Peer Reviewed
Twitter flagged Donald Trump’s tweets with election misinformation: They continued to spread both on and off the platform
Article Metrics
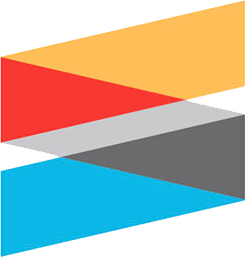
25
CrossRef Citations
Altmetric Score
PDF Downloads
Page Views
We analyze the spread of Donald Trump’s tweets that were flagged by Twitter using two intervention strategies—attaching a warning label and blocking engagement with the tweet entirely. We find that while blocking engagement on certain tweets limited their diffusion, messages we examined with warning labels spread further on Twitter than those without labels. Additionally, the messages that had been blocked on Twitter remained popular on Facebook, Instagram, and Reddit, being posted more often and garnering more visibility than messages that had either been labeled by Twitter or received no intervention at all. Taken together, our results emphasize the importance of considering content moderation at the ecosystem level.
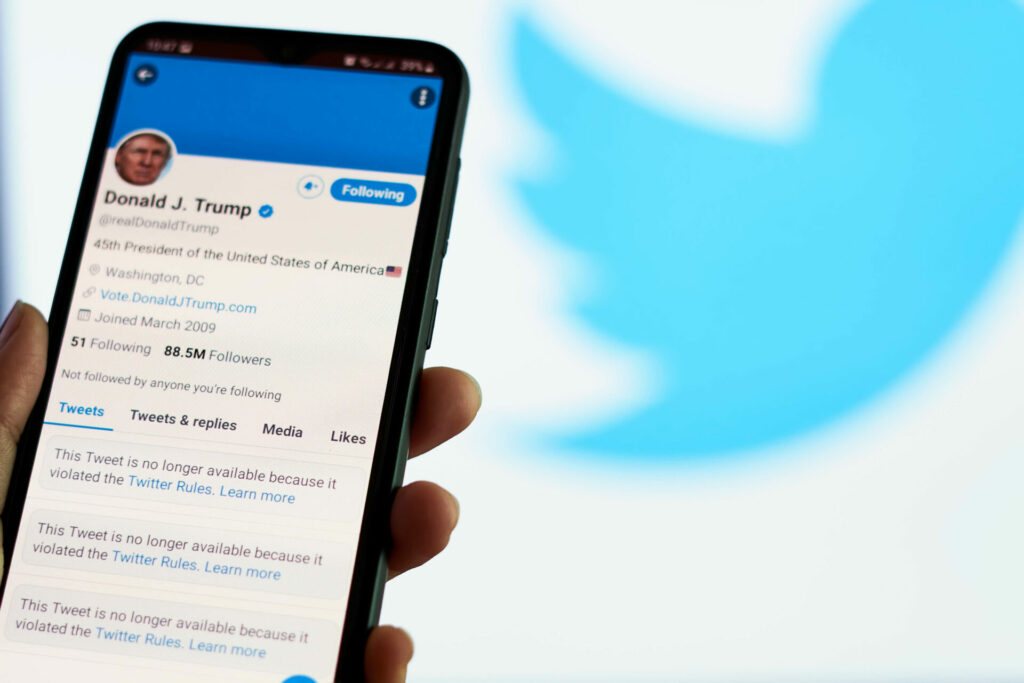
Research Questions
- How did messages flagged by Twitter spread on the platform compared to messages without interventions?
- How did messages flagged by Twitter spread on Facebook, Instagram, and Reddit?
Essay Summary
- We identify tweets from Former President Donald Trump, posted from November 1, 2020 through January 8, 2021, that were flagged by Twitter as containing election-related misinformation. We then collect data from Twitter in order to measure the differential spread of messages that were not flagged and those that were flagged by a warning label or prevented from being engaged with.
- We find that while blocking messages from engagement effectively limited their spread, messages that were flagged by the platform with a warning label spread further and longer than unlabeled tweets.
- To understand the impact of one platform’s intervention on their broader spread, we identify these same messages on Facebook, Instagram, and Reddit and collect data from those platforms.
- We find that messages that had been blocked from engagement on Twitter were posted more often and received more visibility on other popular platforms than messages that were labeled by Twitter or that received no intervention at all.
- These observational data do not enable us to determine whether this finding is a selection effect (i.e., Twitter intervened on posts that were more likely to spread) or causal (Twitter’s intervention increased their spread). It nonetheless provides valuable descriptive evidence of the broad cross-platform diffusion of messages that Twitter had flagged as containing election-related misinformation.
- Our findings underscore the networked nature of misinformation: posts or messages banned on one platform may grow on other mainstream platforms in the form of links, quotes, or screenshots. This study emphasizes the importance of researching content moderation at the ecosystem level, adding new evidence to a growing public and platform policy debate around implementing effective interventions to counteract misinformation.
Implications
Much like four years ago, evidence throughout the 2020 U.S. elections suggests that misinformation related to the presidential campaign once again circulated widely on and offline (Scott & Overly, 2020). These messages were produced by various speakers, covered a wide range of topics, and were distributed within and across different media ecosystems (CIP et al., 2021). Recent work by journalists, civil society, and scholars have provided important new evidence of the role of misinformation in the recent election, building on the substantial body of work around the impact of misinformation on electoral processes (Grinberg et al., 2019; Persily & Tucker, 2020; Tucker et al., 2018). However, important questions remain as to what extent new dynamics for the online information environment were introduced by the 2020 elections (Stroud et al., 2020).
One of the most notable developments was the public commitment by social media platforms, including Facebook, Instagram, and Twitter, to address misinformation in the lead up to and aftermath of November 3, utilizing measures ranging from providing context labels to posts, halting the sharing of posts, and removing posts altogether that contained or linked to election-related misinformation (Conger et al., 2020). There is mixed evidence regarding the effectiveness of interventions in experimental contexts for both decreasing belief in misinformation and for self-reported sharing intentions on social media (Banas & Miller, 2013; Clayton et al., 2019; Freeze et al., 2020; Nyhan, 2021). While new research has added important evidence from studies “in the wild” (e.g., Aslett et al., 2021; Guess et al., 2020)—including a recent study on soft moderation interventions on Twitter (Zannettou, 2021)—we have a limited empirical understanding of the relationship between platform intervention strategies and actual user behavior. Thus, the actions taken by platforms throughout the election period introduce the possibility of contributing not only to the emerging body of research on online interventions, but also to ongoing debates relevant for public and platform policy aimed at improving our information ecosystems.
