Peer Reviewed
The algorithmic knowledge gap within and between countries: Implications for combatting misinformation
Article Metrics
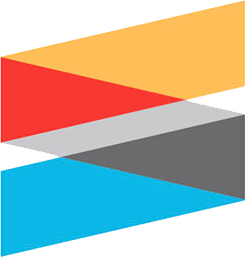
0
CrossRef Citations
Altmetric Score
PDF Downloads
Page Views
While understanding how social media algorithms operate is essential to protect oneself from misinformation, such understanding is often unevenly distributed. This study explores the algorithmic knowledge gap both within and between countries, using national surveys in the United States (N = 1,415), the United Kingdom (N = 1,435), South Korea (N = 1,798), and Mexico (N = 784). In all countries, algorithmic knowledge varied across different sociodemographic factors, even though in different ways. Also, different countries had different levels of algorithmic knowledge: The respondents in the United States reported the greatest algorithmic knowledge, followed by respondents in the United Kingdom, Mexico, and South Korea. Additionally, individuals with greater algorithmic knowledge were more inclined to take actions against misinformation.
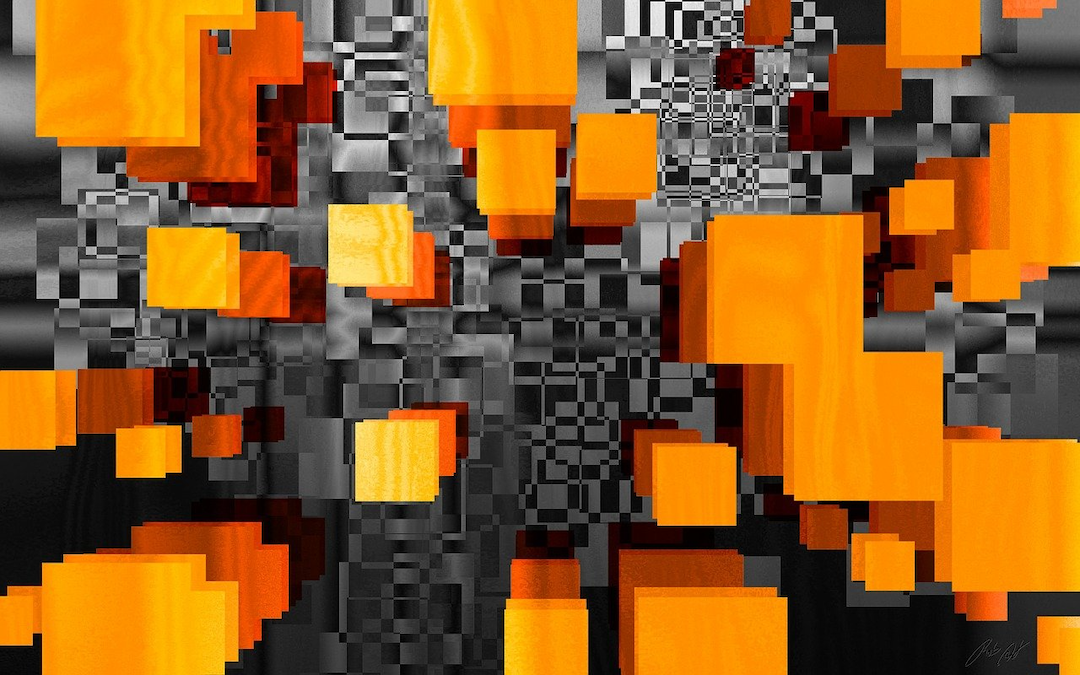
Research Questions
- How are sociodemographic factors (e.g., age, gender, education, income, political ideology, social media use, ethnicity) associated with algorithmic knowledge?
- How is algorithmic knowledge distributed between countries?
- How does algorithmic knowledge shape individuals’ reactions to misinformation on social media?
Essay Summary
- This study explored the unequal distribution of algorithmic knowledge both within and between countries, along with its real-world implications, drawing on national surveys in the United States (N = 1,415), the United Kingdom (N = 1,435), South Korea (N = 1,798), and Mexico (N = 784).
- In the four countries with diverse technological, political, and social landscapes, algorithmic knowledge varied across different sociodemographic factors, even though in different ways.
- Different countries had different levels of algorithmic knowledge: the United States respondents reported the greatest algorithmic knowledge, followed by the United Kingdom, Mexico, and South Korea. Notably, South Korean respondents exhibited the lowest levels of algorithmic knowledge, despite having highest rates of internet penetration and social media access among the four countries.
- Varying levels of algorithmic knowledge prompted individuals to respond to misinformation differently; those possessing higher algorithmic knowledge were more inclined to undertake actions to combat misinformation on social media than those lacking algorithmic knowledge.
- Our findings indicate that algorithmic knowledge can empower social media users to combat misinformation and address social inequalities. This study also highlights the necessity of tailoring algorithmic literacy initiatives to diverse populations across social and cultural backgrounds.
