Peer Reviewed
Feedback and education improve human detection of image manipulation on social media
Article Metrics
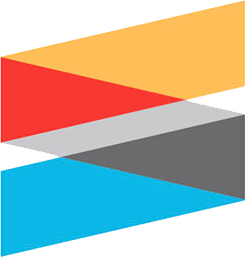
0
CrossRef Citations
Altmetric Score
PDF Downloads
Page Views
This study investigates the impact of educational interventions and feedback on users’ ability to detect manipulated images on social media, addressing a gap in research that has primarily focused on algorithmic approaches. Through a pre-registered randomized and controlled experiment, we found that feedback and educational content significantly improved participants’ ability to detect manipulated images on social media. However, the educational content did not result in a significantly greater improvement compared to feedback alone. These findings underscore feedback as a powerful tool for enhancing digital literacy, with practical implications for combating misinformation.
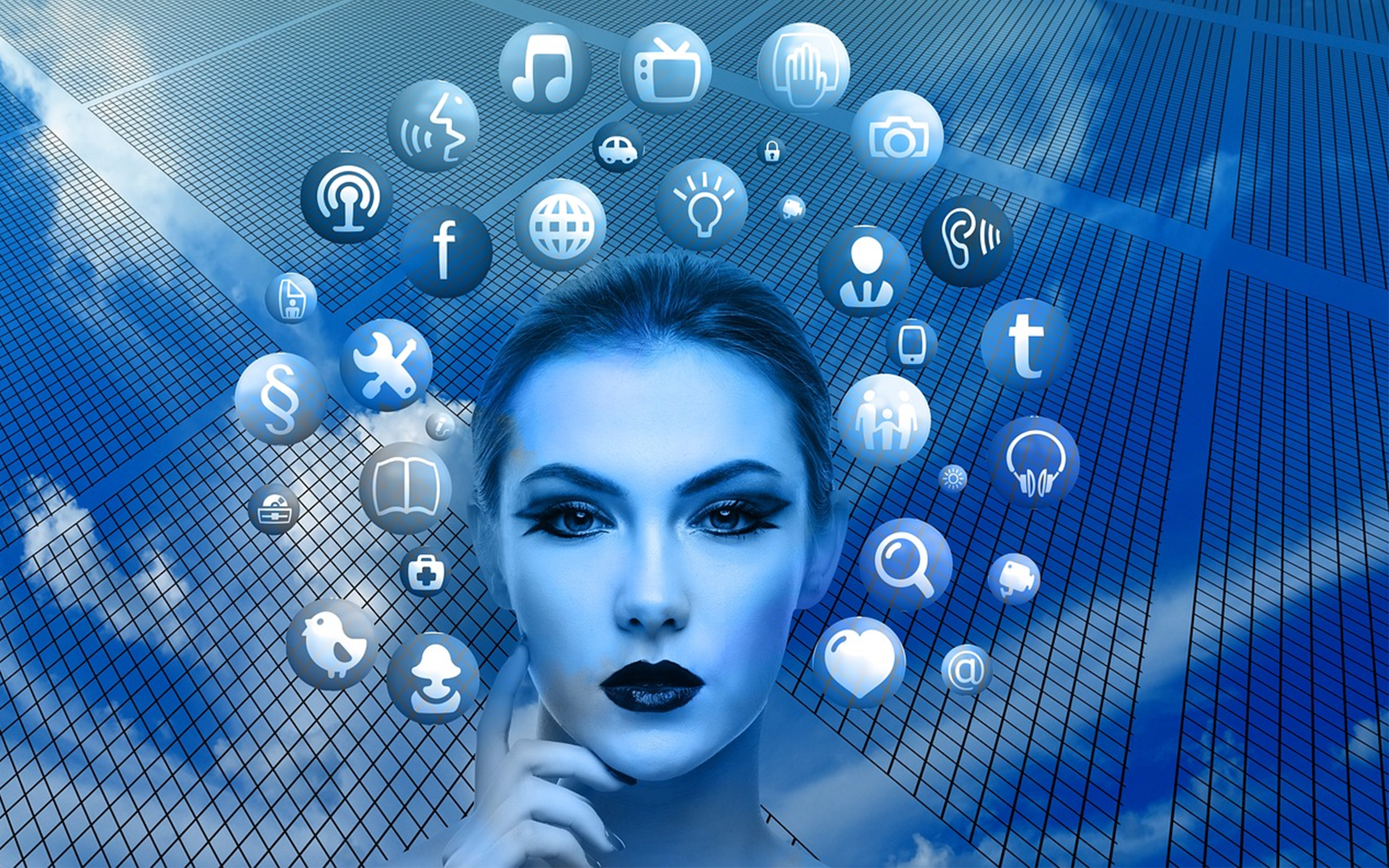
Research Questions
- Does feedback and education improve users’ accuracy in detecting manipulated images compared to no intervention?
- Does accuracy in detecting different types of image manipulation (copy-move, erase-fill, touch-up, etc.) vary across treatment and control groups?
- Does spending more time on image classification predict higher accuracy in detecting manipulation?
Essay Summary
- This study employed a pre-registered randomized and controlled experiment to evaluate the impact of feedback and educational interventions on improving participants’ ability to detect manipulated images on social media.
- Task feedback and feedback combined with educational content both significantly improved participants’ ability to detect manipulated images. However, there was no significant difference between the two groups, which was somewhat unexpected.
- These findings suggest that feedback is an effective tool for enhancing digital literacy and combating misinformation. Given that the educational intervention did not additionally improve accuracy, future interventions should focus on optimizing feedback systems and consider alternative educational strategies to improve image manipulation detection.
