Peer Reviewed
State media warning labels can counteract the effects of foreign misinformation
Article Metrics
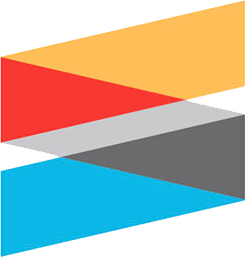
14
CrossRef Citations
Altmetric Score
PDF Downloads
Page Views
Platforms are increasingly using transparency, whether it be in the form of political advertising disclosures or a record of page name changes, to combat disinformation campaigns. In the case of state-controlled media outlets on YouTube, Facebook, and Twitter this has taken the form of labeling their connection to a state. We show that these labels have the ability to mitigate the effects of viewing election misinformation from the Russian media channel RT. However, this is only the case when the platform prominently places the label so as not to be missed by users.
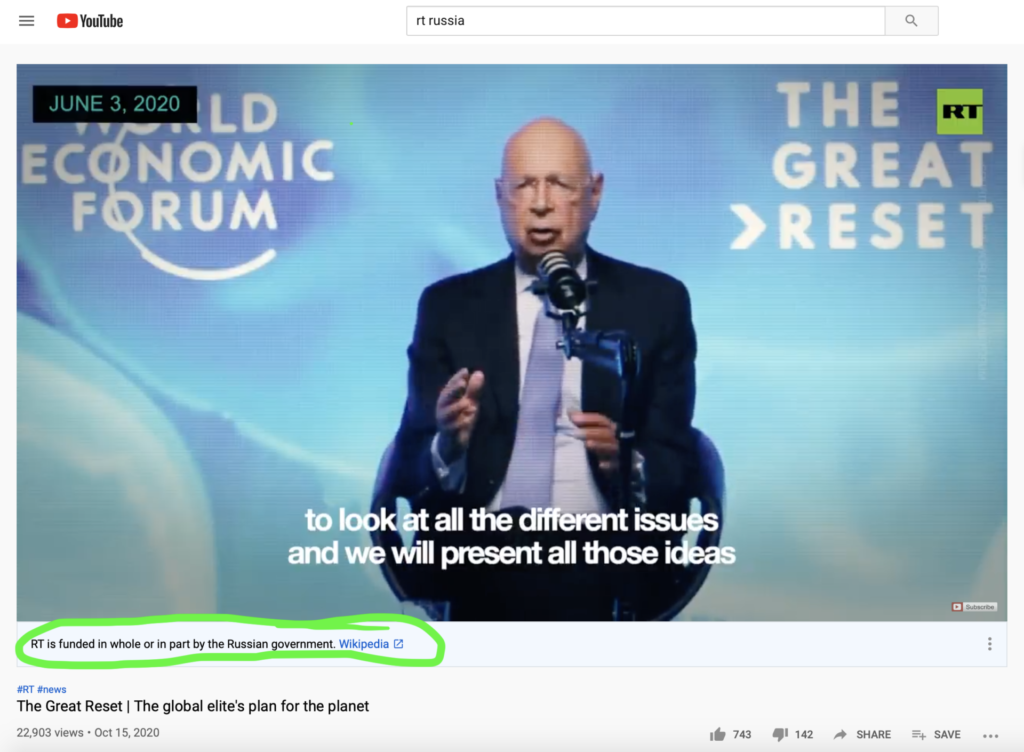
Research Questions
- Are YouTube’s warning labels that RT is state-funded effective at increasing knowledge of RT’s lack of independence?
- What is the effect of RT YouTube videos containing 2016 and 2020 US election misinformation on viewer perceptions?
- Do YouTube’s warning labels mitigate any shifts in perception that the misinformation in the RT video creates?
Essay Summary
- In order to test the efficacy of YouTube’s disclaimers, we ran two experiments presenting participants with one of four videos: A non-political control, an RT video without a disclaimer, an RT video with the real disclaimer, or the RT video with a custom implementation of the disclaimer superimposed onto the video frame.
- The first study, conducted in April 2020 (n = 580) used an RT video containing misinformation about Russian interference in the 2016 election. The second conducted in July 2020 (n = 1,275) used an RT video containing misinformation about Russian interference in the 2020 election.
- Our results show that misinformation in RT videos has some ability to influence the opinions and perceptions of viewers. Further, we find YouTube’s funding labels have the ability to mitigate the effects of misinformation, but only when they are noticed, and the information absorbed by the participants.