Here, we focus on the impact of Twitter’s interventions on the diffusion of election-related messaging from former President Donald Trump. We limit ourselves to Twitter and Trump for two reasons. First, we focus on Twitter’s actions not because we believe it is the most impactful platform for American electoral politics, but because of the availability of data on the platform’s interventions. Second, we focus on messages from former President Trump because of evidence that he acted as a central vector for spreading election-relation misinformation (Benkler et al., 2020). Limiting our analysis to Trump also establishes a clearer metric of comparison given Trump’s meteoric popularity on Twitter. Had we pooled Trump’s tweets with those of other politicians—and had Trump accounted for the majority of tweets flagged (which he did)—then findings regarding flagged tweets could have been picking up both the fact that the tweet was flagged plus a ‘Trump effect.’ By limiting ourselves to Trump, we are able to zero out the Trump effect as both our flagged and unflagged groups are composed solely of Trump’s tweets.
On November 12, 2020, Twitter announced that it had labeled roughly 300,000 election-related tweets as disputed and potentially misleading, but did not remove these tweets or block them from spreading (Gadde & Beykpour, 2020). We call this a “soft intervention.” Twitter reported a harsher response to 456 tweets, which it labeled with a warning message while blocking others from retweeting, replying to, or “liking” the tweet. We call this a “hard intervention.” We have provided an example of each in Figure 1.
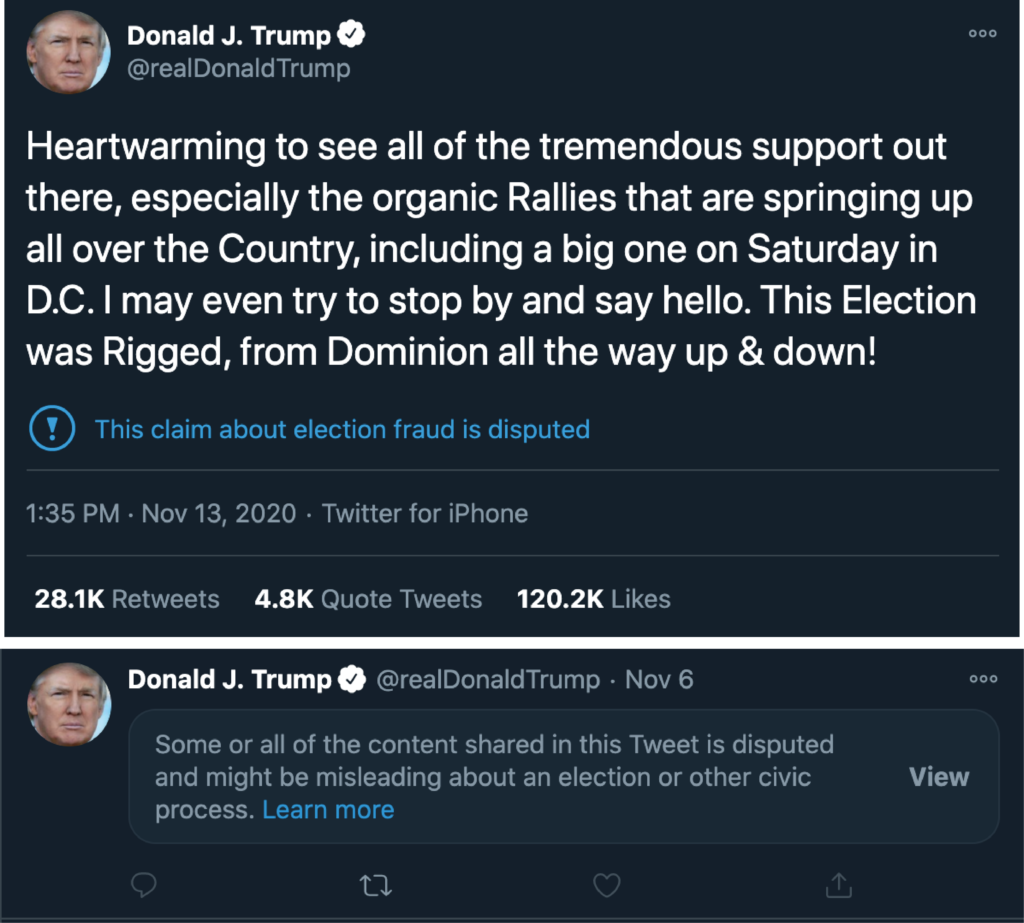
We collected data from November 1, 2020 through January 8, 2021 (the day that Trump’s account was suspended). In our first set of analyses, we measure the extent to which these three categories of messages—soft intervention, hard intervention, and no intervention—spread and were engaged with on Twitter. We find that hard interventions were generally applied to tweets within an hour of publication and that the intervention worked as designed to prevent further spread or engagement. Conversely, Tweets that received a soft intervention spread further, longer, and received more engagement than unflagged tweets.
To be clear, this finding does not necessarily mean that warning labels were ineffective or that they led to a so-called Streisand effect—wherein an attempt to hide or remove information unintentionally draws attention to it. First, it may be the case that the types of tweets Twitter labeled (e.g., falsehoods about the electoral process) were the types of tweets that would have had the highest levels of spread independent of being labeled; nor can we in any way rule out that users might have engaged with them even more if the platform had not applied the label. Second, research suggests that warning labels reduce people’s willingness to believe false information; despite the tweets’ broad exposure, the label could have lowered users’ trust in the false content (Pennycook et al., 2020). However, our data do show the wide reach of election-related messages that the platform had marked as disputed.
We then turn to an analysis of how these messages spread beyond Twitter, specifically across Facebook, Instagram, and Reddit. As Renee DiResta (2021) writes in The Atlantic, “Misinformation is networked; content moderation is not.” In this fragmented moderation landscape, content whose spread is limited on one platform can have wide reach on another. We measure the extent to which Trump’s messages that contained particularly egregious election misinformation — and thus had been prevented from spreading further on Twitter — diffused on these three other popular social media platforms. This approach contributes to a growing body of work that measures the impact of one platform’s actions on the larger informational space, such as a recent study of the effect of YouTube’s intervention on conspiracy videos on conspiracy content across Twitter and Reddit (Buntain et al., 2021).
