Peer Reviewed
The spread of synthetic media on X
Article Metrics
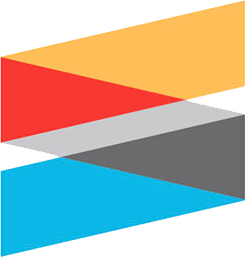
0
CrossRef Citations
Altmetric Score
PDF Downloads
Page Views
Generative artificial intelligence (AI) models have introduced new complexities and risks to information environments, as synthetic media may facilitate the spread of misinformation and erode public trust. This study examines the prevalence and characteristics of synthetic media on social media platform X from December 2022 to September 2023. Leveraging crowdsourced annotations identifying synthetic content, our analysis reveals an increase in AI-generated media over time, with an initial spike in March 2023, following the release of Midjourney V5. While most synthetic media identified is non-political and non-malicious, concerning deepfakes targeting political figures persist, raising questions on the potential for misuse of AI technologies.

Research Questions
- What is the prevalence of AI-generated media in tweets obtained from X’s Community Notes programme, as observed from the date of its global rollout in December 2022 to September 2023?
- What is the media-type distribution in the identified AI-generated content? Specifically, what percentage of this content is video-based as opposed to image-based?
- What fraction of the AI-generated content under analysis can be categorised as political?
- How has X’s paid verification feature, known for enabling the purchase of a “blue tick,” influenced the dissemination of AI-generated media content on the platform?
Essay Summary
- The goal of this study is to assess the extent to which synthetic media may pose threats to information ecosystems. For this purpose, we analysed the prevalence and key characteristics of AI-generated synthetic media content on the social media platform X from December 2022 through September 2023.
- Leveraging crowdsourced annotations from X’s Community Notes programme, we identified 556 unique tweets containing synthetic images or videos. These tweets were viewed over 1.5 billion times in the period under analysis.
- The prevalence of synthetic tweets rose over time, peaking in March 2023 following the release of Midjourney V5. After a subsequent decline, the rate stabilised at around 0.2% of all community notes.
- The majority of synthetic tweets contained non-political, harmless content. More often, this was image rather than video content. While less likely to go viral, synthetic videos were more often political propaganda or concerning deepfakes.
- While less likely to go viral, synthetic videos were more often political propaganda or concerning deepfakes.
- Over half of synthetic tweet posters had verified status. Normalising for followers, verification corresponds to only a minor visibility boost over non-verified users.
- The findings reveal an increase in the prevalence of synthetic media, though current usage leans toward non-deceptive ends. Still, the potential for political weaponisation warrants ongoing monitoring, especially as generative AI continues advancing.
Implications
In a time of rapid advancement of AI technologies, we are witnessing an increased prevalence of sophisticated generative AI models that can produce synthetic media indistinguishable from human content. This transformation in the dynamics of content generation, propelled by widely accessible models such as Stable Diffusion and Midjourney (Borji, 2022), could disrupt conventional processes of knowledge acquisition (Weikmann & Lecheler, 2022), introducing new complexities and challenges to the integrity of information environments. While the risks associated with the spread of synthetic media—broadly defined as artificially generated or manipulated photos, audios, and videos (Whittaker et al., 2020) —have been previously acknowledged in the academic literature as potential but largely unrealised dangers (Brundage et al., 2018; Kalpokas, 2021; Littman et al., 2022), recent breakthroughs in generative AI threaten to make these risks more immediate. In particular, the growing capabilities and availability of cutting-edge AI models capable of creating synthetic media may further accelerate this transition from a hypothetical threat to a tangible concern with broad societal implications (Epstein et al., 2023; Huang & Siddarth, 2023).
The potential proliferation of synthetic-media-driven false information within peer-to-peer social media platforms with low barriers to publication could pose a significant challenge to the safety of public epistemic processes, as users can find themselves increasingly exposed to media that is both misleading and highly realistic (Kerner and Risse, 2021). This pattern was evidenced several times in recent months. For example, in March 2023, an AI-generated image of Pope Francis garbed in a puffer jacket went viral on social media, fooling thousands of users, and igniting a discussion on the credibility of this image (Tolentino, 2023). Similarly, in October 2023, an AI-generated audio portraying UK Labour Party leader Kier Starmer being abusive towards his staff emerged and was viewed 1.5 million times, raising doubts on the resilience of political systems to the rise of synthetic media (Bristow, 2023; “Deepfake audio of Sir Keir Starmer released on first day of Labour conference,” 2023). Ultimately, growing exposure to realistic synthetic media can be hypothesised to have at least two types of impacts on the public. On the one hand, this phenomenon may cause an increase in levels of public deception (Zagni & Canetta, 2023), where users are led to suboptimal decision-making rooted in the acquisition of false information. On the other hand, this development may lead to a decrease in public trust in the reliability of visual information (Europol, 2024; Fallis, 2021; Manohar, 2020), where individuals may be led to adopt a sceptical stance towards visual media. This type of shift in public epistemics may be easily weaponised to manipulate public opinion, particularly in a scenario such as the current one where highly effective approaches to detect AI-generated content are lacking (Baraheem & Nguyen, 2023; Leibowicz et al., 2021; Sabel & Stiff, 2023).