- The findings suggest that platforms should focus on providing increased transparency to users where misinformation is being spread. If users are informed, they can overcome the potential effects of misinformation. At the same time, our findings suggest platforms need to be intentional in how warning labels are implemented to avoid subtlety that may cause users to miss them.
Implications
The rise of disinformation campaigns on social media has led platforms to lay out wide-ranging solutions to mitigate foreign interference — from building out automatic detection mechanisms for coordinated inauthentic behavior to manual investigations of suspicious networks (Gleicher, 2018). However, when it comes to disinformation from state-controlled media sources platforms’ options are more limited. Most often channels like Russia’s RT1RT, formerly Russia Today, is a Russian state media outlet, headquartered in Moscow and aimed at foreign audiences. Founded in 2005 RT’s original purpose was to provide “’perspective on the world from Russia’” (Ioffe, 2010). But following Russia’s invasion of Georgia in 2008 it began to aim to degrade the West and produce disinformation (Elswah & Howard, 2020). Today it operates 22 worldwide bureaus including English, Spanish and Arabic content. It’s budget of over 400 million dollars in the last known year (2015) is sourced entirely from the Russian government, as it does not rely on advertising revenue (Elswah & Howard, 2020). and Iran’s PressTV do not technically violate a platform’s terms of service and so cannot be removed. However, they still play a vital role in the disinformation ecosystem. Not only do they put out disinformation through their websites and social media channels, they are key nodes in coordinated campaigns, as well. For instance, the content originally posted on RT will be reposted down a chain of websites until it appears to be an organic article on an American outlet (Nimmo, 2017). Native RT articles are also promoted by legions of fake accounts purporting to be Americans making the same misinformation claims and citing the RT articles as evidence (Davis et al., 2019). State-media outlets often have no overt connection to their host state on their social media channels or their website. For instance, “In The Now” appears like any other short video news service and racks up millions of views but is a subsidiary of RT (O’Sullivan et al., 2019). Even RT itself has attempted to shed state affiliation by rebranding from Russia Today to RT in 2009 (Zuylen-Wood, 2017). One method platforms have chosen to combat these disinformation outlets is transparency labels that accompany their videos or posts to highlight the connection to a state (see Figure 1). The first platform to take this approach was YouTube in 2018, followed by Facebook and Twitter in June and August 2020 respectively (Gold, 2018; Gleicher, 2020; Robertson, 2020).
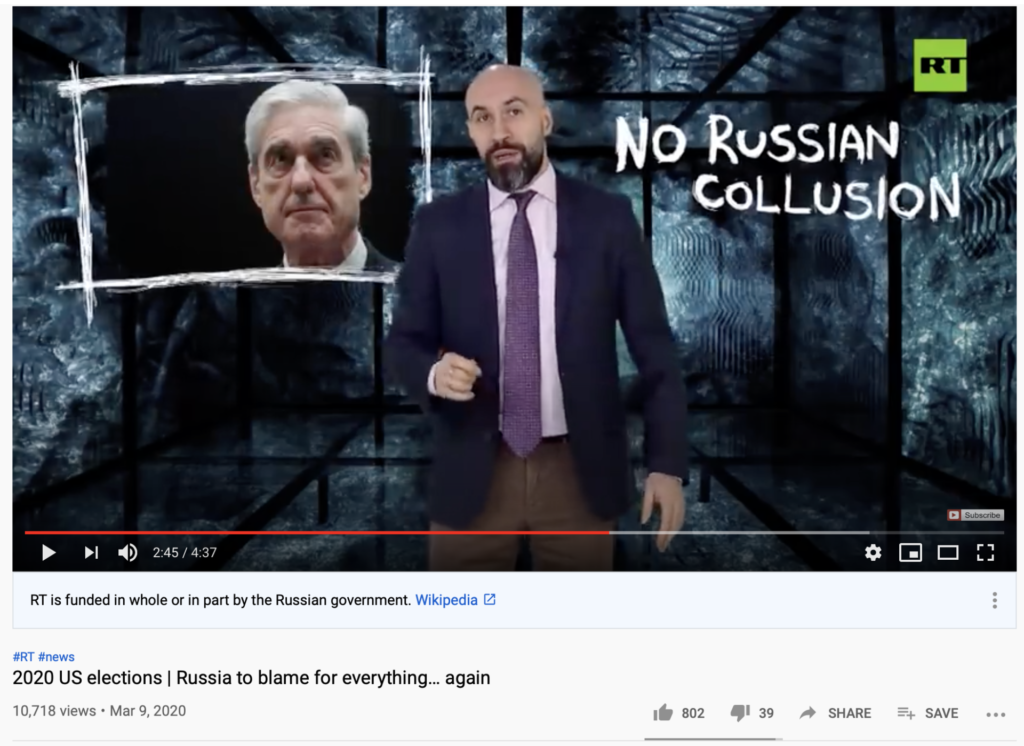
Recent work in correcting misinformation has proven fact-checking can work even in political contexts where opinions are deeply tied to identity (Nyhan et al., 2019). However, this success does not necessarily carry over to the concept of funding labels. The YouTube labels state “[X outlet] is funded in whole or in part by [X] government.” No comment is made directly on the veracity of the information in the video, requiring any labeling effect to be based on viewers’ preconceptions about state-funding for news outlets and the state that is funding it. It therefore functions more in the form of a warning label, aiming to mitigate the impact of exposure to misinformation by affecting trust in the outlet. Warning labels and media literacy interventions have been found to affect perceptions of information accuracy and credibility (Clayton et al., 2019; Hameleers, 2020; Tully et al., 2019). However, these studies generally focused on “media literacy” messages which sought to warn users on the dangers of misinformation broadly. By addressing the ownership behind the outlets, the labels additionally stray into the area of source effects where persuasion is dependent on the credibility of the one attempting to persuade (Druckman, 2001).