Notably, we find that the messages that received hard interventions on Twitter diffused more broadly on Facebook, Instagram, and Reddit than messages that received either soft or no interventions. On Facebook, these messages also received more engagement than messages with soft or no interventions. This evidence echoes previous research on users who are suspended from one platform and then migrate to other platforms; similarly, banned posts or messages may grow on other platforms in the form of links, quotes, or screenshots (Ribeiro et al., 2020).
Taken together, these findings introduce compelling new evidence for the limited impact of one platform’s interventions on the cross-platform diffusion of misinformation, emphasizing the need to consider content moderation at an ecosystem level. For state actors, legislative or regulatory actions focused on a narrow band of platforms may fail to curb the broader spread of misinformation. Alarmingly, YouTube has been largely absent from recent Congressional hearings—as well as from academic and journalistic work—even though the platform is broadly popular and served as a vector of election misinformation (Douek, 2020). Platform rules, procedures, and institutions—such as the widely covered Facebook Oversight Board—may also have limited impact if their jurisdiction remains confined to single platforms. Platforms could instead turn their focus to ecosystem-level solutions, such as multi-platform deliberative institutions (Hatmaker, 2021), middleware (Fukuyama et al., 2021), and standards for value-driven algorithmic design (Helberger, 2019). Finally, and most importantly, this study was only possible due to the work of Baumgartner et al. (2020) to open-source Reddit data; Twitter’s recent expansion of research API access; and Facebook’s decision to make certain data, albeit with significant limitations, available through CrowdTangle (CrowdTangle, 2021). Increasing data access—especially data that are consistent across platforms and that are global in scale—is of utmost importance to measuring the ecosystem-level impact of content moderation, including in understudied contexts outside of the United States and Western Europe. While it will face significant legal, social, and technical challenges (Persily & Tucker, 2020), the expansion of data access is necessary to the production of rigorous research that can inform evidence-based public and platform policy.
Our findings introduce novel observational data to the study of social media interventions, which has generally been limited to experimental approaches. Our findings also emphasize the importance of analyzing the impact of platform actions within the context of the larger social media ecosystem. Recent work has shown how Donald Trump’s tweets about election integrity were amplified by cable news (Benkler at al., 2020; Wardle, 2021), underscoring the connection between traditional and social media. Here, we show how content moderation policies on one platform may fail to contain the spread of misinformation when not equally enforced on other platforms. When considering moderation policies, both technologists and public officials should understand that moderation decisions currently operate in a fractured online information environment structured by private platforms with divergent community standards and enforcement protocols.
Findings
Finding 1: While hard interventions limited the further spread of those messages on Twitter, tweets that received a soft intervention spread further than messages that received no intervention at all.
We first measure the diffusion of Trump’s political tweets on Twitter, categorized by whether it received a hard intervention, soft intervention, or no intervention. Figure 2A visualizes the growth in retweets over the 24-hour period after publication. We find that the hard intervention was, on average, applied within an hour of publication, as evidenced by the sharp plateau in the number of cumulative retweets. While these tweets had a higher rate of retweet growth in the first hour than either of the other two message categories, the more severe intervention effectively blocked further spread (i.e., a plateau in a cumulative distribution plot reveals no further spread). Conversely, while average retweets for the other two categories experienced similar rates of increase over the first two hours, tweets that received a soft intervention continued to spread longer and further than tweets that received no intervention at all. This is not to say that Twitter’s soft intervention did not work. It may be the case that the tweets would have spread more if not for the intervention. Twitter’s soft intervention label also could have backfired: the soft intervention may have drawn more attention to the tweet than it would have otherwise gotten without the intervention. This, however, is not knowable with the available data.
When we turn to possible exposure, we also find that accounts that retweeted messages that received no intervention had the highest number of average followers (Figure 2B). As a result, we would expect these tweets to experience greater overall engagement on the platform. However, as shown in Figure 2C, tweets that received a soft intervention had the highest average engagement (number of likes or “favorites”), suggesting that these tweets were more salient among the users who saw them. Tweets receiving the hard intervention had fewer average followers per retweet and fewer engagements than tweets receiving the soft intervention or no intervention. By all accounts, the hard intervention on Twitter was effective at slowing spread, exposure, and engagement with the tweets containing misinformation or incitement to violence.
Our results echo the findings from Zannettou (2021), which also found that tweets with warning labels experienced more engagement than tweets without warning labels. Our research is complementary in two ways. First, we include analysis of hard interventions. Second, we add a temporal dynamic, showing how engagement with labeled and unlabeled tweets diverged over the 24-hour period post publication.