Implications
The digital divide, originally referring to the gap between people who have access to modern information and communications technology (ICT) and those who don’t (Ragnedda & Muschert, 2013), has been a concern since the advent of ICT and the internet. It has gained renewed attention due to the growing role of algorithms. In particular, algorithms increasingly control information flow on social media, determining what users see in their feeds. Social media algorithms are designed to filter and present online content in ways that optimize user engagement and retention (Golino, 2021), often resulting in the dissemination of misinformation that aligns with users’ biases and beliefs (Bakshy et al., 2015; Hussein et al., 2020; Pariser, 2011). A notable example is Facebook’s algorithm which supported a surge of hate-filled misinformation targeting the Rohingya people, contributing to their genocide by the Myanmar military in 2017 (Mozur, 2018).
Given these dangers, social media users’ understanding of algorithms has become crucial for users’ agency, public discourse, and democracy (Gran et al., 2021; Zarouali et al., 2021). However, algorithmic knowledge (i.e., understanding of how algorithms filter and present information) may not be equally distributed among people with different sociodemographic backgrounds (i.e., the second-level digital divide, Cotter & Reisdorf, 2020; Gran et al., 2021; Klawitter & Hargittai, 2018). In many respects, algorithmic knowledge may resemble civic, political, or economic knowledge in terms of its uneven distribution, but such a point has only recently begun to attract scholarly attention. With the uneven distribution of algorithmic knowledge, some may possess the ability to scrutinize and make informed judgments about the information presented by algorithms, while others may be more susceptible to the false or biased narratives embedded in algorithmic outputs without questioning them.
Some recent studies (e.g., Cotter & Reisdorf, 2020) have explored the development of the algorithmic knowledge gap across sociodemographic backgrounds and how these gaps intersect with our understanding of digital divides. However, prior studies have primarily focused on this algorithmic knowledge gap within individual countries, neglecting potential variations between countries. Hence, it remains unclear whether the algorithmic knowledge gap found in one country is observable in different national contexts (Oeldorf-Hirsch & Neubaum, 2023). Given that algorithms operate within intricate blends of political, technological, cultural, and social dynamics (Roberge & Melançon, 2017), it is possible that certain factors hold greater influence over individuals’ algorithmic knowledge in one country, while exerting less power in other countries. Furthermore, the level of algorithmic knowledge might vary among countries, even if they share similar levels of access to the internet and social media. Lastly, while there is increasing awareness that differences in skills or knowledge of digital resources have inherent consequences in the real world (i.e., the third-level digital divide, Fuchs, 2009; Selwyn, 2004 ), the specific outcomes of the algorithmic knowledge gap have rarely been empirically examined (Scheerder et al., 2017).
Against this backdrop, this study advances research on the algorithmic knowledge gap across multiple fronts. First, by exploring the association between various sociodemographic factors and algorithmic knowledge, this study extends prior research on the second-level digital divide. Across four countries with diverse technological, political, and social landscapes, we found a degree of cross-cultural validity indicating that younger individuals and frequent social media users have greater algorithmic knowledge. Additionally, while the specific predictors varied across countries, algorithmic knowledge was unevenly distributed across education level, ethnicity, gender, and political ideology. Of note, over 90% of respondents in all four countries had access to social media (see Table 3 in Appendix B), suggesting that discussion surrounding today’s digital divide indeed needs to encompass more than just the binary distinction between individuals with access to the internet or social media and those without (i.e., the first-level digital divide).
This study also expands the existing research on the algorithmic knowledge gap which has predominantly focused on single national populations. Our findings indicate that the predictive power of specific sociodemographic factors may differ between countries. In the United States, political ideology and ethnicity were the primary predictors of algorithmic knowledge. In the United Kingdom, age, political ideology, and social media use were the significant factors. In South Korea, the main predictors included age, gender, education, and social media use, whereas in Mexico, only education and social media use were associated with algorithmic knowledge. Certain country-specific determinants warrant further attention. In economically advanced Western democracies, such as the United States and the United Kingdom, where political polarization has reached high levels in recent years (Dimock & Wike, 2020; Grechyna, 2023), political ideology emerged as the most significant factor in explaining differences in algorithmic knowledge; individuals with more liberal views were more likely to understand how social media algorithms function compared to their conservative counterparts. In contrast, in South Korea where women encounter ongoing disparities and obstacles across various societal domains (Lee, 2024), gender emerged as the most powerful predictor of algorithmic knowledge, with male respondents demonstrating a deeper understanding of algorithmic mechanisms than female respondents. These findings suggest that the algorithmic knowledge gap can manifest across various dimensions beyond traditional socioeconomic factors, such as income and education. Future research exploring additional social or cultural determinants may offer a more comprehensive understanding of the algorithmic knowledge gap, particularly in cross-country explorations.
