Peer Reviewed
Cross-platform disinformation campaigns: Lessons learned and next steps
Article Metrics
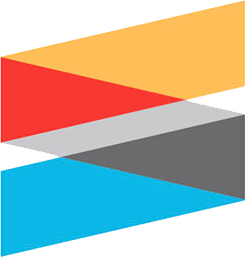
36
CrossRef Citations
Altmetric Score
PDF Downloads
Page Views
We conducted a mixed-method, interpretative analysis of an online, cross-platform disinformation campaign targeting the White Helmets, a rescue group operating in rebel-held areas of Syria that have become the subject of a persistent effort of delegitimization. This research helps to conceptualize what a disinformation campaign is and how it works. Based on what we learned from this case study, we conclude that a comprehensive understanding of disinformation requires accounting for the spread of content across platforms and that social media platforms should increase collaboration to detect and characterize disinformation campaigns.
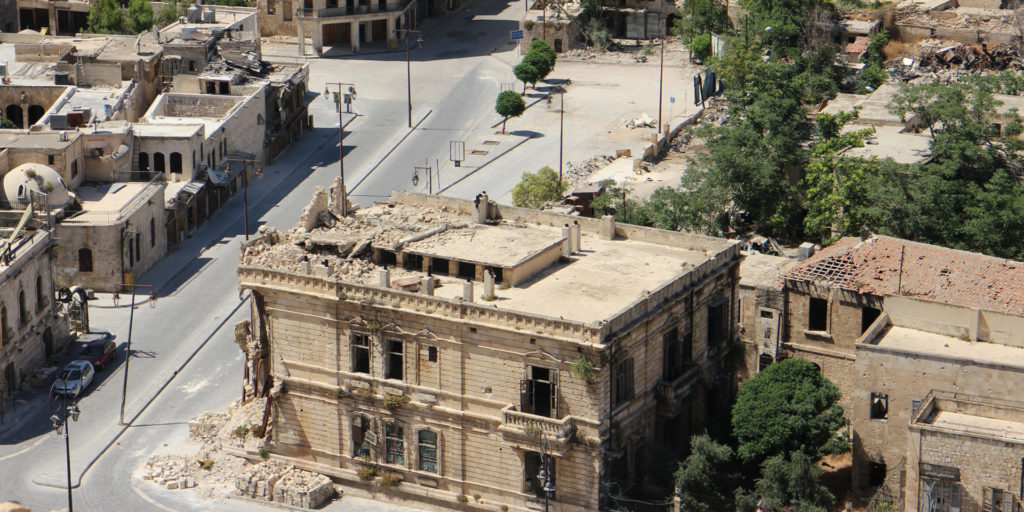
Research Questions
- How do disinformation campaigns work across online platforms to achieve their strategic goals?
- How do governments and other political entities support disinformation campaigns?
Essay Summary
- We adopted a mixed-method approach to examine digital trace data from Twitter and YouTube;
- We first mapped the structure of the Twitter conversation around White Helmets, identifying a pro-White Helmets cluster (a subnetwork of accounts that retweet each other) and an anti-White Helmets cluster;
- Then, we compared activities of the two separate clusters, especially how they leverage YouTube videos (through embedded links) in their efforts;
- We found that, on Twitter, content challenging the White Helmets is much more prevalent than content supporting them;
- While the White Helmets receive episodic coverage from “mainstream” media, the campaign against them sustains itself through consistent and complementary use of social media platforms and “alternative” news websites;
- Influential users on both sides of the White Helmets Twitter conversation post links to videos, but the anti-White Helmets network is more effective in leveraging YouTube as a resource for their Twitter campaign;
- State-sponsored media such as Russia Today (RT) support the anti-White Helmets Twitter campaign in multiple ways, e.g., by providing sourced content for articles and videos and amplifying the voices of social media influencers.
Implications
This paper examines online discourse about the White Helmets, a volunteer rescue group that operates in rebel (anti-regime) areas of Syria. The White Helmet’s humanitarian activities, their efforts to document the targeting of civilians through video evidence, and their non-sectarian nature (that disrupted regime-preferred narratives of rebels as Islamic terrorists) put the group at odds with the Syrian government and their allies, including Russia (Levinger, 2018). Consequently, they became a target of a persistent effort to undermine them.
