Commentary
Tackling misinformation: What researchers could do with social media data
Article Metrics
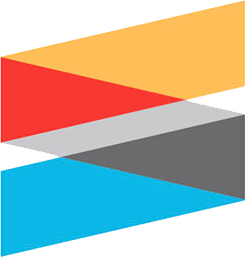
32
CrossRef Citations
Altmetric Score
PDF Downloads
Page Views
Written by Irene V. Pasquetto, Briony Swire-Thompson, Michelle A. Amazeen, Fabrício Benevenuto, Nadia M. Brashier, Robert M. Bond, Lia C. Bozarth, Ceren Budak, Ullrich K. H. Ecker, Lisa K. Fazio, Emilio Ferrara, Andrew J. Flanagin, Alessandro Flammini, Deen Freelon, Nir Grinberg, Ralph Hertwig, Kathleen Hall Jamieson, Kenneth Joseph, Jason J. Jones, R. Kelly Garrett, Daniel Kreiss, Shannon McGregor, Jasmine McNealy, Drew Margolin, Alice Marwick, FiIippo Menczer, Miriam J. Metzger, Seungahn Nah, Stephan Lewandowsky, Philipp Lorenz-Spreen, Pablo Ortellado, Gordon Pennycook, Ethan Porter, David G. Rand, Ronald E. Robertson, Francesca Tripodi, Soroush Vosoughi, Chris Vargo, Onur Varol, Brian E. Weeks, John Wihbey, Thomas J. Wood, & Kai-Cheng Yang
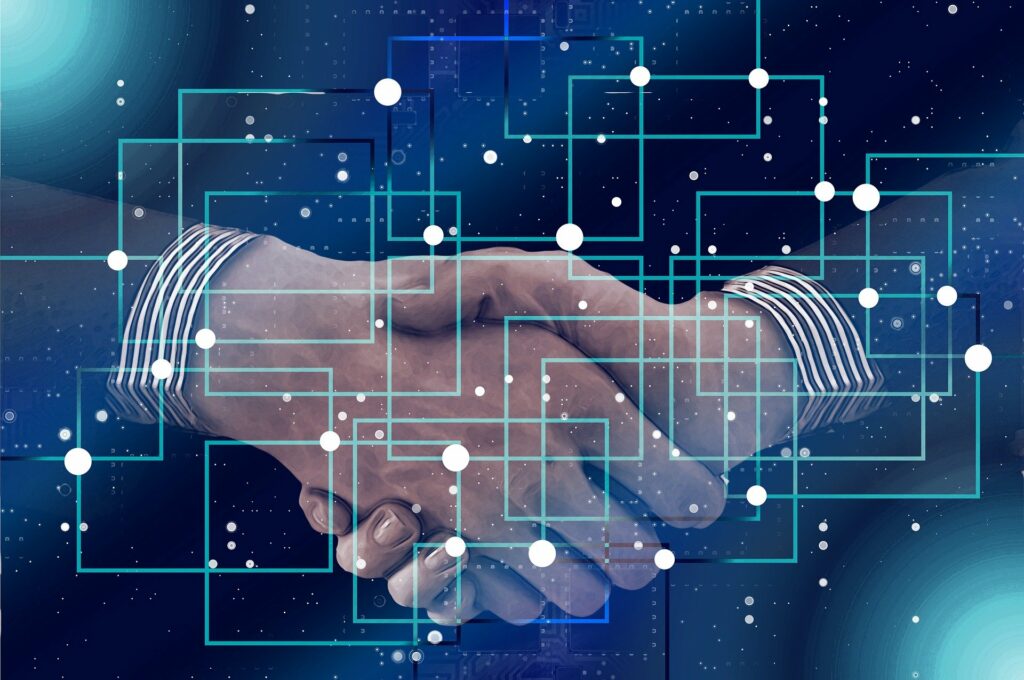
Introduction
Authors: Irene V. Pasquetto (1), Briony Swire-Thompson (2)
Affiliations: (1) School of Information, University of Michigan, USA; Shorenstein Center on Media, Politics and Public Policy, Harvard Kennedy School, USA (2) Network Science Institute, Northeastern University, USA; Institute of Quantitative Social Science, Harvard University, USA
Social media platforms rarely provide data to misinformation researchers. This is problematic as platforms play a major role in the diffusion and amplification of mis- and disinformation narratives. Scientists are often left working with partial or biased data and must rush to archive relevant data as soon as it appears on the platforms, before it is suddenly and permanently removed by deplatforming operations. Alternatively, scientists have conducted off-platform laboratory research that approximates social media use. While this can provide useful insights, this approach can have severely limited external validity (though see Munger, 2017; Pennycook et al. 2020). For researchers in the field of misinformation, emphasizing the necessity of establishing better collaborations with social media platforms has become routine. In-lab studies and off-platform investigations can only take us so far. Increased data access would enable researchers to perform studies on a broader scale, allow for improved characterization of misinformation in real-world contexts, and facilitate the testing of interventions to prevent the spread of misinformation. The current paper highlights 15 opinions from researchers detailing these possibilities and describes research that could hypothetically be conducted if social media data were more readily available. As scientists, our findings are only as good as the dataset at our disposal, and with the current misinformation crisis, it is urgent that we have access to real-world data where misinformation is wreaking the most havoc.
