Peer Reviewed
How do social media users and journalists express concerns about social media misinformation? A computational analysis
Article Metrics
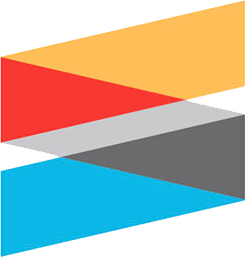
0
CrossRef Citations
Altmetric Score
PDF Downloads
Page Views
This article describes partisan-based, accuracy-based, and action-based discussions through which U.S. social media users and journalists express concerns about social media misinformation. While platform policy stands out as the most highly discussed topic by both social media users and journalists, much of it is cast through a party politics lens. The findings call for shifting the news frame around misinformation for collective problem-solving. At the same time, discussions about user agency are more prevalent on social media than in news, offering hope for platforms and educators to empower social media users to engage in discussions and actions about addressing misinformation.

Research Questions
- How frequently do social media users and journalists express concerns about social media misinformation through partisan-based, accuracy-based, or action-based discussions?
- How do partisan-based, accuracy-based, and action-based discussions co-occur with each other (i.e., in what ways are these topics being brought up simultaneously)?
Essay Summary
- Supervised machine learning is used to classify texts from U.S. mainstream broadcast news, Twitter (now X), and Facebook posts that discuss the topic of social media misinformation.
- Platform policy and party politics are the dominant types of discussions about social media misinformation among both journalists and social media users.
- Discussions about platform policy and party politics are the most likely to co-occur together among all discussions, suggesting that the very idea of platform intervention on misinformation is largely politicized.
- Discussions about user agency are more prevalent on Twitter and Facebook than in the news, frequently co-occurring with discussions about direct misinformation correction.
- Findings 1) call for journalists to shift away from politicized frames around platform policies aimed at mitigating misinformation, focusing on accuracy instead and 2) provide hope for platforms and educators to empower social media users to express and enact agency in addressing misinformation.
Implications
While social media misinformation constitutes a small percentage of an average American’s information diet in volume (Allen et al., 2020; González-Bailón et al., 2023; Guess et al., 2020), concerns and discussions about social media platforms’ power in fueling the spread of misinformation have continued to grow. Social media users frequently express concerns about misinformation spreading on platforms, often through identity-based languages (Li & Su, 2020). On the other hand, misinformation has become a prevalent theme in mainstream news coverage. Mainstream media not only are the gatekeeper and corrector of misinformation (Lwin et al., 2023), but also have routinized the coverage about misinformation, with many creating a “misinformation beat” (McClure Haughey et al., 2020).
This article describes five types of discussions through which U.S. social media users and journalists engage with the topic of social media misinformation: 1) party politics, 2) quality of knowledge and decisions, 3) direct corrections, 4) user agency, and 5) platform policy. These types of discussions are identified both deductively, by explicating the two dimensions of the theory of motivated reasoning in the context of discussions about social media misinformation, and inductively, with researchers and coders iteratively working with the sample data, engaging with existing research, and updating the coding scheme. They are identified based on their different foci, claims, and explanations about the importance of social media misinformation. In other words, we examine what the driving force is behind people’s expressed concern about social media misinformation.
We use the theory of motivated reasoning as a tool to map different lenses people adopt to express concerns about misinformation. While the theory of motivated reasoning is often applied to study how people process a specific piece of information, we argue that it is also useful for studying how people diagnose problems they see in an information environment overall. The theory of motivated reasoning outlines two basic types of motivations underlying all human reasoning (Taber & Lodge, 2006). The first is partisan motivation—the desire to defend preexisting partisan stance. In the context of talking about social media misinformation, being driven by partisan motivation means that people are concerned about social media misinformation because it benefits or harms a certain political party. We call this the party politics discussion, which is used to express concerns over social media misinformation through a lens of party conflict and competition. In this type of discussion, misinformation is seen as an arena where political strategies play out and a place that reflects political (dis)advantages.