Moreover, our findings elucidated disparities in algorithmic knowledge among countries. We found significant variations in knowledge levels across the four countries. The United States exhibited the highest overall algorithmic knowledge, followed by the United Kingdom, Mexico, and South Korea. One insight of note is that South Korea boasted the highest rates of internet penetration (98%) among the four countries examined (DataReportal, 2022). This finding once again underscores the notion that the issue of access to digital resources alone cannot offer a comprehensive understanding of the digital divide today. It also highlights the importance of considering national and cultural contexts in future research and potential interventions to enhance algorithmic knowledge.
Another important contribution of this study pertains to the outcomes of the algorithmic knowledge gap. While numerous scholars have proposed that the digital divide contributes to the perpetuation of existing social inequalities (e.g., Helsper, 2010; Van Dijk, 2006), empirical investigations into the real-world outcomes of the algorithmic knowledge gap have been limited. Bridging this gap, our study furnishes empirical evidence indicating that individuals with greater algorithmic knowledge are more inclined to take actions against misinformation. The rationale behind this link is that when people understand that social media algorithms provide information based on their previous interactions and those of similar users, they may be more aware of the risks of being trapped in filter bubbles (Pariser, 2011) that limit exposure to diverse viewpoints and make them more vulnerable to misinformation (Ciampaglia et al., 2018). Recognizing these risks might prompt people to take steps to avoid or mitigate them. Previous research supports this reasoning, indicating that individuals unaware of how algorithms tailor information to match their existing beliefs may mistakenly believe that all content they encounter on social media is unbiased and accurate (Effron & Raj, 2020), leading them to endorse or share such content without reservations (Shin & Valente, 2020). In particular, those traditionally lagging behind in terms of algorithmic knowledge—such as elderly populations or less educated—were found to be more likely to spread misinformation (Gottfried & Grieco, 2018) and more vulnerable to associated harms (Seo et al., 2021). In this light, the current study suggests the potential role of algorithmic knowledge in empowering those populations to fight misinformation and mitigate the prevailing social inequalities.
Our findings contain significant implications for social media platforms, government, and civil society, as various stakeholders attempt to curb the spread of misinformation. Much of the work to reduce the spread of misinformation has revolved around approaches such as better platform moderation (Chung & Wihbey, 2024), stronger informational labels and cues relating to quality (Chung & Wihbey, 2024), and prebunking or fact-checking (Chung & Kim, 2021; Roozenbeek et al., 2020). Furthermore, civil society and government actors often focus on debunking domain-specific misinformation in spheres such as elections and public health, with varying effectiveness (for a meta-analysis see Walter et al., 2020). However, the findings presented here indicate that developing literacy programs to improve general knowledge around algorithmic mechanisms and flow of information could be a promising alternative.
When designing algorithmic literacy programs, special attention must be given to reaching vulnerable groups identified in this study, such as elderly or less educated populations, who may be more difficult to engage through conventional educational systems (Seo et al., 2021). It is also important to pay careful attention to the varying predictors of the algorithmic knowledge gap across countries, which we have demonstrated in this study, to optimize the effectiveness of algorithmic literacy programs in each country.
Some limitations of this study are worth noting. First, our measurement of algorithmic knowledge focused on knowledge about specific applications (Facebook and X/Twitter) and considered only one type of algorithmic operation (content recommendation for personal feeds). Given that respondents may have different levels of familiarity and experience with other social media platforms and types of algorithms, our findings may not fully represent the breadth and depth of users’ algorithmic knowledge. Therefore, we should not assume that algorithmic knowledge is inherently linked to certain sociodemographic features or nationalities discussed in this study, as these populations’ understanding of other aspects of social media algorithms may present different pictures. Future research employing a more comprehensive measurement of algorithmic knowledge (e.g., Dogruel et al., 2022) would provide us with a deeper understanding of how various sociodemographic factors and nationalities influence perceptions and understanding of social media algorithms. Second, while the anchor population statistics for each country (see Table 1 in Appendix B) were the latest available at the time of data collection, more up-to-date population statistics would offer a more accurate assessment of the representativeness of the samples.
Overall, this study adds significant nuance to the debate about how to strengthen global citizens’ ability to navigate the misinformation-prevalent social media environment. The new frontier in research relating to the algorithmic knowledge gap may in large part relate to some of the factors revealed in this study, such as the apparent role of sociodemographic factors as well as global-cultural embedding. Further research should pursue all these areas, deepening our sense of where new digital divides are emerging, why they might exist, and how interventions might help to close such gaps.
Findings
Finding 1: Algorithmic knowledge is not equally distributed across individuals with different socio-demographic backgrounds.