Given these dual risks of increased public deception and a concurrent erosion of trust in media content, it is increasingly important to closely monitor and understand the dynamics of synthetic media proliferation. To this end, our analysis reveals how synthetic media became markedly more prevalent on X in the period between December 2022 and October 2023, with our limited data sample obtaining over 1.5 billion views in the period under analysis, with a clear spike in March 2023 following the release of Midjourney V5, a model that has been the source of many popular pieces of synthetic media. However, our results also indicate that the majority of the identified synthetic content (77%) remains largely non-political. While we do not use any explicit measures of harm, we also see that the majority of these media appear harmless in nature, mostly comprising humorous or satirical images. This indicates that, at an aggregate level, synthetic media’s principal goal is not malicious deception. However, the landscape of synthetic media is not uniformly benign, as we also identify a limited, yet concerning, quantity of malicious synthetic media, particularly in the form of deepfake videos and audio clips portraying high-profile political figures. Though these instances are less frequent (14% of our data), their potential impact is significant, particularly as these often emerge as believable tools of political propaganda. This aspect warrants a greater focus in future research and monitoring efforts. Furthermore, our study also addresses the implications of recent changes to X’s verification system, which now permits users to purchase verification badges. This development has been a subject of debate, with concerns raised about its potential to enable spreaders of misinformation to enhance their influence and visibility (Biddlestone et al., 2023). Our analysis delivered mixed results, where verified users generally receive more views than their non-verified counterparts, but a closer examination based on normalised view counts presents a different scenario, where the actual amplification effect attributable to verification status appears to be more limited than initially presumed.
Ultimately, our findings underscore the growing presence of synthetic media on X, which, given the current observed trajectory in the popularity of AI models, is likely to represent a broader societal trend and to replicate in similar social media settings. While the current usage predominantly leans towards non-deceptive ends, the significance of this shift in prevalence of synthetic media should not be underestimated. The increasing exposure to synthetic media, even in its less harmful forms, poses a clear risk of eroding public trust in media-based information, a trend which could have far-reaching implications, potentially leading to a wider breakdown in the credibility of online content.
Based on these findings, several policy responses are recommended. First, it is imperative for researchers to continuously monitor and critically evaluate the evolving landscape of synthetic media, especially in light of its added potential to influence public perception and discourse. Second, it is increasingly crucial for the research community to work across the academic and industry divide in developing open, reliable, and transparent methods to identify AI-generated media, providing the public with the necessary tools to grapple with the emergence of this new phenomenon. Lastly, policymakers should accelerate the formulation and adoption of policies that advance those goals, as well as proactively mitigate the social and political risks of malicious synthetic media, all while being thoughtful about some of the observations of this study. For example, the empirical data suggesting that a significant portion of AI-generated content on social media serves satirical purposes implies that outright bans on AI-generated content may be excessive (Fuentes, 2024; LA Times 2023; Stosz, 2019). By contrast, policy measures that mandate the disclosure of content origins, use machine-readable markers, or promote the deployment of detection technologies—without broadly suppressing content circulation—may offer a more balanced approach (Exec. Order No. 14110, 2023; Council of the European Union, 2024; Government of Canada, 2023). Furthermore, targeted restrictions could be considered for high-risk subject matters, such as those involving political manipulation (Stosz, 2019) or sexually explicit material (Defiance Act of 2024, 2024), and in further cases of malicious intent (Deepfakes Accountability Act, 2023). Lastly, the propensity of the latest AI models to evade detection (Jacobsen, 2024; Le et al., 2023; Lu et al., 2023; Lyu, 2020) points to the need for human discretion (like that provided by Community Notes) to assess the origins and risks of a piece of synthetic media. Policymakers should consider monitoring the evolving spread of synthetic media online by leveraging collective intelligence (Groh et al., 2021; Tursman, 2020). Examples of crowdsourcing initiatives include establishing user-feedback portals in addition to refutation mechanisms, which may be an especially critical addition to any policies that propose mandatory filtering or disclosure of AI-generated imagery online (Fox-Sowell, 2024; U.S. Representative Ritchie Torres, 2023). Finally, before outlining our findings in greater detail, it is important to clarify that we do not posit the existence of a linear relationship between the rise in quality and scale of diffusion of AI-generated media and a corresponding “misinformation nightmare” (Gold & Fisher, 2023), as we recognise that the epistemic threats connected to false information do not happen in a vacuum. Rather, several factors—such as the demand and supply for misinformation (Gravino et al., 2021), the level of public resilience to false content (Humprecht et al., 2023), as well as the behaviour of existing information diffusion infrastructures (Vosoughi et al., 2018)—influence the threat level posed by any rises in the prevalence of synthetic misinformation. Instead, in this work, we view the use of AI for the generation of synthetic content as a threat multiplier, where existing information threats—particularly within highly susceptible issue domains, such as international conflicts and political elections—may be significantly worsened by the growing availability of powerful media generation technologies, and we interpret our findings through this lens.
Findings
Finding 1: The prevalence of AI-generated media on X has increased significantly in the period under analysis, with the largest share of this increase seen in March 2023, shortly after the release of Midjourney V5.
The first objective of this study is to quantify changes in the prevalence of AI-generated media on X over the designated time frame. To achieve this, we scrutinise two specific temporal metrics: (1) the percentage of all tweets contained in the Community Notes data marked by crowdsourced judgements and human evaluation as containing AI-generated content and (2) the monthly views obtained by these tweets.
Data on the first temporal metric—the monthly percentage of tweets in our data marked as containing AI-generated media—is visualised in the top panel of Figure 1. Here, results show a sharp rise in the frequency of mentions of AI-generated media in March 2023 (+393% from February 2023), with a peak of over 0.6% of monthly tweets matching our query. After this initial surge, the temporal distribution shows a decline, to then stabilise, between June and September 2023, around a value of 0.2% of monthly tweets, suggesting a moderate yet sustained presence of mentions of AI-generated media within Community Notes. Turning our attention to the second temporal metric—the monthly views obtained by tweets containing AI-generated media, shown in the bottom panel of Figure 1—we observe a contrasting trend. Here, similarly to what was highlighted above, results also show a visible peak in March 2023, with over 300 million monthly views. However, we also observe—after a decline in April 2023—a gradual month-on-month increase of tweet views, indicating a growing aggregate visibility of tweets containing synthetic media. Further analysing the data, this peak is explained by a higher raw number of tweets containing synthetic media, despite a lower percentage of the total, which is the consequence of a general increase in the popularity and use of Community Notes.