Implications
Image manipulation and media literacy interventions
In recent years, there has been a surge in manipulated content on the Internet (Shen et al., 2019; Wang et al., 2022). This sudden increase has made automatic image manipulation detection (e.g., DeepFake detection) a prominent area of research in computing and social sciences (Fridrich, 2009; Novozamsky et al., 2020; Thakur & Rohilla, 2020; Tyagi & Yadav, 2023). Much of the work in computing focuses on developing algorithms that utilize artificial intelligence (AI) systems to detect altered imagery (Bayar & Stamm, 2018; Cao et al., 2012; Mahdian & Saic, 2007; Wang et al., 2022; Zanardelli et al., 2023) with various technological approaches that work to varying degrees on different types of image and video alteration (Armas Vega et al., 2021; Chen et al., 2021; Cozzolino & Verdoliva, 2016; Huang & Ciou, 2019; Liu et al., 2022; Rossler et al., 2019; Yang et al., 2020; Zhang et al., 2020; Zhang et al., 2016; Zhou et al., 2017).
Image manipulation plays a significant role in spreading misinformation and disinformation, as altered visuals can mislead viewers by distorting reality, fueling biased narratives, or amplifying false messages (Ghai et al., 2024; Newman & Schwarz, 2023; Weikmann & Lecheler, 2023). This form of visual misinformation has profound societal impacts, influencing public opinion and trust in media (Langguth et al., 2021; Matatov et al., 2022; Yang et al., 2023); especially because social media users are typically unaware of the scale of image manipulations and are not good at detecting when manipulations are present (Nightingale et al., 2017; Schetinger et al., 2017).
Although advancements in algorithmic detection of image manipulation are essential, they must be paired with a deeper understanding of the user dynamics involved. There is a growing body of research demonstrating the effectiveness of online games that teach media and information literacy to users (Basol et al., 2020; Facciani et al., 2024; Roozenbeek & van der Linden, 2020). To effectively empower users to interact responsibly on social media, interface design decisions should work in tandem with media literacy efforts. This combination enables individuals to navigate online content with discernment, critically evaluate information, and make informed decisions for themselves and their followers.
Epstein and colleagues (2021) evaluated the impact of several different interventions on participant’s willingness to share true versus false headlines. The results showed that simple interventions, such as asking participants to reflect on the accuracy of headlines or providing basic digital literacy tips, significantly improved discernment between real and false news. In March of 2025, Reddit instituted a similar feedback mechanism by issuing warnings to users who repeatedly upvoted violent content, employing behavioral feedback to discourage interaction with policy-violating material.1https://www.reddit.com/r/RedditSafety/comments/1j4cd53/warning_users_that_upvote_violent_content/ The findings from Epstein and colleagues (2021) are consistent with other work showing that simple accuracy nudges improve discernment (Pennycook et al., 2020) along with media literacy training (Guess et al., 2020). As research evolves in this area, researchers should strive to understand if certain interventions are more effective for certain contexts, audiences, or topics.
