Peer Reviewed
Review of social science research on the impact of countermeasures against influence operations
Article Metrics
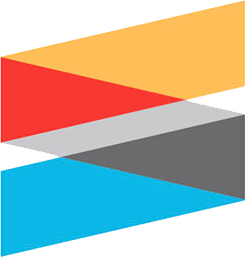
12
CrossRef Citations
Altmetric Score
PDF Downloads
Page Views
Despite ongoing discussion of the need for increased regulation and oversight of social media, as well as debate over the extent to which the platforms themselves should be responsible for containing misinformation, there is little consensus on which interventions work to address the problem of influence operations and disinformation campaigns. To provide policymakers and scholars a baseline on academic evidence about the efficacy of countermeasures, the Empirical Studies of Conflict Project conducted a systematic review of research articles that aimed to estimate the impact of interventions that could reduce the impact of misinformation.
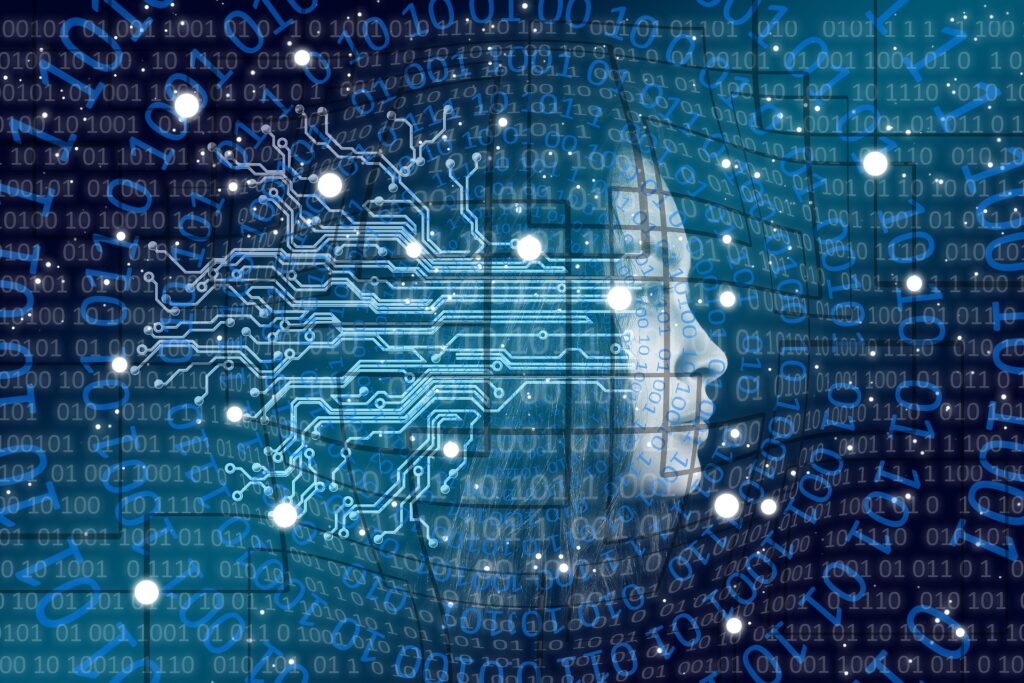
Research Questions
- What is the current state of the empirical literature on the impact of countermeasures against influence operations?
- Which countermeasures are most likely to be effective in mitigating the impact and/or spread of influence operations, and what design aspects matter?
- What research gaps exist in the literature on the impact of countermeasures?
Essay Summary
- Based on key term searches and forward and backward citation mapping, we constructed a review of 223 studies published since 1972 related to countermeasures designed to combat influence operations. Each identified study included: (1) a source of variation in exposure to countermeasures; (2) a clearly defined outcome of interest for some specified population; (3) relevance to thinking about the potential of an intervention to impact real-world behavior; and (4) enough detail to evaluate the credibility of the findings. This approach amounts to sampling the foundational research surrounding countermeasures and thus incorporates the collective judgement of this emerging field.
- All of the studies we identified examined user-focused countermeasures, i.e., those aimed at the consumers of disinformation. None looked at countermeasures aimed at impacting the influence operations directly.
- There exists a mismatch between the major interventions taken by platforms—algorithmic downranking, content moderation, redirection, and deplatforming accounts—and those studied by the research community. Most papers we reviewed focus on one particular method for countering information operations: fact-checking and its many offshoots. The types of interventions employed by social media companies on actual users are understudied.
- We recommend further research on four key areas: (1) measuring the impact of the most common interventions by social media platforms, (2) assessing the impact of countermeasures on real-world behaviors (both online and offline), (3) evaluating the efficacy of countermeasures in non-Western contexts, and (4) studying countermeasures that target the creators of disinformation content in addition to studying consumer-facing policies.
Introduction
On March 25, 2021, CEOs of the world’s largest social media platforms, Facebook, Twitter, and Google, once again testified in front of U.S. Congress on their efforts to curtail the spread of disinformation and misinformation online. Despite ongoing discussion of the need for increased regulation and oversight and debate over the extent to which the platforms themselves should be responsible for containing misinformation, there is no consensus on what should be done to address the problem. The most common policy recommendations for countering influence operations include increased data- and information-sharing, fact-checking and increased platform regulation (Yadav, 2020).
To provide scholars and policymakers with a baseline on academic evidence about the efficacy of countermeasures against influence operations, the Empirical Studies of Conflict Project conducted a systematic review of research articles that aimed to estimate the effect of interventions that could reduce the impact of misinformation.
In line with other research trends in the broader field of influence operations and disinformation, we find that there has been a dramatic increase in the number of studies since 2016. In fact, the majority of studies (62%) we cite have been published since 2019. This recent research complements an existing body of psychological research on mitigating the effects of exposure to false information (Johnson & Seifert, 1994; Wilkes & Leatherbarrow, 1988), including exposure to corrective advertising via traditional mass media (Dyer & Kuehl, 1978), the role of a source’s trustworthiness and expertise in determining how individuals feel about information (McGinnies & Ward, 1980), the effect of content labeling the veracity of claims about consumer products (Skurnik et al., 2005), and the impact of providing corrections to medical misinformation about the Affordable Care Act (Nyhan et al., 2013).