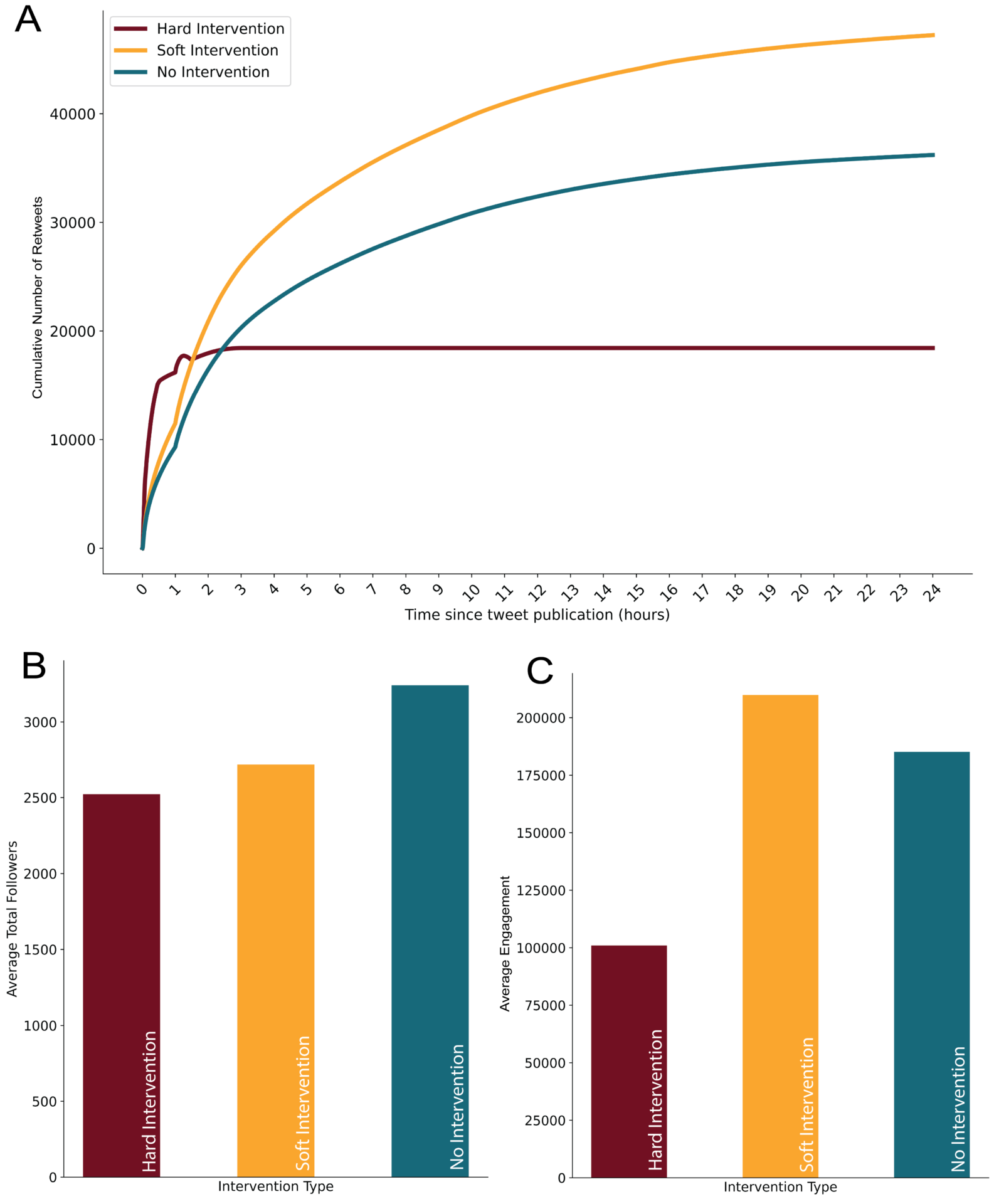
Finding 2: Messages that received hard interventions on Twitter spread longer and further on Facebook, Instagram, and Reddit than messages that received either soft or no interventions on Twitter.
A key contribution of our study is measuring the differential spread of these messages on three other popular social media platforms — Facebook, Instagram, and Reddit. These data provide evidence of how messages moderated on one platform spread on other platforms that employ different moderation policies and practices. To this end, we identified public posts that contained the same message as the tweets in our sample. For Reddit, we only queried the texts of posts; for Facebook and Instagram, we queried both text and images within posts.
In Figure 3 we show the average trajectory of Facebook posts containing Trump’s tweets on public pages grouped by intervention type (Figure 3A), the average visibility of the posts grouped by intervention type (Figure 3B), and the average engagement for the posts grouped by intervention type (Figure 3C). We find that messages that received either a soft or no intervention on Twitter had a similar average number of posts on public Facebook pages and groups. However, messages that received a hard intervention on Twitter had a higher average number of posts, were posted to pages with a higher average number of page subscribers, and received a higher average total number of engagements.
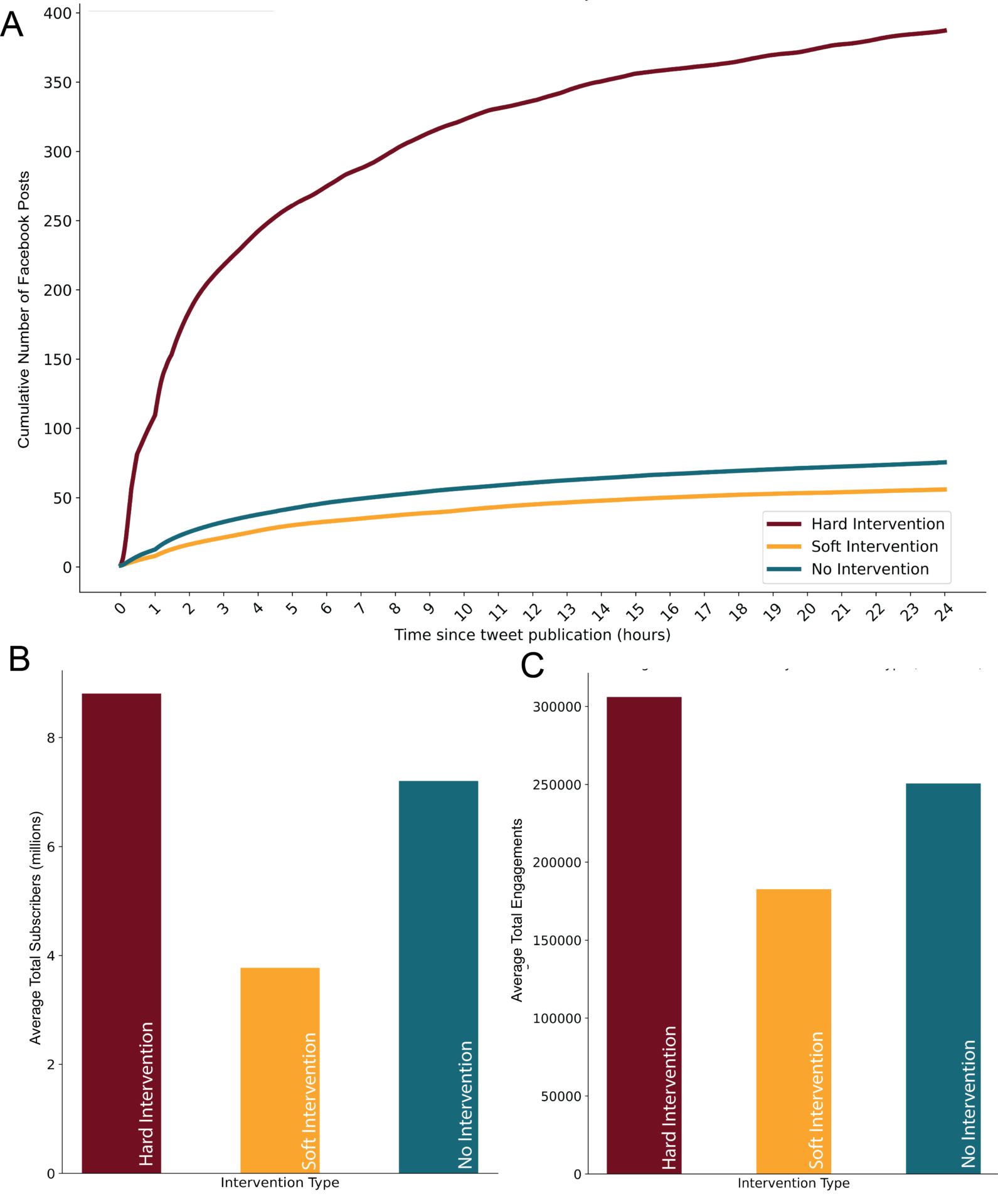
In Figure 4 we show the average trajectory of Instagram posts containing Trump’s tweets on public pages grouped by intervention type (Figure 4A), as well as the average engagement for the posts grouped by intervention type (Figure 4B). We do not report average total subscribers because CrowdTangle does not return subscriber data for Instagram. We find that while messages that received either a soft or no intervention on Twitter had a similar average number of posts on public Instagram pages, messages that received a hard intervention on Twitter had a higher average number of posts. However, on Instagram, posts with a hard intervention received the fewest engagements, while posts with no interventions received the most engagements. The significant difference in engagement by post category may be the result of platform affordances, audiences, or content moderation actions. While our data do not enable us to adjudicate between these explanations, these divergent engagement patterns between Facebook and Instagram introduce compelling new areas for future study, especially since the two platforms are governed by similar community standards and enforcement policies (Facebook Transparency Center, 2020).