Based on our findings the misinformation danger from these outlets is not theoretical. Our study shows RT’s misinformation is effective at shifting the perceptions of viewers. In our first experiment, the video reduced Democrats’ belief in both the accuracy of reports of Russian interference in 2016 and their estimation of its significance. In the second experiment, participants had their faith in mainstream media sources reduced after watching the video without the warning label, where elite media was accused of inventing stories of Russian interference. As one of the only sources of disinformation at the disposal of state actors that is not immediately removed upon discovery, outlets like RT have consistently produced misinformation about the 2020 American election throughout the election cycle.
While the implications if election misinformation is effective are concerning, it also appears that YouTube’s response — state-funding labels — has the potential to mitigate against shifts in perception as a result of misinformation. In our second experiment, when presented with YouTube’s warning label accompanying the RT video, the shift in opinion observed in the unlabeled condition was partially mitigated, shifting back towards the level of trust in the mainstream media held by those who did not view the misinformation. When presented with the same label language superimposed into the video frame instead of under it, we see no shift in opinion as a result of viewing the misinformation. In addition, viewing the labels made participants more concerned about fake news and less trustful of news received through social media or outlets they were not familiar with.
The difference in mitigation between the real label as implemented by YouTube and our superimposed version suggests that the correction effects are dependent on the label being noticed and the information in it being absorbed. The rate at which this happens is strongly dependent on the placement and subtlety of the label. In the time between the first and second experiments, YouTube slightly changed the implementation of their label. The language remained the same, but the color changed from a light grey that blended with the YouTube interface to a more prominent blue. This change alone resulted in a 15-point increase in those reporting having noticed the label and a corresponding increase in those that reported knowing that RT was state funded. However, this rate remains below our version placed in the frame. In the second experiment, with the true disclaimer 51% of respondents answered that RT was state funded compared to 64% of those exposed to the superimposed version.
It is clear that efforts by social media platforms to increase transparency through these labels can be an effective way to increase user knowledge and combat foreign election misinformation. Platforms should not only expand the use of these identification labels, but also increase transparency more broadly when it comes to pages producing misinformation. An informed user is a resilient user. However, when placing a label, platforms must consider its subtlety relative to the interface if they wish for the label to be effective. Facebook’s and Twitter’s state-media labels, for instance, may face difficulty in being noticed in that their small placement and light grey color makes them blend with the page background. However, unlike YouTube’s label, both Facebook and Twitter have placed their labels above the content (see Figure 2) where it may be more readily noticed by users as they scroll. Further research is needed to confirm the efficacy of their labels.