Overall, both the older literature and new work on social media suggest that fact-checking can reduce the impact of exposure to false information on individuals’ beliefs, at least on average, as well as their propensity to share dis/misinformation. Despite this consensus, there are significant gaps. First, there are very few studies on populations outside of the U.S. and Europe, although experimental interventions designed to counter misinformation could be replicated in other regions. Second, the literature provides little evidence regarding the impact of countermeasures delivered in real-world settings. The vast majority of studies occur in the context of lab or survey experiments, though that is beginning to change. Third, the literature provides little evidence on the efficacy of fact-checking on real-world behaviors, i.e., whether those exposed to fact checks choose different actions on subjects about which they have seen misinformation than those who do not.
Beyond fact-checking, the research base is very thin. We found few high-credibility studies which evaluated the key strategies employed by social media platforms to combat influence operations, including: targeted removal of accounts, notifying users of interactions with fake accounts or disinformation, changes to platform monetization policies that reduce the profitability of disinformation, algorithmically-assisted content moderation, and behavioral nudges away from misinformation. Additionally, all identified studies focused on the consumers of disinformation. We did not find any studies that systematically examined the impact of countermeasures targeting the supply side of disinformation. The lack of research on the impact of supply-side countermeasures is worrisome, though understandable given the difficulty of measuring supply-side behavior in this space.
Findings
We identified 223 studies published since 1972 which met our inclusion criteria and focused on various countermeasures designed to counter influence operations. Seed studies were identified based on key term searches (see Methods section for the full list of key terms), followed by backward citation mapping (reviewing sources referenced by included studies) and forward citation mapping (reviewing research which cites included studies).1This approach will miss relevant studies which did not match our seed search terms only if they were not considered significant enough to cite by the studies which did match the seed terms. This approach amounts to sampling the foundational research surrounding countermeasures as viewed by those publishing in the field. It is intended to reflect the collective judgement of this emerging field about what the relevant literature is.
As with many areas of social science, a core challenge for this literature is separating correlations which are evidence of causal relationships from those which are not. We focused our review on studies whose methodology provides evidence regarding causal relationships. We define causal relationships as those in which an increase or decrease in some feature of the world which we call the treatment (e.g., exposure to a form of fact-check) would lead to a change in some other feature of the world which we call the outcome (e.g., belief in misinformation). In order to provide evidence of causal relationships, such studies require a source of variation in exposure to treatment which is unrelated to expected outcomes.
The majority of the studies, 87%, randomized assignment into different treatment conditions (with random assignment guaranteeing that any resulting correlation between treatment and outcome represents the causal effect of the treatment on the outcome). Two-thirds of these, 64% of all studies, presented interventions in an artificial setting such as a lab or online survey (e.g., fact checks displayed in different ways), what we call “experimental” research. The remainder of these randomized trials, 23% of all studies, involved simulated social media in which respondents were randomized into seeing different kinds of content in a setting that aimed to mimic real-world social media. The overwhelming majority of studies that looked at social media, 93%, did so in the form of a simulated social media feed or using post-styled presentation of disinformation content. 4% of the studies examined the impact of variation in real-world media, mostly fact-checks of disputed claims, many of which were also presented in the context of a survey experiment. 7% of studies examined the impact of variation in exposure to real-world events, and 2% looked at the consequences of changes in exposure to real-world social media.
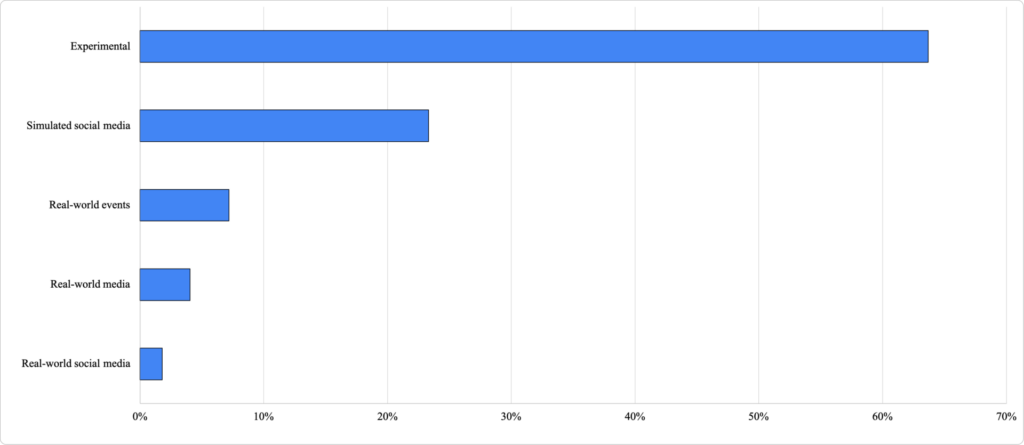
We also categorized studies depending on the type of outcome involved (see Methods for descriptions of outcome types). A plurality of studies primarily measured the impact of interventions on beliefs (42%). Roughly 27% examined self-reported intended behavior, and 23% evaluated participant knowledge. Only a small fraction looked at how interventions mitigated the impact of disinformation on real-world behavior (6%) or online behavior (2%).
