Peer Reviewed
Fact-opinion differentiation
Article Metrics
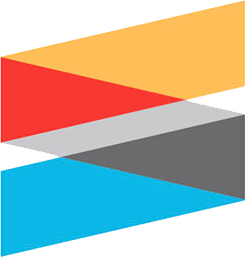
0
CrossRef Citations
Altmetric Score
PDF Downloads
Page Views
Statements of fact can be proved or disproved with objective evidence, whereas statements of opinion depend on personal values and preferences. Distinguishing between these types of statements contributes to information competence. Conversely, failure at fact-opinion differentiation potentially brings resistance to corrections of misinformation and susceptibility to manipulation. Our analyses show that on fact-opinion differentiation tasks, unsystematic mistakes and mistakes emanating from partisan bias occur at higher rates than accurate responses. Accuracy increases with political sophistication. Affective partisan polarization promotes systematic partisan error: as views grow more polarized, partisans increasingly see their side as holding facts and the opposing side as holding opinions.
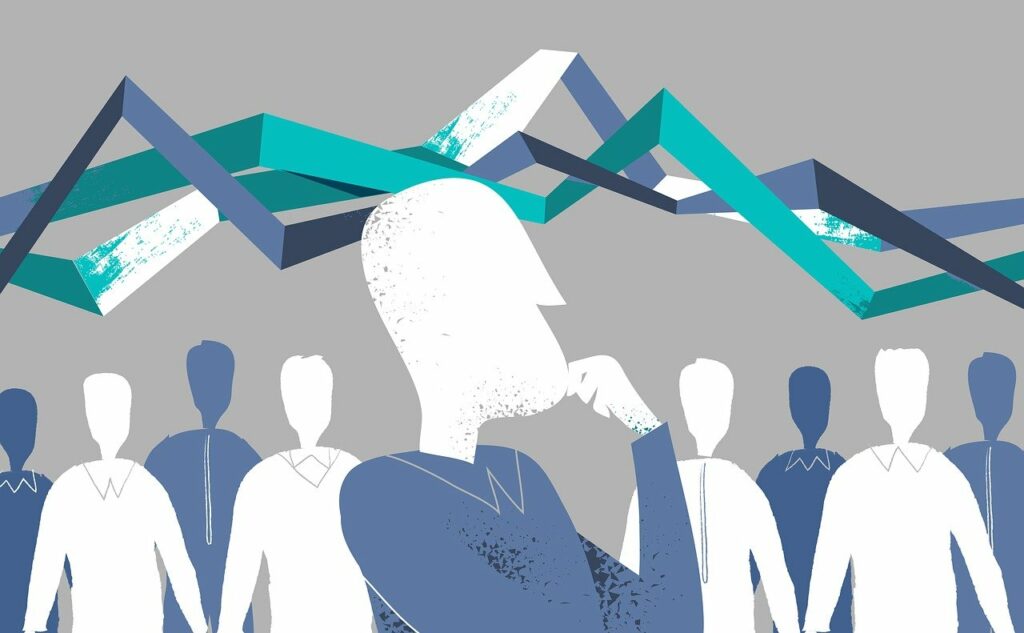
Research Questions
- To what extent do Americans succeed at distinguishing statements of fact from statements of opinion?
- Do civics knowledge, current events knowledge, cognitive ability, and education contribute to success at fact-opinion differentiation?
- Does affective partisan polarization lead to partisan bias in fact-opinion differentiation?
Essay Summary
- Twelve fact-opinion items included on a 2019 YouGov survey asked respondents to determine whether claims were statements of fact or opinion. We grouped responses into three categories: accurate response, partisan error, and unbiased (or nonpartisan) error. Multivariate analyses use grouped-data multinomial logistic regression.
- Accurate fact-opinion differentiation occurs less frequently than partisan error and unbiased error. Accuracy increases with elements of political sophistication including civics knowledge, current events knowledge, cognitive ability, and education. Affective partisan polarization heightens levels of partisan error.
- Faulty fact-opinion differentiation produces meta-level misinformation: Individuals disagree not only on the facts but also on what facts are. This can lead to information polarization because biased partisans tend to see their side as possessing the facts and the other side as possessing opinions. Faulty fact-opinion differentiation may also produce resistance to correction of misinformation if an “agree to disagree” mentality extends to questions of fact.
Implications
During an episode of the sitcom Abbott Elementary, the whiteboard in second-grade teacher Janine Teagues’ classroom displayed the outline of a lesson on fact-opinion differentiation: “Fact vs. Opinion. Fact – A thing that is known or proved to be true. Opinion – A personal judgment, thought, or belief.”1The episode “Teacher Appreciation” originally aired on March 8, 2023; see Murphy and >> & Einhorn (2023). The skill taught in that lesson represents a key component of successful information processing. Statements of fact and statements of opinion differ in form, content, and significance. Absent a capacity to distinguish between statements of fact and statements of opinion, our concern is that individuals will struggle to make sense of the claims they encounter from sources such as politicians, newscasters, and salespersons.