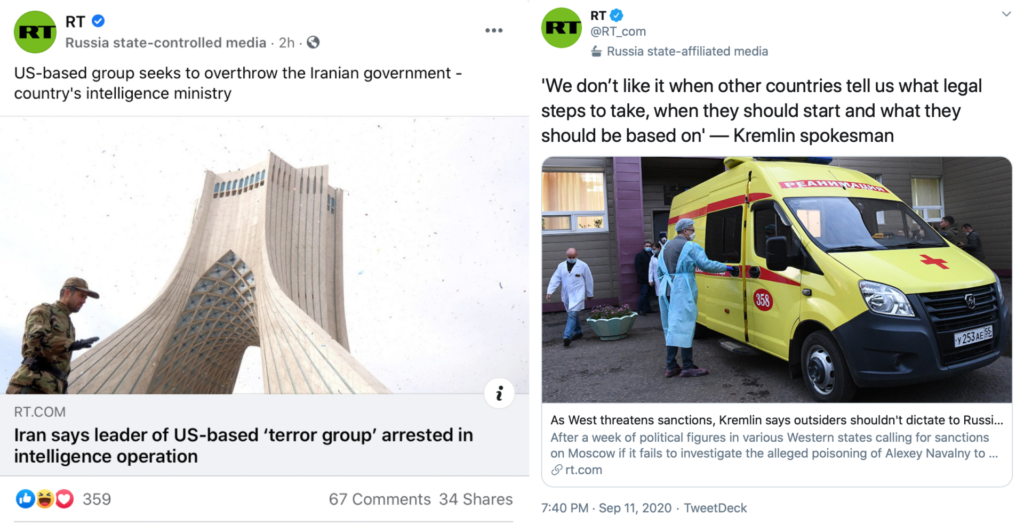
Findings
Finding 1: YouTube’s labels can increase knowledge of state funding, when they are noticed.
YouTube’s state-controlled media labels, which, in the case of RT, state “RT is funded in part or in whole by the Russian government,” have the purpose of informing the user of a media channel’s government connection. Our findings indicate that these labels can be successful, but success depends on their implementation. In our April 2020 experiment, the YouTube label was in a light grey box below the video. In that case, there was no increase in knowledge that RT was state funded between those who saw the real disclaimer and those who saw the video without it. By July 2020, when the second experiment was conducted, YouTube had tweaked the disclaimer to be in a blue, instead of a grey box. This change alone appears to have made the label more effective. In this case, compared to no label, viewing the real YouTube label resulted in a 10-point increase (p < .05) in the percentage of participants that knew RT was state funded. Comparing the real label conditions across studies, we found that YouTube’s change resulted in a significant increase in the proportion who correctly identified RT as state funded (36% compared with 51%, p < .01).
However, even the new YouTube disclaimer only left 51% of participants informed. One reason for this might be that placement below the video can lead the label to be ignored as it is peripheral to the focus of the user’s attention (Chabris & Simmons, 2010). In order to overcome this, we took the language of the disclaimer and superimposed it into the video frame 10 seconds before and after the video. In this way, viewers would be prominently presented with the label before the content they were seeking. In both experiments, this led to a far-greater portion of users reporting knowing RT is a state-funded source, with a 12-point increase from the real label condition (p < .05) in the first experiment, and a 13-point increase in the second (p < .01). Interestingly, the percentage of participants knowing RT is state-funded is distinct from the percentage of participants that reported noticing a label (75% and 71% for the superimposed label in the first and second experiments respectively).2One may argue that 71-75% of participants noticing the superimposed label is a low number given the prominent placement, but we believe that this is in line with the real-world conditions of participants engaging with YouTube in the background while pursuing other tasks, and thus not giving the video their full attention. This is far higher than the 38% and 51% of participants who reported noticing a label in the real YouTube label condition. While more participants reported noticing the label in the superimposed condition, the gap between the proportion who reported noticing a label and who reported knowing RT is state-funded demonstrates that users must not only notice the label, but also absorb and believe the information presented.
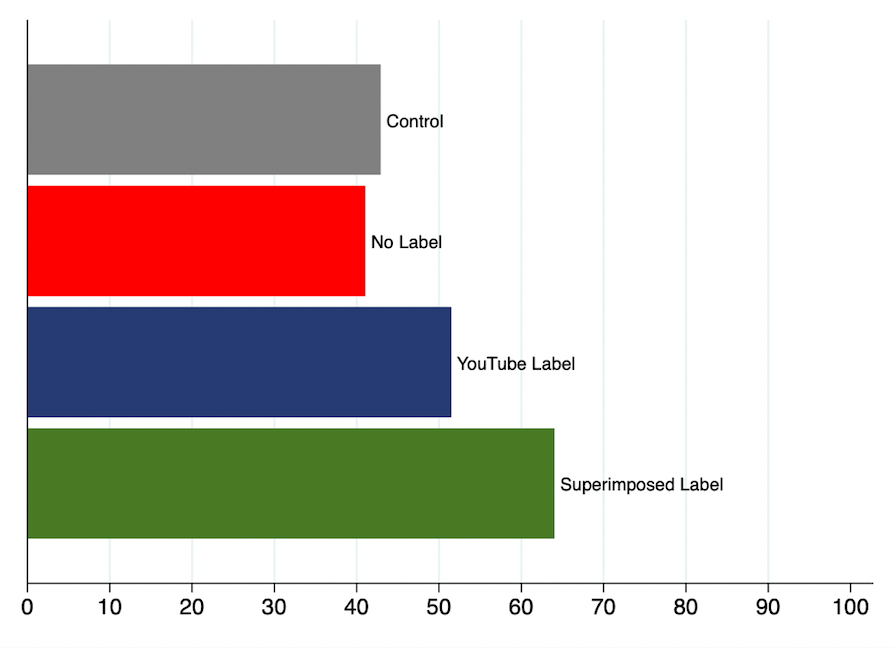
Finding 2: RT’s videos containing election misinformation have the ability to shift the opinions of viewers.