While new collaborative efforts are gradually emerging (e.g., Clegg, 2020; Mervis, 2020), they remain scarce and unevenly distributed across research communities and disciplines. Platforms periodically fund research initiatives on mis- and disinformation, but these rarely include increased access to data and algorithmic models. Most importantly, in these kinds of collaborations, intellectual freedom is easily limited by the fact that the overarching scope of the research is not defined by the researchers, but by the platforms themselves. In the rare case data sharing is a possibility, negotiations have been slow for several reasons, including platforms’ concerns over protecting their brands and reputation, and ethical and legal issues of privacy and data security on a grand scale (Bechmann & Kim, 2020; Olteanu et al., 2019). However, these barriers are not insurmountable (Moreno et al., 2013; Lazer et al. 2020). For instance, establishing a mechanism by which users can actively consent to various research studies, and potentially offering to make the data available to the participants themselves, would be a significant step forward (Donovan, 2020).
We invited misinformation researchers to write a 250-word commentary about the research that they would hypothetically conduct if they had access to consenting participants’ social media data. The excerpts below provide concrete examples of studies that misinformation researchers could conduct, if the community had better access to platforms’ data and processes. Based on the contents of the submission, we have grouped these brief excerpts into five areas that could be improved, and conclude with an excerpt regarding the importance of data sharing:
- measurement and design,
- who engages with misinformation and why,
- unique datasets with increased validity,
- disinformation campaigns,
- interventions, and
- the importance of data sharing.
While these excerpts are not comprehensive and may not be representative of the field as a whole, our hope is that this multi-authored piece will further the conversation regarding the establishment of more evenly distributed collaborations between researchers and platforms. Despite the challenges, on the other side of these negotiations are a vast array of potential discoveries that are needed by both the nascent field of misinformation as well as society.
I. Measurement and design
The need for impression data in misinformation research
Author: Soroush Vosoughi
Affiliation: Department of Computer Science, Dartmouth College, USA
One of the main challenges in studying misinformation on social media is the inability to get the true reach of the content that is being shared. There are two metrics that measure the reach of a piece of content being posted on social media: expressions and impressions. Expressions correspond to the people who engaged with the content (e.g., retweeted it or liked it), while impressions correspond to the people who read that content. While expression data can be used to map how misinformation spreads, the true impact of misinformation can only be measured using impression data. Expression data is usually made available by social media platforms; impression data, however, is kept hidden from researchers. If the social media platforms were to make fine-grained impression data (i.e., who read what, when) available, we would be able to measure the true reach of misinformation. This would allow us to study, amongst other things, the difference between posts containing misinformation that are read and shared to those that are read but not shared; and the difference between the people who read and share misinformation to those who read but do not share. This will shed light on some of the factors involved in people deciding whether to share content that contains misinformation, potentially allowing us to predict in advance the virality and the diffusion path of misinformation with much greater accuracy than is currently possible. Additionally, these findings can help devise more effective intervention strategies to dampen the spread of misinformation.
External randomized controlled trials (RCT) with no internal controls
Authors: Ethan Porter (1), Thomas J. Wood (2)
Affiliations: (1) School of Media & Public Affairs, The George Washington University, USA, (2) Department of Political Science, Ohio State University, USA
The most important contribution social media companies could make to the study of misinformation would be to allow external researchers to regularly conduct randomized controlled trials on their platforms, without interference or involvement from the companies themselves. While randomized control trials (RCTs) are among the strongest tools in a researcher’s toolbox, prior collaborations between academics and social media companies have prohibited them. Some companies have allowed researchers to conduct experiments, but these opportunities have been limited and questions of conflict of interest may arise. What is needed is a rolling opportunity for researchers to conduct independent RCTs on the largest platforms. Whether a proposed RCT is allowed should not be left to the discretion of the companies, but instead determined by a group of outside ethical and legal specialists, similar to a university Institutional Review Board (IRB). This group would minimize conflicts of interest and ensure that any research conducted would adhere to the IRB principles of confidentiality and privacy. Given such a system, a wide range of questions would suddenly become possible to answer. What kinds of interventions are most effective at reducing an individual’s propensity to share misinformation? Does exposure to one’s political opponents or allies affect willingness to share misinformation? To what extent, if any, does revealing the source of factual interventions affect behavior? This would just be the tip of the iceberg. Answers would come via individual-level data gathered on the platforms themselves, thus offering immediate external validity. Both the scientific and public understanding of the challenges of—and potential solutions to—misinformation would be significantly enhanced as a result.
What does the public need from social media platforms to really study fake news?
Authors: Kenneth Joseph (1), Nir Grinberg (2), John Wihbey (3)
Affiliations: (1) Department of Computer Science and Engineering, University at Buffalo, USA, (2) Department of Software and Information Systems Engineering, Ben-Gurion University, Israel, (3) School of Journalism and Ethics Institute, Northeastern University, USA
Social media platforms, to various degrees, already provide data for academic purposes. However, these data are often quite limited in their ability to answer critical research questions. Rather than ask “what would we do with platform data?” then, we here address the question, “what data and tools do we really need to make progress?” We identify five broad avenues of future collaboration between platforms and the academic community that will be a leap forward in public understanding of the usage and impacts of these platforms: (a) A framework for targeting academic-led experiments and survey to relevant populations (e.g., based on online user activity, profile information); (b) Information about individual-level exposure to (mis)information and the ways individuals interacted with that (mis)information; (c) Signals about message authenticity and the origination of actions taken on the platform (e.g. was this action likely taken via automated or human-like behavior?); (d) Transparent and up-to-date information about content curation and moderation by algorithms and company personnel, including political ads; and (e) broader data access to larger subsets of historical data and to a wider range of platforms, especially in the form of well-behaved APIs for Instagram, TikTok, WhatsApp, and YouTube. Forming these tighter collaborations raises important questions about privacy and trust, but finding solutions to these issues may be in the public’s best interest if we as a society were to understand the full gravity of the post-truth trends.