Disinformation can be defined as information that is deliberately false or misleading (Jack, 2017). Its purpose is not always to convince, but to create doubt (Pomerantsev & Weiss, 2014). Bittman (1985) describes one tactic of disinformation as “public relations . . . in reverse” meant to damage an adversary’s image and undermine their objectives. We argue (Starbird et al., 2019) that disinformation is best understood as a campaign—an assemblage of information actions—employed to mislead for a strategic, political purpose. Prior research (Levinger, 2018; Starbird et al., 2018) and investigative reporting (Solon, 2017; di Giovanni, 2018) have characterized the campaign against the White Helmets as disinformation, due to its connection to Russia’s influence apparatus, its use of false and misleading narratives to delegitimize the group, and its function to create doubt about their evidence documenting atrocities perpetrated by the Syrian regime and their Russian allies.
This research examines “both sides” of the White Helmets discourse—exploring how the White Helmets promote their work and foster solidarity with online audiences through their own social media activity and through episodic attention from mainstream media, and examining how the campaign against the White Helmets attempts to counter and delegitimize their work through strategic use of alternative and social media. We do not make any claims about the veracity of specific pieces of content or specific narratives shared by accounts on either side of this conversation. However, we do highlight how the campaign against the White Helmets reflects emerging understandings of disinformation in this context (Pomerantsev & Weiss, 2014; Richey, 2018; Starbird et al., 2019).
Implications for researchers
Prior work on online disinformation has tended to focus on activities on a single social media platform such as Twitter (e.g., Arif et al., 2018; Broniatowski et al., 2018; Wilson et al., 2018, Keller et al., 2019; Zannettou et al., 2019), or YouTube (e.g., Hussain et al., 2018), or in the surrounding news media ecosystem (e.g., Starbird, 2017; Starbird et al., 2018). Here, we look cross-platform, demonstrating how Twitter and YouTube are used in complementary ways, in particular by those targeting the White Helmets.
Tracing information across platforms, our findings show materials produced by state-sponsored media become embedded in content (videos, articles, tweets) attributed to other entities. Aligned with emerging practices among researchers and analysts (e.g., Diresta & Grossman, 2019; Brandt & Hanlon, 2019), our findings suggest that to understand the full extent of disinformation campaigns, researchers need to look across platforms—to trace and uncover the full trajectories of the information-sharing actions that constitute disinformation campaigns.
Implications for social media companies
Researchers have begun to look beyond orchestrated campaigns to consider the participatory nature of online propaganda and disinformation (Wanless and Berk, 2017). In this view, the work of paid agents becomes entangled with the activities of “unwitting crowds” of online participants (Starbird et al., 2019). Here, we reveal some of those entanglements, demonstrating how state-sponsored media play a role in shaping a disinformation campaign, providing content and amplification for voices aligned with their strategic objectives. And, expanding upon prior research on online political organizing (Lewis, 2018; Thorson et al., 2013), we show how these participatory campaigns take shape across social media platforms.
This highlights the need for platforms to work together to identify disinformation campaigns. Social media companies are taking action to detect malicious content on their platforms, but those determinations often stay in their silos. The sharing of data pertaining to disinformation campaigns remains largely informal and voluntary (Zakrzewski, 2018). The establishment of more formal channels would aid the detection of cross-platform campaigns. However, such data sharing raises concerns about protecting consumer privacy and issues of surveillance of users across platforms, particularly if there is no consensus as to what disinformation is. Therefore, the first step is for social media companies to agree upon what constitutes a disinformation campaign—and our hope is that this research contributes to that debate.
Findings
Finding 1: The structure of the White Helmets discourse has two clear clusters of accounts—a pro-White Helmets cluster that supports the organization and an anti-White Helmets cluster that criticizes them.