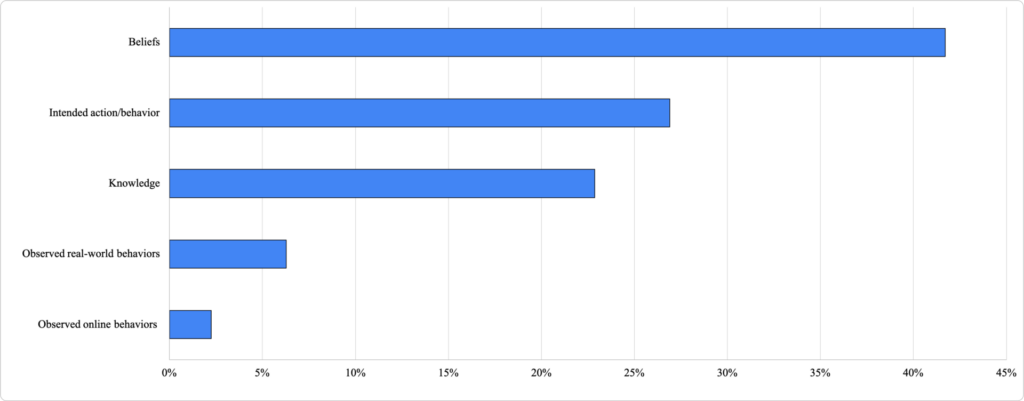
Of 223 studies, 167 (75%) evaluated the impact of disinformation disclosure, 67 (30%) studied content labeling, 24 (11%) examined disinformation literacy, one evaluated content distribution/sharing, and one content reporting.2See Methods section for definitions of countermeasure types. These categories were not mutually exclusive. Many studies of disinformation disclosure also included variations in how content was labeled. We note that most forms of fact-checking fall under the disinformation disclosure category, though some fit best under content labeling. 48 studies looked at multiple forms of interventions, mostly a combination of disinformation disclosure and content labeling. Ten studies examined countermeasures that did not fit within the existing set of platform interventions.3These included evaluating: how the presentation (text versus media) and source of disinformation impacted perceptions of its credibility; whether initial priming about evaluating the accuracy of information impacted user ability to better discern disinformation; if legislators were less likely to produce disinformation in their public statements when presented with information about the reputational risks of negative fact-checking ratings; how differences in judgement processes impact whether individuals will update false beliefs after being presented with corrective information, differences in effectiveness of various accuracy prompt intervention approaches; the difference in effectiveness of crowdsourced versus academic corrections to false information; the effectiveness of audience-targeted communication strategies for disinformation disclosure; and the roles of inattention emotionality and deliberation in influencing accurate identification of disinformation. Critically, deplatforming, the most prominent countermeasure employed by social media platforms, was not studied by any of the research articles included in this review.
This focus reflects the most common approaches taken by civil society organizations working against disinformation and influence operations, which include fact-checking and information verification (Bradshaw & Neudert, 2021). Figure 3 provides a comparison of the types of interventions taken by social media platforms broken down by percentage of total and compared to the intervention’s representation in the research literature.4Categories were developed by the Partnership for Countering Influence Operations at the Carnegie Endowment, in Yadav (2021). See coding description in Methods section. There is a clear mismatch between the share of methods employed by platforms and studied interventions, which are almost always disinformation disclosure and content labeling (Yadav, 2021).
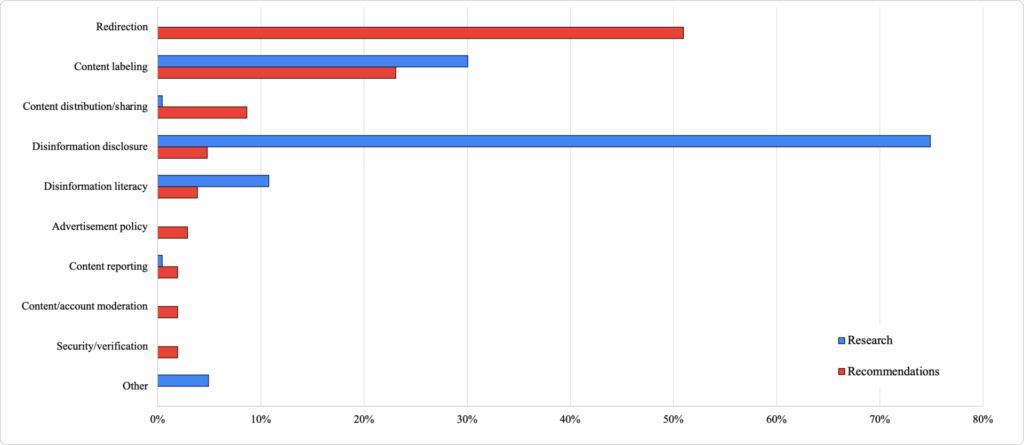
Importantly, all of the studies we identified for this review focused on user-targeted countermeasures (i.e., the consumers of disinformation). None looked at countermeasures aimed at impacting the influence operations directly (i.e., suppliers of disinformation); this would include interventions such as advertising policies, security and verification at the account level, and long-term moderation at the account level (of which deplatforming is the most extreme example).
Of 223 studies, 56 (25%) dealt directly with disinformation on social media platforms. The majority of these (52) involved creating a simulation of a social media feed or showing participants disinformation in a platform post format (e.g., as a Tweet or Facebook post). Only four studies sought to directly evaluate the impact of interventions on real-world social media usage. Bowles et al. (2020) examine the impact of exposure to WhatsApp messages with information about COVID-19 or debunking COVID-19 misinformation. Nassetta & Gross (2020) evaluate the impact of placing state-sponsored media labels and disclaimers below RT YouTube videos on participant perceptions. Bor et al. (2020) measured how much fake news and disinformation was shared on the Twitter feeds of users after exposure to fact-checking information videos. Mosleh et al. (2021) measured the impact of fact-checking by human-looking bot accounts on the subsequent sharing behavior of Twitter users.
The vast majority of studies utilized U.S.-based populations. Additional countries studied include Australia, Bulgaria, Brazil, Canada, Denmark, France, Germany, India, Israel, the Netherlands, Poland, Sweden, Ukraine, South Korea, the U.K., and Zimbabwe. Systematically examining differences in fact-checking efficacy across these countries is not yet possible because core findings have not been widely replicated in similar experiments across countries.
Finally, the research base suggests that design can play a key role in mediating the efficacy of fact-checking insofar as the presentation of interventions appears to impact effectiveness. Young et al. (2017), for example, finds that “video formats demonstrated significantly greater belief correction than [a]… long-form Factcheck.org article.” Nassetta & Gross (2020) found that YouTube’s media label became more effective by simply changing the color. Bor et al. (2020) show that while exposure to fact-checking videos “improved participants’ ability to assess the credibility of news story headlines,” they continued to share “false and untrustworthy news sources on Twitter.” The importance of the medium also applied to disinformation itself: Hameleers et al. (2020) found that “multimodal disinformation was perceived as slightly more credible than textual disinformation” and that “the presence of textual and visual fact checkers resulted in lower levels of credibility.” Ternovski et al. (2021) found that the impact of warning labels on textual disinformation also applied to video clips (including deep fakes), but that the label “increase(s) disbelief in accompanying video clips—regardless of whether the video is fake or real. This is particularly problematic as the warnings driving this effect are vague.”