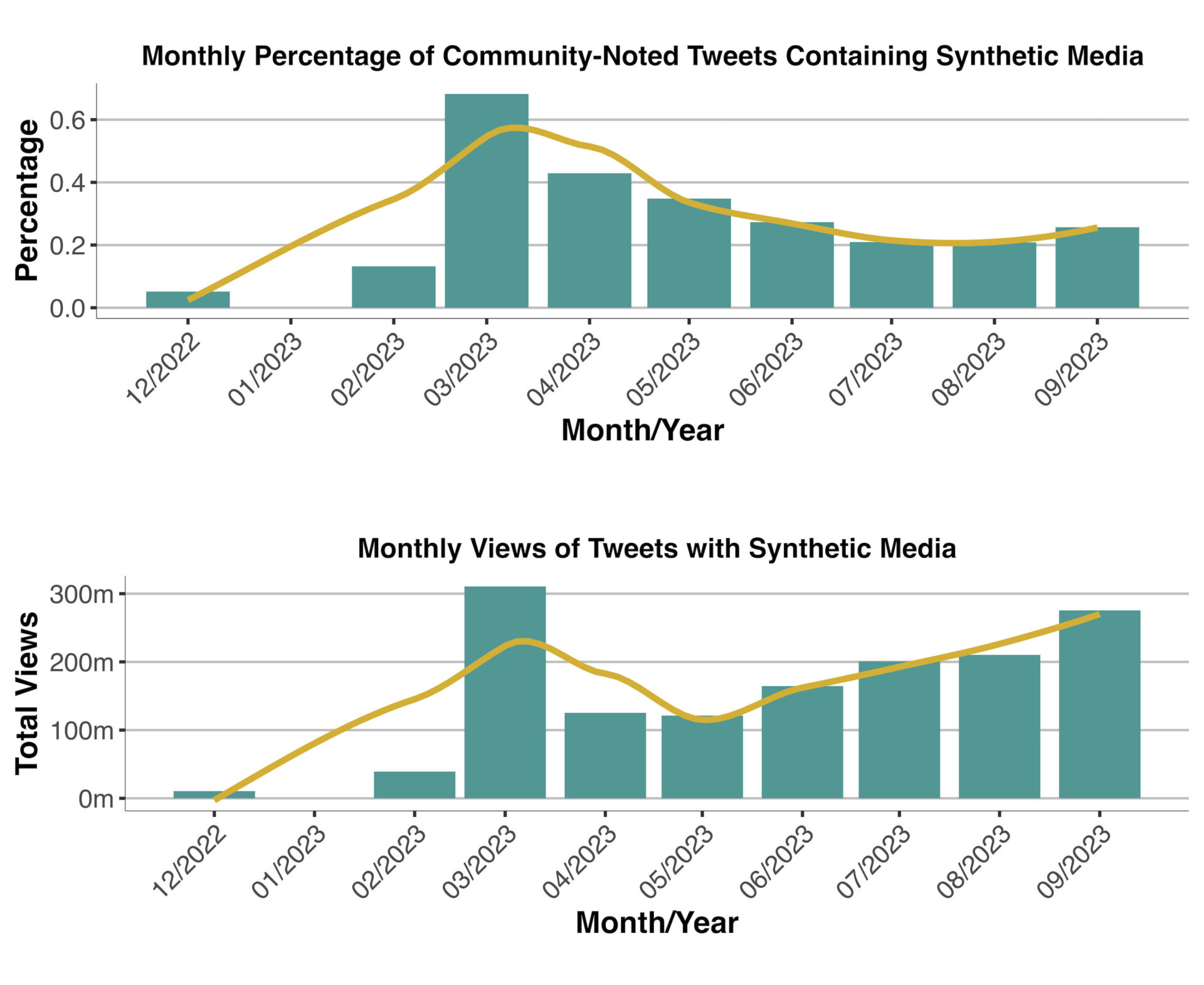
Furthermore, the synchronous peak observed in both metrics during March 2023 is worth additional investigation, as the alignment of these trends suggests an external event that influenced the sudden rise in prevalence of tweets containing AI-generated media. Qualitatively analysing the data, it appears that this initial surge can be largely attributed to the release of Midjourney V5 on March 15, 2023, which was used in the following week to produce several highly viral AI-generated images, including a widely circulated image of Pope Francis wearing a puffer jacket and false images of Donald Trump being arrested (see Appendix A). Figure 2 shows the weekly cumulative distribution function of the views of tweets containing AI-generated media, portraying the initial rise following the release of Midjourney V5, corresponding to the yellow dotted line.