This study examines how user behavior, interface design, and media literacy interventions intersect to improve detection of image manipulation. We evaluate the impact of feedback and educational strategies on participants’ ability to identify manipulated images, analyzing their interactions with a curated dataset of real (non-manipulated) and altered (manipulated) visuals to identify common errors and decision-making challenges. Figure 1 highlights key interventions implemented. This work contributes to the integration of technological tools with human-centered approaches to support critical media literacy skills.
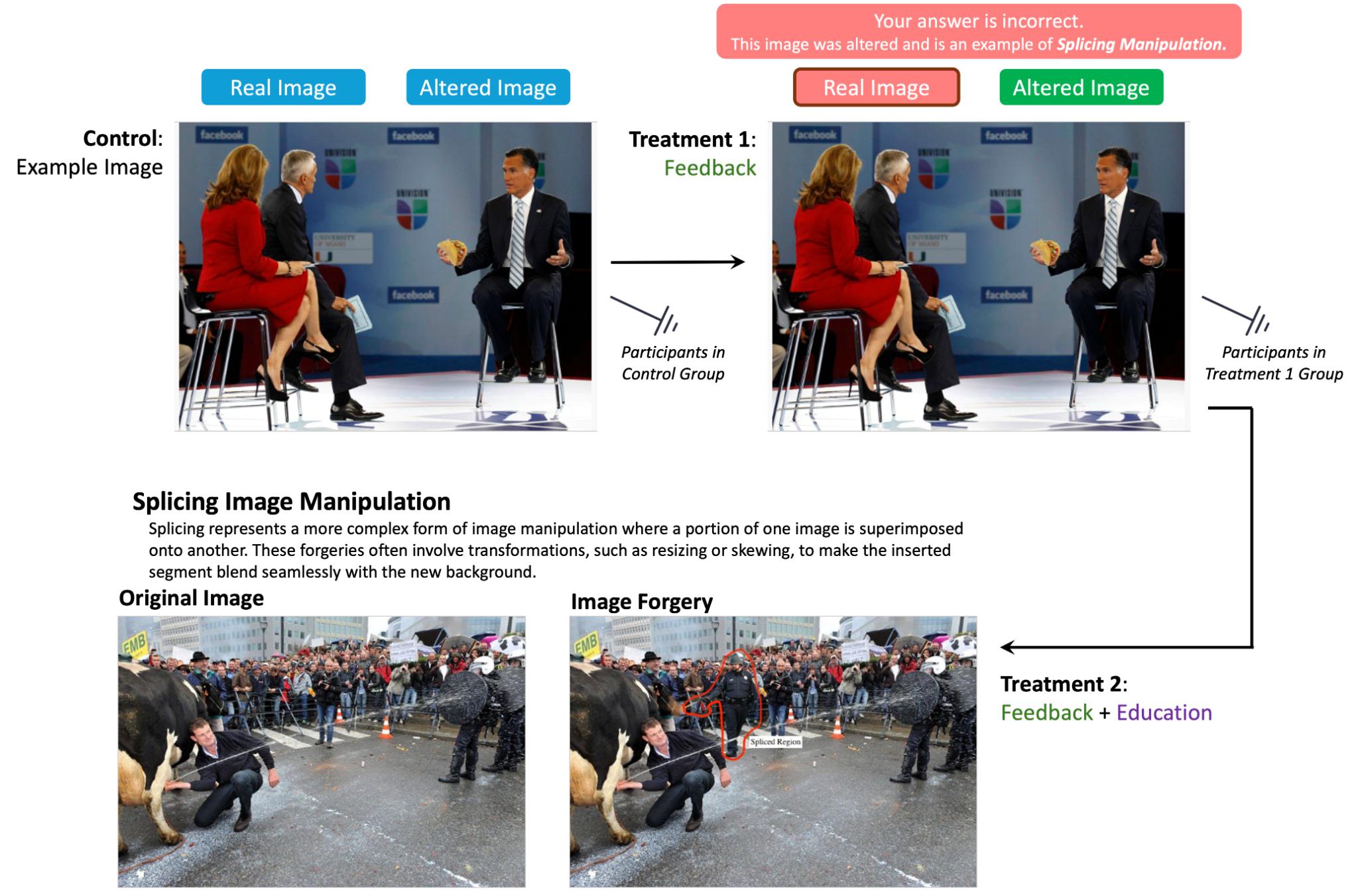
Implications from the present study
This study suggests that rapid, structured feedback significantly enhances users’ ability to detect manipulated content. These findings may inform user interface design, media literacy education, and public policy, emphasizing three actionable strategies:
- Enhanced user feedback systems. Real-time feedback systems, such as user-friendly notifications and algorithmic prompts, can effectively flag suspicious content. Features like tooltips on Instagram or links on Twitter/X could provide context for users. Transparency and nonpartisanship are essential to foster trust in these systems. Tailored interventions should consider political motivations behind manipulations and users’ varying levels of media literacy. Periodic reminders could further encourage critical engagement.
- Educational integration. Incorporating hands-on workshops and structured feedback into school curricula can strengthen digital literacy. Gamified and personalized learning experiences can increase user engagement while fostering collaborative and critical evaluation skills.
- Policy and platform recommendations. Our findings suggest that policymakers could consider supporting digital literacy campaigns and encouraging platforms to adopt enhanced feedback tools. Social media companies could benefit from prioritizing user empowerment by offering accessible educational features and tools that encourage discerning content evaluation, which may contribute to a more reliable information-sharing environment.
By combining these approaches, stakeholders can address misinformation more effectively and create a media landscape that supports informed and critical users.
Findings
Finding 1: Providing feedback and media literacy improves user detection of manipulated images.
We used ordinary least squares (OLS) regression analysis, a statistical method that examines how different factors influence an outcome, to evaluate the impact of feedback and media literacy interventions. Participants were shown images and asked to classify them as real (non-manipulated) or altered (manipulated). The outcome (i.e., dependent variable [DV]) was the total number of correct classifications, while the factors (i.e., independent variables [IVs]) represented each treatment condition compared to a control group. As shown in Figure 2, the OLS results indicated that participants in both the feedback and the feedback+education groups achieved significantly higher accuracy than those in the control group (p < .001). Additionally, the OLS regression results revealed that familiarity with manipulated content had a small yet statistically significant positive effect on accuracy, while political orientation did not have a significant effect.
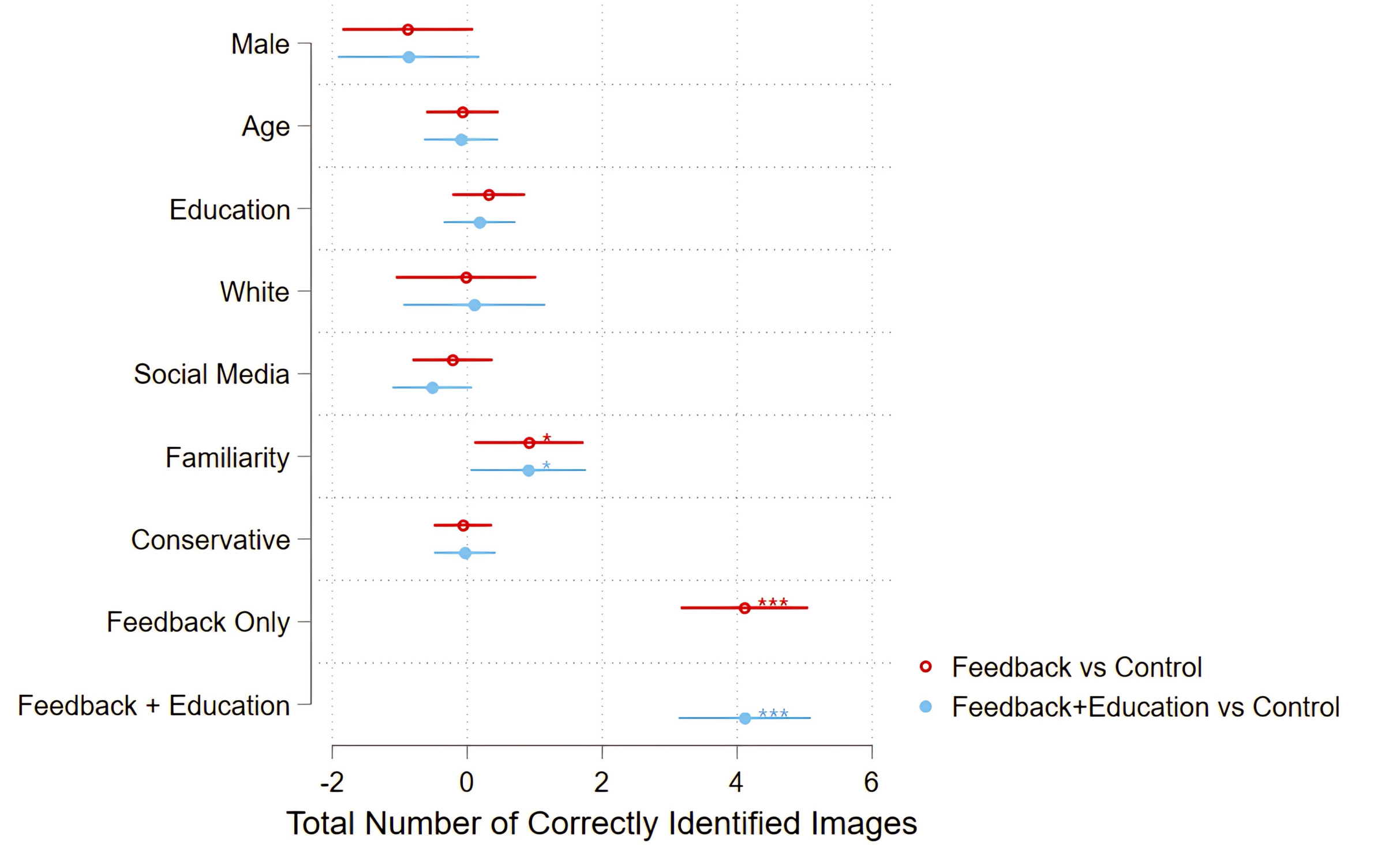