Across all the studies which engaged in disinformation disclosure or content labeling of some kind: 133 studies indicated that they reduced false beliefs. Given recent interest in accuracy nudges, partisan labels, and source credibility rankings, it is worth noting that three studies induce subjects to think about accuracy, and all three indicate that doing so either changes beliefs or sharing behavior. One study provides information on partisan bias impacting disinformation disclosure, but it had an unclear effect on reducing false beliefs. Eight studies focus on source credibility. We found only one that provides evidence on the impact of countermeasures on subsequent real-world behavior, and one that relies on a survey-based method to elicit information on the behavior—it does not measure the behavior directly.
The research community’s focus on fact-checking’s impacts on belief, knowledge, and intentions likely reflects both researcher preferences and choices by companies. On the researcher side experiments on fact-checking are straightforward to carry out, predictably publishable, and fit well in the disciplinary norms of relevant fields (i.e., behavioral psychology and communications). Studies which measure real-world outcomes are more challenging to execute and it is very hard to identify which populations were treated when.
On the company side, platforms rarely disclose the specifics of algorithmic measures aimed at targeting dis/misinformation on their platforms, much less sharing sufficient detail about how those measures were rolled out (including variance geographically) to enable reliable inference about their causal impact. And many remove content from both the live websites and research APIs (e.g., Facebook and Twitter), meaning it is hard for researchers to retroactively figure out who was exposed to the content and thus who might be impacted by its removal or by algorithm changes.
While both of these company-level gaps could be rectified by platforms, academics can work around them. Public announcements of platform policy initiatives and content/account removals provide the information needed to measure short-run changes due to new policies using time-series techniques.5Facebook and Twitter have both published data on accounts they removed for violating policies on information operations, although only Twitter’s data include the raw content. And for platforms that take action on publicly visible content (such as Facebook and YouTube), continuous monitoring of content enables observation of when it is removed, which can be used to measure changes in engagement/discussions.6Mitts et al. (2021) study the impact of terrorist propaganda videos by following ISIS-related discussions, identify posting dates of videos, and then study changes in the activity of those exposed to the videos compared to those not exposed. The same approach could be taken for account removals, but one would need to be following the relevant community before the accounts were removed or be able to identify them after the fact and know the date of removal. Methods such as redirection or targeting content distribution/sharing are clearly understudied relative to their prominence.7For an exception, see Saltman et al. (2021). More studies on the underlying causal mechanisms/effectiveness of these strategies, even in an artificial lab setting, would help further our understanding.
Key takeaways
Takeaway 1: Some interventions definitely work.
In reviewing the field of research, several core findings emerge:
- Fact-checking can reduce the impact of misinformation on beliefs, although studies find varying degrees of efficacy depending on the type of misinformation and intervention.
For decades, researchers have documented the impact of fact-checking and analogous forms of corrective information on altering target beliefs. Corrective advertisements mandated by the Federal Trade Commission (FTC) in the 1970s helped to alter beliefs and purchasing intentions around misrepresented products (Bernhardt et al., 1986). Contemporary fact checks of the form used widely on social media platforms have been shown to induce resilient belief updating, particularly when accurate information is repeated (Carnahan et al., 2020) and when fact checks provide an alternative account to misinformation (Nyhan and Reifler 2015). A group of studies has identified a “continued influence effect” (CIE) whereby corrected facts linger in memory and continue to shape how people interpret events (e.g., Ecker et al., 2010; O’Rear & Radvansky, 2020). While some papers have found that partisan, ideologically congruent misinformation is particularly resistant to change (i.e., Nyhan & Reifler, 2010; Walter & Salovich, 2021), others did not identify a partisan effect (Nyhan & Reifler, 2016). In general, while fact-checks do not appear to eliminate CIE, they do reduce its intensity (Gordon et al., 2019).
Two contrasting sets of studies focus on the “backfire effect,” in which “corrections actually increase misperceptions among the group in question” (Nyhan & Reifler, 2010). Some researchers have replicated the backfire effect, particularly in the context of health myths and vaccine misinformation (Peter & Koch, 2016; Pluviano et al., 2017). Nyhan et al. (2014) found that for certain groups, pro-vaccination messaging actually decreased intention to vaccinate. Still, an extensive body of literature has found that “the backfire effect is stubbornly difficult to induce,” even when “testing precisely the kinds of polarized issues where backfire should be expected” (Wood & Porter, 2019).
While the fact-checking literature also focuses primarily on the alteration of beliefs and knowledge, beliefs are not necessarily indicative of political preferences or social media behavior. Nyhan et al. (2019) and Swire-Thompson et al. (2020), for example, both find that while fact-checks of politicians’ false claims successfully reduce beliefs in the claims, they do not impact support for the politician. With respect to intended sharing behavior, Bor et al. (2020) and Pennycook et al. (2020) found that identification of fake news may not prevent sharing on Twitter. Such outcomes are significant in designing effective fact-checking interventions.
- Inducing people to think about accuracy or inoculating against fake news can reduce misinformation sharing.
Over the past few years, a growing number of studies have tested interventions designed to preemptively combat the impacts of misinformation. Andı & Akesson (2021) identified a significant impact of social norm-based nudges on sharing intentions, as did Pennycooket al. (2020) with accuracy-oriented messaging. Numerous studies tested forms of inoculation, explaining the “flawed argumentation technique used in the misinformation” (Cook et al., 2017) or highlighting the scientific consensus surrounding climate change (Maertens et al., 2020). Playing games to enhance misinformation identification skills can have a similar effect (Roozenbeek et al., 2020).
- Providing information on the partisan bias or trustworthiness of sources is more important than specifying source credibility in mediating the impact of misinformation.
One form of intervention designed to combat misinformation focuses on providing source information to viewers. Across a number of studies, manipulating source trustworthiness was more impactful than knowledge of source credibility (i.e., Ecker & Antonio, 2021; Pluviano & Della Sala, 2020). In some cases, emphasizing publisher information had little to no effect on accuracy ratings (Dias et al., 2020; Wintersieck et al., 2018). One outlier is A. Kim et al. (2019), which found that source ratings impacted believability and even made participants skeptical of unrated sources. J. W. Kim (2019) also found a significant impact of source expertise on attitudes about an anti-vaccination rumor. In general, the literature surrounding vaccine misinformation and countermeasures produces unique results.
Takeaway 2: Fact-checking is overstudied relative to its centrality in platforms’ regulatory toolkits.