The producers of Abbott Elementary were right to incorporate a lesson on fact-opinion differentiation in their fictional curriculum. Faulty fact-opinion differentiation leaves individuals misinformed not because they are wrong on the facts but because they are wrong on what facts are. Consequently, we posit that fact-opinion differentiation may play an underappreciated role in how individuals come to be misinformed and why misinformation can be difficult to correct. Research on the nature and significance of factual misinformation abounds, with much of this research exploring basic matters regarding how much misinformation exists, the conditions in which it flourishes, and the factors that lead individuals to be susceptible to it. Building on that research requires attention to the information-processing skills that can empower individuals to be more sophisticated information consumers. Fact-opinion differentiation is one such skill because understanding what is and is not a factual claim fosters more discerning categorization and evaluation of new information. Statements of fact are claims that can be “proved or disproved by objective evidence” (Mitchell et al., 2018, p. 3). Similar terminology is used in educational resources. As one example, a review sheet published by Palm Beach State College (n.d.) explains that “A fact is a statement that can be verified. It can be proven to be true or false through objective evidence.”
Objective evidence is often quantifiable (e.g., the number of votes cast in an election and the change in the national unemployment rate from one month to another) and comes from verifiable sources and methods such as official government records. In some instances, objective evidence can be obtained from scientific testing. For example, if someone claimed, “the color green can be formed by mixing blue and yellow” or “the boiling point of water is 212° F,” others could conduct their own tests to corroborate those assertions.
Statements of opinion are claims that cannot be proved true or false with objective evidence because their assessment depends on individual preferences and values. For Mitchell et al. (2018), a statement of opinion “reflects the beliefs and values of whoever expressed it” (p. 3). Examples are “the unemployment rate is too high” and “green is the most beautiful color.” Both these claims reflect personal values and preferences, and other people may feel differently.2We focused on statements that clearly either can be proved true or false with objective evidence or that depend on individual preferences and values. We acknowledge that some statements are borderline. For these, objective evidence can be used to assess the statements, but ambiguity remains because the statements are predictive or because room exists for disagreement about what standards should be used to evaluate the claims. A Pew Report by Mitchell et al. (2018) offers a useful discussion of borderline cases. The two examples the authors tested are “Applying additional scrutiny to Muslim Americans would not reduce terrorism in the U.S.” and “Voter fraud across the U.S. has undermined the results of our elections.” The first statement is predictive, meaning current data cannot definitively speak to the statement’s accuracy. The second statement is subjective because people can disagree regarding the point at which election results have been “undermined.” The authors’ survey data showed that most respondents determined these to be statements of opinion.
Importantly, statements of fact are not inherently true. Factual claims can get the facts wrong. For instance, “2 + 2 = 22” is a statement of fact, but it is factually incorrect. Making errors in statements of fact does not transform those statements of fact into statements of opinion. They are incorrect statements of fact. As an example, a news source might see a 4.4% unemployment rate in a Bureau of Labor Statistics document but inadvertently report it as 44%. That would be an incorrect statement of fact, and it would be a statement that could be proved false with review of objective evidence—the actual rate as reported by the BLS. Thus, statements of fact and statements of opinion both come in two forms. For statements of fact, some are correct, and others are incorrect. For statements of opinion, some are opinions we share, and others are opinions we reject.
Most scholarly research on misinformation examines whether people get the facts right.3The most common dependent variable in misinformation research represents whether people accept or reject misinformation. For meta-analyses, see Walter and Murphy (2018) and Murphy et al. (2023). The implicit assumption is that people agree that factual matters are under consideration but sometimes get the facts wrong. At any given moment, the U.S. unemployment rate is below 5% or it is greater than or equal to 5%. Therefore, if one person says unemployment is less than 5% while another person insists it is higher, one of them is right, and the other is wrong. When one person claims “the U.S. unemployment rate currently is 10.5%,” a second person might answer, “That’s wrong. Here is the Bureau of Labor Statistics website. The current unemployment rate is 3.7%.” In this scenario, an obvious path to correction of misinformation exists, and corrective efforts can be expected to yield at least some success.
Faulty fact-opinion differentiation produces a different form of misinformation. Instead of disagreeing on the facts, there is disagreement about whether the matter under consideration even involves facts. In this circumstance, the hypothetical conversation would play out differently. When the first person asserts that the national unemployment rate is 10.5% and the second shows that the rate is actually 3.7%, the first person might react by saying, “we can agree to disagree. We’re each entitled to our own opinion.” This is meta-level misinformation. The first person has misconstrued the empirical world due to a misunderstanding about the very nature of statements of fact and statements of opinion.