To understand the potential effects of electoral misinformation in the RT videos, we compare a non-political control video with an unlabeled RT video. In the first experiment, the RT video repeatedly dismissed claims that Russia had interfered in the 2016 US presidential election. As compared to the control group, Democrats who watched this video were less likely to believe reports that Russia interfered in the 2016 election (p < .05) and less likely to see that interference as significant (p < .01). The shift in belief being limited to Democrats and Democratic leaning independents may be because Republican belief in Russian interference in the 2016 election was already low. The misinformation did not lessen Republicans’ belief in Russian interference or the significance of that interference because Republicans already did not believe that Russia interfered.
In the second experiment, participants were shown an RT video focusing on the 2020 US election, which claimed that elite media sources were spreading fake news about Russian attempts to interfere. In this case, the misinformation was successful in shifting opinions in reference to the control, and not just for Democrats, but all study participants who saw the RT video without a disclaimer. In this study group, the mean participant had a 5% decrease in trust in mainstream media sources, represented by “news outlets like CNN and NBC.” (p < .05).
Finding 3: State-funding labels have the ability to counteract the effects of misinformation in videos.
By increasing participants’ knowledge of RT’s connection to Russia, the YouTube disclaimer was able to successfully mitigate the shift in opinion created by misinformation in the second experiment. In our July 2020 experiment, participants who were exposed to misinformation claiming that elite media had invented “Russiagate,” were asked their level of trust in mainstream media sources. As noted above, trust was significantly lower among those who saw the video without the label. However, for those that saw the video with the YouTube state-funding label, the decrease in trust was lessened and the misinformation effect was only marginally significant (p = .08, see Figure 4). It was in the condition with the superimposed label that the strongest mitigation of the misinformation effects was seen. Trust in mainstream media among participants who watched the RT video with the superimposed disclaimer was indistinguishable from the control condition.
The labels also increased concern among participants about the dangers of misinformation. In the second experiment, after seeing the superimposed label, participants reported being more concerned that made up news could affect the outcome of an election (p < .05). And, specifically in regard to the 2020 election, participants were more concerned that fake news could endanger it (p = .054). Seeing the real implementation disclaimer also reduced future trust in news received from social media (p < .01) and reduced future trust in news outlets the participant does not frequently encounter (p < .05).