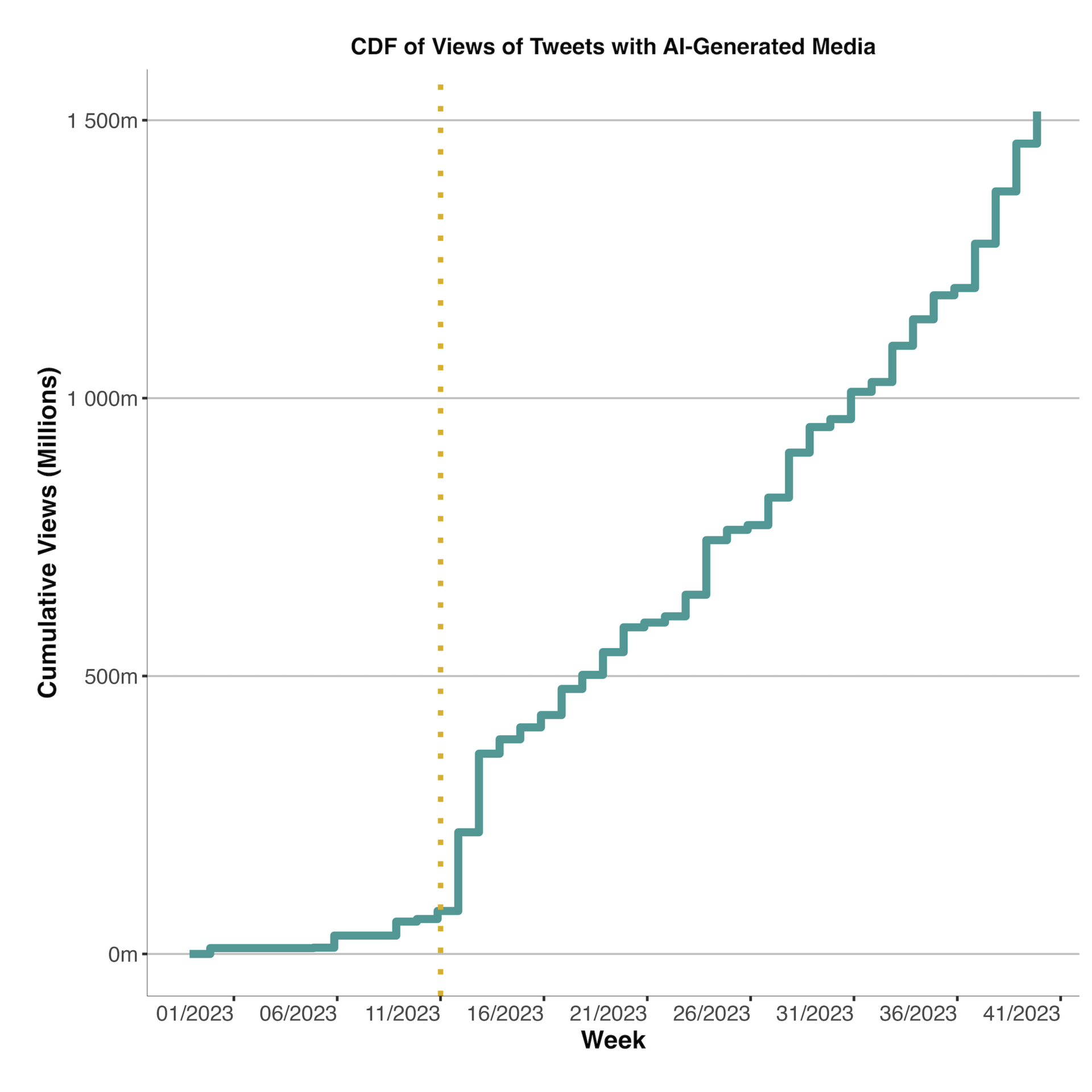
Finding 2: The majority of synthetic media in the data are non-political images, often of satirical and harmless nature. While non-political content and images have a higher probability of reaching virality, political AI-generated media and videos have higher median views.
Building upon our previous analysis, the second objective of the study is to assess the most common characteristics of AI-generated media, particularly in terms of the nature of content (political vs. non-political) and the media contained in the tweet (images vs. videos). Here, results reveal that the majority of tweets containing AI-generated media are non-political, with a ratio of 59.3% to 40.7%, and are largely images, with a ratio of 76.4% to 23.6%. Tweets with non-political images are often of harmless and satirical nature—for example, the most viewed images in the data include a Midjourney-generated image of Elon Musk with 77m views, the rendering of an imaginary Netflix-themed restaurant with 69m views, and an AI-generated image of cats containing a hidden message with 61.7m views. These images can be seen in Appendix A.
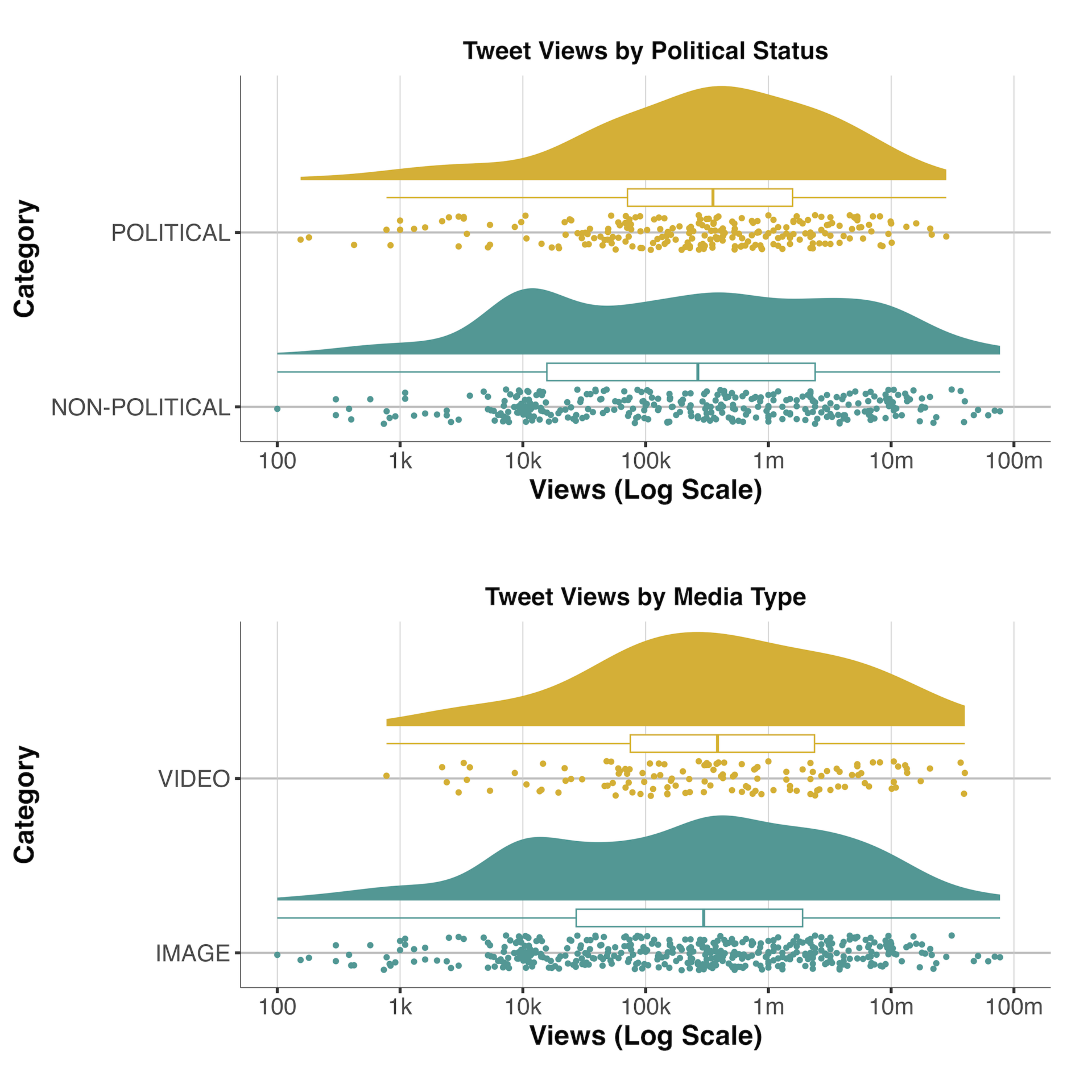
Results also show that while videos and media of a political nature have higher median views than their counterparts, non-political tweets and tweets with images are significantly more likely to achieve virality, which we define as a condition where a tweet obtains at least ten times the median impressions to followers ratio observed across the dataset. This approach to measuring variety is partially based on the idea of structural virality from Goel et al. (2015) and focuses on how far outside a poster’s network a tweet travelled. Here, we see that 64% of viral tweets are non-political and 77% contain images rather than videos. The most viral tweets in our data include several harmless images such a rendering of Super Mario character Princess Peach playing golf with 9.1m views (and a ratio of 5,687 times the dataset median), an image of Stitch from Lilo and Stitch, and an image of Manchester City supporters parading.