Most of the research is looking at one particular method for countering information operations: fact-checking and its many offshoots. The bulk of the literature points to the idea that fact-checking can effectively reduce the impact of misinformation on individual factual beliefs and social media sharing intentions in the short term (though not necessarily ideological beliefs). The literature is also promising on the efficacy of warning people about misinformation before they see it (also known as prebunking), media literacy training, and crowdsourcing the identification of misinformation. But there is little work on the effects of interventions such as removing fake accounts or changing monetization policies, and few studies look beyond misinformation spread on Facebook, Twitter, or media outlets.
Takeaway 3: There exists a significant mismatch between interventions taken by platforms and those studied by the research community.
The types of interventions employed by social media companies on actual users are understudied. Dias et al. (2020) pointed out that neither “Facebook nor YouTube has released data about the effectiveness of their source-based interventions, and the existing academic literature is inconclusive.” Further, literature has done little to study the platforms’ major actions. Only one study directly measures the impact of platform interventions on real-time social media behavior (Bor et al., 2020) and few studies (2%) sought to measure the impact of interventions on online behavior broadly. This was achieved by having participants disclose their Twitter usernames and asking that their feeds remain public in order for the research team to engage in data scraping over a period of one year. No study included in our review relied on access to data from a social media platform. There is an important opportunity for platforms to collaborate with academics because the fact that social media data are so rich and observed with high frequency means there are a range of statistical approaches which can be used to understand the causal impact of their interventions.
Takeaway 4: Most countermeasures and forms of intervention have yet to be studied.
Almost all included cases studied the efficacy of fact-checking in some capacity, and some studied the effect of emphasizing source, “pre-bunking” misinformation, or inoculating against misinformation via news literacy messages and games. No identified studies looked at such interventions as removing accounts, notifying users of interacting with fake accounts, or changing monetization policies. And no studies examined countermeasures targeting creators of disinformation content.
Takeaway 5: A limited population set has been studied.
The overwhelming majority of studies involved participants from the United States; at least 106 studies explicitly involved U.S.-based populations. In addition, a pre-analysis study plan pointed out that almost all research on fake news has focused on western democracies (Rosenzweig et al., 2020). Yet, cultural differences appear to matter. Swire-Thompson et al. (2020) replicated a study on American and Australian voters and found significantly different effect sizes, indicating that cultural context may impact the efficacy of social media interventions. Additionally, the majority of studies recruited participants from universities (67 studies) or used Amazon’s Mechanical Turk (72 studies), a crowd-sourcing platform that can also be used to administer research surveys. Greater attention should be paid to moving some of those studies to representative populations in multiple countries.
Recommendations
In reviewing the literature discussed above, we learned that the field has not yet addressed five key questions. We summarize them and offer suggestions for how to address them.
- Do social media platform interventions actually work? Only four studies sought to examine specific platform policies. There is a particular need for studies examining the impacts of deplatforming and redirection. The academic literature should begin moving away from its focus on fact-checking, which can clearly work on average. But this shift will be significantly easier with greater cooperation between social media platforms and the academic community.
- How do countermeasures affect online behaviors? Few studies examined the impact of interventions on actual online behavior. In some ways this is surprising. Opt-in field experiments on user-level interventions appear feasible and could readily be conducted with platform cooperation. And even without that, opt-in studies in which respondents are randomized into receiving different kinds of information would enable assessments of real-world impact. Compensating subjects for participation could reduce self-selection concerns, as it has in other areas of social research.
- How might cultural factors influence the effectiveness of countermeasures? The literature is largely limited to interventions applied to Western populations, though there are important exceptions (e.g., Bowles et al., 2020). We don’t have a clear sense of how countermeasures vary in effectiveness as a reflection of cultural differences, though replicating many existing experimental studies on non-Western populations is feasible.
- What happens in the tails? Almost all the studies we found examined average effects, e.g., delivering a fact-check reduced beliefs in statement X by Y percent. Such statistical approaches tell us little about how the fact-checks influence those at different points in the belief distribution before they received the fact-check. This is important as the worst outcomes of misinformation exposure, such as participation in events like the January 6 attack on the U.S. Capitol, tend to be perpetrated by those with extreme beliefs. Future studies should consider measuring beliefs before any fact-check is provided and then looking for differences in treatment effects at different points in the belief distribution.
- What is the impact of countermeasures on real-world behaviors? The policy community needs more studies on real-world behaviors which could be influenced by misinformation countermeasures. Public health is an obvious place for such studies as the outcomes which are impacted by misinformation are readily measurable (e.g., compliance with stay-at-home orders can be measured through cell phone metadata, and vaccination status is verifiable). The key for such studies will be getting better information on when countermeasures were rolled out and to which populations. Deplatforming of those promoting public health misinformation is one promising avenue. A feasible design would be to see if the vaccination propensity of people who followed key anti-vax influencers who have been deplatformed went up after the deplatforming compared to that of people who followed active influencers.
We end by noting that our study has clear implications for how policymakers should respond to the academic literature. First, and foremost, the evidence in favor of fact-checking is strong. This suggests governments should find ways to support civil society efforts to make fact-checks more readily accessible. Second, a key challenge in understanding countermeasures is lack of information on the treatments. Policymakers should enact regulations requiring transparency about platform changes, perhaps in secure environments to prevent malign actors from exploiting the information, to enable the academic literature to better reflect what is actually taking place on the platform. And since we currently lack a robust research base for understanding the effectiveness of most countermeasures, policymakers should create incentives for research on countermeasures.8The U.S. National Science Foundation’s recent Convergence Accelerator grant on Trust and Authenticity in Communication Systems is an encouraging move in this direction.
Methods
We included 223 studies in our review, with an initial set of articles drawn from the bibliography of Martin et al. (2020) and keyword searches on the following set of terms using both Google Scholar and the Princeton University Library catalogue:
- Account removal
- “Account removal” social media
- Algorithmic deranking / algorithmic de-ranking
- Algorithmic downranking / algorithmic down-ranking
- Content moderation
- Countering fake news
- Countering misinformation
- Correcting fake news
- Correcting misinformation
- Demonetization social media
- Deplatforming/deplatform
- Intervention countering misinformation
- Regulation and w/15 social media
- Shadow ban
We conducted two stages of forward and backward citation mapping based on this initial list (e.g., for backward mapping we checked the pieces each article cited, as well as the ones cited by those articles).