Consistent with the extant literature on the second-level digital divide in algorithmic knowledge (Cotter & Reisdorf, 2020; Gran et al., 2021; Klawitter & Hargittai, 2018), we found that algorithmic knowledge is not equally distributed across individuals with different sociodemographic backgrounds. As illustrated in Table 1, age has a statistically significant but modest effect on algorithmic knowledge, with younger individuals exhibiting a better understanding of algorithmic mechanisms compared to older individuals.1Further analyses of the data revealed that individuals between 18–24 years (the United States and the United Kingdom) and 25-34 years (South Korea) demonstrated the highest levels of algorithmic knowledge (see Table 2 in Appendix A). We also found that social media use has a modest predictive power for algorithmic knowledge, with frequent social media users having a better understanding of algorithmic operations than less active users. Additionally, individuals with higher levels of education exhibit a greater understanding of how social media algorithms curate and present content compared to less educated people in two countries. Political ideology, gender, and ethnicity were also identified as predictors of algorithmic knowledge in certain countries.
It is important to note that the specific pattern of the algorithmic knowledge gap varied among countries. For instance, while age negatively predicted algorithmic knowledge in the United States, the United Kingdom, and South Korea, it did not have any predictive power in Mexico. Conversely, social media use was positively correlated with algorithmic knowledge in the United Kingdom, South Korea, and Mexico, but not in the United States. Education positively predicted algorithmic knowledge in South Korea and Mexico, but did not have any significant influence in the United States and the United Kingdom. Furthermore, in the United States and the United Kingdom, political ideology emerged as the primary predictor of the algorithmic knowledge gap, with individuals leaning more liberal demonstrating a greater understanding of social media algorithms compared to their conservative counterparts. In South Korea, gender was a notable predictor of the algorithmic knowledge gap, with male respondents demonstrating a significantly higher understanding of how social media algorithms operate compared to female respondents.
Variable | United States | United Kingdom | South Korea | Mexico |
β | β | β | β | |
Antecedents of Algorithmic Knowledge | ||||
Age | -.01* | -.01** | -.02*** | -.00 |
Gender | -.00 | .03 | .23** | -.05 |
Income | .00 | -.02 | .02 | .02 |
Education | .02 | -.00 | .17*** | .05* |
Political ideology | .15*** | .11*** | .01 | .01 |
Social media use | .04 | .08* | .09* | .11* |
Ethnicity | -.04* | -.02 | N/A | N/A |
Outcome of Algorithmic Knowledge | ||||
Corrective actions | 2.93** | 1.43*** | .07*** | 1.11* |
Finding 2: Algorithmic knowledge predicts actions to counter misinformation.
Varying levels of algorithmic knowledge prompt individuals to respond to misinformation differently, elucidating the real-world outcomes of the algorithmic knowledge gap (i.e., the third-level digital divide, Fuchs, 2009; Scheerder et al., 2017). Specifically, algorithmic knowledge was positively associated with intentions to take corrective actions in all four countries (see Table 1). Those possessing higher algorithmic knowledge were more inclined to undertake actions to combat misinformation, such as commenting to caution against potential biases or risks in media messages, sharing counter-information or opinions, disseminating information exposing the flaws in the provided media content, and reporting a misinformation post to the social media platform, as compared to those lacking algorithmic knowledge.
Finding 3: There are cross-country disparities in algorithmic knowledge.
There was unequal distribution of algorithmic knowledge across countries. Respondents from the four countries exhibited significantly different levels of understanding about how social media algorithms work, F(3, 5428) = 107.28, p < .001. Notably, the U.S. respondents reported the greatest algorithmic knowledge (M = 6.12, SD = 1.73), followed by the United Kingdom (M = 6.05, SD = 1.74), Mexico (M = 5.70, SD = 1.49), and South Korea (M = 5.16, SD = 1.76). A Bonferroni post-hoc test indicated that there were no significant differences in the algorithmic knowledge level between respondents from the United States and the United Kingdom, whereas both groups exhibited significantly distinct levels of algorithmic knowledge compared to respondents from South Korea and Mexico, all ps < .001. Furthermore, respondents from South Korea exhibited notably lower levels of algorithmic knowledge compared to those from all other countries, all ps < .001.