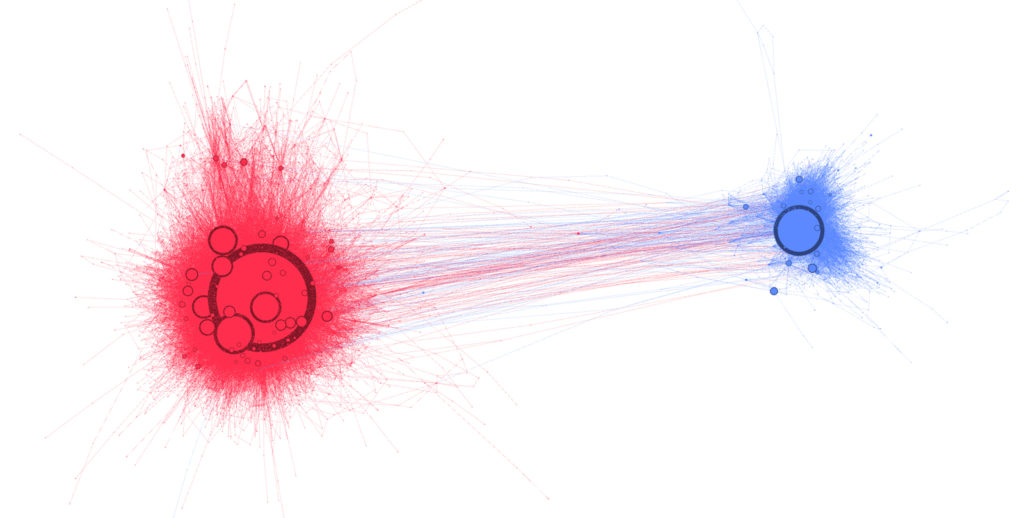
Analyzing the English-language White Helmets conversation on Twitter (see Methods), we detected two distinct “clusters” or sub-networks of accounts that share information with each other through retweets (Figure 1): one cluster that was highly critical of the White Helmets (the anti-White Helmets cluster, red, on the left) and another cluster that was predominantly supportive of the group (the pro-White Helmets cluster, blue, on the right).
The Blue cluster coalesced around the Twitter activity of the White Helmets themselves. The organization’s official English-language account (@SyriaCivilDef) was the most retweeted account (and largest node) in Blue. @SyriaCivilDef posted 444 “white helmets” tweets, including the example below:
White Helmets firefighter teams managed to extinguish massive fires, that broke out after surface to surface missile carrying cluster bombs hit civilians’ farms south of town of #KhanShaykhun in #Idlib countryside and were controlled without casualties. <image of a bloodied victim>
The White Helmets used their Twitter account to promote their humanitarian work and to document civilian casualties—including specifically calling out the impacts of the Syrian regime’s shelling and Russian air raids. Other accounts in the Blue cluster participated by retweeting the White Helmets’ account (29,375 times) and sharing other content supportive of the group.
Content within the Red cluster was acutely and consistently critical of the White Helmets. The example tweet below was posted by the most highly retweeted account in the data, @VanessaBeeley:
@UKUN_NewYork @MatthewRycroft1 @UN While funding barbarians #WhiteHelmets? You are nothing but vile hypocrites: <link to article in alternative news website, 21stCenturyWire>
Beeley is a self-described journalist whose work focuses almost exclusively on the White Helmets. Her tweet referred to the White Helmets as “barbarians” and criticized the United Nations for funding them. It cited an article she wrote, published on the alternative news website, 21st CenturyWire.
Almost all of the tweets in the Red cluster were similarly critical of the White Helmets, including claims that the White Helmets are terrorists, that they stage chemical weapons attacks, and that they are a “propaganda construct” of western governments and mainstream media. Taken together, these narratives attempted to foster doubt in the White Helmets’ messages by damaging their reputation, revealing the kind of “public relations in reverse” strategy that Bittman (1985) described as a tactic of disinformation campaigns.
We provide more details about our content analysis, including example tweets and a list of prominent narratives from the clusters, in Appendix D.
Finding 2: On Twitter, efforts to undermine and discredit the White Helmets are more active and prolific than those supporting the group.
As illustrated by the size of the clusters (Figure 1) and tweet counts within them (Table 1), anti-White Helmets voices dominated the Twitter conversation—the Red cluster contained more accounts (38% more) and produced three times as much Twitter content.
