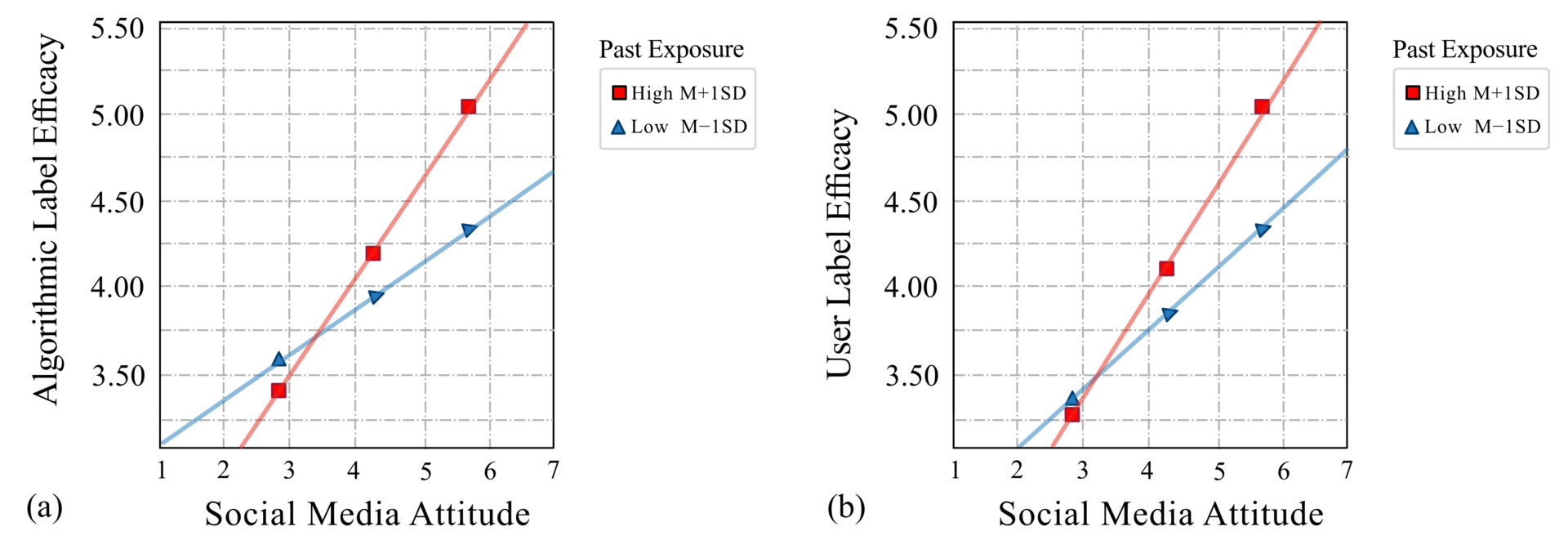
Methods
We conducted a national survey of U.S. adults (N = 1,003) in March 2022. Respondents were recruited online by Dynata (formerly known as Survey Sampling International, SSI), which maintains a large online panel of U.S. adults. The demographic quotas were established to reflect the U.S. population in terms of gender, age, race/ethnicity, education, and income, and our sample is comparable to the U.S. internet population (see Table 1 in Appendix B).
The dependent variable of the study Perceived Efficacy of Fact-checking Labels4See Table 2 in Appendix B for question wording, scales, means, standard deviations, and reliability of the variables used in this study. was measured by asking respondents to rate both effectiveness and confidence (1 = extremely ineffective/unconfident, 7 = extremely effective/confident) for each fact-checking label created by different sources (i.e., algorithms, social media users, third-party fact checkers, and news media), and averaged into four separate indices, following previous research (Moravec et al., 2020). The order of each label source was randomized to avoid any order effects. For participants to understand what we meant by fact-checking labels, we provided an explanation and an example (see Figure 1 in Appendix B).
News Credibility was measured using five items (i.e., the news media are fair, unbiased, accurate, tell the whole story, separate facts from opinions) adapted from Gaziano and McGrath (1986). To measure Reliance on Algorithmic News, we asked respondents to indicate how much they agree or disagree with the following two statements adapted from Gil de Zúñiga and Cheng (2021) and Lee et al. (2023): I rely on social media algorithms 1) to tell me what’s important when news happens, 2) to provide me with important news and public affairs. Respondents were also asked to indicate their party identification on a 7-point scale (1 = strong Republican, 2 = weak Republican, 3 = lean Republican, 4 = independent, 5 = lean Democrat, 6 = weak Democrat, 7 = strong Democrat). Republicans were coded 1–3 (n = 296), Democrats 5–7 (n = 403), and independents as 4 (n = 304).
For Attitudes toward Social Media, participants rated their favorability towards four different platforms (Facebook, Twitter, Instagram, and YouTube) (1 = very unfavorable, 7 = very favorable), drawn from Ahluwalia et al. (2000), which were averaged together. An item (adapted from Saltz et al., 2021), asking how often participants have encountered fact-checking labels in any of their social media feeds since the 2020 U.S. presidential election (1 = never, 7 = very frequently, 8 = not sure) was used to measure Prior Exposure to Fact-checking Labels. Those who chose “notsure” (n = 76) were excluded from the regression models.