We included studies that met four inclusion criteria. First, the study must have a source of variation in exposure to countermeasures, what we will call the treatment, to create a contrast between those who experienced it (e.g., saw a fact-check), and those who did not. Second, the outcome of interest must be clearly defined and measured for some clearly specified population (e.g., sharing content by a sample of people recruited on M-Turk). Third, the study must be relevant to thinking about the potential of an intervention to impact real-world behavior. Fourth, the study procedures must be described in enough detail to enable evaluation of the credibility of the findings. We categorize each studied countermeasure according to treatment type, outcome type, and type of intervention.
For treatment types we recorded the source of variation in exposure to both the disinformation content and the associated countermeasure in five categories:
- Experimental: Used when the variation in subjects’ exposure to countermeasures occurred as a result of a manipulation by the researcher in the context of a laboratory or survey experiment unless efforts were made to present the countermeasure in a realistic setting.
- Real-world events: Used for studies where the variation in subjects’ exposure occurs as a result of interactions with information about an event or debate that took place in the real world, such as a political rally or discussion over immigration.
- Real-world media: Used when variation in subjects’ exposure occurred as a result of interactions with news media content on mediums such as television, newspapers, and the radio.
- Real-world social media: Used when the variation in subjects’ exposure occurred as a result of interactions with social media content on platforms such as Facebook, Twitter, and WhatsApp.
- Simulated social media: Used when the variation in subjects’ exposure occurred as a result of manipulation by the researcher, with information presented in an artificial social media environment, such as using the formatting of a standard social media post or via a simulated social media feed. We coded simulated social media as a separate category, but it could be considered a subcategory of ‘Experimental’. We coded this as a separate category to highlight the fact that the vast majority of studies on countermeasures relating to social media present interventions in settings very different from actual social media platforms. The goal was to highlight a key gap in methodological approaches to studying countermeasures.
Outcomes were categorized into observed behaviors and three outcomes measured through survey questions: intended behaviors, beliefs, and factual knowledge. Experiments that measured both actions and beliefs were coded according to the behavioral outcome. These outcomes were defined as follows:
- Beliefs: The outcome captures a change in the subjects’ beliefs, emotions, or views of the world. It is measured through survey instruments asking subjects questions about their opinions on a range of topics, including their political views, vaccination beliefs, anti-foreign sentiment, perceptions of credibility, and so on.
- Intended actions or behaviors: The outcome captures a change in the subjects’ intentions or their willingness to behave in a particular way in the future. It is measured through self-expression intentions or plans on survey instruments, including subjects’ intent to vaccinate and their intended vote choice.
- Observed real-world behaviors: The outcome captures a change in the subjects’ actions in the real world and in their interactions with others. This change in behavior is measured or observed by the researcher and includes physical actions like crime, combat participation, protest, voting, movement, and so on.
- Observed online behaviors: The outcome captures a change in the subjects’ actions in the digital world. This change in behavior is measured or observed by the researcher and includes subjects’ reactions to posts, online responses or comments, agreement or disagreement with digital content, and so on.
Finally, drawing on the typology developed by Yadav (2021), we classified studies according to the types of interventions announced by social media platforms: redirection, content labeling, content distribution/sharing, disinformation disclosure, disinformation literacy, advertisement policy, content reporting, content account moderation, security/verification, or other. These categories are defined as follows:
- Advertisement policy: If the intervention changes the advertisement policy of the platform and has a user-facing component; e.g., (i) Facebook requires the “Paid for by” label, (ii) Facebook has an information button for advertisements.
- Content labeling: If the intervention labels posts, accounts, stories with (i) a fact-checking tag, (ii) funding tag, (iii) outdated tag, or any other forms of tagging, including providing further context without the user having to click through to receive the additional information; e.g., (i) Facebook adds fact-check labels to posts, (ii) Twitter labels tweets by state-media, etc.
- Content/account moderation: If the intervention involves the following action: (i) Takedown: removes content/posts/accounts (takedowns), (ii) Suspension: suspends accounts/blocks accounts, (iii) AI: modifies feed, trends, content appearances, and order (algorithmic and AI changes included); e.g., (i) YouTube downranks unauthoritative content, (ii) Twitter reduces interactions with accounts that users don’t follow, etc.
- Content reporting: If the intervention changes how users report problematic content on the platform; e.g., (i) TikTok introduced a ‘misinformation’ option in the content reporting options.
- Content distribution/sharing: If the intervention targets the distribution of content on platforms either by the platforms themselves or by users; e.g., (i) if Instagram limits a post from being found in Discover or stories, (ii) if WhatsApp limits forwards, (iii) if Pinterest prevents pinning or saving posts.
- Disinformation disclosure: If the intervention informs a user they have come in contact, shared, or interacted with disinformation; e.g., (i) Reddit telling users they’ve interacted with misinformation.
- Disinformation literacy: If the intervention aims to educate users to identify disinformation (or misinformation) online; e.g., (i) Snapchat’s myth-busting game, (ii) Facebook’s tool to help users identify misinformation.
- Redirection: If the intervention redirects users to different information, accounts, posts, either by taking them to a different link or by offering in-app notices or if the intervention imparts and curates accurate information (including but not limited to COVID-19); e.g., (i) Instagram showing content from CDC and WHO when users search for COVID-19, (ii) Facebook and Twitter’s U.S. election or COVID information hubs.
- Security/verification: If the intervention increases or decreases the security or verification requirements on the platform; e.g., (i) Twitter’s protection program for political officials.