Cluster | Number Accounts | Total Tweets | Tweets per Account | Number Original Tweets | Number Retweets | % Retweets | % incl URL |
Blue | 70670 | 208265 | 2.95 | 27733 | 180532 | 86.7% | 22.7% |
Red | 97440 | 633762 | 6.50 | 123167 | 510595 | 80.6% | 37.0% |
Accounts in the anti-White Helmets cluster were also more committed to tweeting about the White Helmets. They posted more tweets per account. Temporal analysis (Appendix C) suggests that Red-cluster tweeters are more consistent in their “white helmets” tweeting over time. At the same time, the Blue cluster engaged more episodically, typically in alignment with mainstream news cycles.
Finding 3. The pro-White Helmets discourse was shaped by the White Helmets’ self-promotion efforts and mainstream media, while the anti-White Helmets discourse was shaped by non-mainstream media and Russia’s state media apparatus.
Analysis of the top influencers in terms of retweets (Appendix G) reveals the pro-White Helmets discourse to be largely shaped by the White Helmets themselves. @SyriaCivilDef is by far the most retweeted account in the pro-White Helmets cluster. The list of top influencers in Blue includes accounts of three other White Helmets members as well. Journalists and accounts associated with mainstream media are also prominent. In particular, journalist @oliviasolon appears as the second-most influential account, gaining visibility after publishing an article in the Guardian asserting the White Helmets were the targets of a disinformation campaign (Solon, 2017).
The list of influencers in the Red cluster tells a different story. The most retweeted account is @VanessaBeeley, who received 63,380 retweets—more than twice as many as @SyriaCivilDef. @VanessaBeeley was also more active than the @SyriaCivilDef account, posting almost five times as many “white helmets” tweets (2043). The list of influential accounts in Red includes six other journalists, contributors, and outlets associated with “alternative” or non-mainstream media. It also features three accounts associated with the Russian government and one state-sponsored media outlet (@RT_com).
Finding 4: Both clusters feature dedicated accounts, many operated by “sincere activists.”
Both clusters also feature accounts—among their influencers and across their “rank and file”—that appear to be operated by dedicated online activists. Activist accounts tend to tweet (and retweet) more than other accounts. We can view their activities as the “glue” that holds together the structure we see in the network graph.
Interestingly, dedicated activist accounts in Red play a larger role in sustaining the campaign against the White Helmets than activist accounts in Blue play in defending them. A k-core analysis of the two retweet-network clusters (Appendix H) reveals that the Red cluster retains a much larger proportion of its graph at higher k values. In other words, a larger core group of accounts in Red has many mutual connections (retweet edges) between themselves. At k=60, while the Blue cluster has disappeared, the Red cluster still has 653 accounts, including most of the highly retweeted accounts.
This supports a view of the pro-White Helmets cluster taking shape around the activities of the organization and small group of supportive activists, with occasional attention from mainstream media and their broader, but less engaged audience. Meanwhile, the anti-White Helmets campaign is sustained by a relatively large and inter-connected group of dedicated activists, journalists, and alternative and state-sponsored media. The entanglement of sincere activists, journalists, and information operations complicates simplistic views of disinformation campaigns as being the work of “bots” and “trolls” (Starbird et al., 2019).
Finding 5: YouTube is the most frequently linked-to website from the White Helmets conversation on Twitter, predominantly by accounts in the anti-White Helmets cluster.
About a third of all tweets in our data contained a URL to a domain (or website) outside of Twitter. Though many linked to news media domains, several social media platforms appear among the most-tweeted domains in each cluster (Appendix I).
YouTube is, by far, the most cited social media domain. Due to how Twitter displays YouTube content, a YouTube link would have appeared as a playable video embedded in the tweet. In this way, content initially uploaded to the YouTube platform can be brought in (cross-posted) as a resource for a Twitter conversation—or information campaign.
Comparing patterns of cross-posting, we find that the anti-White Helmets cluster introduces YouTube content in their tweets far more often (Table 2). 43,340 tweets from the Red cluster contain links to YouTube content, compared to 2225 tweets from the Blue. More anti-White Helmets accounts are doing this cross-platform video-sharing (10 times as many), and they also receive more retweets (overall and proportionally) for those videos.