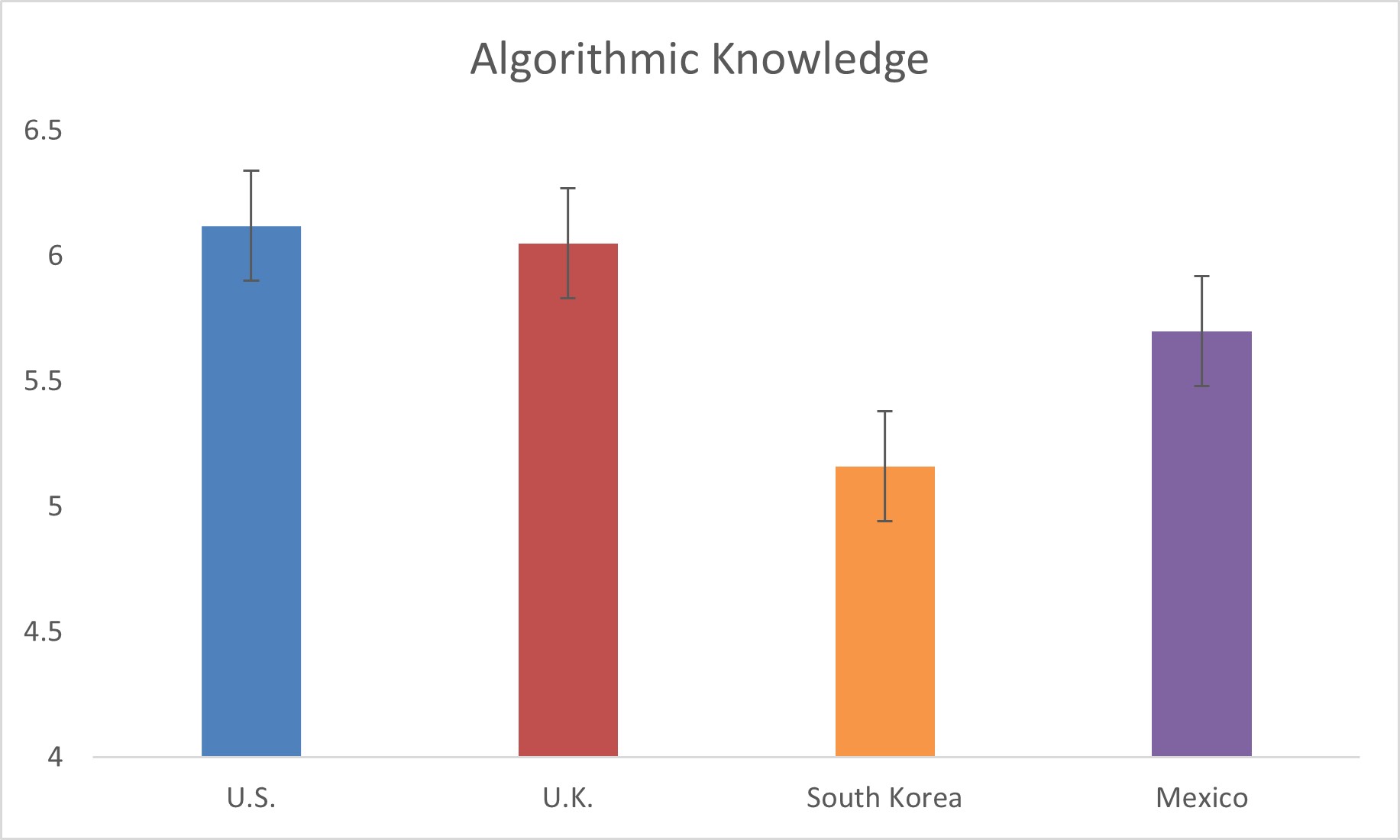
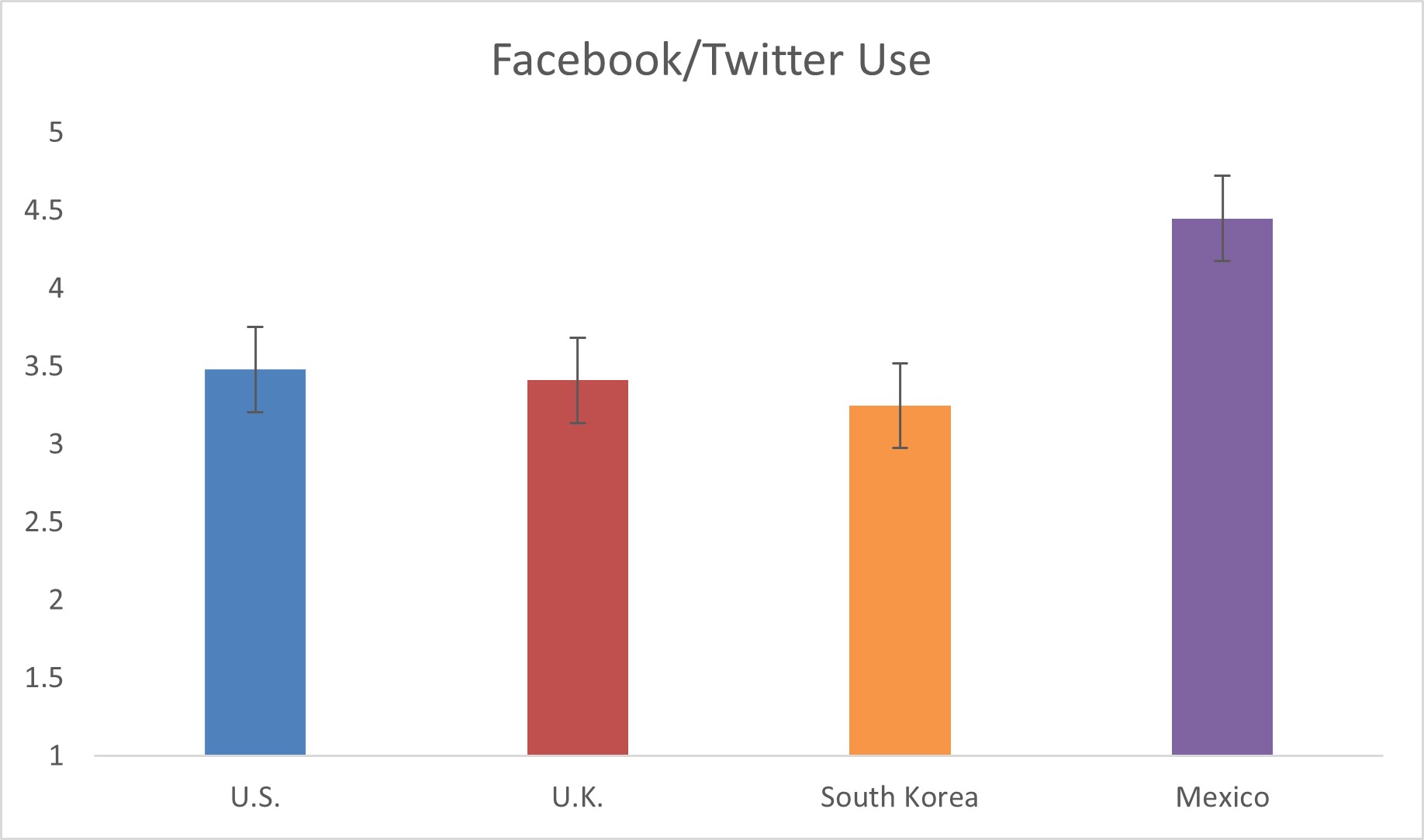
Relatedly, the levels of Facebook and X/Twitter use also differed significantly across countries, F(3, 5431) = 216.12, p < .001. A Bonferroni post-hoc test revealed significant differences in Facebook and X/Twitter use among respondents from the United States, Korea, and Mexico, all ps < .001. Notably, respondents from South Korea exhibited significantly lower levels of Facebook and X/Twitter use compared to those from all other countries, all ps < .001. Respondents from the United States and the United Kingdom did not show distinct levels of Facebook and X/Twitter use from each other. Although the varying levels of Facebook and X/Twitter use (see Figure 2) did not perfectly align with the pattern of the algorithmic knowledge gap between countries (see Figure 1), it is reasonable to suggest that social media use levels contributed to the between-country algorithmic knowledge gap to some extent. Nevertheless, considering that South Korea had higher overall social media access (83%) than the other three countries (see Table 3 in Appendix B), this issue may be related to the limitations of our algorithmic knowledge measurement discussed above.
Methods
We conducted national surveys in the United States (N = 1,415), the United Kingdom (N = 1,435), South Korea (N = 1,718), and Mexico (N = 784) between April and September 2021. We used a non-probability-based quota sample to resemble the demographics of each country (see Table 1 in Appendix B). The four countries were chosen to reflect geographic diversity, a range of Internet experiences, and divergent approaches to digital literacy education (see Table 3 in Appendix B).