II. Who engages with misinformation and why
Understanding how communication about misinformation can help to combat it
Authors: Miriam J. Metzger (1), Andrew J. Flanagin (1)
Affiliations: (1) Department of Communication, University of California, Santa Barbara, USA
People spread misinformation by intentionally sharing it among their network contacts, and it is widely presumed that those sharing and receiving misinformation find it to be veracious. However, there are many reasons that people may share misinformation. For example, although people might share information because they believe it to be true, they may also share misinformation for entertainment purposes, sarcastic reasons, or to challenge the misinformation. Under such circumstances, the alleged danger of fake news may be mitigated or, perhaps, even reversed. While it is possible to study motivations for sharing misinformation using standard social scientific methods, self-report data are subject to social desirability biases because people may be reluctant to admit believing or sharing misinformation for any reason. It is also difficult to study motivations on a large scale in a fashion that is representative of social media users. Ideally, we would like to collaborate with social media platforms to capture at scale (a) the communication surrounding the sharing of mis- and disinformation by both information sharers and receivers (e.g., captions, comments, emojis, annotations, etc.), (b) people’s network relationship data, and (c) users’ demographic information to analyze the extent to which people believe misinformation as they share or receive it, as mediated by user characteristics and the sharer-receiver relationship. Such data could be appropriately anonymized to protect user identities. Understanding what misinformation is shared with whom and why would help to develop effective means to combat the spread of misinformation and would offer pathways to design and test interventions to help users to interpret misinformation correctly.
Why do older adults share more misinformation? We need social media data to find out
Authors: Nadia M. Brashier (1), Lisa K. Fazio (2)
Affiliations: (1) Department of Psychology, Harvard University, USA, (2) Department of Psychology and Human Development, Vanderbilt University, USA
Tackling the misinformation crisis requires a lifespan perspective, as older adults engage with (Grinberg et al., 2019) and share (Guess et al., 2019) more false political news on social media than any other age group. This is disturbing because older adults also vote at the highest rate (File, 2017). Similarly, misleading information about coronavirus is especially dangerous for older adults, who are at elevated risk of dying from COVID-19. Yet, without accurate data from social media companies, it is impossible to know exactly what false stories older adults see or why they are more likely to share them. For example, given that we lose peripheral acquaintances with age (Wrzus et al., 2013), older adults likely have close relationships with the people they friend and follow. With fewer weak ties, older adults may assume that content in their newsfeeds is accurate and quickly click ‘share’ (Brashier & Schacter, 2020), rather than pausing to think (Fazio, 2020). With access to users’ ages, the composition of their social networks, time spent viewing posts, and engagement with posts, we could better understand how older users experience social media. Given that repetition makes claims seem more credible to both young (Fazio et al., 2015) and older (Brashier et al., 2018) adults, it is also essential to know not just what people see, but also how often they see it. The data exist to test which social and cognitive factors leave older users particularly vulnerable to misinformation, but scientists need access.
Emotion, social media, and misinformation
Author: Brian E. Weeks
Affiliation: Department of Communication and Media and Center for Political Studies, University of Michigan, USA
Misinformation thrives on social media because of emotion. False, emotional content is clicked on, diffuses widely and rapidly through social networks, and is often believed, particularly when it fits with one’s political worldview. Yet, the degree to which emotion influences exposure to, engagement with, and belief in misinformation on social media remains shrouded by insufficient data from prominent platforms. What is needed is a more comprehensive picture of the emotional nature of misinformation in social media environments. Open data from social media platforms would help address critical, unanswered questions like how often do people encounter emotionally evocative misinformation? How are individuals exposed to emotional content that is false (e.g., social sharing/incidental exposure, selective exposure, algorithmic filtering)? To what degree does misinformation play on emotions stemming from ideological, political, racial, or religious biases? How frequently do people engage (e.g., click, share, comment) with emotional misinformation and to what effect? Does encountering emotional falsehoods drive exposure to more extreme or partisan political content, contribute to polarization, and promote acceptance of false beliefs and conspiracy theories? Open data from social media platforms would also facilitate understanding of how different emotions like anger and fear uniquely amplify misinformation and deepen misperceptions. These questions demonstrate the urgent need to better understand the emotional environments in which misinformation flourishes on social media. More transparency and open data practices from social media platforms would illuminate the processes and mechanisms through which emotional misinformation is encountered, spread, and believed.
III. Richer datasets with improved validity
The case for studying obscure falsehoods
Authors: Robert M. Bond (1), Lia C. Bozarth (2), Ceren Budak (2), R. Kelly Garrett (1), Jason J. Jones (3), Drew Margolin (4)
Affiliations: (1) School of Communication, Ohio State University, USA, (2) School of Information, University of Michigan, USA, (3) Department of Sociology and Institute for Advanced Computational Science, Stony Brook University, USA, (4) Department of Communication, Cornell University, USA
Research on misinformation often focuses on non-representative sets of claims. Sometimes this decision is theoretically motivated, as when a falsehood is uniquely harmful (e.g., the thoroughly debunked “link” between vaccines and autism, see Motta et al., 2018). More often the decision reflects practical concerns, as when researchers use fact checkers’ archives to identify which claims to study (e.g., Vosoughi et al., 2018), despite the significant selection bias introduced by focusing on high-diffusion cases (Goel et al., 2012). Emphasizing “successful” misinformation also overlooks important variants, as most political falsehoods fail to find a large audience (Allen et al., 2020; Guess et al., 2019) and are shared primarily within small enclaves (Bail et al., 2019; Grinberg et al., 2019; Guess et al., 2020). Including both popular and unpopular misinformation in analyses would allow researchers to answer important questions. Do the two types of falsehoods differ in the extent to which they rely on viral or broadcast diffusion (i.e., their structural diffusion, see Goel et al., 2016)? Under what conditions will a falsehood go viral? Are there attributes that consistently characterize networks in which misinformation thrives? Are some types of events, policies, or technologies uniquely susceptible to mis- or disinformation campaigns? Answering these questions requires access to fine-grained temporal data about when content is shared, to aggregate characteristics about the populations that share them, and to characteristics about the networks in which the messages are shared. Importantly, though, it does not require access to individuals’ personal information or behavior.