Cluster | Number tweets | Number Original Tweets | Number (%) Total Tweets w/ URL to YouTube | Number (%) Original Tweets w/ URL to YouTube | Number (%) Total Tweets w/ Video Embed in Twitter | Number (%) Original Tweets w/ Video Embed in Twitter |
Blue | 208265 | 27733 | 2225(1.07%) | 631(2.28%) | 37,614(18.1%) | 3104(11.2%) |
Red | 633762 | 123167 | 43,340(6.84%) | 11,184(9.08%) | 89,717(14.2%) | 7610(6.2%) |
Accounts in the Red cluster also shared a larger number of videos: 1604 YouTube videos were cross-posted onto Twitter from anti-White Helmets accounts, compared to only 254 from pro-White Helmets accounts.
Finding 6: Accounts in the pro-White Helmets cluster primarily shared video content using Twitter’s native video-embedding functionality.
Tweets from the pro-White Helmets cluster included video content at similar rates to the anti-White Helmets cluster, but rather than cross-posting from YouTube, they primarily relied on Twitter’s native video-sharing functionality (Table 2). These Twitter-embedded videos differ from cross-posted YouTube videos in that they are shorter (limited to 140 seconds), and they automatically play within Twitter.
@SyriaCivilDef repeatedly used Twitter’s video-embedding functionality. 17.4% (33) of their original tweets contained a video embedded through Twitter. These videos typically featured the White Helmets responding to Syrian regime attacks. They were widely shared, both through retweets and through other accounts, embedding them in their own original tweets. Conversely, only three of @SyriaCivilDef’s original tweets contained a YouTube video.
The White Helmets organization did have a YouTube presence, operating several YouTube channels1Channels on YouTube are similar to accounts on Twitter—a user uploads videos to their channel., and these channels were utilized—to some extent—by pro-White Helmets Twitter accounts. Eight YouTube channels with White Helmet branding were linked-to from tweets in our data. Together, they hosted 77 different cross-posted videos. However, the number of tweets and retweets of these videos was small—less than 1000 total. The YouTube engagements of these videos were relatively few as well—109,225 total views.
Finding 7: The Anti-White Helmets cluster is more effective in leveraging YouTube as a resource for their Twitter campaign.
Accounts in the anti-White Helmets cluster shared 43,340 total tweets, linking to 1604 distinct YouTube videos, hosted by 641 channels. YouTube videos cross-posted by accounts in Red were longer and had more views and likes (on YouTube) than videos cross-posted by Blue.
2762 Red accounts shared a YouTube video through an original tweet. The most influential cross-poster was journalist @VanessaBeeley. Examining her activity reveals a set of techniques for distributing content across platforms, networks, and channels. In our Twitter data, she posted 49 different YouTube videos through 77 original tweets and received 7644 retweets for those cross-posts. Like her other tweet content (Appendix D), the YouTube videos she shared were consistently critical of the White Helmets across a wide range of narratives. Her cross-posted videos were hosted on 13 different YouTube channels, including RT. But the majority of her cross-posted videos (33) were sourced from her own YouTube channel. Other accounts were cross-posting from her channel too: in our data, 72 different videos from the Vanessa Beeley YouTube channel were cross-posted by 446 distinct Twitter accounts—through 1304 original tweets, which were retweeted 8325 times. On YouTube, these videos have a combined duration of 458 minutes (more than 7 hours) and have been viewed 381,420 times. Here again, her impact on the White Helmets conversation was substantially more (in terms of production and exposure) than the activity of the White Helmets themselves.
Finding 8: Tracing information trajectories across platforms demonstrates how Russia’s state-sponsored media shapes the anti-White Helmets campaign by providing content and amplifying friendly voices.