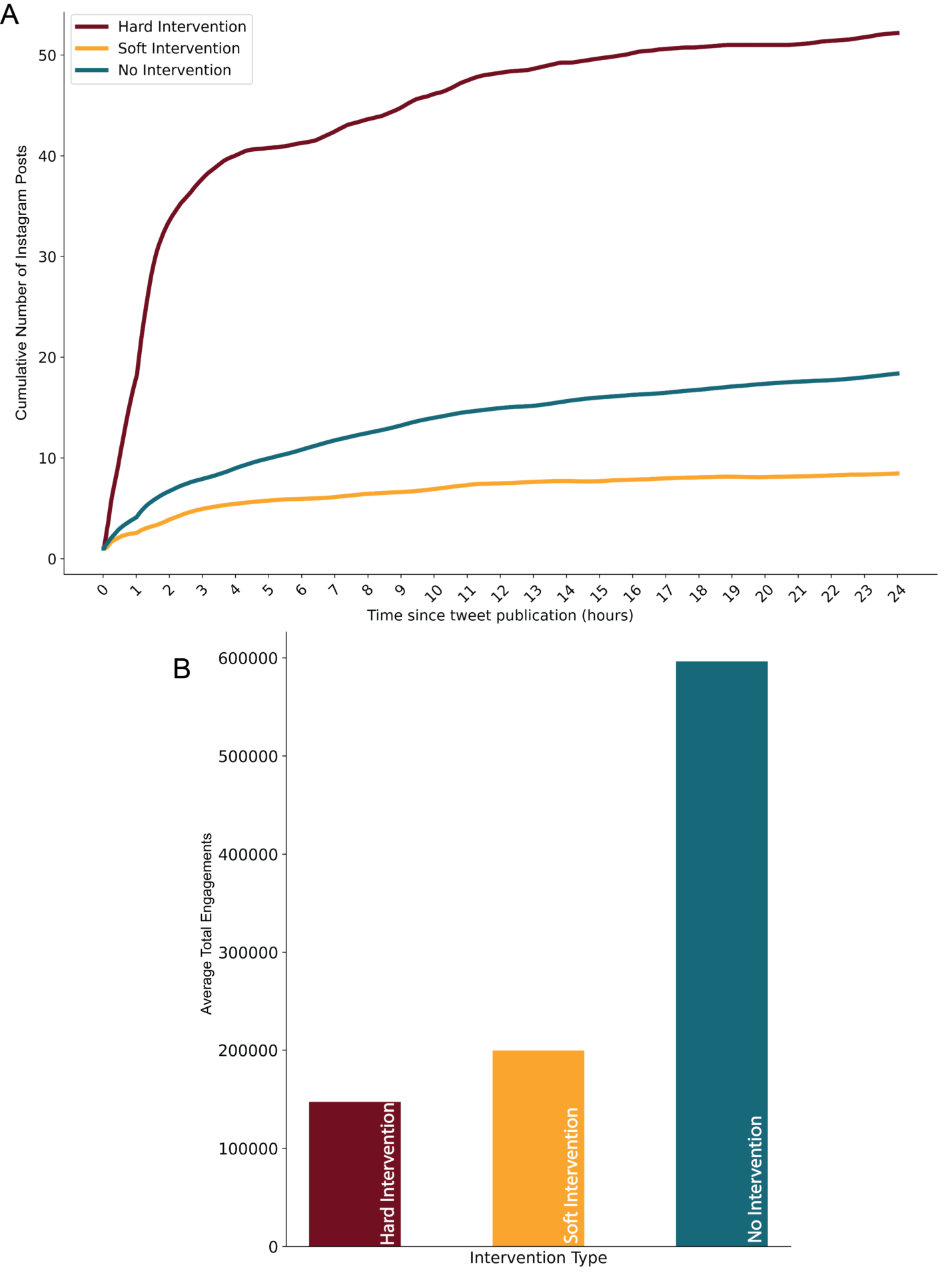
In Figure 5, we report similar statistics for Reddit: the trajectory of Reddit posts containing Trump’s tweets grouped by intervention type (Figure 5A) and the average visibility per message grouped by the type of intervention (Figure 5B). We do not report engagement since the Reddit platform does not report engagement in the form of raw counts. Reddit followed a similar pattern to Facebook and Instagram. On average, messages that received a hard intervention on Twitter were posted more frequently and on pages with over five times as many followers as pages in which the other two message types were posted.