Topics
Bibliography
Andı, S., & Akesson, J. (2021). Nudging away false news: Evidence from a social norms experiment. Digital Journalism, 9(1), 106–125. https://doi.org/10.1080/21670811.2020.1847674
Bernhardt, K. L., Kinnear, T. C., & Mazis, M. B. (1986). A field study of corrective advertising effectiveness. Journal of Public Policy & Marketing, 5(1), 146–162. https://doi.org/10.1177/074391568600500110
Bor, A., Osmundsen, M., Rasmussen, S. H. R., Bechmann, A., & Petersen, M. B. (2020). “Fact-checking” videos reduce belief in, but not the sharing of fake news on Twitter. PsyArXiv. https://doi.org/10.31234/osf.io/a7huq
Bowles, J., Larreguy, H., & Liu, S. (2020). Countering misinformation via WhatsApp: Preliminary evidence from the COVID-19 pandemic in Zimbabwe. PLOS ONE, 15(10). https://doi.org/10.1371/journal.pone.0240005
Bradshaw, S., & Neudert, L. (2021, January). The road ahead: Mapping civil society responses to disinformation. National Endowment for Democracy. https://www.ned.org/wp-content/uploads/2021/01/The-Road-Ahead-Mapping-Civil-Society-Responses-to-Disinformation-Bradshaw-Neudert-Jan-2021-2.pdf
Carnahan, D., Bergan, D. E., & Lee, S. (2020). Do corrective effects last? Results from a longitudinal experiment on beliefs toward immigration in the U.S. Political Behavior, 43, 1227–1246. https://doi.org/10.1007/s11109-020-09591-9
Christenson, D., Kreps, S. E., & Kriner, D. (2020). Going public in an era of social media: Tweets, corrections, and public opinion. SSRN. https://doi.org/10.2139/ssrn.3717823
Cook, J., Lewandowsky, S., & Ecker, U. K. H. (2017). Neutralizing misinformation through inoculation: Exposing misleading argumentation techniques reduces their influence. PLOS ONE, 12(5), e0175799. https://doi.org/10.1371/journal.pone.0175799
Dias, N., Pennycook, G., & Rand, D. G. (2020). Emphasizing publishers does not effectively reduce susceptibility to misinformation on social media. Harvard Kennedy School (HKS) Misinformation Review, 1(1). https://doi.org/10.37016/mr-2020-001
Dyer, R., & Kuehl, P. (1978). A longitudinal study of corrective advertising. Journal of Marketing Research, 15(1), 39–48. https://doi.org/10.1177/002224377801500106
Ecker, U. K. H., & Antonio, L. M. (2021). Can you believe it? An investigation into the impact of retraction source credibility on the continued influence effect. Memory & Cognition, 49, 631–644. https://doi.org/10.3758/s13421-020-01129-y
Ecker, U. K. H., Lewandowsky, S., & Tang, D. T. W. (2010). Explicit warnings reduce but do not eliminate the continued influence of misinformation. Memory & Cognition, 38, 1087–1100. https://doi.org/10.3758/MC.38.8.1087
Facebook. (2021, May). Threat report: The state of influence operations 2017–2020. https://about.fb.com/wp-content/uploads/2021/05/IO-Threat-Report-May-20-2021.pdf
Gordon, A., Ecker, U. K., & Lewandowsky, S. (2019). Polarity and attitude effects in the continued-influence paradigm. Journal of Memory and Language, 108, 104028. https://doi.org/10.1016/j.jml.2019.104028
Hameleers, M., Powell, T. E., van der Meer, T. G., & Bos, L. (2020). A picture paints a thousand lies? The effects and mechanisms of multimodal disinformation and rebuttals disseminated via social media. Political Communication, 37(2), 281–301. https://doi.org/10.1080/10584609.2019.1674979
Johnson, H. M., & Seifert, C. M. (1994). Sources of the continued influence effect: When misinformation in memory affects later inferences. Journal of Experimental Psychology: Learning, Memory, and Cognition, 20(6), 1420–1436. https://doi.org/10.1037/0278-7393.20.6.1420
Kim, J. W. (2019). Countering anti-vaccination rumors on Twitter [Doctoral dissertation, Syracuse University]. Surface. https://surface.syr.edu/etd/1089
Kim, A., Moravec, P. L., & Dennis, A. R. (2019). Combating fake news on social media with source ratings: The effects of user and expert reputation ratings. Journal of Management Information Systems, 36(3), 931–968. https://doi.org/10.1080/07421222.2019.1628921
Maertens, R., Roozenbeek, J., Basol, M., & van der Linden, S. (2020). Long-term effectiveness of inoculation against misinformation: Three longitudinal experiments. Journal of Experimental Psychology: Applied, 27(1), 1–16. https://doi.org/10.1037/xap0000315
Martin, D., Shapiro, J., & Ilhardt, J. (2020). Trends in online influence efforts. Empirical Studies of Conflict Project. https://esoc.princeton.edu/publications/trends-online-influence-efforts
McGinnies, E., & Ward, C. (1980). Better liked than right: Trustworthiness and expertise as factors in credibility. Personality and Social Psychology Bulletin, 6(3), 467–472. https://doi.org/10.1177/014616728063023
Mitts, T., Phillips, G., & Walter, B.F. (in press). Studying the impact of ISIS propaganda campaigns. Journal of Politics.
Mosleh, M., Martel, C., Eckles, D., & Rand, D. (2021, May). Perverse downstream consequences of debunking: Being corrected by another user for posting false political news increases subsequent sharing of low quality, partisan, and toxic content in a Twitter field experiment. Proceedings of the 2021 CHI Conference on Human Factors in Computing Systems, pp. 1–13. https://doi.org/10.1145/3411764.3445642
Nassetta, J., & Gross, K. (2020). State media warning labels can counteract the effects of foreign misinformation. Harvard Kennedy School (HKS) Misinformation Review, 1(7). https://doi.org/10.37016/mr-2020-45
National Science Foundation. (2021, March). Accelerating research into practice new funding opportunity: NSF Convergence Accelerator phase I and II for the 2021 cohort. https://www.nsf.gov/od/oia/convergence-accelerator/2021-solicitation.jsp
Nyhan, B., & Reifler, J. (2015). Displacing misinformation about events: An experimental test of causal corrections. Journal of Experimental Political Science, 2(1), 81–93. https://doi.org/10.1017/XPS.2014.22
Nyhan, B., & Reifler, J. (2016). Do people actually learn from fact-checking? Evidence from a longitudinal study during the 2014 campaign [Unpublished manuscript]. Dartmouth College.