Accounts from the Russian government authored three of the most-tweeted tweets and were among the top-20 most retweeted accounts in the anti-White Helmets cluster (Appendix D and G). Websites associated with Russia’s state media apparatus—including Russia Today (RT) and Sputnik News—were among the most highly cited domains in anti-White Helmets cluster (Appendix I). RT also operates a YouTube channel that was highly tweeted within the Red cluster. Thus, a high-level view shows that Russian state-sponsored media are information resources for the anti-White Helmets campaign. Tracing information trajectories across YouTube, Twitter, and non-mainstream news domains reveals how Russia’s media apparatus shaped the anti-White Helmets campaign in other, more subtle ways.
The information work of anti-White Helmets influencer Vanessa Beeley provides a window into this shaping. Through her Twitter account, Beeley posted 18 tweets linking to RT and 11 tweets linking to SputnikNews. She also cross-posted nine videos from RT’s YouTube channel to Twitter. Within the videos she cross-posted from her own YouTube channel, there is content sourced from videos produced by RT and InTheNOW (an online-first outlet affiliated with RT). Beeley also appeared as an interviewee on RT-affiliated programs, which became source material for videos hosted on her channel and on RT. She embedded RT videos within her articles, published on non-mainstream media websites. She also wrote articles that are published on RT’s website. And she is not alone—other journalists participate in similar ways but are not as prolific or visible as Beeley. Taken together, these information-sharing trajectories demonstrate how Russian state-sponsored media provide multi-dimensional support in the form of source content and amplification for influencers in the anti-White Helmets campaign.
Summary
Our research reveals the White Helmets discourse to have two distinct and oppositional sides. Though both sides shared video content, anti-White Helmets accounts did more “cross-posting” from YouTube to Twitter. This created a bridge between the two platforms, directing Twitter users to a large set of videos critiquing the White Helmets. Tracing information trajectories across platforms shows the anti-White Helmets cluster received multi-dimensional support from Russia’s state-sponsored media, including content production and amplification of friendly voices.
Limitations and future work
Our research has two significant limitations. Because we relied upon tweets as “seed” data, our findings provide a Twitter-centered view of the campaign targeting the White Helmets. From this perspective we can show that YouTube functions as a resource for Twitter users working to discredit the group, but we are unable to make strong claims about how YouTube fits into the broader White Helmets discourse. Additionally, our focus on English-language tweets means that we are unable to account for the Twitter conversation and YouTube links within, for example, the Arabic conversation.
Avenues for future research include understanding more about the financial and reputational incentives for participating in these campaigns and developing better methods to distinguish between sincere activists and paid agents. There is some evidence that several “activist” accounts in the anti-White Helmets cluster—and at least one influential activist in the pro-White Helmets cluster—are fake personas operated by political entities. We currently have a rough measure of bad behavior as measured by account suspensions (Appendix F) but would need more information from the platforms, e.g. about the nature of those suspensions, to fully understand the role of coordinated and/or inauthentic actors.
Methods
We adopt a mixed-methods approach, extending methodologies developed from the interdisciplinary field of crisis informatics (Palen et al., 2016) and prior studies of misinformation (Maddock et al., 2015). Our research is iterative and involves a combination of quantitative methods, for example to uncover the underlying structure of the online discourse and information flows (e.g. Figure 1), and qualitative methods to develop a deeper understanding of the content and accounts that are involved in seeding and propagating competing strategic narratives about the White Helmets.
Initial Twitter dataset
This research is centered around a Twitter dataset of English-language tweets referencing the “White Helmets,” collected using the Twitter streaming API between 27 May 2017 and 15 June 2018. We focused on English-language tweets to understand how these campaigns were framed for western audiences. The White Helmets Twitter dataset contains 913,028 tweets from 218,302 distinct Twitter accounts. More details about the collection and data are available in Appendix A.
Mapping the structure of the Twitter conversation
To understand the structure of the White Helmets discourse, we built a retweet network graph (Figure 1). In this graph, nodes represent accounts, and they are connected via edges (lines) when one account retweeted another. Nodes are sized relative to the number of retweets of that account, providing an indication of influential accounts within each cluster. We applied a community-detection algorithm (Blondel, 2008), revealing two main clusters (Red and Blue, Figure 1). These clusters include more than 75% of all accounts in our White Helmets tweets. Content analysis (Appendix D) revealed the Blue cluster to be consistently supportive of the White Helmets and the Red cluster to be consistently critical of the group. Appendix B contains more details of our network analysis.