Respondents in the four countries were presented with the same questionnaire. We conducted a pre-test of the survey in English with students and faculty members (N = 29) from the researchers’ university network, approximately one-third of whom were from international/non-U.S. populations. Feedback from respondents regarding question wording and order was integrated into the final survey questionnaire.2Pre-test feedback primarily concerned confusing or unclear language in the survey. For instance, some pre-test respondents noted that “Report the post to the social media platform” was not specific enough to measure a corrective action. Therefore, we revised the item to “Report the post as misinformation to the social media platform.” The survey questionnaire was administered in English in the United States and the United Kingdom Respondents in South Korea received a Korean version, while respondents in Mexico received a Spanish version of the survey questionnaire. The English version was translated into Korean by a native Korean speaker and into Spanish by a native Spanish speaker with advanced English skills. We then performed back-translations to ensure accuracy.
Algorithmic knowledge was measured with nine questions with yes/no options that gauged participants’ understanding of social media algorithms (modified from Brodsky et al., 2020; Powers, 2017; see Table 1 in Appendix C for question items). The number of correct answers constituted the algorithmic knowledge index (possible range of 0 to 9, M = 6.12, SD = 1.73 in the United States; M = 6.05, SD = 1.74 in the United Kingdom; M = 5.16, SD = 1.76 in South Korea; M = 5.70, SD = 1.49 in Mexico).
Intention to take actions to counter misinformation was measured by asking respondents how likely they are to take the following actions when they encounter misinformation on social media (modified from Barnidge & Rojas, 2014; Lim, 2017, 1 = extremely unlikely, 5 = extremely likely, M = 2.82, SD = 1.13, α = .84 in the United States; M = 2.58, SD = 1.07, α = .83 in the United Kingdom; M = 2.19, SD = .93, α = .85 in South Korea; M = 3.58, SD = .93, α = .77 in Mexico).