WhatsApp data that could help research on misinformation
Authors: Fabrício Benevenuto (1), Pablo Ortellado (2)
Affiliations: (1) Computer Science Department, Universidade Federal de Minas Gerais, Brazil, (2) Escola de Artes, Ciências e Humanidades, Universidade de São Paulo, Brazil
WhatsApp allegedly has been widely used to spread misinformation during elections, especially in Brazil and India (Tardaguila et al., 2018). Due to the private encrypted nature of the messages on WhatsApp, it is hard for researchers to track the dissemination of misinformation at scale and, ultimately, to investigate approaches able to mitigate the problem. Most of the research in this space has explored data shared in public groups (Resende et al., 2019). The research community would greatly benefit from WhatsApp disclosing the following information:
- Aggregated information about users and uses of the platform. Number of users, number of groups, distribution of the size of those groups, distribution of frequency of messages sent to individual users and groups etc. This could be used for any researcher studying the platform and would allow anyone to better comprehend the use of the platform across countries.
- Viral and widely-spread content. WhatsApp has been limiting the spread of content they consider to be viral. Viral content reaches a large number of users and may represent information that is of public interest. This content might be of interest not only for researchers studying misinformation, but also for journalism and fact checkers. While protecting privacy, WhatsApp could record the number of times a given content has been distributed and provide this information through an API. This information could be similar to that provided by Facebook through its Index API. This would allow researchers to measure the spread of a given content inside WhatsApp.
- A random sample of names of groups. This would allow studies about how different groups use WhatsApp.
Misinformed citizens across social media platforms: Unraveling the effects of misinformation on social capital and civic participation
Authors: Jasmine McNealy (1), Seungahn Nah (2)
Affiliations: (1) College of Journalism and Communication, University of Florida, USA, (2) School of Journalism and Communication, University of Oregon, USA
While research has long shown that informational use of news media has democratic value, there are few empirical findings on the civic consequences of exposure to misinformation across social media platforms. The proposed study develops a data infrastructure theory (DIT) grounded in communication infrastructure theory (CIT). CIT assumes that connections to community storytelling networks, such as local media, residents, and community organizations, foster civic engagement (Kim & Ball-Rokeach, 2006). In comparison, DIT posits that data available across social media will activate communication infrastructure, which, in turn may stimulate democratic values such as public discussion, social capital (e.g., social trust), and civic participation. We conceive social media platforms as data infrastructure for creating, conveying, and communicating misinformation embedded in a sheer volume of data. This study proposes several testable hypotheses: (a) exposure to misinformation across social media platforms will be negatively associated with trust in social media and social trust or trust in others, (b) exposure to misinformation across social media platforms will be negatively associated with less participation in public discussion, and (c) this exposure will lead to less involvement in civic and political activities. The hypotheses will be tested using a unique dataset that would include user consumption and production habits, as well as content exposure, and time spent on several social media platforms coupled with other information like an online survey and in-depth interviews of users who have been exposed to misinformation across social media platforms. In particular, we would examine the accuracy of the self-reported habits and engagement, as well as investigate social capital and civic participation in relation to the social media data set. Implications of misinformation exposure on democracy, and civic engagement will be discussed. We will also explore the impacts of misinformation on different communities in relation to both demographic data, as well as construct-developed categories of users.
IV. Disinformation campaigns
Data sharing protocols for content deletion and identity change activities to counter online manipulation
Authors: Onur Varol (1), Kai-Cheng Yang (2), Emilio Ferrara (3), Alessandro Flammini (2), FiIippo Menczer (2)
Affiliations: (1) Faculty of Engineering and Natural Sciences, Sabanci University, Turkey, (2) Observatory on Social Media, Indiana University, USA, (3) Information Sciences Institute, University of Southern California, USA
Efforts to disseminate disinformation and manipulate public opinion show similarities with early propaganda and persuasion campaigns (Lazer et al. 2018; Starbird, 2019; Varol & Uluturk, 2018). However, social media platforms now empower online information operations with improved means of concealing provenance, targeting vulnerable individuals, and rapid evaluation/optimization of strategies and narratives. All social media platforms offering programmatic interfaces (APIs) are vulnerable — and exploited. Inauthentic, coordinated, malicious accounts, whether human-controlled or automated (Ferrara et al., 2016; Shao et al., 2018; Varol et al., 2017; Yang et al., 2019), domestic or state-sponsored (Zannettou et al., 2019), or anywhere in between, have been weaponized to influence public conversations by amplifying and flooding content. Bot and troll accounts can systematically delete old posts and change identities to evade detection. Platforms should provide APIs for accredited researchers to access data needed to study, detect, and combat manipulation, such as statistics on deletions, profile changes, and 3rd-party application activities without breaching privacy preferences set by the users. Another severe limitation is the lack of access to historical data. Temporal anomalies leave marks of coordinated activities and remain detectable years after an operation (Varol & Uluturk, 2019). Access to past content deletions and changes of identities should be facilitated. Consistent data sharing protocols are needed to make temporal data about API activity, removed content, abuse reports, and suspended accounts available for research. The protocols should sanction research according to ethical principles and privacy regulations rather than inconsistent and ever-changing terms of service. Greater transparency is crucial to move research efforts from observational analyses to science- and data-driven policies (Aral & Eckles, 2019) to protect our marketplaces of ideas and our democracies.