In contrast, the second type of motivation outlined by the theory of motivated reasoning is the accuracy motivation—the desire to make accurate, optimal judgments. In the context of discussing the problem of social media misinformation, being motivated by accuracy means that people are concerned about misinformation primarily because it negatively affects the ability to hold accurate beliefs and make best decisions, for example, leading people to form inaccurate views about public affairs or make health decisions that result in illness for themselves or others. We call this the quality of knowledge and decisions discussion. The central concern in this discussion is not political gains or losses, but the verifiable truth itself, and the negative consequences that might flow from not believing the verifiable truth. Besides the quality of knowledge and decisions discussion that focuses on the negative impacts on accuracy, the direct corrections discussion shows accuracy on the spot. In the direct corrections discussion, accuracy is demonstrated through directly debunking misinformation, offering explanations on why a piece of misinformation is false, and transparently providing relevant facts.
While partisan- and accuracy-based discussions are direct applications of motivated reasoning, we also observed frequent discussions on solutions to misinformation from our data and from existing research on misinformation intervention (for a review, see Aghajari et al., 2023) that inherently cut across the binary of accuracy- or partisan-motivated reasoning. These solutions most frequently include calls for action from individual social media users and from social media platforms (Barthel et al., 2016; de Cock Buning, 2018). As such, we describe the user agency discussion that focuses on actions by ordinary social media users to address misinformation. The user agency discussion can express both accuracy and partisan concerns about misinformation. While the call for user agency can advocate for accuracy goals by offering tips on discerning fabricated headlines or encouraging people to read fact checks (Vraga et al., 2020), it can be also articulated to justify selectively seeking favorable information and turning away from uncongenial sources (Nelson & Lewis, 2023).
Finally, we describe the platform policy discussion that focuses on actions to counter misinformation by social media platforms. Platform policies such as exposing people to fact checks (Bode & Vraga, 2015) and banning elite accounts that repeatedly spread misinformation (Dwoskin & Timberg, 2021) may improve belief accuracy, but support for such policies is divided along partisan lines (Saltz et al., 2021). Platform actions aiming at reducing the harm of misinformation quickly become a fertile ground for debates over political bias and censorship. Taken together, action-based discussions, including user agency and platform policy, can intersect with and be fueled by both accuracy- and partisan-based discussions.
A worrying finding from our study is that platform policy and party politics are not only the most frequent types of discussions about misinformation but also most likely to co-occur together among all discussions about misinformation by both journalists and social media users. Tromble and McGregor (2019) argue that scholars should both weigh in on practical solutions for platforms to curb misinformation and provide a critical view on the very nature of platform policies and answer normative questions on social good and harm. This article offers a case for their argument. While empirical evidence shows the effectiveness of specific solutions that can be implemented on platforms, the very idea of platform intervention has been largely politicized, even in mainstream news sources widely thought as following norms of objectivity and nonpartisan reporting. This is consequential: News coverage on platform policy that centers partisan conflict and claims of bias can feed into the strategic frame, turning truth and falsehood into a matter of winning and losing. Such strategic frame in news coverage can decrease knowledge, increase cynicism, and demobilize the public (Zoizner, 2021). When party politics becomes the primary lens to talk about platform policy, it is more likely that political groups will talk past each other in debating partisan bias as compared to engaging in meaningful collective problem-solving, allowing technology companies to continue the current business model and keep the power in deciding how misinformation gets addressed. Moreover, party politics-style coverage can obscure attention to what is verifiably true and what is not as it tends to index competing partisan claims about issues (Bennett, 1990), failing to adjudicate between claims that are verifiably true and those that are verifiably false.
On the other hand, we also find that although less frequent than platform policy and party politics, accuracy-based discussions that highlight quality of knowledge and decisions or offer direct misinformation corrections still occupy a visible part of how both journalists and social media users talk about misinformation. Moreover, discussion about user agency is more frequent on social media than in news, frequently co-occurring with direct misinformation corrections. Contributing to the emerging research that demonstrates the potential for accuracy motivations to help combat misinformation (Pennycook et al., 2021), this finding offers hope that accuracy motivations—a normative ideal for an informed citizen—exist in real-world discussions about misinformation and that social media can be a productive space to foster accuracy-based discussions.