Sociodemographic variables including age, gender, education (1 = less than high school, 9 = doctoral degree), ethnicity (in the United States and the United Kingdom only, see Table 1 in Appendix B), monthly household income (the range varied across countries), and political views (1 = very conservative, 7 = very liberal) were measured.
Topics
Bibliography
Barnidge, M., & Rojas, H. (2014). Hostile media perceptions, presumed media influence, and political talk: Expanding the corrective action hypothesis. International Journal of Public Opinion Research, 26(2), 135–156. https://doi.org/10.1093/ijpor/edt032.
Bakshy, E., Messing, M., & Adamic, L. A. (2015). Exposure to ideologically diverse news and opinion on Facebook. Science, 348(6239), 1130–1132. https://doi.org/10.1126/science.aaa1160.
Brodsky, J. E., Zomberg, D., Powers, K. L., & Brooks, P. J. (2020). Assessing and fostering college students’ algorithm awareness across online contexts. Journal of Media Literacy Education, 12(3), 43–57. https://doi.org/10.23860/JMLE-2020-12-3-5.
Chung, M., & Kim, N. (2021). When I learn the news is false: How fact-checking information stems the spread of fake news via third-person perception. Human Communication Research, 47(1), 1–24. https://doi.org/10.1093/hcr/hqaa010
Chung, M., & Wihbey, J. (2024). Social media regulation, third-person effect, and public views: A comparative study of the United States, the United Kingdom, South Korea, and Mexico. New Media & Society, 26(8), 4534–4553. https://doi.org/10.1177/14614448221122996
Ciampaglia, G. L., Nematzadeh, A., Menczer, F., & Flammini, A. (2018). How algorithmic popularity bias hinders or promotes quality. Scientific Reports, 8(1), 15951. https://doi.org/10.1038/s41598-018-34203-2
Cotter, K., & Reisdorf, B. C. (2020). Algorithmic knowledge gaps: A new dimension of (digital) inequality. International Journal of Communication, 14, 745–765. https://ijoc.org/index.php/ijoc/article/view/12450
DataReportal. (2022). Digital 2022 global digital overview. https://datareportal.com/reports/digital-2022-global-overview-report.
Dimock, M., & Wike, R. (2020). America is exceptional in the nature of its political divide. Pew Research Center. https://www.pewresearch.org/short-reads/2020/11/13/america-is-exceptional-in-the-nature-of-its-political-divide/.
Dogruel, L., Masur, P., & Joeckel, S. (2022). Development and validation of an algorithm literacy scale for internet users. Communication Methods and Measures, 16(2), 115–133. https://doi.org/10.1080/19312458.2021.1968361
Effron, D. A., & Raj, M. (2020). Misinformation and morality: Encountering fake-news headlines makes them seem less unethical to publish and share. Psychological Science, 31(1), 75–87. https://doi.org/10.1177/0956797619887896
Fuchs, C. (2009). The role of income inequality in a multivariate cross-national analysis of the digital divide. Social Science Computer Review, 27(1), 41–58. https://doi.org/10.1177/0894439308321628
Gran, A. B., Booth, P., & Bucher, T. (2021). To be or not to be algorithm aware: a question of a new digital divide? Information, Communication & Society, 24(12), 1779–1796. https://doi.org/10.1080/1369118X.2020.1736124
Grechyna, D. (2023). Political polarization in the UK: measures and socioeconomic correlates. Constitutional Political Economy, 34(2), 210–225. https://doi.org/10.1007/s10602-022-09368-8
Golino, M. A. (2021). Algorithms in social media platforms. Institute for Internet and the Just Society. https://www.internetjustsociety.org/algorithms-in-social-media-platforms.
Gottfried, J., & Grieco, E. (2018, October 23). Younger Americans are better than older Americans at telling factual news statements from opinions. Pew Research Center. https://www.pewresearch.org/short-reads/2018/10/23/younger-americans-are-better-than-older-americans-at-telling-factual-news-statements-from-opinions/.
Heikkilä, M. (2022, October 4). The White House just unveiled a new AI Bill of Rights. MIT Technology Review. https://www.technologyreview.com/2022/10/04/1060600/white-house-ai-bill-of-rights/
Helsper, E. J. (2010). Gendered Internet use across generations and life stages. Communication Research, 37(3), 352–374. https://doi.org/10.1177/0093650209356439
Hussein, E., Juneja, P., & Mitra, T. (2020). Measuring misinformation in video search platforms: An audit study on YouTube. Proceedings of the ACM on Human-Computer Interaction, 4(CSCW1), 1–27. https://doi.org/10.1145/3392854
Klawitter, E., & Hargittai, E. (2018). “It’s like learning a whole other language”: The role of algorithmic skills in the curation of creative goods. International Journal of Communication, 12, 3490–3510. https://ijoc.org/index.php/ijoc/article/view/7864
Lee, M. Y. H. (2024, February 21). South Korea, a nation of rigid gender norms, meets its changemakers. The Washington Post. https://www.washingtonpost.com/world/2024/02/21/south-korea-women-gender-equality-gap/.