Finally, it is worth noting that while tweets containing AI-generated videos are less common than those containing images, the majority of videos (58.2%) were classified as political, indicating that video content is more likely to be weaponised for political purposes. These videos are often deepfakes used to smear or support high-profile-political figures. For example, the most viewed tweets in this category include a montage of Donald Trump firing the former Director of the U.S. National Institute of Allergy and Infectious Diseases, Anthony Fauci, a deepfake of Joe Biden announcing a national draft to deploy the U.S. Army in Ukraine and Taiwan, and a deepfake of a conversation between Joe Biden and U.S. Representatives Nancy Pelosi and Alexandria Ocasio-Cortez. These are also available in Appendix A.
Finding 3: The majority of users sharing AI-generated media have verified status on X and these users get significantly more views than users without a verified status. However, normalising tweet-views by follower counts, this difference is reduced significantly, suggesting that the verified status only has a marginal impact on visibility.
Finally, the last step of this research involves an analysis of the presence of verification ticks in the users sharing tweets containing synthetic media. This analysis provides valuable insights into the impact of X’s recent changes enabling users to purchase a verification tick, a key reputational signal on the platform. Here, results reveal that the majority (57%) of users sharing synthetic content possess a verification tick and that in terms of raw views, this majority of verified users obtain substantially more views than unverified users, as shown in the top panel of Figure 4. However, normalising views by follower count delivered significantly different results, with the gap in median views between verified and unverified users narrowing significantly (from +744% to +20%), as shown in the bottom panel of Figure 4. This finding is noteworthy, as it suggests that users with high follower counts may be more likely to purchase a verification tick and that the amplification warranted by the new blue tick may be contained, particularly considering the size of the difference in the raw data.