A one-way analysis of variance (ANOVA) was conducted to compare the mean number of correct classifications among the three groups, yielding significant differences, F(2, 267) = 67.29, p < .001. ANOVA is a statistical test used to compare the means of multiple groups to determine if any significant differences exist among them. The control group achieved a mean of correct classifications of 18.02 out of 32. The feedback and feedback+education treatment groups achieved means of 22.09 and 22.25, respectively. Thus, our treatment groups increased image classification by about 4 images out of 32 possible images. Importantly, both treatment groups appeared to improve performance equally well and there was no significant difference between their image classification scores (p > .05). We also conducted an exploratory analysis that found this treatment effect significantly improved image accuracy at the p < .001 level when only comparing the scores of the first four images, suggesting a rapid learning effect from our treatments. The results of ANOVA, regression, and power analysis can be found in the Appendix.
Both treatment groups significantly improved participants’ accuracy in identifying manipulated images compared to the control group, with an average increase of about four correctly classified images out of 32. However, there was no significant difference between the feedback and feedback+education groups, suggesting that both interventions were equally effective. This result was somewhat unexpected, as we anticipated education would have a more pronounced effect. One possible explanation is that the educational intervention was too brief and simple, which did not lead to any additional effect. Feedback, which is itself a type of educational intervention (Epstein et al., 2021), appeared to be sufficient to improve accuracy. These findings underscore the value of feedback and suggest that more robust or long-term educational interventions may be needed to enhance participants’ detection abilities. Future research could explore the role of different types of educational interventions to better understand their impact.
Finding 2: The type of image manipulation matters.
We conducted one-way ANOVAs to evaluate how well participants classified images based on manipulation types (erase-fill, splicing, copy-move, and Photoshop touch-up) and intervention groups (control, feedback, and feedback+education). The analysis revealed a significant effect of manipulation type within the control group, F(3, 1485) = 19.38, p < .001, meaning that both the type of image manipulation and the intervention influenced participants’ accuracy.
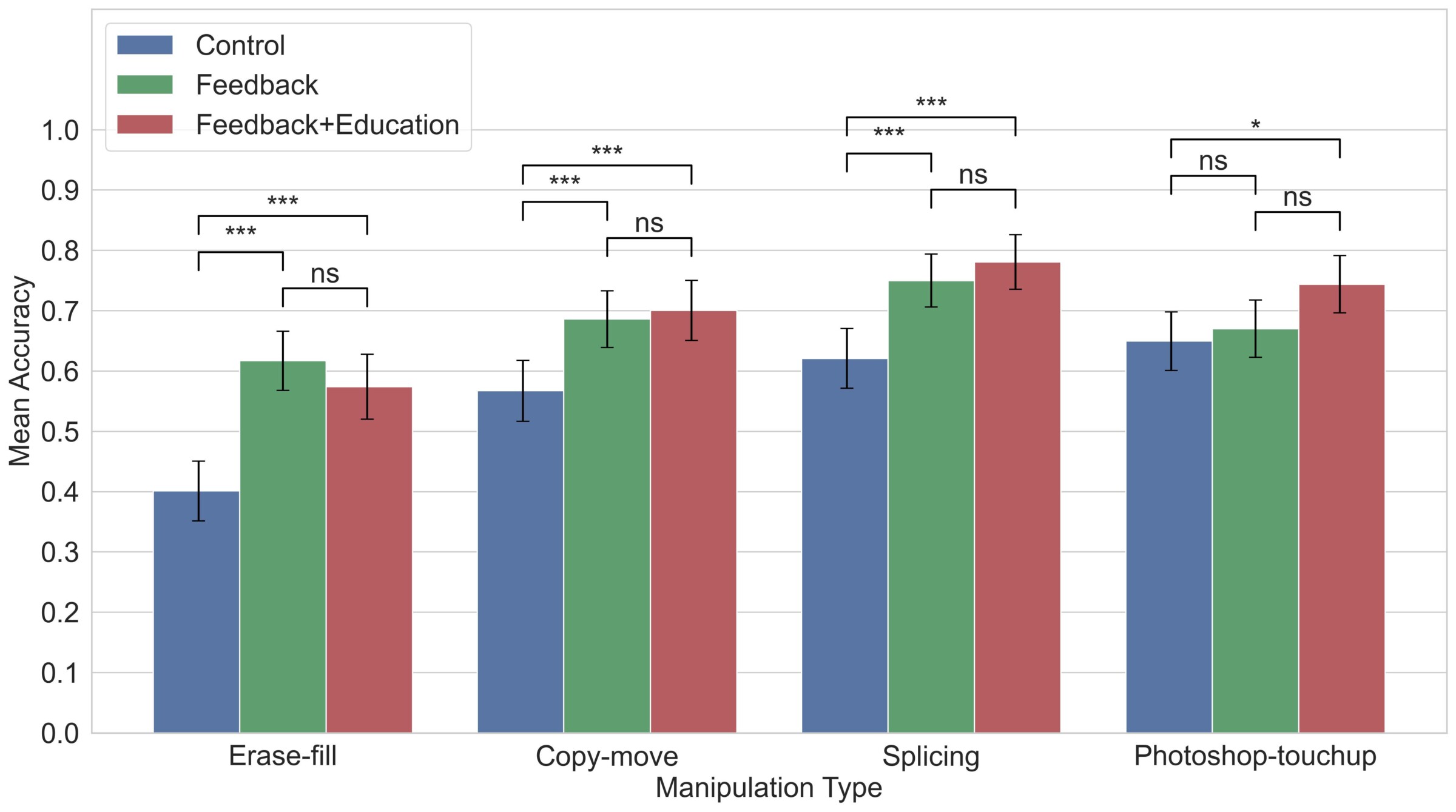
To better understand these results, we performed post hoc Bonferroni tests, which are follow-up analyses used to pinpoint specific differences between groups while accounting for multiple comparisons. As shown in Figure 3, these tests revealed that erase-fill manipulations were significantly harder to detect compared to splicing, copy-move, and Photoshop touch-up manipulations (p < .001). These findings highlight that some manipulation techniques, like erase-fill, pose a greater challenge for users, even when interventions are in place.