Flipping our example around, flawed fact-opinion differentiation can also lead people to see facts where they do not exist. When a candidate insists “unemployment is too high,” that is an opinion. It is an interpretation of current conditions. A voter who accepts that claim on its face as being a statement of fact would misconstrue the empirical world. Upon making that error, a risk exists that the voter would see no need to acquire factual information, such as what the unemployment rate is and how that rate compares with historical averages. Thinking we have the facts when we do not is a form of misinformation.
Only a handful of prior studies on the link between fact-opinion differentiation and mass opinion have been reported. Mitchell et al. (2018) produced an exploratory, descriptive analysis of Americans’ levels of success at fact-opinion differentiation. Subsequent research has contributed analyses with data from college students (Bak, 2022; Peterson, 2019),4Peterson’s study was an undergraduate senior honor’s thesis at the University of Illinois that was completed under the supervision of the current paper’s second author. As work on the current paper was completed, two new studies were reported; see Goldberg and Marquart (2024) and Graham and Yair (2024). patterns in the presentation of factual and opinion content on cable news programs (Meacham, 2020), and the impact of linguistic structure on readers’ success in determining whether textual claims are statements of fact or opinion (Kaiser & Wang, 2021). Building on this literature, we introduce data from twelve fact-opinion items included on a 2019 survey and conduct multivariate analyses expanding on the findings in Mitchell et al. (2018).
Across ten items, Mitchell et al. (2018) found that respondents had an overall success rate of 69.3%. That percentage risks being interpreted unduly positively. The items are dichotomous—the only choice options are that the statement being evaluated is a statement of fact or a statement of opinion—meaning, on average, a respondent guessing randomly would have scored 50%. To acknowledge this 50% baseline, we describe success rates in terms of respondents’ levels of improvement over chance. Our reassessment of the prevalence of faulty fact-opinion differentiation suggests that judgmental failures are widespread. On our survey, nearly half of respondents (45.7%) exhibited no improvement over chance.5The mean on our 12-item battery is 59.9%. Ten of our twelve items are those used in Mitchell et al. (2018). On our survey, the mean on these is 64.6%.
Two processes can generate errors in fact-opinion differentiation. One, labeled here as unbiased error, is largely unsystematic. Some individuals struggle with the task in a manner that leads them toward random guessing. Mitchell et al. (2018) show via crosstabs that fact-opinion differentiation success increases with political awareness and media savvy. Consistent with that, our multivariate analyses reveal that accuracy increases and unsystematic error decreases as a function of four components of political sophistication: civics knowledge, current events knowledge, cognitive ability, and education.6Although empirical representations of political sophistication are often narrow, theoretical conceptualizations include multiple dimensions. Luskin (1990) effectively bridges the conceptual and operational realms. His discussion of the roles of intelligence, education, and exposure to political information motivate our focus on cognitive ability, education, civics knowledge, and current events knowledge. In our conceptualization, unbiased error is a residual category that comprises incorrect responses that reflect neither accuracy nor error rooted in partisan bias. Although most such responses likely emanate from random guessing, some may not. For instance, a respondent who believes all politicians always lie would record systematic rather than random errors on our items, but those errors would not reflect partisan preference. Put differently, our terminology of unbiased error is shorthand for “incorrect responses resulting from any cause other than partisan bias.”
The second process, which we label as partisan bias, is systematic. Mitchell et al. (2018) provide preliminary evidence that partisans are more likely to see claims that align with their views as being statements of fact and claims inconsistent with their views as being statements of opinion. Expanding on this, we focus not on partisan identity but instead on affective partisan polarization. Iyengar and Westwood (2015) define affective polarization as “the tendency of people identifying as Republicans or Democrats to view opposing partisans negatively and co-partisans positively” (p. 691). Our analyses indicate that affective polarization increases the response tendency observed by Mitchell et al. (2018). As affective partisan polarization intensifies, respondents become more likely to answer that their party has the facts on its side while the other party has opinions. Hence, respondents do not merely misconstrue the empirical world. Instead, they systematically reconstruct it so that it aligns with their partisan orientations. Democrats and Republicans do not just disagree on the facts; they disagree on what facts are. This partisan bias is quite powerful. When success at fact-opinion differentiation increases as a function of civics knowledge, current events knowledge, cognitive ability, and education, it mostly does so by converting unbiased error to correct response; partisan error largely persists. Thus, faulty fact-opinion differentiation not only contributes to political misinformation but also does so in a manner that activates partisan biases, thereby amplifying polarization in perceptions of American politics and society.