Nyhan, B., Reifler, J., Richey, S., & Freed, G. L. (2014). Effective messages in vaccine promotion: A randomized trial. Pediatrics, 133(4), e835–e842. https://doi.org/10.1542/peds.2013-2365
Nyhan, B., & Reifler, J. (2010). When corrections fail: The persistence of political misperceptions. Political Behavior, 32(2), 303–330. https://doi.org/10.1007/s11109-010-9112-2
Nyhan, B., Reifler, J., & Ubel, P. A. (2013). The hazards of correcting myths about health care reform. Medical Care, 51(2), 127–132. https://doi.org/10.1097/mlr.0b013e318279486b
Nyhan, B., & Reifler, J. (2019). The roles of information deficits and identity threat in the prevalence of misperceptions. Journal of Elections, Public Opinion and Parties, 29(2), 222–244. https://doi.org/10.1080/17457289.2018.1465061
O’Rear, E. A., & Radvansky, G. A. (2020). Failure to accept retractions: A contribution to the continued influence effect. Memory & Cognition, 48, 127–144. https://doi.org/10.3758/s13421-019-00967-9
Pennycook, G., Epstein, Z., Mosleh, M., Arechar, A. A., Eckles, D., & Rand, D. (2019). Shifting attention to accuracy can reduce misinformation online. PsyArXiv. https://doi.org/10.31234/osf.io/3n9u8
Pennycook, G., McPhetres, J., Zhang, Y., Lu, J. G., & Rand, D. G. (2020). Fighting COVID-19 misinformation on social media: Experimental evidence for a scalable accuracy-nudge intervention. Psychological Science, 31(7), 770–780. https://doi.org/10.1177/0956797620939054
Peter, C., & Koch, T. (2016). When debunking scientific myths fails (and when it does not): The backfire effect in the context of journalistic coverage and immediate judgments as prevention strategy. Science Communication, 38(1), 3–25. https://doi.org/10.1177/1075547015613523
Pluviano, S., Della Sala, S., & Watt, C. (2020). The effects of source expertise and trustworthiness on recollection: The case of vaccine misinformation. Cognitive Processing, 21(3), 321–330. https://doi.org/10.1007/s10339-020-00974-8
Pluviano, S., Watt, C., & Sala, S. D. (2017). Misinformation lingers in memory: Failure of three pro-vaccination strategies. PLOS ONE, 12(7), e0181640. https://doi.org/10.1371/journal.pone.0181640
Roozenbeek, J., van der Linden, S., & Nygren, T. (2020). Prebunking interventions based on “inoculation” theory can reduce susceptibility to misinformation across cultures. Harvard Kennedy School (HKS) Misinformation Review, 1(2). https://doi.org/10.37016//mr-2020-008
Rosenzweig, L., Bago, B., Berinsky, A., & Rand, D. (2020, April 6). Misinformation and emotions in Nigeria: The case of COVID-19 fake news [Pre-analysis plan]. https://mitsloan.mit.edu/shared/ods/documents/?PublicationDocumentID=7588
Rosenzweig, L. R., Bago, B., Berinsky A. J., & Rand, D. G. (2021). Happiness and surprise are associated with worse truth discernment of COVID-19 headlines among social media users in Nigeria. Harvard Kennedy School (HKS) Misinformation Review, 2(4). https://doi.org/10.37016/mr-2020-75
Saltman, E., Kooti, F., & Vockery, K. (2021). New models for deploying counterspeech: Measuring behavioral change and sentiment analysis. Studies in Conflict & Terrorism, 1–24. https://doi.org/10.1080/1057610x.2021.1888404
Skurnik, I., Yoon, C., Park, D. C., & Schwarz, N. (2005). How warnings about false claims become recommendations. Journal of Consumer Research, 31(4), 713–724. https://doi.org/10.1086/426605
Swire‐Thompson, B., Ecker, U. K., Lewandowsky, S., & Berinsky, A. J. (2020). They might be a liar but they’re my liar: Source evaluation and the prevalence of misinformation. Political Psychology, 41(1), 21–34. https://doi.org/10.1111/pops.12586
Ternovski, J., Kalla, J., & Aronow, P. M. (2021). Deepfake warnings for political videos increase disbelief but do not improve discernment: Evidence from two experiments. OSF Preprints. https://doi.org/10.31219/osf.io/dta97
Twitter. (2021). Information operations – Twitter Transparency Center. https://transparency.twitter.com/en/reports/information-operations.html
Walter, N., & Salovich, N. A. (2021). Unchecked vs. uncheckable: How opinion-based claims can impede corrections of misinformation. Mass Communication and Society, 24(4), 500–526. https://doi.org/10.1080/15205436.2020.1864406
Wilkes, A. L., & Leatherbarrow, M. (1988). Editing episodic memory following the identification of error. The Quarterly Journal of Experimental Psychology, 40(2), 361–387. https://doi.org/10.1080/02724988843000168
Wintersieck, A., Fridkin, K., & Kenney, P. (2018). The message matters: The influence of fact-checking on evaluations of political messages. Journal of Political Marketing, 20(2), 93–120. https://doi.org/10.1080/15377857.2018.1457591
Wood, T., & Porter, E. (2019). The elusive backfire effect: Mass attitudes’ steadfast factual adherence. Political Behavior, 41(1), 135–163. https://doi.org/10.1007/s11109-018-9443-y
Yadav, K. (2020, November 30). Countering influence operations: A review of policy proposals since 2016. Partnership for Countering Influence Operations, Carnegie Endowment for International Peace. https://carnegieendowment.org/2020/11/30/countering-influence-operations-review-of-policy-proposals-since-2016-pub-83333
Yadav, K. (2021, January 25). Platform interventions: How social media counters influence operations. Partnership for Countering Influence Operations, Carnegie Endowment for International Peace. https://carnegieendowment.org/2021/01/25/platform-interventions-how-social-media-counters-influence-operations-pub-83698
Young, D. G., Jamieson, K. H., Poulsen, S., & Goldring, A. (2018). Fact-checking effectiveness as a function of format and tone: Evaluating FactCheck.org and FlackCheck.org. Journalism & Mass Communication Quarterly, 95(1), 49–75. https://doi.org/10.1177/1077699017710453
Funding
We acknowledge generous financial support from Microsoft and the Carnegie Endowment for International Peace.
Competing Interests
We have no competing interests to declare.
Ethics
This research did not involve human subjects.
Copyright
This is an open access article distributed under the terms of the Creative Commons Attribution License, which permits unrestricted use, distribution, and reproduction in any medium, provided that the original author and source are properly credited.
Data Availability
All materials needed to replicate this study are available through the ESOC website.
Acknowledgements
We thank Alicia Chen, Elonnai Hickock, Samikshya Siwakoti, Isra Thange, Alicia Wanless, and Kamya Yadav, as well as our anonymous referees, for invaluable feedback.