The interconnected nature of these platforms and the online social media environment presents challenges for content moderation, where the policies are chosen and enforced by individual platforms without coordination with other platforms. While Twitter’s hard intervention—reserved for the most egregious policy violations—stamped out the spread of the content on the platform, links to and copies of the same posts and messages continually spread to millions of other users on Facebook, Instagram, and Reddit. This finding may be driven by messages containing misinformation being more viral based on their content; alternatively, people may have used other platforms as a substitute to spread messages that were blocked on Twitter or may have protested Twitter’s intervention by posting the same messages elsewhere. While our descriptive data do not enable us to adjudicate among these interpretations, our findings provide new evidence that one platform’s content moderation policies do not necessarily mitigate the spread of misinformation in a networked media ecosystem.
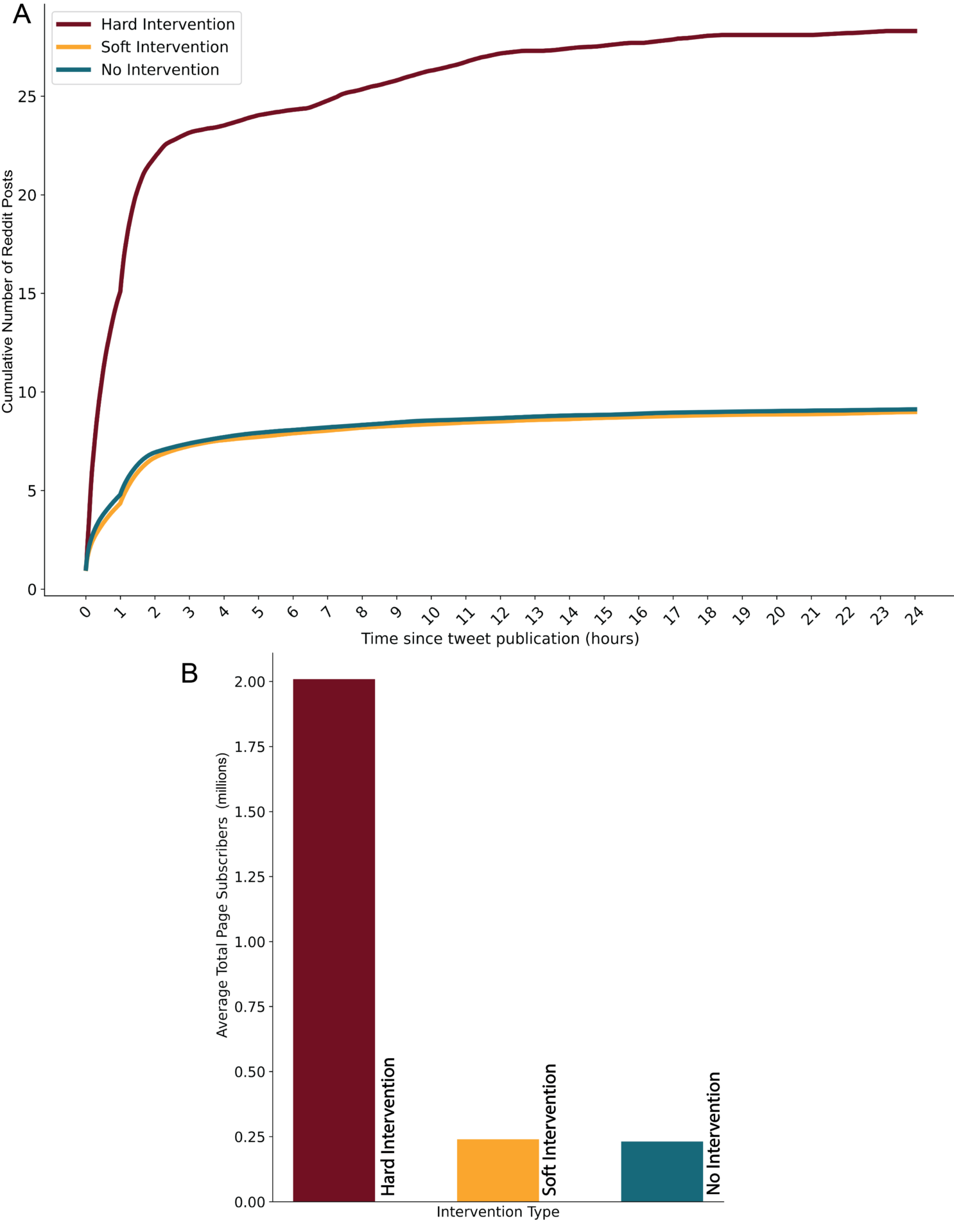
Methods
We investigate how Twitter’s public interest exception interventions affected the overall spread of the tweets on Twitter itself and on Facebook, Instagram, and Reddit. We aim to understand (1) how the tweets spread differently across intervention types, (2) exposure and engagement by intervention type, and (3) how 1 and 2 varied across platforms. Every day, we collected a 10% sample of all tweets on Twitter via the Twitter Decahose, including retweets. For this research, we are specifically interested in tweets by Donald Trump, which fell under Twitter’s public interest exception policy while Trump was still in office. For each day of tweets from November 1, 2020 through January 8, 2021, we filtered for retweets of tweets originally authored by Trump. For each retweet of a tweet by the accounts in question, we retained the timestamp of the retweet, the engagement metrics (number of likes or “favorites”, retweets, quote tweets, and replies) for the original tweet at the time of retweet, and the original tweet ID. For the Decahose, original tweets have a 10% chance of being in the sample, but so too do retweets. Due to the popularity of Trump’s account, his tweets were retweeted enough times to ensure that we collected all of Trump’s tweets in the 10% sample via retweets.1If 10,000 people retweet a tweet, there is an infinitesimally small chance that one of those retweets will not be contained in a 10% sample of all tweets. We also confirmed that we had full coverage of Trump tweets by checking our data against thetrumparchive.com.