Feedback significantly improved user accuracy in detecting image manipulations across all types, with no additional benefit from the educational intervention. Erase-fill manipulations remained the most challenging for users, even with feedback, while splicing, copy-move, and Photoshop touch-up were relatively easier to classify. Bonferroni tests confirmed that feedback enhanced accuracy for more difficult manipulations, highlighting its role as the primary driver of improvement. These results suggest that tailoring feedback systems to address particularly challenging manipulation types, like erase-fill, could maximize their effectiveness.
Finding 3: Longer response times are associated with lower accuracy.
Our results show that each additional second spent on a decision slightly reduced the likelihood of a correct response (b = -0.034, 95% CI [-0.040, -0.027]; Table A12). Correlation analysis confirmed this negative relationship (rpb= -0.12, p < 0.001). One explanation is that overthinking led to poorer judgments (Junghaenel et al., 2022). Alternatively, participants may have simply spent more time on difficult images, which were harder to classify regardless of time spent. In other words, rather than increased time causing lower accuracy, both prolonged response times and reduced accuracy may stem from the underlying difficulty of detecting certain manipulations.
Cognitive overload may also play a role—complex manipulations like erase-fill edits likely required more mental effort, leading to decision fatigue and lower accuracy. Additionally, distractions such as smartphone use may have further impacted performance (Ward et al., 2017). Future research could explore how task difficulty, cognitive load, and environmental factors interact to influence accuracy in detecting image manipulation.
Our study is not without limitations. It focused on images from a specific online community (/r/photoshopbattles), and the intervention’s effectiveness for politically motivated or other specialized manipulations remains uncertain. Notably, there was no significant difference in accuracy between participants receiving feedback alone and those receiving feedback plus an educational intervention, suggesting that reflecting on the accuracy of their classifications during feedback may enhance critical thinking (Epstein et al., 2021). Additionally, as prior research has shown, media literacy interventions often have short-lived effects (Maertens et al., 2021). While we did not assess long-term retention, this remains an important consideration for future studies. Tailoring interventions to evolving manipulation techniques and measuring their lasting impact could further enhance detection accuracy over time.
Methods
Procedure
This pre-registered study employed a between-subjects design with one factor (educational intervention) across three groups. As shown in Figure 4, participants were randomly assigned to one of three groups: a control group (no feedback), Treatment Group 1 (feedback), or Treatment Group 2 (feedback plus an educational intervention). The educational intervention covered four image manipulation techniques: copy-move, splicing, erase-fill, and Photoshop touch-up.To ensure a balanced and randomized sample, each participant was shown 16 real (non-manipulated) and 16 altered (manipulated) images, with four from each manipulation type. This setup reflects the variety and unpredictability of social media content, making the findings more applicable to real-world scenarios. Instructions clarified the terms real image as non-manipulated and altered image as manipulated before participants began the task. Accuracy in detecting manipulated images was the primary outcome measure, evaluated based on responses to specific manipulation techniques.