We examined the phenomenon’s general contours through development and application of a novel multivariate analytical strategy. Specifically, we used a carefully constructed measure of fact-opinion differentiation success, one that enabled us to distinguish between correct responses, unbiased errors, and errors resulting from partisan bias. We then examined these outcomes using a multinomial estimation strategy. Analyses proceeded in three steps. First, we assessed the extent to which people have an easy or difficult time distinguishing between statements of fact and opinion. Second, with focus on four components of political sophistication, we considered what factors may contribute to successful fact-opinion differentiation. Third, where errors occurred, their properties were examined. Errors may emanate from nonpolitical processes such as blind guessing, but systematic processes rooted in partisan bias may also shape what people view as being included in or excluded from the empirical world.
Collectively, our analyses call attention to faulty fact-opinion differentiation and show that errors rooted in partisan bias are especially persistent. For media organizations and public interest groups committed to combatting misinformation, our analyses suggest a second form of action may be needed. Fact checks strive to be curative, but they are not preventative. Lessons in fact-opinion differentiation, lessons such as those in the Abbott Elementary episode described above, strive to be preventative. Beyond being careful not to blur the line between fact and opinion themselves, media organizations can also explain and reiterate the difference between statements of fact and statements of opinion to their viewers and readers. They can help their audiences to appreciate the difference between statements of fact and statements of opinion and to become more adept at distinguishing between the two forms of claims. With more such efforts by journalists to highlight the fundamental distinction between statements of fact and statements of opinion, news consumers may be inoculated against reflexively concluding that favored claims are always fact-based and disfavored ones are only opinions.
Findings
Finding 1: Success rates at fact-opinion differentiation are low.
Respondents on the 2019 survey rated twelve claims, including ten drawn from Mitchell et al. (2018), as statements of fact or statements of opinion. Results are shown in Table 1. Parallel results for the ten Pew items examined by Mitchell et al. (2018) are also reported. Across all items, accuracy ranges between 26% and 80% and averages 64%.
Question | 2019 YouGov | 2018 Pew |
1. Immigrants who are in the U.S. illegally have some rights under the Constitution (statement of fact). | 54 | 54 |
2. ISIS lost a significant portion of its territory in Iraq and Syria in 2017 (statement of fact). | 73 | 68 |
3. President Barack Obama was born in the United States (statement of fact). | 70 | 774. |
4. Health care costs per person in the U.S. are the highest in the developed world (statement of fact). | 78 | 76 |
5. Spending on Social Security, Medicare, and Medicaid make up the largest portion of the U.S. federal budget (statement of fact). | 51 | 57 |
6. The Earth is between 5,000 and 10,000 years old (statement of fact). | 26 | n/a |
7. Immigrants who are in the U.S. illegally are a very big problem for the country today (statement of opinion). | 59 | 68 |
8. Abortion should be legal in most cases (statement of opinion). | 73 | 80 |
9. Increasing the federal minimum wage to $15 an hour is essential for the health of the U.S. economy (statement of opinion). | 69 | 73 |
10. Government is almost always wasteful and inefficient (statement of opinion). | 61 | 71 |
11. Democracy is the greatest form of government (statement of opinion). | 58 | 69 |
12. Diversity helps make America great (statement of opinion). | 48 | n/a |
Mean | 59.9 | 69.3 |
Note: Pew data are from Mitchell et al. (2018). For the ten common items, the YouGov mean is 64.6. |
Two items warrant mention. First, as noted by Firey (2018),7Firey (2018) rejects the entire enterprise of fact-opinion differentiation. He makes multiple errors in doing so, such as conflating “statements of fact” with “facts” and misunderstanding the concept of statistical significance. These errors understandably leave Firey confused about basic matters, such as how a statement of fact can be factually incorrect. item 2 perhaps could be categorized as a statement of opinion because the phrase “a significant portion” is subjective. We repeated Pew’s coding on our survey, and solid majorities on both surveys rated the item as a statement of fact. In retrospect, we would categorize the statement as borderline (see note 4 above). By the end of 2017, ISIS had lost 95% of its territory (Wilson Center, 2019). Because such an overwhelming loss would be difficult to deem insignificant, we retain the item as a statement of fact for present purposes.8A total of 129 respondents (5.2% of the sample) answered the other eleven items correctly. Of these, only seven (5.4%, or 0.3% of the full sample) coded item 2 as opinion. This indicates that respondents who were very good at fact-opinion differentiation overwhelmingly—but not quite unanimously—viewed item 2 as a statement of fact.
Item 6 is a statement of fact that is factually incorrect. Only 26% of respondents answered this correctly. We suspect many of the errors may reflect thinking to the effect of “people who claim that are wrong, but they’re entitled to their opinion.” Such rationalizations potentially enable misinformation to survive by reclassifying factual error as opinion.