Our main research focus was differential diffusion of tweets based on whether the message contained election-related misinformation or not. We limit ourselves to Trump’s tweets about politics because those are the tweets that would be eligible for an intervention. We define political tweets as content related to elections, governance, political parties, political candidates, policy positions, international relations, government officials, or social movements. Two research assistants coded Trump’s tweets as political or not. If there was a tie, one of the co-authors made the final determination. In total, we had 1,149 tweets rated as political in our sample and excluded the 157 tweets rated as not political. We examined the tweets 48 hours after their initial publication to see if they received a hard intervention, soft intervention, or no intervention, ensuring that there was time for Twitter to apply the intervention before we categorized it in our analysis. We considered a tweet to have “no intervention” if there was no indication that Twitter took steps to limit the spread of the tweet or added additional context to the information in the tweet. In total, 303 of Trump’s tweets about politics received a soft intervention and 16 received a hard intervention. Throughout this period, his account also posted 830 tweets about politics that did not receive an intervention.
We defined a soft intervention as a tweet to which Twitter gave a context label. These labels appeared in conjunction with the tweet and provided a link to more information about the disputed topic. These tweets remained fully visible, but additional friction was added before a user could retweet it, asking them to provide a quote with additional context before sharing the tweet.
We defined a hard intervention as a tweet that received Twitter’s more severe form of intervention. This intervention included being blocked from the timeline, meaning that a user must click on the Tweet to see its contents. Additionally, Twitter prevented users from retweeting, favoriting, or replying to the tweet. Users could only amplify the tweet by quote tweeting. We also considered tweets that Trump was required to remove before being able to tweet again “hard interventions.” Of the original tweets, 303 received the soft intervention, and 16 received the hard intervention.
For each retweet in our dataset, we calculated the amount of time that transpired between the publication of the original tweet and the retweeting of that tweet, in minutes. Then, for each tweet group (no intervention, soft intervention, and hard intervention), we calculated the average number of retweets for any given minute after the tweet was posted using the retweet_count field of the tweet, which reflects the number of retweets the original tweet had received at the time that we collect the retweet. Given the large number of retweets on Trump’s tweets, and because we get the actual retweet count at the time the retweet was posted, we believe these data are sufficient to measure retweet trajectory.
To collect data from Reddit, we used the Pushshift API (Baumgartner et al., 2020). The Pushshift API contains all posts from Reddit. For each tweet by Donald Trump, we queried for either a link to the tweet using its tweet ID, or we queried for the text of the tweet. Then, for each Reddit post containing the tweet, we calculated the time difference between the post of the tweet and the Reddit post containing the tweet and cumulatively counted the number of posts on Reddit for each minute after the original tweet, up until 24 hours after the tweet was posted. We then averaged the number of shares on Reddit at each minute for each intervention type and plotted it. We averaged the number of page subscribers for the subreddits in which the tweets were shared by intervention type.
To collect data from Facebook and Instagram, we used the CrowdTangle API, a public insights tool owned and operated by Facebook (CrowdTangle, 2021). A notable limitation in this data is that it only shows shares for public pages and groups that are indexed in CrowdTangle (typically pages and groups with more than 50k followers, or public pages or groups that have been manually added). However, we were more interested in how the spread varied by intervention rather than overall, so we believed this sample of larger accounts and public pages to be sufficient to estimate the difference in spread within this tightly defined author-topic focus. For each tweet by Donald Trump, we queried for either a link to the tweet using its tweet ID, or we queried for the text of the tweet. Note that on the CrowdTangle API, image text was included as a searchable field, so we were also able to identify screenshots of the tweets in question. Then, for each Facebook or Instagram post containing the tweet, we calculated the time difference between the post of the tweet and the Facebook or Instagram post containing the tweet and cumulatively counted the number of posts on Facebook or Instagram for each minute after the original tweet, up until 24 hours after the tweet was posted. We then averaged the number of shares on Facebook or Instagram at each minute for each intervention type and plotted it. We summed the number of total interactions (including likes, hahas, wows, etc.) and averaged the number of interactions by intervention type. We summed the total number of page followers for each tweet share on Facebook or Instagram and averaged them by intervention type.
Finally, we used hand coding to validate whether a post on Facebook, Instagram, and Reddit was directly referencing the relevant Trump tweet. From a random sample of posts, we found that 100% of the posts from Instagram and Facebook were directly referencing Trump’s tweets, as well as 76% of the posts from Reddit. See S.2 in the Supplemental Appendix for more information on validation.
Topics
Bibliography
Aslett, K., Guess, A., Nagler, J., Bonneau, R., & Tucker, J. (2021). News credibility labels have limited but uneven effects on news diet quality and fail to reduce misperceptions [Manuscript submitted for publication].
Banas, J. A., & Miller, G. (2013). Inducing resistance to conspiracy theory propaganda: Testing inoculation and metainoculation strategies. Human Communication Research, 39(2), 184–207. https://doi.org/10.1111/hcre.12000
Baumgartner, J., Zannettou, S., Keegan, B., Squire, M., & Blackburn, J. (2020). The pushshift Reddit dataset. Proceedings of the International AAAI Conference on Web and Social Media, 14(1), 830–839. https://ojs.aaai.org/index.php/ICWSM/article/view/7347
Benkler, Y., Tilton, C., Etling, B., Roberts, H., Clark, J., Faris, R., Kaiser, J. & Schmitt, C. (2020). Mail-in voter fraud: Anatomy of a disinformation campaign (Berkman Center Research Publication No. 2020-6). Berkman Klein Center for Internet & Society at Harvard University. https://dx.doi.org/10.2139/ssrn.3703701
Buntain, C., Bonneau, R., Nagler, J., & Tucker, J. (2021). YouTube recommendations and effects on sharing across online social platforms. Proceedings of the ACM Human-Computer Interaction, 5(CSCW1), 1–26. https://doi.org/10.1145/3449085