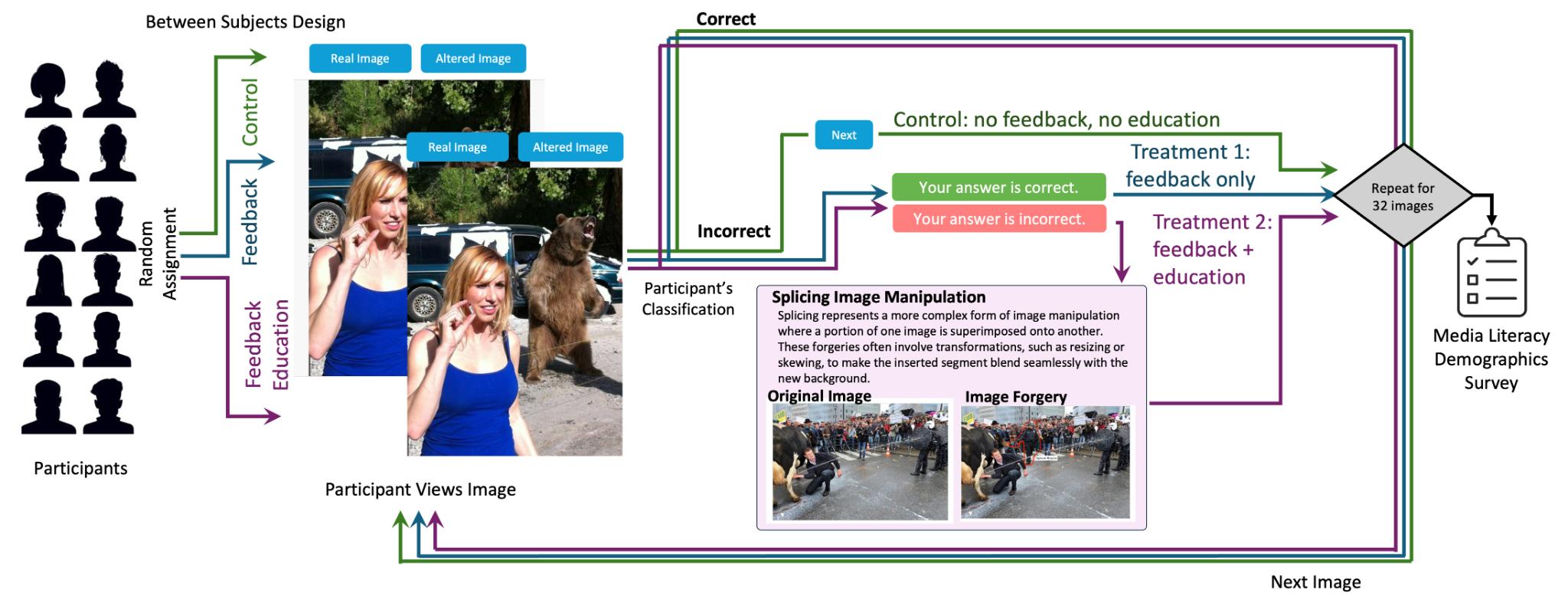
Participants
A total of 300 participants were recruited via Prolific, a platform for crowdsourcing tasks for academic research. Of those, 268 participants completed the survey. Participants were compensated $1.75 for completing the task. Upon completion, they provided demographic information, including race, age, educational background, and experience with digital image manipulation, allowing us to understand how different groups engaged with the task. The sample was 67% White and 33% non-White. Gender demographics were 42% women and 58% men or other identities. Ages were 53% under 35 and 47% over 35. Education levels were 64% with college or graduate degrees and 36% without. Social media usage was 78% daily and 22% less frequent. Familiarity with image manipulation was 93% somewhat familiar or higher and 7% not familiar. Politically, 56% were liberal or somewhat liberal and 44% moderate, conservative, or apolitical. A detailed breakdown is in the Appendix.
Image sourcing
This study used a dataset of 202 images from the subreddit /r/photoshopbattles, an online community focused on image manipulation. This dataset was chosen over deepfake datasets because it better represents the types of manipulations commonly encountered on social media, ranging from subtle edits to complex composites. The dataset included 79 authentic images (which provided enough manipulated images to ensure even distribution across types, enabling balanced random sampling) and 123 manipulated ones, categorized into four manipulation types:
- Erase-fill (28 images): Removing parts of an image and seamlessly filling the gaps using tools like content-aware fill (Thakur & Rohilla, 2020).
- Copy-move (29 images): Duplicating and repositioning regions within the same image, often with scaling or rotation (Khudhair et al., 2021).
- Splicing (36 images): Combining portions of multiple images into a seamless final product, making detection challenging (Thakur & Rohilla, 2020).
- Photoshop touchup (30 images): Subtle adjustments such as color correction, smoothing, or selective edits (Swerzenski, 2021; Wang et al., 2019).