Extending our Twitter data to include YouTube
We then used the YouTube API to scrape the video metadata of all distinct YouTube video IDs (unique identifiers for each YouTube video) referenced in the White Helmets Twitter data as of 22 November 2018. This was a total of 1747 distinct videos. At the time of the request, 260 videos were no longer available on YouTube due to copyright infringements, the removal of videos by their users, ephemeral content that no longer existed, and other unspecified reasons. We used the remaining 1487 videos to extend our view beyond Twitter to include YouTube data and to help us to understand the cross-platform nature of the White Helmets discourse.
Additional details of relevant analyses used in this research can be found in the Methodological Appendix.
Topics
Bibliography
Arif, A., Stewart, L.G., and Starbird, K., 2018. Acting the Part: Examining Information Operations within #BlackLivesMatter Discourse. PACMHCI, 2(CSCW). Article 20. https://doi.org/10.1145/3274289
Beeley, V., 2018. NATO-friendly media & organizations refuse to address White Helmets’ atrocities. (October 24, 2019). RT.com. Retrieved from: https://www.rt.com/op-ed/442168-white-helmets-syria-propaganda/
Bittman, L. 1985. The KGB and Soviet Disinformation: An Insider’s View. Washington: Pergamon-Brassey’s.
Brandt, J. & Hanlon, B. 2019. Online Information Operations Cross Platforms. Tech Companies’ Responses Should Too. The Lawfare Institute. Retrieved from: https://www.lawfareblog.com/online-information-operations-cross-platforms-tech-companies-responses-should-too
Blondel, V. D.; Guillaume, J.L.; Lambiotte, R.; and Lefebvre, E., 2008. Fast Unfolding of Communities in Large Networks. Journal of Statistical Mechanics: Theory and Experiment, 2008(10):P10008. 10.1088/1742-5468/2008/10/P10008
Carr, C. T., & Hayes, R. A., 2015. Social media: Defining, developing, and divining. Atlantic Journal of Communication, 23(1), 46-65. https://doi.org/10.1080/15456870.2015.972282.
di Giovanni, J., 2018. Why Assad and Russia Target the White Helmets. The New York Review of Books. Retrieved from: https://www.nybooks.com/daily/2018/10/16/why-assad-and-russia-target-the-white-helmets/
Diresta, R. & Grossman, S., 2019. Potemkin Pages & Personas: Assessing GRU Online Operation, 2014-2019. Stanford Internet Observatory, Cyber Policy Center. Retrieved from: https://fsi-live.s3.us-west-1.amazonaws.com/s3fs-public/potemkin-pages-personas-sio-wp.pdf
Jack, C., 2017. Lexicon of Lies: Terms for Problematic Information. Data & Society. Retrieved from: https://datasociety.net/pubs/oh/DataAndSociety_LexiconofLies.pdf
Levinger, M., 2018. Master narratives of disinformation campaigns. Journal of International Affairs, 71(1.5), pp.125-134. https://jia.sipa.columbia.edu/master-narratives-disinformation-campaigns
Lewis, R., 2018. Alternative influence: Broadcasting the reactionary right on YouTube. Data & Society Research Institute. Retrieved from: https://datasociety.net/wp-content/uploads/2018/09/DS_Alternative_Influence.pdf
Maddock, J., Starbird, K., Al-Hassani, H.J., Sandoval, D.E., Orand, M. and Mason, R.M., 2015. Characterizing online rumoring behavior using multi-dimensional signatures. In Proceedings of the 18th ACM conference on Computer Supported Cooperative Work & Social Computing (pp. 228-241). ACM. http://dx.doi.org/10.1145/2675133.2675280
Pomerantsev, P., and Weiss, M. 2014. The menace of unreality: How the Kremlin weaponizes information, culture and money. New York: Institute of Modern Russia. https://imrussia.org/media/pdf/Research/Michael_Weiss_and_Peter_Pomerantsev__The_Menace_of_Unreality.pdf
Solon, O. 2017. How Syria’s White Helmets became victims of an online propaganda machine. The Guardian (December 18, 2017). Retrieved from: https://www.theguardian.com/world/2017/dec/18/syria- white-helmets-conspiracy-theories
Starbird, K., Arif, A. and Wilson, T. 2019. Disinformation as collaborative work: Surfacing the participatory nature of strategic information operations. Proceedings of the ACM on Human-Computer Interaction, 3(CSCW), p.127. https://faculty.washington.edu/kstarbi/StarbirdArifWilson_DisinformationasCollaborativeWork-CameraReady-Preprint.pdf
Starbird, K.; Arif, A.; Wilson, T.; Van Koevering, K.; Yefimova, K.; and Scarnecchia, D. 2018. Ecosystem or echo- system? exploring content sharing across alternative media domains. In AAAI ICWSM.