Lastly, demographics such as age (M = 46.08, SD = 16.94), education (measured as the last degree respondents completed (ranging from 1 = “less than high school degree” to 3 = “college graduate or more;” M = 1.98, SD = .82), and household income (ranging from 1 = “less than $30,000” to 6 = “$150, 000 or more”; M = 2.91, SD = 1.69) were measured and controlled for analysis.
Topics
Bibliography
Ahluwalia, R., Burnkrant, R. E., & Unnava, H. R. (2000). Consumer response to negative publicity: The moderating role of commitment. Journal of Marketing Research, 37(2), 203–214. https://doi.org/10.1509/jmkr.37.2.203.1873
Bang, Y., Cahyawijaya, S., Lee, N., Dai, W., Su, D., Wilie, B., Lovenia, H., Ji, Z., Yu, T., Chung, W., & Fung, P. (2023). A multitask, multilingual, multimodal evaluation of ChatGPT on reasoning, hallucination, and interactivity. Proceedings of the 13th International Joint Conference on Natural Language Processing and the 3rd Conference of the Asia-Pacific Chapter of the Association for Computational Linguistics, Volume 1: Long Papers (pp. 675–718). Association for Computational Linguistics. https://aclanthology.org/2023.ijcnlp-main.45
Bozarth, L., Im, J., Quarles, C., & Budak, C. (2023). Wisdom of two crowds: Misinformation moderation on Reddit and how to improve this process—A case study of COVID-19. Proceedings of the ACM on Human-Computer Interaction, 7(CSCW1), 1–33. https://doi.org/10.1145/3579631
Bradshaw, S., Elswah, M., & Perini, A. (2021). Look who’s watching: Platform labels and user engagement on state-backed media outlets. American Behavioral Scientist. https://doi.org/10.1177/00027642231175639
Chaiken, S. (1980). Heuristic versus systematic information processing and the use of source versus message cues in persuasion. Journal of Personality and Social Psychology, 39(5), 752–766. https://doi.org/10.1037//0022-3514.39.5.752
Dijkstra, J. J., Liebrand, W. B. G., & Timminga, E. (1998). Persuasiveness of expert systems. Behaviour & Information Technology, 17(3), 155–163. https://doi.org/10.1080/014492998119526
Epstein, Z., Pennycook, G., & Rand, D. (2020). Will the crowd game the algorithm? Using layperson judgments to combat misinformation on social media by downranking distrusted sources. In CHI ’19: Proceedings of the 2020 CHI conference on human factors in computing systems (pp. 1–11). Association for Computing Machinery. https://doi.org/10.1145/3313831.3376232
Gaziano, C., & McGrath, K. (1986). Measuring the concept of credibility. Journalism & Mass Communication Quarterly, 63, 451–462. https://doi.org/10.1177/10776990860630030
Gil de Zúñiga, H., & Cheng, Z. (2021). Origin and evolution of the News Finds Me perception: Review of theory and effects. Profesional de la información, 30(3), e300321. https://doi.org/10.3145/epi.2021.may.21
Godel, W., Sanderson, Z., Aslett, K., Nagler, J., Bonneau, R., Persily, N., & Tucker, J. A. (2021). Moderating with the mob: Evaluating the efficacy of real-time crowdsourced fact-checking. Journal of Online Trust and Safety, 1(1). https://doi.org/10.54501/jots.v1i1.15
Graves, L. (2016). Deciding what’s true: The rise of political fact-checking in American journalism. Columbia University Press.
Hemmer, N. (2016). Messengers of the right: Conservative media and the transformation of American politics. University of Pennsylvania Press.