Topics
Bibliography
Armas Vega, E. A., González Fernández, E., Sandoval Orozco, A. L., & García Villalba, L. J. (2021). Copy-move forgery detection technique based on discrete cosine transform blocks features. Neural Computing and Applications, 33, 4713–4727. https://doi.org/10.1007/s00521-020-05433-1
Basol, M., Roozenbeek, J., & van der Linden, S. (2020). Good news about bad news: Gamified inoculation boosts confidence and cognitive immunity against fake news. Journal of Cognition, 3(1). https://doi.org/10.5334%2Fjoc.91
Bayar, B., & Stamm, M. C. (2018). Constrained convolutional neural networks: A new approach towards general purpose image manipulation detection. IEEE Transactions on Information Forensics and Security, 13(11), 2691–2706. https://doi.org/10.1109/TIFS.2018.2825953
Cao, Y., Gao, T., Fan, L., & Yang, Q. (2012). A robust detection algorithm for copy-move forgery in digital images. Forensic Science International, 214(1–3), 33–43. https://doi.org/10.1016/j.forsciint.2011.07.015
Chen, X., Dong, C., Ji, J., Cao, J., & Li, X. (2021). Image manipulation detection by multi-view multi-scale supervision. In 2021 IEEE/CVF international conference on computer vision (ICCV) (pp. 14185–14193). IEEE. https://doi.org/10.1109/ICCV48922.2021.01392
Cozzolino, D., & Verdoliva, L. (2016). Single-image splicing localization through autoencoder-based anomaly detection. In 2016 IEEE international workshop on information forensics and security (WIFS) (pp. 1–6). IEEE. https://doi.org/10.1109/WIFS.2016.7823921
Epstein Z., Berinsky A. J., Cole, R., Gully, A., Pennycook, G., & Rand, D. G. (2021). Developing an accuracy-prompt toolkit to reduce COVID-19 misinformation online. Harvard Kennedy School (HKS) Misinformation Review, 2(3). https://doi.org/10.37016/mr-2020-71
Facciani, M. J., Apriliawati, D., & Weninger, T. (2024). Playing Gali Fakta inoculates Indonesian participants against false information. Harvard Kennedy School (HKS) Misinformation Review, 5(4). https://doi.org/10.37016/mr-2020-152
Fridrich, J. (2009). Digital image forensics. IEEE Signal Processing Magazine, 26(2), 26–37. https://doi.org/10.1109/MSP.2008.931078
Ghai, A., Kumar, P., & Gupta, S. (2024). A deep-learning-based image forgery detection framework for controlling the spread of misinformation. Information Technology & People, 37(2), 966–997. https://doi.org/10.1108/ITP-10-2020-0699
Guess, A. M., Lerner, M., Lyons, B., Montgomery, J. M., Nyhan, B., Reifler, J., & Sircar, N. (2020). A digital media literacy intervention increases discernment between mainstream and false news in the United States and India. Proceedings of the National Academy of Sciences, 117(27), 15536–15545. https://doi.org/10.1073/pnas.1920498117
Huang, H. Y., & Ciou, A. J. (2019). Copy-move forgery detection for image forensics using the superpixel segmentation and the Helmert transformation. EURASIP Journal on Image and Video Processing, 2019, 68. https://doi.org/10.1186/s13640-019-0469-9
Junghaenel, D. U., Schneider, S., Orriens, B., Jin, H., Lee, P. -J., Kapteyn, A., Meijer, E., Zelinski, E., Hernandez, R., & Stone, A. A. (2022). Inferring cognitive abilities from response times to web-administered survey items in a population-representative sample. Journal of Intelligence, 11(1), 3. https://doi.org/10.3390/jintelligence11010003
Khudhair, Z. N., Mohamed, F., & Kadhim, K. A. (2021, April). A review on copy-move image forgery detection techniques. Journal of Physics: Conference Series, 1892(1), 012010. https://doi.org/10.1088/1742-6596/1892/1/012010
Langguth, J., Pogorelov, K., Brenner, S., Filkuková, P., & Schroeder, D. T. (2021). Don’t trust your eyes: Image manipulation in the age of DeepFakes. Frontiers in Communication, 6, 632317. https://doi.org/10.3389/fcomm.2021.632317
Liu, X., Liu, Y., Chen, J., & Liu, X. (2022). PSCC-Net: Progressive spatio-channel correlation network for image manipulation detection and localization. IEEE Transactions on Circuits and Systems for Video Technology, 32(11), 7505–7517. https://doi.org/10.1109/TCSVT.2022.3189545
Maertens, R., Roozenbeek, J., Basol, M., & van der Linden, S. (2021). Long-term effectiveness of inoculation against misinformation: Three longitudinal experiments. Journal of Experimental Psychology: Applied, 27(1), 1–16. https://doi.org/10.1037/xap0000315
Mahdian, B., & Saic, S. (2007). Detection of copy–move forgery using a method based on blur moment invariants. Forensic Science International, 171(2–3), 180–189. https://doi.org/10.1016/j.forsciint.2006.11.002
Matatov, H., Naaman, M., & Amir, O. (2022). Stop the [Image] steal: The role and dynamics of visual content in the 2020 US election misinformation campaign. Proceedings of the ACM on human-computer interaction, 6(CSCW2), 1–24. https://doi.org/10.1145/3555599
Newman, E. J., & Schwarz, N. (2023). Misinformed by images: How images influence perceptions of truth and what can be done about it. Current Opinion in Psychology, 56, 101778. https://doi.org/10.1016/j.copsyc.2023.101778
Nightingale, S. J., Wade, K. A., & Watson, D. G. (2017). Can people identify original and manipulated photos of real-world scenes? Cognitive research: Principles and Implications, 2, 30. https://doi.org/10.1186/s41235-017-0067-2
Novozamsky, A., Mahdian, B., & Saic, S. (2020). IMD2020: A large-scale annotated dataset tailored for detecting manipulated images. In 2020 IEEE winter applications of computer vision workshops (pp. 71–80). IEEE. https://doi.org/10.1109/WACVW50321.2020.9096940
Pennycook, G., McPhetres, J., Zhang, Y., Lu, J. G., & Rand, D. G. (2020). Fighting COVID-19 misinformation on social media: Experimental evidence for a scalable accuracy-nudge intervention. Psychological Science, 31(7), 770–780. https://doi.org/10.1177/0956797620939054