Center for an Informed Public, Digital Forensic Research Lab, Graphika, & Stanford Internet Observatory (2021). The long fuse: Misinformation and the 2020 election. Stanford Digital Repository: Election Integrity Partnership. https://purl.stanford.edu/tr171zs0069
Clayton, K., Blair, S., Busam, J. A., Forstner, S. Glance, J., Green, G., Kawata, A., Kovvuri, A., Martin, J., Morgan, E., Sandhu, M., Sang, R., Scholz-Bright, R., Welch, A. T., Wolff, A. G., Zhou, A. & Nyhan, B. (2019). Real solutions for fake news? Measuring the effectiveness of general warnings and fact-check tags in reducing belief in false stories on social media. Political Behavior, 42(4), 1073–1095. https://doi.org/10.1007/s11109-019-09533-0
Conger, K., Isaac, M., & Wakabayashi, D. (2020, November 4). Twitter and Facebook worked to crack down on election disinformation, but challenges loom. The New York Times. https://www.nytimes.com/2020/11/04/us/politics/twitter-and-facebook-worked-to-crack-down-on-election-disinformation-but-challenges-loom.html
CrowdTangle (2021). CrowdTangle. Facebook, Menlo Park, California, United States. https://help.crowdtangle.com/en/articles/4558716-understanding-and-citing-crowdtangle-data
DiResta, R. (2021, March 15). The misinformation campaign was distinctly one-sided. The Atlantic. www.theatlantic.com/ideas/archive/2021/03/right-wing-propagandists-were-doing-something-unique/618267/
Douek, E. (2020, November 17). Why isn’t Susan Wojcicki getting grilled by Congress? Wired. https://www.wired.com/story/why-isnt-susan-wojcicki-getting-grilled-by-congress/
Facebook Transparency Center. (2020, May). Community standards enforcement report.https://transparency.fb.com/data/community-standards-enforcement/
Freeze, M., Baumgartner, M., Bruno, P., Gunderson, J. R., Olin, J., Ross, M. Q., & Szafran, J. (2020). Fake claims of fake news: Political misinformation, warnings, and the tainted truth effect. Political Behavior, 1–33. https://doi.org/10.1007/s11109-020-09597-3
Fukuyama, F., Richman, B., Goel, A., Katz, R., Melamed, D., & Schaake, M. (2020). Report of the working group on platform scale. [White paper]. Stanford Cyber Policy Center. https://cyber.fsi.stanford.edu/publication/report-working-group-platform-scale
Gadde, V., & Beykpour, K. (2020, November 12). An update on our work around the 2020 US elections. Twitter. blog.twitter.com/en_us/topics/company/2020/2020-election-update.html
Grinberg, N., Joseph, K., Friedland, L., Swire-Thompson, B., & Lazer, D. (2019). Fake news on Twitter during the 2016 U.S. presidential election. Science, 363(6425), 374–378. https://doi.org/10.1126/science.aau2706
Guess, A., Lerner, M., Lyons, B., Montgomery, J., Nyhan, B., Reifler, J., & Sircar, N. (2020). A digital media literacy intervention increases discernment between mainstream and false news in the United States and India. Proceedings of the National Academy of Sciences, 117(27), 15536–15545. https://doi.org/10.1073/pnas.1920498117
Hatmaker, T. (2021, February 11). Facebook oversight board says other social networks ‘welcome to join’ if project succeeds. TechCrunch. https://techcrunch.com/2021/02/11/facebook-oversight-board-other-social-networks-beyond-facebook/
Helberger, N. (2019). On the democratic role of news recommenders. Digital Journalism, 7(8), 993–1012. https://doi.org/10.1080/21670811.2019.1623700
Nyhan, B. (2021). Why the backfire effect does not explain the durability of political misperceptions. Proceedings of the National Academy of Sciences, 118(15), e1912440117. https://doi.org/10.1073/pnas.1912440117
Ribeiro, M., Jhaver, S., Zannettou, S., Blackburn, J., De Cristofaro, E., Stringhini, G., & West, R. (2020). Does platform migration compromise content moderation? Evidence from r/The_Donald and r/Incels. ArXiv. https://arxiv.org/abs/2101.07183
Pennycook, G., Bear, A., Collins, E. T., & Rand, D. G. (2020). The implied truth effect: Attaching warnings to a subset of fake news headlines increases perceived accuracy of headlines without warnings. Management Science, 66(11), 4944–4957. https://doi.org/10.1287/mnsc.2019.3478
Persily, N., & Tucker, J. (2020). Conclusion: The challenges and opportunities for social media research. In N. Persily & J. Tucker (Eds.), Social media and democracy: The state of the field, prospects for reform (pp. 313–331). Cambridge University Press. https://doi.org/10.1017/9781108890960
Scott, M., & Overly, S. (2020, August 4). Silicon Valley is losing the battle against election misinformation. Politico. https://www.politico.com/news/2020/08/04/silicon-valley-election-misinformation-383092
Stroud, T., Tucker, J., Franco, A., & Kiewiet de Jonge, C. (2020, August 31). A proposal for understanding social media’s impact on elections: Rigorous, peer-reviewed scientific research. Medium. https://medium.com/@2020_election_research_project/a-proposal-for-understanding-social-medias-impact-on-elections-4ca5b7aae10
Tucker, J., Guess, A., Barbera, P., Vaccari, C., Siegel, A., Sanovich, S., Stukal, D., & Nyhan, B. (2018). Social media, political polarization, and political disinformation: A review of the scientific literature. William + Flora Hewlett Foundation. https://dx.doi.org/10.2139/ssrn.3144139
Wardle, C. (2021, February 12). Broadcast news’ role in amplifying Trump’s tweets about election integrity. First Draft. https://firstdraftnews.org/long-form-article/cable-news-trumps-tweets/
Zannettou, S. (2021). “I won the election!”: An empirical analysis of soft moderation interventions on Twitter. ArXiv. https://arxiv.org/abs/2101.07183
Funding
The NYU Center for Social Media and Politics is generously supported by funding from the Knight Foundation, the Charles Koch Foundation, the Hewlett Foundation, Craig Newmark Philanthropies, the Siegel Family Endowment, and NYU’s Office of the Provost.
Competing Interests
Joshua A. Tucker received a small fee from Facebook to compensate him for administrative time spent in organizing a 1-day conference for approximately 30 academic researchers and a dozen Facebook product managers and data scientists that was held at NYU in the summer of 2017 to discuss research related to civic engagement; the fee was paid before any data collection for the current project began. He did not provide any consulting services nor any advice to Facebook as part of this arrangement.
Ethics
Data collection of publicly available social media data has been ruled exempt from IRB oversight (NYU IRB #12-9058). As the unit of analysis for this study is public aggregated exposure to and engagement with a public figure’s tweets, we did not seek additional institutional review board approval for this research project.
Copyright
This is an open access article distributed under the terms of the Creative Commons Attribution License, which permits unrestricted use, distribution, and reproduction in any medium, provided that the original author and source are properly credited.
Data Availability
All materials needed to replicate this study are available via the Harvard Dataverse: https://doi.org/10.7910/DVN/DDJNEF