What release of Russian 2016 troll data could reveal
Author: Kathleen Hall Jamieson
Affiliation: Annenberg School of Communication, University of Pennsylvania, USA
Since the Trump presidency has reshaped the post-Cold War US-Russia-NATO relationship, history deserves an answer to the question: How likely is it that the 2016 Kremlin interventions made the presidential election close enough to be decided by 78,000 votes in three battleground states? In the second edition of Cyberwar: How Russian Hackers and Trolls Helped Elect a President, I argue that the changed media agenda created by press use of Russian-hacked Democratic content likely had a decisive effect on Democratic nominee Hillary Clinton’s standing in the polls and that the impact of the Russian trolls was negligible by comparison. Ironically, since much of the accessible Russian content is not directly election related, and the trolls used spam to artificially inflate their engagement and following metrics, it is likely that full transparency would further deflate estimates of the Russian social media saboteurs’ impact. To confirm that the platforms deserve to be let off the hook, they should do two things: (a) release all of the organic Russian content, as Twitter has done, to make it possible to determine how much was election-related and (b) reveal what they know about troll uptake in battleground states and across the nation by groups that the Kremlin-tied social media imposters were trying to demobilize (e.g., Black voters), mobilize (e.g., conservative Catholics and evangelical Protestants and those in military households), and shift (young Sanders’ supporters to Green party candidate Jill Stein).
V. Interventions
Evaluating interventions to fight misinformation
Authors: Gordon Pennycook (1), David G. Rand (2)
Affiliations: (1) Hill/Levene Schools of Business, University of Regina, Canada, (2) Sloan School of Management, Massachusetts Institute of Technology, USA
Our primary interest is in exploring the impact of interventions to reduce the spread of false and misleading content online. Experimental investigation of interventions, rather than implementation based on intuitive appeal, is essential for effectively meeting the misinformation challenge (Pennycook & Rand, 2020). Thus, our ideal experiments would involve randomly assigning users of platforms such as Facebook and Twitter to various intervention versus control conditions. We would then measure the interventions’ impact on which pieces of content (conditional on exposure) were shared, reacted to, and commented on, which links (e.g., to news sites) were clicked, and how long users spent reading each piece of content. Ideally, we would also pair these on-platform metrics with follow-up surveys in which we could directly assess users’ beliefs and attitudes. Specific interventions we would want to investigate include labeling news headlines with fact-checking warnings (Clayton et al., 2019; Pennycook, Bear, et al., 2020), prompts that nudge users to consider accuracy before sharing (Fazio, 2020; Pennycook, Epstein, et al., 2020; Pennycook, McPhetres et al., in press), and attempts to increase digital literacy (which likely also prime accuracy) (Guess et al., 2020). Finally, we would also be interested in assessing the impact of incorporating layperson (user) accuracy ratings, either of news sources (Epstein et al, 2020; Pennycook & Rand, 2019) or individual pieces of content (Allen, Arechar, et al. 2020), into social media ranking algorithms. Such investigations would provide clear, concrete evidence about which approaches are most effective (and which may actually be counterproductive). Without such evidence, it is impossible for the public to have faith in social media platforms’ efforts to curb misinformation.
The influence of fact checks and advertising
Authors: Michelle A. Amazeen (1), Chris Vargo (2)
Affiliations: (1) Department of Mass Communication, Advertising and Public Relations, Boston University, USA, (2) Department of Advertising, Public Relations and Media Design, University of Colorado Boulder, USA
Social media platforms, including Facebook, have entered into agreements with third parties to provide fact-checks of content circulating on their platforms. Despite having partners around the world (Goldshlager, 2020), misinformation continues (Robertson, 2020). Fact-checking partners don’t know how well their efforts perform at reducing the spread of misinformation (Lu, 2019). Our dream research, consequently, centers around the transparency and accountability of social media efforts to address misinformation. We need an API endpoint that shows the specific actions platforms take once a message is identified as containing misinformation, including removal, warning labels, and downranking. When considering downranking or shadow banning, even more unknowns exist. Who still sees downranked content? How does that vary across demographics and psychographics? How do mitigation tactics affect the way audiences respond (liking, sharing, commenting, etc.)? Researchers need visibility into these actions to assess how political ideology, media use, and media literacy interact with the steps platforms are taking to correct misinformation. Furthermore, content on social media is narrowly targeted to specific audiences. Both political and commercial ads are targeted to users based on their pre-existing attitudes, beliefs, and fears (Borden King, 2020; Young & McGregor, 2020). While Facebook and Twitter have robust APIs, there is no way for researchers to identify ads in real-time. We also desire the ability to assess the damage targeted influence has on platforms and believe that researchers and platforms can work together to understand these consequences and ultimately build better systems.
Understanding digital mis- and disinformation: Origins, algorithms, and interventions
Authors: Alice E. Marwick (1), Deen Freelon (2), Daniel Kreiss (2), Shannon McGregor (2), Francesca Tripodi (3)
Affiliations: (1) Department of Communication, University of North Carolina at Chapel Hill, USA, (2) Hussman School of Journalism, University of North Carolina at Chapel Hill, USA, (3) School of Information and Library Science, University of North Carolina at Chapel Hill, USA
1. Where and with whom does viral mis/disinformation originate? Determining the origin of a misleading story is currently next-to-impossible. With cross-platform data access, we could identify the first time a conspiratorial YouTube video or debunked political claim is shared on social media. Current research suggests that disinformation often comes from fringe networks and spreads through mainstream social media (Freelon et al., 2020), but we don’t know for sure, and we don’t know who creates and disseminates much of it. Determining where disinformation originates, and which platforms are most hospitable to its spread is the first step in decreasing its amplification and reach.