Lim, J. S. (2017). The third-person effect of online advertising of cosmetic surgery: A path model for predicting restrictive versus corrective actions. Journalism & Mass Communication Quarterly, 94(4), 972–993. https://doi.org/10.1177/1077699016687722
Mozur, P. (2018, October 15). A genocide incited on Facebook, with posts from Myanmar’s military. The New York Times. https://www.nytimes.com/2018/10/15/technology/myanmar-facebook-genocide.html
OECD. (2021). 21st-century readers: Developing literacy skills in a digital world. https://www.oecd.org/publications/21st-century-readers-a83d84cb-en.htm.
Oeldorf-Hirsch, A., & Neubaum, G. (2023). What do we know about algorithmic literacy? The status quo and a research agenda for a growing field. New Media & Society, 14614448231182662. https://doi.org/10.1177/14614448231182662
Pariser, E. (2011). The filter bubble: How the new personalized web is changing what we read and how we think. Penguin Books.
Polizzi, G. (2020). Digital literacy and the national curriculum for England: Learning from how the experts engage with and evaluate online content. Computers and Education, 152, 103859. https://doi.org/10.1016/j.compedu.2020.103859
Powers, E. (2017). My news feed is filtered? Awareness of news personalization amon– college students. Digital Journalism, 5(10), 1315–1335. https://doi.org/10.1080/21670811.2017.1286943
Ragnedda, M., & Muschert, G. W. (2013). The digital divide. Routledge.
Roberge, J., & Melançon, L. (2017). Being the King Kong of algorithmic culture is a tough job after all: Google’s regimes of justification and the meanings of Glass. Convergence, 23(3), 306–324. https://doi.org/10.1177/1354856515592506
Roozenbeek, J., Van Der Linden, S., & Nygren, T. (2020). Prebunking interventions based on “inoculation” theory can reduce susceptibility to misinformation across cultures. Harvard Kennedy School (HKS) Misinformation Review, 1(2). https://doi.org/10.17863/CAM.48846
Scheerder, A., Van Deursen, A., & Van Dijk, J. (2017). Determinants of Internet skills, uses and outcomes. A systematic review of the second-and third-level digital divide. Telematics and Informatics, 34(8), 1607–1624. https://doi.org/10.1016/j.tele.2017.07.007
Seo, H., Blomberg, M., Altschwager, D., & Vu, H. T. (2021). Vulnerable populations and misinformation: A mixed-methods approach to underserved older adults’ online information assessment. New Media & Society, 23(7), 2012–2033. https://doi.org/10.1177/1461444820925041
Selwyn, N. (2004). Reconsidering political and popular understandings of the digital divide. New Media & Society, 6(3), 341–362. https://doi.org/10.1177/1461444804042519
Shin, J., & Valente, T. (2020). Algorithms and health misinformation: A case study of vaccine books on Amazon. Journal of Health Communication, 25(5), 394–401. https://doi.org/10.1080/10810730.2020.1776423
Statistica. (n.d.). Internet demographics & use. https://www.statista.com/markets/424/topic/537/demographics-use/#overview
UNESCO. (2024). Network for media and information literacy in Mexico. https://www.unesco.org/en/articles/network-media-and-information-literacy-mexico
University of Oxford. (2023). Digital literacy: Being a digitally literate student. https://www.ox.ac.uk/event/digital-literacy-being-digitally-literate-student
Van Dijk, J. A. (2006). Digital divide research, achievements and shortcomings. Poetics, 34(4–5), 221–235. https://doi.org/10.1016/j.poetic.2006.05.004
Walter, N., Cohen, J., Holbert, R. L., & Morag, Y. (2020). Fact-checking: A meta-analysis of what works and for whom. Political Communication, 37(3), 350–375. https://doi.org/10.1080/10584609.2019.1668894
Zarouali, B., Helberger, N., & De Vreese, C. H. (2021). Investigating algorithmic misconceptions in a media context: Source of a new digital divide? Media and Communication, 9(4), 134–144. https://doi.org/10.17645/mac.v9i4.4090
Funding
This study was supported by the Tier 1 grant (grant number: 351179) from Northeastern University.
Competing Interests
The authors declare no competing interests.
Ethics
The research protocol employed was approved by an institutional review board (IRB#: 21-03-22) at Northeastern University. All survey participants were provided informed consent. Ethnicity/gender categories used in this study were based on the American Community Survey and the U.K. Census survey.
Copyright
This is an open access article distributed under the terms of the Creative Commons Attribution License, which permits unrestricted use, distribution, and reproduction in any medium, provided that the original author and source are properly credited.
Data Availability
All materials needed to replicate this study are available via the Harvard Dataverse: https://doi.org/10.7910/DVN/7EHLMI