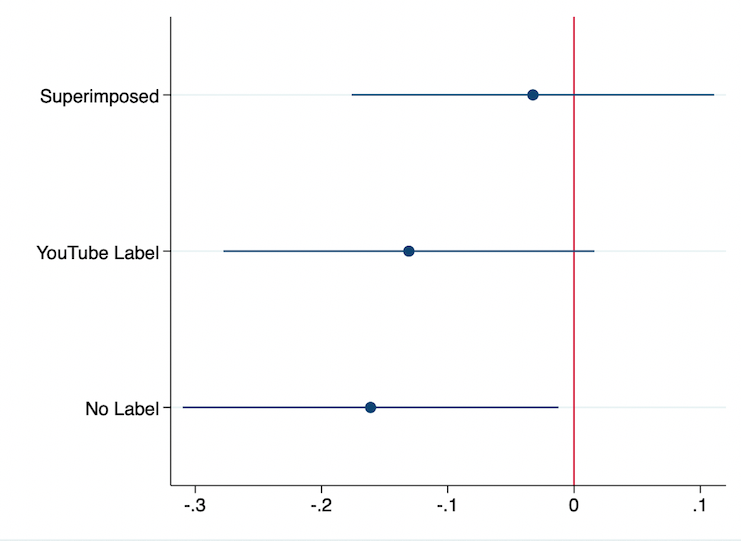
Methods
Data collection
In order to test our research questions, we conducted two experiments, the first of which was conducted on April 11, 2020. We recruited 580 participants from the United States through Amazon’s Mechanical Turk (see Berinsky et al.’s work on its efficacy for social science experimental studies). Participants were randomly assigned to one of four treatment conditions. After answering demographic questions, participants were directed to a YouTube video and then answered questions measuring our key outcomes. The video for the experimental conditions (Figure 5) was a December 2018 news clip from the RT YouTube channel, entitled “$4,700 worth of ‘meddling’: Google questioned over ‘Russian interference.’” The clip covers the CEO of Google testifying before Congress that Russian operatives purchased $4,700 in ads. The majority of the video is commentary from an RT “legal and media analyst” who claims that this testimony undermines the Russia investigation as a whole and that “not one bit of evidence” has been found that Russia affected the election. For participants assigned to see the real YouTube foreign ownership disclaimer, a standard YouTube video link was provided. Upon navigating, participants saw the video with the label directly underneath stating “RT is funded in whole or in part by the Russian government” and a link to the RT Wikipedia page. For participants assigned to see the video without a disclaimer, the video was directly embedded in the survey. Those assigned to see the superimposed disclaimer were also given an embedded version of the video that has an extra 10 seconds cut in at the beginning and end with the disclaimer language. This superimposed condition was based on Pennycook et al.’s (2020) work with Facebook disclaimers. Those assigned to the control condition were given an embedded video produced by AARP3AARP is an interest group that focuses on issues affecting those over fifty, their mission is “to empower people to choose how they live as they age.” entitled “Birdwatching for Beginners with Barbara Hannah Grufferman” which was edited for length to match the RT videos.
The second experiment was conducted on July 25, 2020. Again, we used Mechanical Turk to recruit 1,330 participants. This experiment used the same structure but a different RT video. This video was “2020 US elections | Russia to blame for everything… again.” It focused on creating a narrative that “elites” in the media such as The New York Times were creating fake news about Russia interfering in the 2020 election. The video created an “us vs them” narrative with quotes like “they are elite – you are not” with the goal of undermining trust in mainstream media sources.
Our Mechanical Turk samples have higher educational attainment, are more male, and are younger than the US adult population.
- Experiment 1: 48% identify as Democrat, 28% as Republican and 21% as Independent; 60% have a college or graduate degree; 68% are white; 63% are male; and 27% are between 18-29 years old, 56% between 30-49.
- Experiment 2: 36% identify as Democrat, 44% as Republican, and 18% Independent; 68% have a college or graduate degree, 58% are white; 61% are male; and 21% are between 18-29 years old, 60% between 30-49.