2. Which mis/disinformation correction strategies work best with which audiences or demographics? Current scholarship offers mixed findings and scant data on the effectiveness of oft-proposed solutions to mis/disinformation like fact-checking (e.g., Ecker et al., 2020; Lyons et al., 2020). With increased access and user consent, researchers could observe things such as organic fact-checking—how people react when a friend or group member points out incorrect information they have shared—and track whether corrections are equally effective across demographics, issues, and sources. This would allow us to create robust and effective intervention strategies with minimal unforeseen negative effects.
3. Which platform algorithms play the biggest role in spreading—or curbing—mis/disinformation? Critics claim that platform algorithms like Facebook’s news feed amplify harmful content, which we could test empirically. Does YouTube send people down a rabbit hole of radicalization? What role does Facebook’s Ad Auction play in driving mis/disinformation? Does TikTok use its algorithm to suppress or amplify demographics or viewpoints? This baseline knowledge is needed to assess, plan, and implement technical interventions, which are currently shrouded in obscurity. Providing scholars with the data to answer these questions is vital to understanding the cross- and multi-platform nature of—and solutions to—mis- and disinformation.
VI. Why data sharing is important
Dream research and the ownership of cultural artefacts: The need to reclaim the information ecology
Authors: Stephan Lewandowsky (1,2), Ullrich K. H. Ecker (2), Ralph Hertwig (3), Philipp Lorenz-Spreen (3), Ronald E. Robertson (4)
Affiliations: (1) School of Psychological Science, University of Bristol, UK, (2) School of Psychological Science, The University of Western Australia, Australia, (3) Max-Planck Institute for Human Development, Germany, (4) Network Science Institute, Northeastern University, USA
The information ecology produced by social media platforms and the data they collect is part of our cultural heritage. These data represent “libraries” of the present and the future, and they should be considered cultural artefacts that, like their physical counterparts, ought to be “owned” by the people who produced them—which is all of us. We must reclaim the information ecology for the people who created it. This is crucial for independent research in the public interest: to conduct such “dream research,” researchers must not be supplicants to social-media companies but should go straight to the users, recruiting people as “citizen scientists” and knowledge-co-creators. This can be achieved via dedicated research platforms and browser plug-ins, mobile applications, and other digital data collection tools that allow researchers access to people’s online activity subject to strict confidentiality and anonymity constraints. These data could constitute a common cultural good, permitting vital research without involvement or control by corporate interests. Some of the questions we could then address include examinations of (a) how malicious disinformation (e.g., concerning COVID-19) affects subsequent content engagement, and how this is shaped by countermeasures already in place or being designed by empirical research; (b) people’s motives to share information, in particular intentional sharing of information that is known to be false; (c) drivers of polarization and de-polarization; and (d) ways in which a common ground for evidence and rules of arguments can be re-established.
Bibliography
Allen, J., Arechar, A. A., Pennycook, G., & Rand, D. G. (2020). Scaling up fact-checking using the wisdom of crowds. Preprint available at https://psyarxiv.com/9qdza/
Allen, J., Howland, B., Mobius, M., Rothschild, D., & Watts, D. J. (2020). Evaluating the fake news problem at the scale of the information ecosystem. Science Advances, 6(14). https://doi.org/10.1126/sciadv.aay3539
Aral, S., & Eckles, D. (2019). Protecting elections from social media manipulation. Science, 365(6456), 858-861. https://doi.org/10.1126/science.aaw8243
Bail, C. A., Guay, B., Maloney, E., Combs, A., Hillygus, D. S., Merhout, F., Freelon, D., & Volfovsky, A. (2019). Assessing the Russian Internet Research Agency’s impact on the political attitudes and behaviors of American Twitter users in late 2017. Proceedings of the National Academy of Sciences, 117(1), 243-250. https://doi.org/10.1073/pnas.1906420116
Bechmann, A., & Kim, J. Y. (2020). Big data: A focus on social media research dilemmas. In R. Iphofen, Handbook of Research Ethics and Scientific Integrity (pp. 427-444). Springer.