Practically, what can journalists, social media platforms, and ordinary social media users do in light of our findings? For journalists, changing news frames on misinformation is necessary to move past a contentious understanding of truth and falsehood. A first step is to de-emphasize party conflicts in coverage of misinformation policy and instead foster accuracy-based discussions by foregrounding how misinformation can impact the quality of people’s knowledge and decisions in multilayered ways above and beyond partisan differences. Journalists can also foster accuracy-based discussions by explicitly offering factual corrections when sources in the story make false statements. It is fruitful for future research to examine journalistic norms about covering misinformation to understand the reasons behind the dominance of certain types of coverage.
For social media platforms, our research points to the potential for platforms to implement interventions that highlight accuracy to motivate social media users to productively engage in discussions, corrections, and actions addressing misinformation. In line with this argument, a growing number of experiments has suggested that simple accuracy nudges that ask people to think about how accurate a headline is can be an effective strategy to curb misinformation across national contexts such as the United States (Pennycook et al., 2021), Australia (Butler et al., 2023), Korea (Shin et al., 2023), and China (Xiang et al., 2023).
For everyday social media users, it is important to be self-reflective about the underlying reasons for why one is skeptical towards misinformation before enacting agency. Emerging evidence suggests that skepticism rooted in accuracy can help combat misinformation while skepticism rooted in partisan identity cannot (Li, 2023). Complementing these experimental studies on accuracy nudges and accuracy-motivated skepticism, the current article looks at large-scale “real talk” by social media users and provides hopeful evidence that a substantial proportion of social media users do use an accuracy lens when expressing concerns about misinformation, signaling the realistic promise of fostering accuracy-based discussions among users. The descriptive findings in this study also provide a useful map of the variety of competing considerations that social media users have about misinformation, pointing to the need to ask why people are concerned about misinformation as compared to how much they are concerned.
Findings
Finding 1: Platform policy and party politics are the dominant lens through which misinformation is covered on mainstream broadcast news in the United States.