Because the fact-opinion items are dichotomous, 2019 data are recoded for multivariate analyses. Using 50% as the baseline success rate for random guessing, the mean level of improvement over chance is 26.9%. Error is decomposed into error reflecting partisan bias (28.5%) and unbiased residual error (44.6%).9As noted above, unbiased error is a residual category formed after we accounted for accurate response and partisan error. Hence, unbiased, in this case, means an absence of partisan error, but it does not rule out other systematic processes. For multivariate analyses, the dependent variable is a proportion, and there are three categories or outcomes: accurate response, partisan error, and unbiased error. Models were estimated using grouped-data multinomial logistic regression.10Grouped-date multinomial logit is similar to multinomial logit except that the dependent variable is measured as three or more proportions that sum to 1 rather than with three or more 0-1 indicators that sum to 1. In multinomial logit with three choice options, the dependent variable for a given case might be coded 0, 1, 0; in a grouped-data, or proportions, scenario, the coding might be 0.27, 0.51, 0.22. For a prior application of grouped-data multinomial logit in research on civics knowledge, see Mondak (2000).
Finding 2: Success at fact-opinion differentiation increases with civics knowledge, current events knowledge, cognitive ability, and education.
Consistent with Mitchell et al.’s (2018) finding that success at fact-opinion differentiation improves with political awareness, our multivariate analyses revealed that higher levels of civics knowledge, current events knowledge, cognitive ability, and education correspond with greater accuracy. This is seen in the green lines in Figure 1, which depict predicted levels of accurate response. These lines all slope modestly upward as the political sophistication variables increase in value. Figure 1 also shows that the variables operate mostly by reducing unbiased error (the blue lines, which provide predicted levels of unbiased error, have significant downward slopes). In contrast, effects on partisan error are minimal (the red lines that show estimates for partisan error slope slightly upward for civics knowledge and current events knowledge and very slightly downward for cognitive ability and education).
Finding 3: Affective partisan polarization produces systematic partisan error in fact-opinion differentiation.
Affective partisan polarization was operationalized as the difference in average feeling thermometer scores for Democrats (Obama, H. Clinton, Pelosi, Schumer) and Republicans (Trump, Pence, Cruz, McConnell), with separate 0 to 100 variables used to represent pro-Democratic and pro-Republican polarization. Figure 2 shows that partisan polarization leads individuals to assess political statements differently depending upon whether the statements align with partisan preferences. For biased partisans, the tendency is to perceive statements amenable to their political interests as statements of fact and claims inconsistent with their interests as statements of opinion (the red lines, denoting partisan error, slope significantly upward as affective partisan polarization increases). This effect is more pronounced for Republicans than for Democrats. Moreover, increasing levels of affective partisan polarization decrease accurate responses only for Republicans.