Thorson, K., Driscoll, K., Ekdale, B., Edgerly, S., Thompson, L.G., Schrock, A., Swartz, L., Vraga, E.K. and Wells, C., 2013. YouTube, Twitter and the Occupy movement: Connecting content and circulation practices. Information, Communication & Society, 16(3), pp.421-451. https://doi.org/10.1080/1369118X.2012.756051
Palen, L. and Anderson, K.M., 2016. Crisis informatics—New data for extraordinary times. Science, 353(6296), pp.224-225. 10.1126/science.aag2579
Wanless, A. and Michael, B. 2017. Participatory propaganda: The engagement of audiences in the spread of persuasive communications. In Proceedings of the Social Media and Social Order, Culture Conflict 2.0 Conference, 2017.
Weedon, J., Nuland, W. and Stamos, A., 2017. Information operations and Facebook. Retrieved from: https://fbnewsroomus.files.wordpress.com/2017/04/facebook-and-information-operations-v1.pdf.
Wilson, T.; Zhou, K.; and Starbird, K. 2018. Assembling strategic narratives: Information operations as collaborative work within an online community. Proceedings of the ACM on Human-Computer Interaction, 2(CSCW): 183. https://doi.org/10.1145/3274452
Zakrzewski, C., 2018. The Technology 202: Social media companies under pressure to share disinformation data with each other. The Washington Post. December 19 2018. https://www.washingtonpost.com/news/powerpost/paloma/the-technology-202/2018/12/19/the-technology-202-social-media-companies-under-pressure-to-share-disinformation-data-with-each-other/5c1949241b326b2d6629d4e9/
Aknowledgments
We wish to thank the reviewers for their constructive feedback that helped refine this paper, and Ahmer Arif, Melinda McClure Haughey, and Daniel Scarnecchia for their contribution to this research project.
Funding
This research has been supported by the National Science Foundation (grants 1715078 and 1749815) and the Office of Naval Research (grants N000141712980 and N000141812012).
Competing Interests
None
Ethics
Our IRB classifies social media data as “not human subjects”. However, we apply ethical practices regarding how we collect, analyze and report on Twitter data. In particular, we attempt to obscure the identity of accounts of private individuals (unaffiliated with media or government). In this research, we have anonymized the names of most accounts in the data. However, we preserve the “real” account names for public individuals and organizations—including media outlets, journalists, non-profit organizations, government organizations and officials, as well as verified and highly visible accounts (with more than 100,000 followers).
Copyright
This is an open access article distributed under the terms of theCreative Commons Attribution License, which permits unrestricted use, distribution, and reproduction in any medium, provided that the original author and source are properly credited.
Data Availability
The data used for the analyses in this article are available within the Harvard Dataverse Network:
Wilson, Tom; Starbird, Kate, 2020, “White Helmets Twitter Dataset”, https://doi.org/10.7910/DVN/OHVXDD, Harvard Dataverse, V1
Wilson, Tom; Starbird, Kate, 2020, “White Helmets YouTube Dataset”, https://doi.org/10.7910/DVN/TJSBTS, Harvard Dataverse, V1
In particular, those data include the tweet-ids and limited metadata for all of the tweets in the White Helmets dataset, and limited metadata from YouTube for all the YouTube videos linked-to in those tweets (for videos that were available, i.e. not deleted or removed, on November 22, 2018).