Roozenbeek, J., & van der Linden, S. (2020). Breaking Harmony Square: A game that “inoculates” against political misinformation. Harvard Kennedy School (HKS) Misinformation Review, 1(8). https://doi.org/10.37016/mr-2020-47
Rossler, A., Cozzolino, D., Verdoliva, L., Riess, C., Thies, J., & Nießner, M. (2019). Faceforensics++: Learning to detect manipulated facial images. In 2019 IEEE/CVF international conference on computer vision (pp. 1–11). https://doi.org/10.1109/ICCV.2019.00009
Schetinger, V., Oliveira, M. M., da Silva, R., & Carvalho, T. J. (2017). Humans are easily fooled by digital images. Computers & Graphics, 68, 142–151. https://doi.org/10.1016/j.cag.2017.08.010
Shen, C., Kasra, M., Pan, W., Bassett, G. A., Malloch, Y., & O’Brien, J. F. (2019). Fake images: The effects of source, intermediary, and digital media literacy on contextual assessment of image credibility online. New Media & Society, 21(2), 438–463. https://doi.org/10.1177/1461444818799526
Swerzenski, J. D. (2021). Fact, fiction or Photoshop: Building awareness of visual manipulation through image editing software. Journal of Visual Literacy, 40(2), 104–124. https://doi.org/10.1080/1051144X.2021.1902041
Thakur, R., & Rohilla, R. (2020). Recent advances in digital image manipulation detection techniques: A brief review. Forensic Science International, 312, 110311. https://doi.org/10.1016/j.forsciint.2020.110311
Tyagi, S., & Yadav, D. (2023). A detailed analysis of image and video forgery detection techniques. The Visual Computer, 39(3), 813–833. https://doi.org/10.1007/s00371-021-02347-4
Wang, J., Wu, Z., Chen, J., Han, X., Shrivastava, A., Lim, S. N., & Jiang, Y. G. (2022). ObjectFormer for image manipulation detection and localization. In 2022 IEEE/CVF conference on computer vision and pattern recognition (pp. 2364–2373). IEEE. https://doi.ieeecomputersociety.org/10.1109/CVPR52688.2022.00240
Wang, S. Y., Wang, O., Owens, A., Zhang, R., & Efros, A. A. (2019). Detecting photoshopped faces by scripting Photoshop. In 2019 IEEE/CVF international conference on computer vision (pp. 10072–10081). IEEE. https://doi.org/10.1109/ICCV.2019.01017
Ward, A. F., Duke, K., Gneezy, A., & Bos, M. W. (2017). Brain drain: The mere presence of one’s own smartphone reduces available cognitive capacity. Journal of the Association for Consumer Research, 2(2), 140–154. https://doi.org/10.1086/691462
Weikmann, T., & Lecheler, S. (2023). Visual disinformation in a digital age: A literature synthesis and research agenda. New Media & Society, 25(12), 3696–3713. https://doi.org/10.1177/14614448221141648
Yang, Y., Davis, T., & Hindman, M. (2023). Visual misinformation on Facebook. Journal of Communication, 73(4), 316–328. https://doi.org/10.1093/joc/jqac051
Yang, C., Li, H., Lin, F., Jiang, B., & Zhao, H. (2020, July). Constrained R-CNN: A general image manipulation detection model. In 2020 IEEE International conference on multimedia and expo (ICME) (pp. 1–6). IEEE. https://doi.org/10.1109/ICME46284.2020.9102825
Zanardelli, M., Guerrini, F., Leonardi, R., & Adami, N. (2023). Image forgery detection: A survey of recent deep-learning approaches. Multimedia Tools and Applications, 82(12), 17521–17566. https://doi.org/10.1007/s11042-022-13797-w
Zhang, Y., Goh, J., Win, L. L., & Thing, V. (2016). Image region forgery detection: A deep learning approach. In A. Mathur, & A. Roychoudhury (Eds.), Proceedings of the Singapore cyber-security conference (SG–CRC) 2016 (pp. 1–11). IOS Press. https://doi.org/10.3233/978-1-61499-617-0-1
Zhang, D., Chen, X., Li, F., Sangaiah, A. K., & Ding, X. (2020). Seam‐Carved Image Tampering Detection Based on the Cooccurrence of Adjacent LBPs. Security and Communication Networks, 2020(1), 8830310. https://doi.org/10.1155/2020/8830310
Zhou, P., Han, X., Morariu, V. I., & Davis, L. S. (2017). Two-stream neural networks for tampered face detection. In 2017 IEEE conference on computer vision and pattern recognition workshops (CVPRW) (pp. 1831–1839). IEEE. https://doi.org/10.1109/CVPRW.2017.229
Funding
This work was funded cooperative agreement from USAID, award 7200AA18CA00059.
Competing Interests
The authors declare no competing interests.
Ethics
This study was conducted in accordance with ethical guidelines and principles governing research involving human participants. We obtained ethics review approval from Notre Dame Institutional Review Board (IRB) to ensure that our research adhered to ethical standards (Protocol #24-03-8475).
Prior to participation, all individuals were required to read and sign an informed consent form, which provided detailed information about the study’s purpose, procedures, potential risks, and benefits. Participants were assured that their involvement was voluntary and that they could withdraw from the study at any time without penalty.
To protect participant privacy and confidentiality, no personally identifying information (PII) was collected during the study. Data were anonymized and stored securely to prevent unauthorized access.
Copyright
This is an open-access article distributed under the terms of the Creative Commons Attribution License, which permits unrestricted use, distribution, and reproduction in any medium, provided that the original author and source are properly credited.
Data Availability
Pre-registration available at https://osf.io/pvq4d/?view_only=a111c2cfa40f4e05a615bf47040a3c64. All materials needed to replicate this study are available via the Harvard Dataverse: https://doi.org/10.7910/DVN/CKMBCG
Acknowledgments
We thank the reviewers for their feedback.