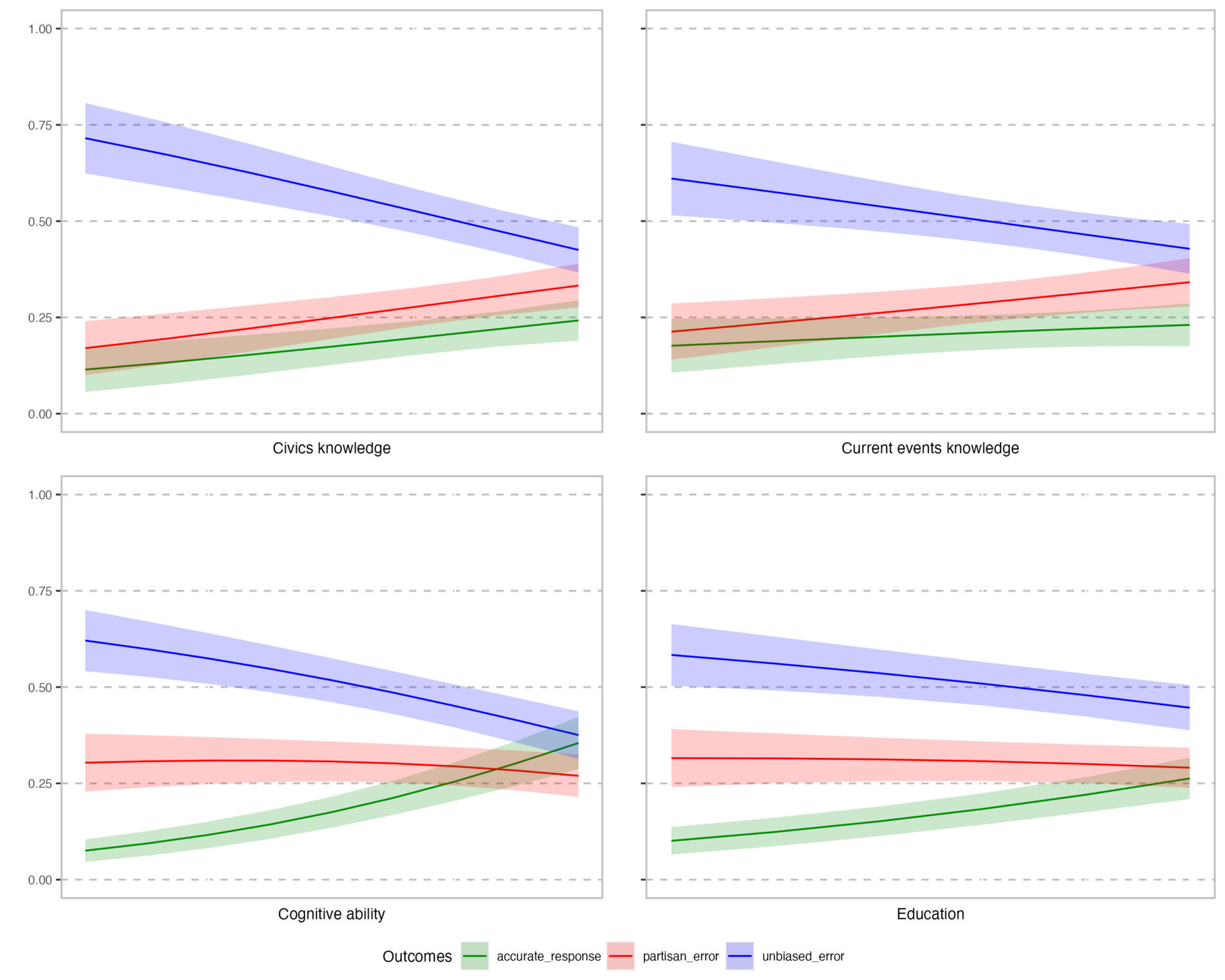
Methods
Data are from a national online survey we designed. The survey was fielded by YouGov from March 9 to March 14, 2019. There are 2,500 respondents.
The dependent measure is a proportions variable with three outcomes: accurate response, partisan error, and unbiased error. Accurate response is operationalized as improvement over chance. With twelve dichotomous fact-opinion items, accuracy ranges from 0 (six or fewer items were answered correctly) to 1 (all items were answered correctly).11Accuracy was set to 0 for respondents who answered six or fewer items correctly because their performance provided no indication of accuracy. For respondents who answered seven or more questions correctly, the formula for calculating accuracy scores was (number of correct responses – number of incorrect responses) / 12. Partisan bias exists when respondents answer that claims more aligned with their party are facts and claims more aligned with the opposing party are opinions. The items were structured such that partisan preferences coincided with three statements of fact and three statements of opinion for each party.12Items coded as more aligned with Democrats’ interests are 1, 3, 4, 8, 9, and 12. Because every partisan need not agree with all twelve of our classifications, our test of the possible impact of affective partisan polarization on partisan bias in fact-opinion differentiation is inherently conservative. Therefore, respondents exhibited partisan bias—and committed partisan error—if seven or more of their answers favored their own political party. Thus, partisan bias ranged from 0 (six or fewer answers favor the respondent’s party) to 1 (all 12 answers favor the respondent’s party). Unbiased error was a residual category that captured errors in fact-opinion differentiation that did not arise from favoritism toward one’s own party: 1 – (accurate response + partisan bias).13The measures of accurate response and partisan bias provide maximum estimates. For accurate response, for instance, a respondent’s maximum level of accuracy cannot exceed the number of items the respondent answered correctly, but respondents who guess randomly may get seven or more answers correct by chance, meaning our scale would credit them with a level of accurate response greater than zero. It follows that our measure of unbiased error provides a minimum or conservative estimate. Despite this, unbiased error is the modal category on our dependent variable, providing further evidence that Americans struggle with fact-opinion differentiation. For both accurate response and partisan bias, the observed means are well over double what they would be from chance alone. Our findings corroborate that these classifications capture accuracy and partisan bias, respectively: individuals with high levels of education, analytical ability, civics knowledge, and current events knowledge answered more questions correctly, whereas null results would have been expected were all responses the products of random guessing. Likewise, respondents with high levels of affective partisan polarization gave more answers favoring their party.