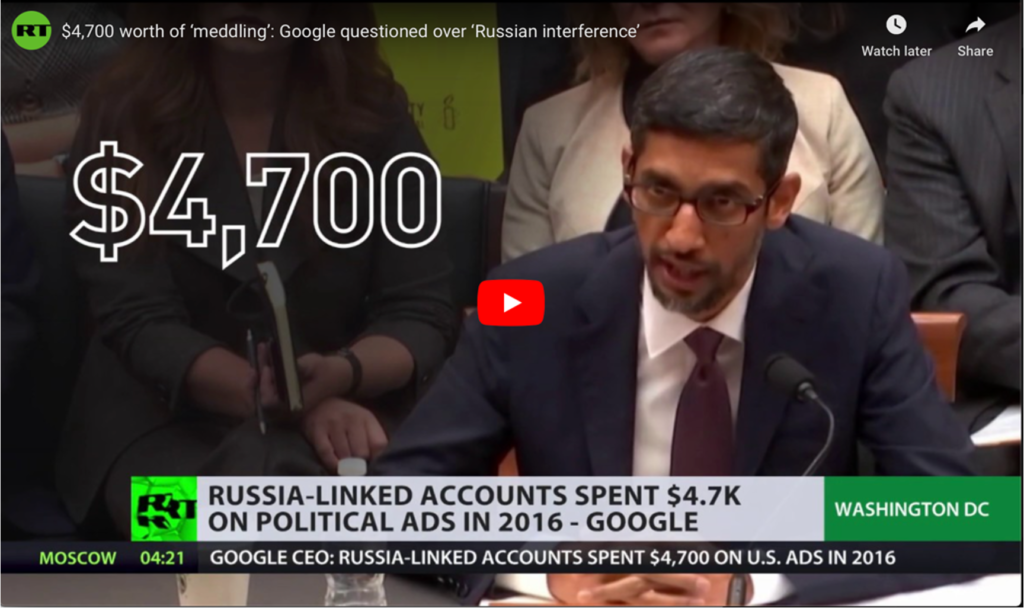
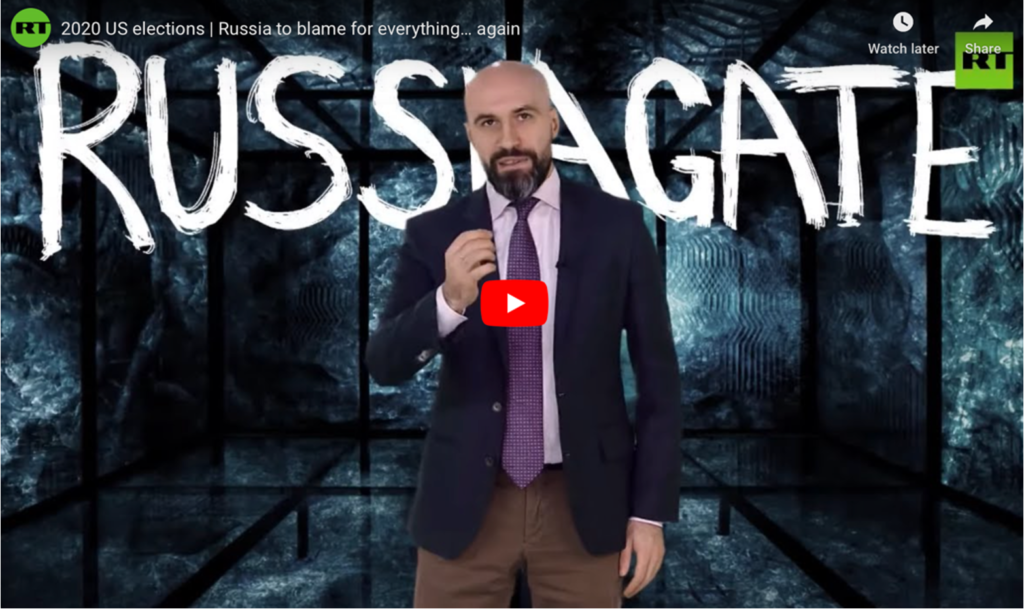
Analysis
We excluded responses submitted in less than 60 seconds, for final ns of 580 and 1,275. Beyond cutting those who finished in less than a minute, a clear indication that they bypassed the survey, we include all participants in the analyses. This was done to simulate real-world conditions where one may only see part of a YouTube video, or listen to it in the background. Using a series of multilinear regressions, we estimated the effects of condition on our key dependent variables. Due to indications that education and party identification were not evenly distributed in the second experiment, they were included as control variables.
Limitations
As with any study employing Mechanical Turk, our study demographics are not directly generalizable to the national population. Our samples are more male, younger and have a higher average level of education compared with the US adult population. Further study with a nationally representative sample would be beneficial.
Further limitations stem from the study design. In order to present the real RT video with the label to some participants and not others, some participants were shown the embedded version of the video where the label is not visible, and some directed to the YouTube environment creating differences in treatment, such as recommended videos being visible on the side. In order to create complete parity, a further study could be conducted with a simulated YouTube environment where the label can be removed at will.
Suggestions for further study
There are a number of questions that the authors were not able to address in this study that merit exploration. Primary among these is the efficacy of state media labels across different platforms. At the time the first experiment in this study was conducted, YouTube was the only major social network using state media labels. At the time of publication, this list has expanded to include Facebook and Twitter. Analysis of the efficacy of these differing implementations of the same concept is necessary. In addition, this study only tested the effects of misinformation from a Russian state outlet. Due to the critical nature of prior attitudes toward the state supporting the outlet in the efficacy of these labels, testing with different state media may provide different results. For this same reason, different tests across political subgroups, with differing prior biases, could be conducted as well. Finally, it would be beneficial to study the efficacy of the warning labels outside of the context of an election. RT and other state media sources broadcast disinformation concerning numerous topics that viewers may be primed to react to differently than election coverage.
Topics
- Disinformation
- / Politics
- / Russia
- / Youtube
Bibliography
Berinsky, A. J., Huber, G. A., & Lenz, G. S. (2012). Evaluating online labor markets for experimental research: Amazon.com’s Mechanical Turk. Political Analysis, 20(3), 351–368. https://doi.org/10.1093/pan/mpr057
Chabris, C. F., & Simons, D. (2010). The invisible gorilla: And other ways our intuitions deceive us (1st edition). Harmony.