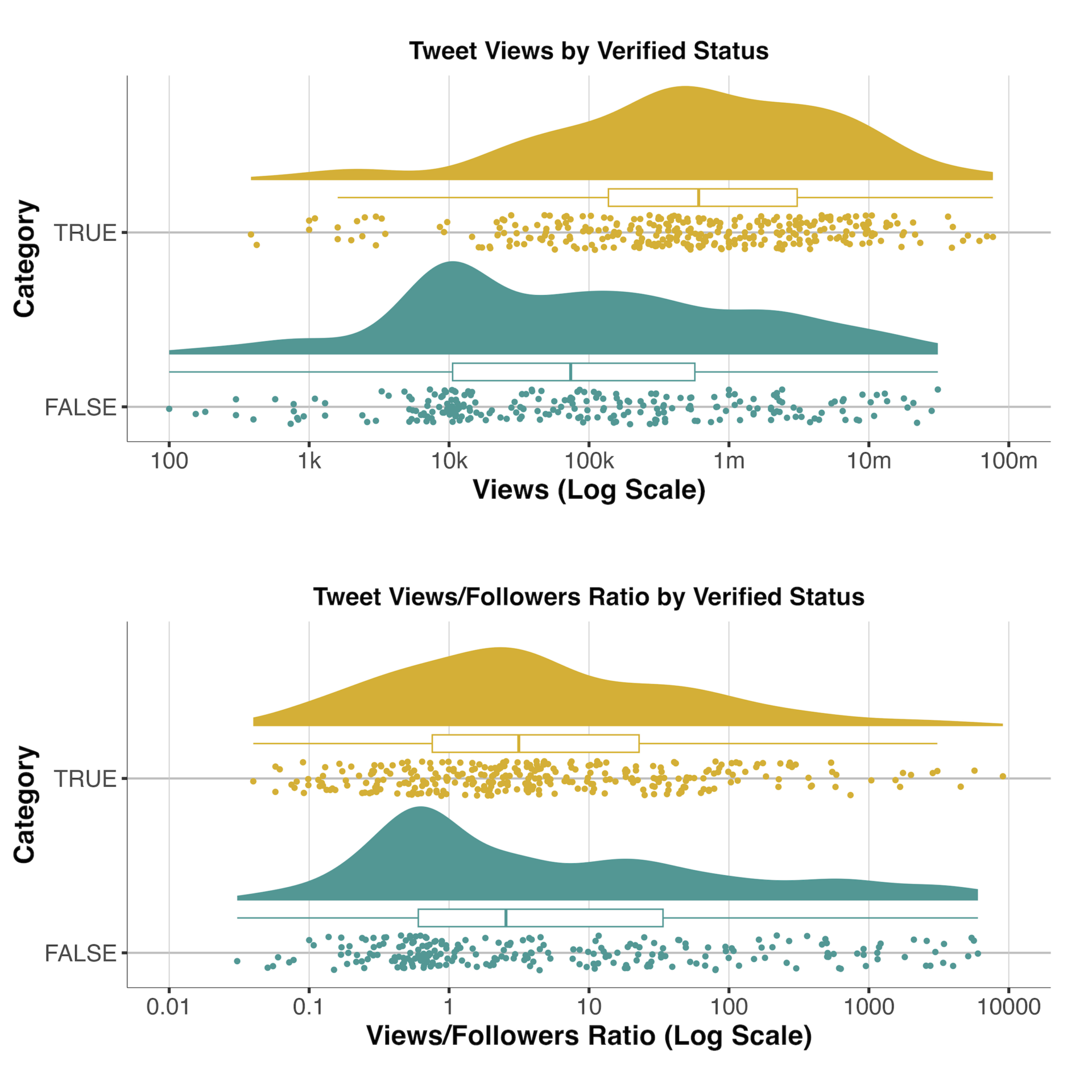
Methods
This study seeks to empirically measure and analyse any changes in the prevalence and key characteristics of synthetic content appearing on the social media platform X (formerly Twitter) since December 2022. This starting date was specifically chosen for this analysis not only because it marks the global rollout of the Community Notes programme, but also because it coincides with the initial stage of the proliferation of first-generation image models such as Stable Diffusion V1 and Midjourney V3, with more powerful models to be released shortly thereafter. Faced with the complex task of identifying tweets containing AI-generated media, an area where a clear methodological consensus is still lacking (Sabel & Stiff, 2023), we propose an empirical approach to identify and study synthetic media circulating on the social media platform X, which relies on the analysis of crowdsourced judgements written by the platform users through the Community Notes programme. This programme acts as both a de facto system of crowdsourced moderation and contextualisation and a system of information quality-signals (Allen et al., 2022; Pröllochs, 2022).
To answer our research questions, we collected data on Community Notes contributions made publicly available by X through regular data releases. The data provided by X is quite granular and contains information on several variables of interest, such as the text of each Community Note, their reference tweet, the note’s author, and the rating difficulty experienced by the author. After acquiring this dataset, the first step of our analysis involved applying a first layer of data selection through a keywords-based filtering process. For this purpose, we used a query aimed at identifying all Community Notes mentioning instances of synthetic content that could be potentially considered misleading, such as “deepfake,” “synthetic image,” and “AI-generated media.” Notably, our query deliberately excluded strings related to clearly marked AI-generated content, such as tweets containing the hashtag “#aiart,” as the focus was on capturing only cases where the audience may be misled due to the absence of clear labelling. The full query can be found in the code supporting this work.
After obtaining a dataset of Community Notes mentioning AI-generated media in different forms, we extracted the IDs of all reference tweets mentioned in our Community Notes, and we built an ad hoc crawler to extract data of interest. For this purpose, in Python, we leveraged Selenium’s headless browser capabilities to systematically navigate to each tweet and obtain a wide range of metrics including usernames, follower count, tweet impressions, retweets, likes, bookmarks, as well as the content of the tweet itself. This process returned 682 unique tweets posted on the platform since December 2022. This is indeed likely to only represent a small subset of all tweets containing synthetic content appearing on the platform in this timeframe, but in this case, in absence of valid approaches to detect synthetic content at scale within social media platforms, we give up gains in dataset size in favour of greater accuracy by using this approach reliant on the use of Community Notes data.
At this stage, the authors proceeded to manually annotate the data, validating whether the source tweets confirmed the Community Notes’ assessment of containing synthetic media and labelling whether a tweet’s poster was verified on the platform, what type of media a tweet contained, and whether a tweet could be classified as political or non-political. For this purpose, we developed an annotation codebook (see Appendix B). Through this process, we identified 9 tweets where notes mentioned AI-generated media but no such media were present, 89 removed tweets, 13 tweets with media that was non-synthetic, and 7 tweets with missing data, for a final dataset of 566 tweets containing synthetic media.
Finally, as the data validation and labelling process was shared among the three authors, we computed Krippendorff’s alpha on a sample of the data to determine raters’ coefficient of agreement. Krippendorff’s alpha is a statistical measure of inter-rater reliability used to evaluate the agreement between multiple coders on how they apply codes to content, and it is considered one of the most robust and flexible statistical measures of inter-rater reliability (Krippendorff, 2011). To compute Krippendorff’s alpha on our annotated tweet dataset, we first had all three coders independently code a random sample of 30 tweets, and we then calculated observed agreement and expected agreement between raters on each variable. In our sample, Krippendorff’s alphas were 0.79 for type of media, 0.89 for political content, and 0.80 for verified accounts. This indicates a high level of inter-rater reliability, giving us confidence to proceed with analysis on the fully coded dataset. We can conclude our coders had a common understanding of constructs and could reliably identify key characteristics of interest in the tweets.