Borden King, A. (2020, July 10). I have cancer: Now my Facebook feed is full of ‘alternative care’ ads. The New York Times. https://www.nytimes.com/2020/07/10/opinion/facebook-cancer-ads.html
Brashier, N. M., & Schacter, D. L. (2020). Aging in an era of fake news. Current Directions in Psychological Science, 29(3), 316-323. https://doi.org/10.1177/0963721420915872
Brashier, N. M., Umanath, S., Cabeza, R., & Marsh, E. J. (2017). Competing cues: Older adults rely on knowledge in the face of fluency. Psychology and Aging, 32(4), 331-337. https://doi.org/10.1037/pag0000156
Clayton, K., Blair, S., Busam, J. A., Forstner, S., Glance, J., Green, G., Kawata, A., Kovvuri, A., Martin, J., Morgan, E., Sandhu, M., Sang, R., Scholz-Bright, R., Welch, A. T., Wolff, A.G., Zhou, A., & Nyhan, B. (2019). Real solutions for fake news? Measuring the effectiveness of general warnings and fact-check tags in reducing belief in false stories on social media. Political Behavior, 42, 1073–1095. https://doi.org/10.1007/s11109-019-09533-0
Clegg, N., & Nayak, C. (2020). New Facebook and Instagram research initiative to look at US 2020 presidential election. Facebook.https://about.fb.com/news/2020/08/research-impact-of-facebook-and-instagram-on-us-election/
Donovan, J. (2020). Redesigning consent: Big data, bigger risks. The Harvard Kennedy School (HKS) Misinformation Review, 1(1). https://doi.org/10.37016/mr-2020-006
Ecker, U. K., Lewandowsky, S., & Chadwick, M. (2020). Can corrections spread misinformation to new audiences? Testing for the elusive familiarity backfire effect. Cognitive Research: Principles and Implications, 5(1), 1-25. https://doi.org/10.31219/osf.io/et4p3
Epstein, Z., Pennycook, G., & Rand, D. G. (2020). Will the crowd game the algorithm? Proceedings of the 2020 CHI Conference on Human Factors in Computing Systems (pp. 1–11). https://doi.org/10.1145/3313831.3376232
Fazio, L. K. (2020). Pausing to consider why a headline is true or false can help reduce the sharing of false news. Harvard Kennedy School (HKS) Misinformation Review, 1(2), https://doi.org/10.37016/mr-2020-009
Fazio, L. K., Brashier, N. M., Payne, B. K., & Marsh, E. J. (2015). Knowledge does not protect against illusory truth. Journal of Experimental Psychology: General, 144(5). http://dx.doi.org/10.1037/xge0000098
Ferrara, E., Varol, O., Davis, C., Menczer, F., & Flammini, A. (2016). The rise of social bots. Communications of the ACM, 59(7), 96-104. https://doi.org/10.1145/2818717
File, Thom. (2017). Voting in America: A look at the 2016 presidential election. The United States Census Bureau. https://www.census.gov/newsroom/blogs/random-samplings/2017/05/voting_in_america.html
Freelon, D., Bossetta, M., Wells, C., Lukito, J., Xia, Y., & Adams, K. (2020). Black trolls matter: Racial and ideological asymmetries in social media disinformation. Social Science Computer Review, 0894439320914853.https://doi.org/10.1177%2F0894439320914853
Goel, S., Anderson, A., Hofman, J., & Watts, D. J. (2016). The structural virality of online diffusion. Management Science, 62(1), 180-196. https://doi.org/doi:10.1287/mnsc.2015.2158
Goel, S., Watts, D. J., & Goldstein, D. G. (2012). The structure of online diffusion networks. Proceedings of the 13th ACM Conference on Electronic Commerce (pp. 623-638). https://doi.org/10.1145/2229012.2229058
Goldshlager, K. (2020, June 26). Expanding Facebook’s U.S. fact-checking program and supporting the fact-checking ecosystem.Facebook. https://www.facebook.com/journalismproject/fact-checking-expansion-and-investment-2020
Grinberg, N., Joseph, K., Friedland, L., Swire-Thompson, B., & Lazer, D. (2019). Fake news on Twitter during the 2016 U.S. presidential election. Science, 363(6425), 374-378. https://doi.org/10.1126/science.aau2706
Guess, A. M., Lerner, M., Lyons, B., Montgomery, J. M., Nyhan, B., Reifler, J., & Sircar, N. (2020). A digital media literacy intervention increases discernment between mainstream and false news in the United States and India. Proceedings of the National Academy of Sciences, 117 (27) 15536-15545. https://doi.org/10.1073/pnas.1920498117
Guess, A. M., Nyhan, B., & Reifler, J. (2020). Exposure to untrustworthy websites in the 2016 US election. Nature Human Behaviour, 4(5), 472-480. https://doi.org/10.1038/s41562-020-0833-x
Guess, A., Nagler, J., & Tucker, J. (2019). Less than you think: Prevalence and predictors of fake news dissemination on Facebook. Science Advances, 5(1), eaau4586. https://doi.org/10.1126/sciadv.aau4586
Lazer, D. M., Baum, M. A., Benkler, Y., Berinsky, A. J., Greenhill, K. M., Menczer, F., Nyhan, B., Pennycook, G., Rothschild, D., Schudson, M., Sloman, S. A., Sunstein, C. R., Thorson, E. A.,Watts, D. J., & Zittrain, J. L. (2018). The science of fake news. Science, 359(6380), 1094-1096. https://doi.org/10.1126/science.aao2998
Lazer, D. M., Pentland, A., Watts, D. J., Aral, S., Athey, S., Contractor, N., Freelon, D., Gonzalez-Bailon, S., King, G., Margetts, H., Nelson A., Salganik, M.J., Strohmaier, M., Vespignani, A., & Wagner, C. (2020). Computational social science: Obstacles and opportunities. Science, 369(6507), 1060-1062. https://doi.org/10.1126/science.aaz8170
Lu, D. (2019, July 30). Facebook’s fact-checking process is too opaque to know if it’s working. New Scientist. https://www.newscientist.com/article/2211634-facebooks-fact-checking-process-is-too-opaque-to-know-if-its-working/
Lyons, B., Mérola, V., Reifler, J., & Stoeckel, F. (2020). How politics shape views toward fact-checking: Evidence from six European countries. The International Journal of Press/Politics, 25(3), 469-492.
Mervis, J. (2020) Researchers finally get access to data on Facebook’s role in political discourse. Science. https://www.sciencemag.org/news/2020/02/researchers-finally-get-access-data-facebook-s-role-political-discourse
Motta, M., Callaghan, T., & Sylvester, S. (2018). Knowing less but presuming more: Dunning-Kruger effects and the endorsement of anti-vaccine policy attitudes. Social Science & Medicine, 211, 274-281. https://doi.org/10.1016/j.socscimed.2018.06.032
Moreno, M. A., Goniu, N., Moreno, P. S., & Diekema, D. (2013). Ethics of social media research: Common concerns and practical considerations. Cyberpsychology, Behavior, and Social Networking, 16(9), 708-713. https://dx.doi.org/10.1089%2Fcyber.2012.0334
Munger, K. (2017). Tweetment effects on the tweeted: Experimentally reducing racist harassment. Political Behavior, 39(3), 629-649.