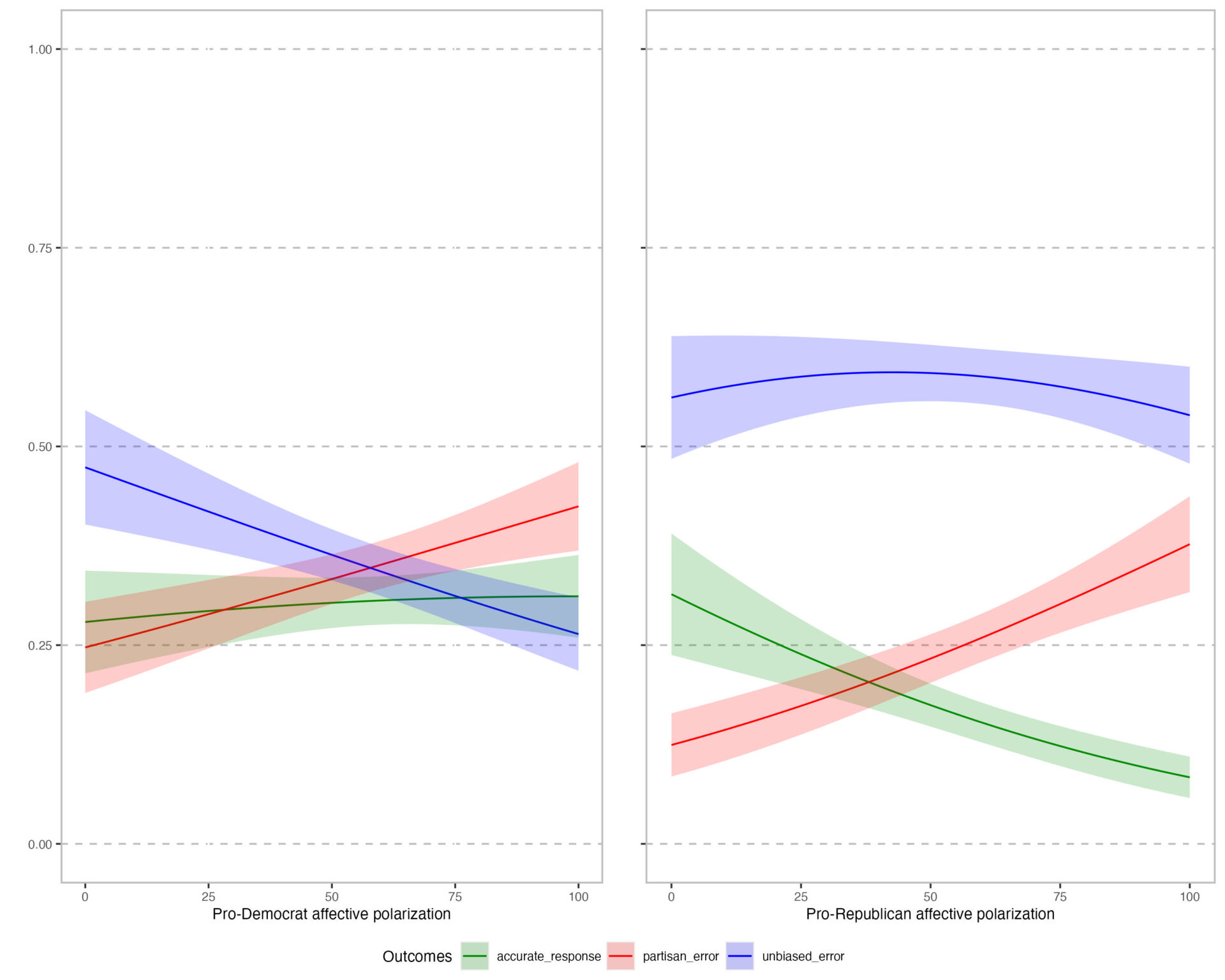
Multivariate models include controls for age and partisanship. Political sophistication is represented with four variables: civics knowledge, current events knowledge, cognitive ability, and education. Pro-Democratic and pro-Republican affective partisan polarization is operationalized by differencing feeling thermometer scores for four Democrats (Obama, H. Clinton, Pelosi, Schumer) and four Republicans (Trump, Pence, Cruz, McConnell). Descriptive statistics for all variables are reported in the Appendix.
Because the dependent measure is a proportions variable, models are estimate using grouped-data multinomial logistic regression (see Greene, 2016). We used the nnet package in R. Full results are reported in the Appendix.