Clayton, K., Blair, S., Busam, J. A., Forstner, S., Glance, J., Green, G., Kawata, A., Kovvuri, A., Martin, J., Morgan, E., Sandhu, M., Sang, R., Scholz-Bright, R., Welch, A. T., Wolff, A. G., Zhou, A., & Nyhan, B. (2019). Real solutions for fake news? Measuring the effectiveness of general warnings and fact-check tags in reducing belief in false stories on social media. Political Behavior. https://doi.org/10.1007/s11109-019-09533-0
Davis, T., Livingston, S., & Nassetta, J. (2019, March 29). Hybrid media framing: Understanding perpetrator attempts to undermine accountability. ISA Annual Conference, Toronto.
Druckman, J. N. (2001). On the limits of framing effects: Who can frame? The Journal of Politics, 63(4), 1041–1066. https://doi.org/10.1111/0022-3816.00100
Elswah, M., & Howard, P. N. (2020). “Anything that Causes Chaos”: The organizational behavior of Russia Today (RT). Journal of Communication, 70(5), 623–645. https://doi.org/10.1093/joc/jqaa027
Gleicher, N. (2018, December 6). Coordinated inauthentic behavior explained. About Facebook. https://about.fb.com/news/2018/12/inside-feed-coordinated-inauthentic-behavior/
Gleicher, N. (2020, June 4). Labeling state-controlled media on Facebook. Facebook Newsroom. https://about.fb.com/news/2020/06/labeling-state-controlled-media/
Gold, H. (2018, February 2). YouTube to start labeling videos posted by state-funded media. CNNMoney. https://money.cnn.com/2018/02/02/media/youtube-state-funded-media-label/index.html
Hameleers, M. (2020). Separating truth from lies: Comparing the effects of news media literacy interventions and fact-checkers in response to political misinformation in the US and Netherlands. Information, Communication & Society. https://doi.org/10.1080/1369118X.2020.1764603
Ioffe, J. (2010). What is Russia Today? Columbia Journalism Review. https://www.cjr.org/feature/what_is_russia_today.php
Nimmo, B. (2017, December 26). How the alt-right brought #SyriaHoax to America. DfrLab. https://medium.com/dfrlab/how-the-alt-right-brought-syriahoax-to-america-47745118d1c9
Nyhan, B., Porter, E., Reifler, J., & Wood, T. J. (2020). Taking fact-checks literally but not seriously? The effects of journalistic fact-checking on factual beliefs and candidate favorability. Political Behavior, 42 939-960. https://doi.org/10.1007/s11109-019-09528-x
O’Sullivan, D., Griffin, D., Devine, C., & Shubert, A. (2019, February 18). Russia is backing a viral video company aimed at American millennials. CNN. https://www.cnn.com/2019/02/15/tech/russia-facebook-viral-videos/index.html
Pennycook, G., Bear, A., Collins, E., & Rand, D. G. (2020). The implied truth effect: Attaching warnings to a subset of fake news headlines increases perceived accuracy of headlines without warnings. Management Science. https://doi.org/10.1287/mnsc.2019.3478
Robertson, A. (2020, August 6). Twitter will label government officials and state-affiliated media accounts. The Verge. https://www.theverge.com/2020/8/6/21357287/twitter-government-officials-state-affiliated-media-labels-algorithm
Tully, M., Vraga, E. K., & Bode, L. (2020). Designing and testing news literacy messages for social media. Mass Communication and Society, 23(1), 22–46. https://doi.org/10.1080/15205436.2019.1604970
Zuylen-Wood, S. van. (2017, May 4). At RT, news breaks you. Bloomberg Businessweek. https://www.bloomberg.com/features/2017-rt-media/
Funding
This research was funded by the School of Media and Public Affairs and the Columbian College of Arts and Sciences at The George Washington University.
Competing Interests
The authors have no competing interests.
Ethics
This study was approved by the Institutional Review Board at The George Washington University. Participants provided informed consent before participating.
Copyright
This is an open access article distributed under the terms of the Creative Commons Attribution License, which permits unrestricted use, distribution, and reproduction in any medium, provided that the original author and source are properly credited.
Data Availability
All materials needed to replicate this study are available via the Harvard Dataverse: https://doi.org/10.7910/DVN/BTLAGG