Olteanu, A., Castillo, C., Diaz, F., & Kiciman, E. (2019). Social data: Biases, methodological pitfalls, and ethical boundaries. Frontiers in Big Data, 2, 13. https://doi.org/10.3389/fdata.2019.00013
Pennycook, G., & Rand, D. G. (2019). Fighting misinformation on social media using crowdsourced judgments of news source quality. Proceedings of the National Academy of Sciences, 116(7) 2521-2526. https://doi.org/10.1073/pnas.1806781116
Pennycook, G., Epstein, Z., Mosleh, M., Arechar, A. A., Eckles, D., & Rand, D. (2020). Understanding and reducing the spread of misinformation online. Preprint available at: https://psyarxiv.com/3n9u8/
Pennycook, G., & Rand, D. G. (2020, March 24). The right way to fight fake news. The New York Times.https://www.nytimes.com/2020/03/24/opinion/fake-news- social-media.html
Pennycook, G., Bear, A., Collins, E., & Rand, D. G. (2020). The implied truth effect: Attaching warnings to a subset of fake news stories increases perceived accuracy of stories without warnings. Management Science, 66(11), 4944-4957. https://doi.org/10.1287/mnsc.2019.3478
Pennycook, G., McPhetres, J., Zhang, Y., Lu, J. G., & Rand, D. G. (in press). Fighting COVID-19 misinformation on social media: Experimental evidence for a scalable accuracy nudge intervention. Psychological Science. https://doi.org/10.31234/OSF.IO/UHBK9
Resende, G., Melo, P., Sousa, H., Messias, J., Vasconcelos, M., Almeida, J., & Benevenuto, F. (2019, May). (Mis)Information dissemination in WhatsApp: Gathering, analyzing and countermeasures. The World Wide Web Conference (pp. 818-828). https://doi.org/10.1145/3308558.3313688
Robertson, A. (2020, March 3). Facebook fact-checking is becoming a political cudgel. The Verge.https://www.theverge.com/2020/3/3/21163388/facebook-fact-checking-trump-coronavirus-hoax-comment-politico-daily-caller
Shao, C., Ciampaglia, G. L., Varol, O., Yang, K. C., Flammini, A., & Menczer, F. (2018). The spread of low-credibility content by social bots. Nature Communications, 9(1), 1-9. https://doi.org/10.1038/s41467-018-06930-7
Silverman, C., Mac, R., & Dixit, P. (2020). “I have blood on my hands”: A whistleblower says Facebook ignored global political manipulation. Buzzfeed News. https://www.buzzfeednews.com/article/craigsilverman/facebook-ignore-political-manipulation-whistleblower-memo
Starbird, K. (2019). Disinformation’s spread: Bots, trolls and all of us. Nature, 571(7766), 449-450.
Tardáguila, C., Benevenuto, F., & Ortellado, P. (2018, Oct. 17). Fake news is poisoning Brazilian politics. WhatsApp can stop it. The New York Times. https://www.nytimes.com/2018/10/17/opinion/brazil-election-fake-news-whatsapp.html
Varol, O., Ferrara, E., Davis, C. A., Menczer, F., & Flammini, A. (2017). Online human-bot interactions: Detection, estimation, and characterization. Proceedings of the Eleventh International Conference on Web and Social Media (ICWSM).https://arxiv.org/pdf/1703.03107.pdf
Varol, O., & Uluturk, I. (2018). Deception strategies and threats for online discussions. First Monday, 23(5). https://doi.org/10.5210/fm.v22i5.7883
Varol, Onur, & Uluturk, I. (2019) Journalists on Twitter: Self-branding, audiences, and involvement of bots. Journal of Computational Social Science, 3, 83-101. https://doi.org/10.1007/s42001-019-00056-6
Vosoughi, S., Roy, D., & Aral, S. (2018). The spread of true and false news online. Science, 359(6380), 1146-1151. https://doi.org/10.1126/science.aap9559
Wrzus, C., Hanel, M., Wagner, J., & Neyer, F. J. (2013). Social network changes and life events across the life span: A meta-analysis. Psychological Bulletin, 139(1), 53-80. https://doi.org/10.1037/a0028601
Yang, K. C., Varol, O., Davis, C. A., Ferrara, E., Flammini, A., & Menczer, F. (2019). Arming the public with artificial intelligence to counter social bots. Human Behavior and Emerging Technologies, 1(1), 48-61. https://doi.org/10.1002/hbe2.115
Young, D. G., & McGregor, S. (2020, February 14). Mass propaganda used to be difficult, but Facebook made it easy. The Washington Post. https://www.washingtonpost.com/outlook/2020/02/14/mass-propaganda-used-be-difficult-facebook-made-it-easy/
Zannettou, S., Caulfield, T., De Cristofaro, E., Sirivianos, M., Stringhini, G., & Blackburn, J. (2019, May). Disinformation warfare: Understanding state-sponsored trolls on Twitter and their influence on the web. Companion Proceedings of the 2019 World Wide Web Conference (pp. 218-226). https://doi.org/10.1145/3308560.3316495
Copyright
This is an open access article distributed under the terms of the Creative Commons Attribution License, which permits unrestricted use, distribution, and reproduction in any medium, provided that the original author and source are properly credited.