Topics
Bibliography
Allen, J., Martel, C., & Rand, D. G. (2022). Birds of a feather don’t fact-check each other: Partisanship and the evaluation of news in Twitter’s Birdwatch crowdsourced fact-checking program. In CHI ’22: Proceedings of the 2022 conference on human factors in computing systems (pp. 1–19). Association for Computing Machinery. https://doi.org/10.1145/3491102.3502040
Baraheem, S. S., & Nguyên, T. (2023). AI vs. AI: Can AI detect AI-generated images? Journal of Imaging, 9(10), 199. https://doi.org/10.3390/jimaging9100199
Borji, A. (2022). Generated faces in the wild: Quantitative comparison of Stable Diffusion, Midjourney and DALL-E 2. arXiv. https://doi.org/10.48550/arXiv.2210.00586
Bristow, T. (2024, October 9). Keir Starmer suffers UK politics’ first deepfake moment. It won’t be the last. Politico. https://www.politico.eu/article/uk-keir-starmer-labour-party-deepfake-ai-politics-elections/
Brundage, M., Avin, S., Clark, J., Toner, H., Eckersley, P., Garfinkel, B., Dafoe, A., Scharre, P., Zeitzoff, T., Filar, B., Anderson, H., Roff, H., Allen, G. C., Steinhardt, J., Flynn, C., Ó hÉigeartaigh, S., Beard, S., Belfield, H., Farquhar, S., & Lyle, C. (2018). The malicious use of artificial intelligence: Forecasting, prevention, and mitigation. arXiv. https://doi.org/10.48550/arXiv.1802.07228
Deepfakes Accountability Act, H.R. 5586. (2023). https://www.congress.gov/bill/118th-congress/house-bill/5586/text
Defiance Act of 2024, S.3696. (2024). https://www.congress.gov/bill/118th-congress/senate-bill/3696
Dunlap, R. E., & Brulle, R. J. (2020). Sources and amplifiers of climate change denial. In D. C. Holmes & L. M. Richardson (Eds.), Research handbook on communicating climate change (pp. 49–61). Edward Elgar Publishing. https://doi.org/10.4337/9781789900408.00013
Epstein, Z., Hertzmann, A., Memo Akten, Farid, H., Fjeld, J., Frank, M. R., Groh, M., Herman, L., Leach, N., Mahari, R., Pentland, A. S., Russakovsky, O., Schroeder, H., & Smith, A. (2023). Art and the science of generative AI. Science, 380(6650), 1110–1111. https://doi.org/10.1126/science.adh4451
Europol. (2024). Facing reality? Law enforcement and the challenge of deepfakes. An observatory report from the Europol Innovation Lab. Publications Office of the European Union. https://doi.org/10.2813/158794
Exec. Order No. 14110, DCPD-202300949 (2023) https://www.govinfo.gov/app/details/DCPD-202300949
Fallis, D. (2020). The epistemic threat of deepfakes. Philosophy & Technology, 34(4), 623–643. https://doi.org/10.1007/s13347-020-00419-2
Gold, A., & Fischer, S. (2023, February 21). Chatbots trigger next misinformation nightmare. Axios. https://www.axios.com/2023/02/21/chatbots-misinformation-nightmare-chatgpt-ai
Fox-Sowell, S. (2024, February 20). Wisconsin requires labelling of AI-generated materials in campaign ads. State Scoop. https://statescoop.com/wisconsin-law-restricts-ai-generated-materials-campaign-ads/
Fuentes, Z. (2024, March 9). Biden calls for ban on AI voice generations during State of the Union. ABC News. https://abc7news.com/biden-state-of-the-union-address-ai-voice-generations-artificial-intelligence-regulations-ban/14505536/
Goel, S., Anderson, A., Hofman, J., & Watts, D. J. (2015). The structural virality of online diffusion. Management Science, 62(1), 150722112809007. https://doi.org/10.1287/mnsc.2015.2158
Groh, M., Epstein, Z., Firestone, C., & Picard, R. (2021). Deepfake detection by human crowds, machines, and machine-informed crowds. Proceedings of the National Academy of Sciences, 119(1). https://doi.org/10.1073/pnas.2110013119
Government of Canada. (2023). Canadian guardrails for generative AI – Code of practice. Innovation, Science and Economic Development Canada. https://ised-isde.canada.ca/site/ised/en/consultation-development-canadian-code-practice-generative-artificial-intelligence-systems/canadian-guardrails-generative-ai-code-practice
Gravino, P., Prevedello, G., Galletti, M., & Loreto, V. (2022). The supply and demand of news during COVID-19 and assessment of questionable sources production. Nature Human Behaviour, 6(8), 1069–1078. https://doi.org/10.1038/s41562-022-01353-3
Huang, S., & Siddarth, D. (2023). Generative AI and the digital commons. arXiv. https://doi.org/10.48550/arXiv.2303.11074
Humprecht, E., Esser, F., Aelst, P. V., Staender, A., & Morosoli, S. (2021). The sharing of disinformation in cross-national comparison: Analyzing patterns of resilience. Information, Communication & Society, 26(7), 1–21. https://doi.org/10.1080/1369118x.2021.2006744
Jacobsen, B. N. (2024). Deepfakes and the promise of algorithmic detectability. European Journal of Cultural Studies. https://doi.org/10.1177/13675494241240028
Kalpokas, I. (2020). Problematising reality: the promises and perils of synthetic media. SN Social Sciences, 1(1). https://doi.org/10.1007/s43545-020-00010-8
Krippendorff, K. (2011). Computing Krippendorff’s alpha-reliability. University of Pennsylvania. https://citeseerx.ist.psu.edu/document?repid=rep1&type=pdf&doi=de8e2c7b7992028cf035f8d907635de871ed627d