Topics
Bibliography
Bak, H. (2022). College students’ fake news discernment: Critical thinking, locus of control, need for cognition, and the ability to discern fact from opinion [Doctoral dissertation, Florida State University]. DigiNole: FSU’s Digital Repository. https://diginole.lib.fsu.edu/islandora/object/fsu%3A875318/datastream/PDF/view
Firey, T. (2018). Facts, opinions, and the Pew Research Center’s pseudoscience. Econlib – The Library of Economics and Liberty. https://www.econlib.org/facts-opinions-and-the-pew-research-centers-pseudoscience
Goldberg, A. & Marquart, F. (2024). “That’s just, like, your opinion” – European citizens’ ability to distinguish factual information from opinion. Communications. Advance online publication. https://doi.org/10.1515/commun-2023-0076
Graham, M. H., & O. Yair (2024). Less partisan but no more competent: Expressive responding and fact-opinion discernment. [Manuscript submitted for publication]. https://m-graham.com/papers/GrahamYair_FactOpinion.pdf
Greene, W. H. (2018). NLOGIT version 6 reference guide. Econometric Software, Inc. https://pages.stern.nyu.edu/~wgreene/DiscreteChoice/Software/manual/NLOGIT%206%20Reference%20Guide.pdf
Iyengar, S., & Westwood, S. J. (2015). Fear and loathing across party lines: New evidence on group polarization. American Journal of Political Science, 59(3), 690–707. https://doi.org/10.1111/ajps.12152
Kaiser, E., & Wang, C. (2021). Packaging information as fact versus opinion: Consequences of the (information-) structural position of subjective adjectives. Discourse Processes, 58(7), 617–641. https://doi.org/10.1080/0163853X.2020.1838196
Luskin, R. C. (1990). Explaining political sophistication. Political Behavior, 12(4), 331–360. https://doi.org/10.1007/BF00992793
Meacham, M. C. (2020). I think; therefore, it’s news! The convergence of opinions versus facts on cable news networks. Review of Arts and Humanities, 9(1), 1–8. https://doi.org/10.15640/rah.v9n1a1
Mitchell, A., Gottfried, J., Barthel, M., & Sumida, N. (2018). Distinguishing between factual and opinion statements in the news. Pew Research Center. https://www.pewresearch.org/journalism/2018/06/18/distinguishing-between-factual-and-opinion-statements-in-the-news/
Mondak, J. J. (2000). Reconsidering the measurement of political knowledge. Political Analysis, 8(1), 57–82. https://doi.org/10.1093/oxfordjournals.pan.a029805
Murphy, G., de Saint Laurent, C., Reynolds, M., Aftab, O., Hegarty, K. Sun, Y. & Greene, C. M. (2023). What do we study when we study misinformation? A scoping review of experimental research (2016-2022). Harvard Kennedy School (HKS) Misinformation Review, 4(6). https://doi.org/10.37016/mr-2020-130
Murphy, M. (Writer), & Einhorn, R. (Director). (2023, March 8). Teacher appreciation (Season Two, Episode 18) [Television series episode]. In Q. Brunson (Creator), Abbott Elementary. Fifth Chance; ABC.
Palm Beach State College (n.d.). Fact or opinion? – Tutor hints. https://www.palmbeachstate.edu/slc/Documents/fact%20or%20opinion%20hints.pdf
Peterson, V. J. (2019). The ramifications of social media: Differentiating truths from falsities and facts from opinions [Senior honor’s thesis, University of Illinois]. https://files.webservices.illinois.edu/8689/valeriepetersonthesis.pdf
Rogers, T., Leys, M., & Pearson, D. (1985, March 7–9). Teaching a reading comprehension skill: Fact and opinion [Paper presentation]. Annual Meeting of the Illinois Reading Council (Peoria, IL, United States). https://files.eric.ed.gov/fulltext/ED258140.pdf
Schell, L. M. (1967). Distinguishing fact from opinion. Journal of Reading, 11(1), 5–9. https://www.jstor.org/stable/40009335
Thaneerananon, T., Triampo, W., & Nokkaew, A. (2016). Development of a test to evaluate students’ analytical thinking based on fact versus opinion differentiation. International Journal of Instruction, 9(2), 123–138. https://doi.org/10.12973/iji.2016.929a
Wilson Center (2019, October 28). Timeline: The rise, spread, and fall of the Islamic State. https://www.wilsoncenter.org/article/timeline-the-rise-spread-and-fall-the-islamic-state
Walter, N. & Murphy, S. (2018). How to unring the bell: A meta-analytic approach to correction of misinformation. Communication Monographs, 85(3), 423–441. https://doi.org/10.1080/03637751.2018.1467564
Weddle, P. (1985). Fact from opinion. Informal Logic, 7(1), 19–26. https://doi.org/10.22329/il.v7i1.2698
Funding
Financial support was provided by the Benson Fund at the University of Illinois.
Competing Interests
The authors report no conflicts or competing interests.
Ethics
This project was approved by the Institutional Review Board at the University of Illinois (approval 19430). Survey respondents provided informed consent.
Copyright
This is an open access article distributed under the terms of the Creative Commons Attribution license, which permits unrestricted use, distribution, and reproduction in any medium, provided that the original author and source are properly credited.
Data Availability
All materials needed to replicate this study are via the Harvard Dataverse: https://doi.org/10.7910/DVN/KG5KPI
Acknowledgements
Work on this project was begun while the second author was a senior research fellow at the Center for the Study of Democratic Institutions at Vanderbilt University.