Hovland, C. I., & Weiss, W. (1951). The influence of source credibility on communication effectiveness. Public Opinion Quarterly, 15, 635–650. https://doi.org/10.1086/266350
Jennings, J., & Stroud, N. J. (2021). Asymmetric adjustment: Partisanship and correcting misinformation on Facebook. New Media & Society. https://doi.org/10.1177/14614448211021720
Jia, C., Boltz, A., Zhang, A., Chen, A., & Lee, M. K. (2022). Understanding effects of algorithmic vs. community label on perceived accuracy of hyper-partisan misinformation. Proceedings of the ACM on Human-Computer Interaction, 6(CSCW2), 1–27. https://doi.org/10.1145/3555096
Kim, B., & Kim, Y. (2019). Facebook versus Instagram: How perceived gratifications and technological attributes are related to the change in social media usage. The Social Science Journal, 56(2), 156–167. https://doi.org/10.1016/j.soscij.2018.10.002
Kozyreva, A., Lorenz-Spreen, P., Herzog, S., Ecker, U., Lewandowsky, S., & Hertwig, R. (2022). Toolbox of interventions against online misinformation and manipulation. PsyArXiv. https://psyarxiv.com/x8ejt
Lee, T. & Jia, C. (2023). Curse or cure? The role of algorithm in promoting or countering information disorder. In M. Filimowicz. (Ed.) Algorithms and society: Information disorder (pp. 29–45). Routledge. https://doi.org/10.4324/9781003299936-2
Lee, T., Johnson, T., Jia, C., & Lacasa-Mas, I. (2023). How social media users become misinformed: The roles of news-finds-me perception and misinformation exposure in COVID-19 misperception. New Media & Society. https://doi.org/10.1177/14614448231202480
Lu, Z., Li, P., Wang, W., & Yin, M. (2022). The effects of AI-based credibility indicators on the detection and spread of misinformation under social influence. Proceedings of the ACM on Human-Computer Interaction, 6(CSCW2), 1–27. https://doi.org/10.1145/3555562
Morrow, G., Swire-Thompson, B., Polny, J., Kopec, M., & Wihbey, J. (2020). The emerging science of content labeling: Contextualizing social media content moderation. SSRN. http://dx.doi.org/10.2139/ssrn.3742120
Nekmat, E. (2020). Nudge effect of fact-check alerts: Source influence and media skepticism on sharing of news misinformation in social media. Social Media + Society, 6(1). https://doi.org/10.1177/2056305119897322
Oeldorf-Hirsch, A., Schmierbach, M., Appelman, A., & Boyle, M. P. (2020). The ineffectiveness of fact-checking labels on news memes and articles. Mass Communication and Society, 23(5), 682–704. https://doi.org/10.1080/15205436.2020.1733613
Papakyriakopoulos, O., & Goodman, E. (2022, April). The Impact of Twitter labels on misinformation spread and user engagement: Lessons from Trump’s election tweets. In WWW ’22: Proceedings of the ACM web conference 2022 (pp. 2541–2551). Association for Computing Machinery. https://doi.org/10.1145/3485447.3512126
Pennycook, G., McPhetres, J., Zhang, Y., Lu, J. G., & Rand, D. G. (2020). Fighting COVID-19 misinformation on social media: Experimental evidence for a scalable accuracy-nudge intervention. Psychological Science, 31(7), 770–780. https://doi.org/10.1177/0956797620939054
Pennycook, G., & Rand, D. G. (2022). Accuracy prompts are a replicable and generalizable approach for reducing the spread of misinformation. Nature Communications, 13(1), 2333. https://doi.org/10.1038/s41467-022-30073-5
Saltz, E., Barari, S., Leibowicz, C., & Wardle, C. (2021). Misinformation interventions are common, divisive, and poorly understood. Harvard Kennedy School (HKS) Misinformation Review, 2(5). https://doi.org/10.37016/mr-2020-81
Seo, H., Xiong, A., & Lee, D. (2019). Trust it or not: Effects of machine-learning warnings in helping individuals mitigate misinformation. In WebSci ’19: Proceedings of the 10th ACM conference on web science (pp. 265–274). Association for Computing Machinery. https://doi.org/10.1145/3292522.3326012
Sundar, S. (2008). The MAIN model: A heuristic approach to understanding technology effects on credibility. In M. J. Metzger & J. Flanagin (Eds.), Digital media, youth, and credibility (pp. 72–100). MIT Press.
Sundar, S. S., & Kim, J. (2019). Machine heuristic: When we trust computers more than humans with our personal information. In CHI ’19: Proceedings of the 2019 CHI conference on human factors in computing systems (pp. 1–9). Association for Computing Machinery. https://doi.org/10.1145/3290605.3300768
Vogels, E. A., Perrin, A., & Anderson, M. (2020). Most Americans think social media sites censor political viewpoints. Pew Research Center. https://www.pewresearch.org/internet/2020/08/19/most-americans-think-social-media-sites- censor-political-viewpoints/
Vraga, E. K., & Bode, L. (2017). Using expert sources to correct health misinformation in social media. Science Communication, 39(5), 621–645. https://doi.org/10. 1177/1075547017731776
Yaqub, W., Kakhidze, O., Brockman, M. L., Memon, N., & Patil, S. (2020, April). Effects of credibility indicators on social media news sharing intent. In CHI ’19: Proceedings of the 2020 CHI conference on human factors in computing systems (pp. 1–14). Association for Computing Machinery. https://doi.org/10.1145/3313831.3376213
Funding
The Good Systems Grand Challenge Research effort at The University of Texas at Austin supported this work, which is a project of UT Austin’s Digital Media Research Program.
Competing Interests
The authors declare no competing interests.
Ethics
This research involved human subjects who provided informed consent. The research protocol employed was approved by the institutional review board (IRB, STUDY00002374) at The University of Texas at Austin. The sample demographics are comparable to the U.S. internet population in terms of gender, age, race/ethnicity, education, and income.
Copyright
This is an open access article distributed under the terms of the Creative Commons Attribution License, which permits unrestricted use, distribution, and reproduction in any medium, provided that the original author and source are properly credited.
Data Availability
All materials needed to replicate this study are available via the Harvard Dataverse: https://doi.org/10.7910/DVN/0B3ER9
Authorship
The first and second authors contributed equally to this work and are listed alphabetically.