Le, B., Tariq, S., Abuadbba, A., Moore, K., & Woo, S. (2023, July). Why do facial deepfake detectors fail? In WDC ’23: Proceedings of the 2nd workshop on security implications of deepfakes and cheapfakes (pp. 24–28). Association for Computing Machinery. https://doi.org/10.1145/3595353.3595882
Leibowicz, C. R., McGregor, S., & Ovadya, A. (2021). The deepfake detection dilemma: A multistakeholder exploration of adversarial dynamics in synthetic media. In AIES ’21: Proceedings of the 2021 AAAI/ACM conference on AI, ethics, and society (pp. 736–744).https://doi.org/10.1145/3461702.3462584
Littman, M. L., Ajunwa, I., Berger, G., Boutilier, C., Currie, M., Doshi-Velez, F., Hadfield, G., Horowitz, M. C., Isbell, C., Kitano, H., Levy, K., Lyons, T., Mitchell, M., Shah, J., Sloman, S., Vallor, S., & Walsh, T. (2022). Gathering strength, gathering storms: The one hundred year study on artificial intelligence (AI100) 2021 study panel report. arXiv. https://doi.org/10.48550/arXiv.2210.15767
Lyu, S. (2020, July). Deepfake detection: Current challenges and next steps. In 2020 IEEE International Conference on Multimedia & Expo Workshops (ICMEW) (pp. 1–6). IEEE. https://doi.org/10.1109/ICMEW46912.2020.9105991
Lu, Z., Huang, D., Bai, L., Liu, X., Qu, J., & Ouyang, W. (2023). Seeing is not always believing: A quantitative study on human perception of AI-generated images. arXiv. https://doi.org/10.48550/arXiv.2304.13023
Manohar, S. (2020). Seeing is deceiving: The psychology and neuroscience of fake faces. PsyArXiv. https://doi.org/10.31234/osf.io/hz4yf
Pröllochs, N. (2022). Community-based fact-checking on Twitter’s Birdwatch platform. Proceedings of the International AAAI Conference on Web and Social Media, 16(1), 794–805. https://doi.org/10.1609/icwsm.v16i1.19335
Kerner, C., & Risse, M. (2021). Beyond porn and discreditation: epistemic promises and perils of deepfake technology in digital lifeworlds. Moral Philosophy and Politics, 8(1), 81–108. https://doi.org/10.1515/mopp-2020-0024
Biddlestone, M., Roozenbeek, J., & van der Linden, S. (2023, April 25). Twitter blue ticks: 5 ways to spot misinformation without verified accounts. The Conversation. https://theconversation.com/twitter-blue-ticks-5-ways-to-spot-misinformation-without-verified-accounts-204313
Sabel, J., & Stiff, H. Detecting generated media: A case study on Twitter data. NATO Publications. https://www.foi.se/download/18.3e84653f17d703503b9139/1639413985032/Detecting-generated-media_FOI-S–6422–SE.pdf
Deepfake audio of Sir Keir Starmer released on first day of Labour conference. (2024, October 9). Sky News. https://news.sky.com/story/labour-faces-political-attack-after-deepfake-audio-is-posted-of-sir-keir-starmer-12980181
Stosz, C. (2019, February 3). Policy options for fighting deepfakes. Georgetown Security Studies Review. https://georgetownsecuritystudiesreview.org/2019/02/03/policy-options-for-fighting-deepfakes/
Tolentino, D. (2023, March 27). AI-generated images of Pope Francis in puffer jacket fool the internet. NBC News. https://www.nbcnews.com/tech/pope-francis-ai-generated-images-fool-internet-rcna76838
Tursman, E. (2020). Detecting deepfakes using crowd consensus. XRDS: Crossroads, The ACM Magazine for Students, 27(1), 22–25. https://doi.org/10.1145/3416061
U.S. Representative Ritchie Torres. (2023, June 05). U.S. Rep. Ritchie Torres introduces federal legislation requiring mandatory disclaimer for material generated by Artificial Intelligence [Press release]. https://ritchietorres.house.gov/posts/u-s-rep-ritchie-torres-introduces-federal-legislation-requiring-mandatory-disclaimer-for-material-generated-by-artificial-intelligence
Vosoughi, S., Roy, D., & Aral, S. (2018). The spread of true and false news online. Science, 359(6380), 1146–1151. https://doi.org/10.1126/science.aap9559
Weikmann, T., & Lecheler, S. (2022). Visual disinformation in a digital age: A literature synthesis and research agenda. New Media & Society, 25(12), 3696–3713. https://doi.org/10.1177/14614448221141648
Whittaker, L., Kietzmann, T. C., Kietzmann, J., & Dabirian, A. (2020). “All around me are synthetic faces”: The mad world of AI-generated media. IT Professional, 22(5), 90–99. https://doi.org/10.1109/mitp.2020.2985492
Zagni, G., & Canetta, T. (2023, April 5). Generative AI marks the beginning of a new era for disinformation. European Digital Media Observatory. https://edmo.eu/edmo-news/generative-ai-marks-the-beginning-of-a-new-era-for-disinformation/
Funding
No funding has been received to conduct this research.
Competing Interests
The authors declare no competing interests.
Ethics
This research made use of publicly available data provided by X and of data openly published on the platform. Replication materials produced as part of this research do not disclose identifying information. No ethical review was required.
Copyright
This is an open access article distributed under the terms of the Creative Commons Attribution License, which permits unrestricted use, distribution, and reproduction in any medium, provided that the original author and source are properly credited.
Data Availability
All materials needed to replicate this study are available via the Harvard Dataverse: https://doi.org/10.7910/DVN/QYS1VH