We started by describing discussions about social media misinformation in mainstream broadcast news (ABC, NBC, CBS) (Figure 1). The party politics discussion appeared in over a third of the mainstream broadcast news coverage about social media misinformation (36.63%). In comparison, accuracy-based discussions were less frequent: the quality of knowledge and decision discussion appeared in 23.76% of the segments and the direct corrections discussion appeared in 12.87% of the segments. As for action-based discussions, only 7.92% of mainstream broadcast news coverage about social media misinformation discussed user agency, while 56.44% discussed platform policy.
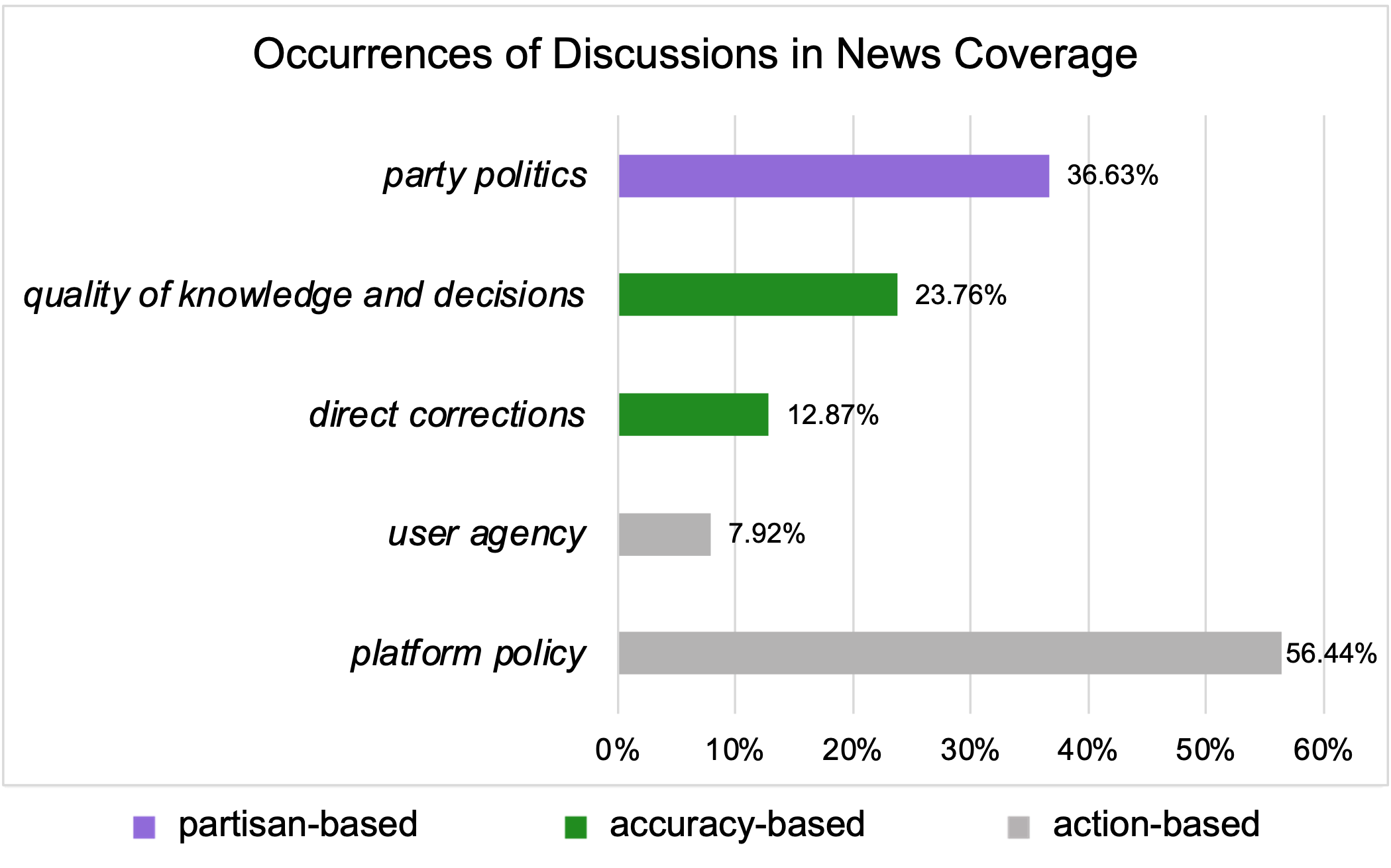
Finding 2: In mainstream broadcast news, platform policy, party politics, and accuracy concerns are concurrent rather than isolated considerations.
Table 1 illustrates the ways in which different types of discussions co-occur with each other in mainstream broadcast news. Table 1 reports both (a) the relative percentage of co-occurrence and (b) the absolute percentage of co-occurrence. The relative percentage of co-occurrence is measured by the Jaccard index (the number of news segments where two types of discussions are both present divided by the number of news segments where either type is present). The relative percentage should be the primary focus to interpret co-occurrence, as it takes account of the discussions being very rare in the whole dataset. The absolute percentage of co-occurrence is measured by the number of news segments where two types of discussions are both present divided by total number of news segments.
A main takeaway from Table 1 is that platform policy and party politics not only were the most prevalent discussions but also most frequently co-occurred with each other. On average, when news coverage does address platform policy or party politics, about a third of the times these two topics are discussed together (28.77%). For example, a CBS Evening News segment that aired on October 15, 2020, discussed how Twitter reacted faster to misinformation against Hunter Biden in 2020 than to misinformation against Hillary Clinton in 2016, both of which were endorsed by Trump campaign. By explaining how in both cases misinformation was used “to cast a cloud over the front runner in the closing weeks of the campaign” and reflecting on the previous election loss and “all the headaches” (CBS Evening News, 2020) of Democratic Party in 2016, the segment emphasized how Twitter’s actions in 2016 versus in 2020 have implications for competitive advantages of political candidates.
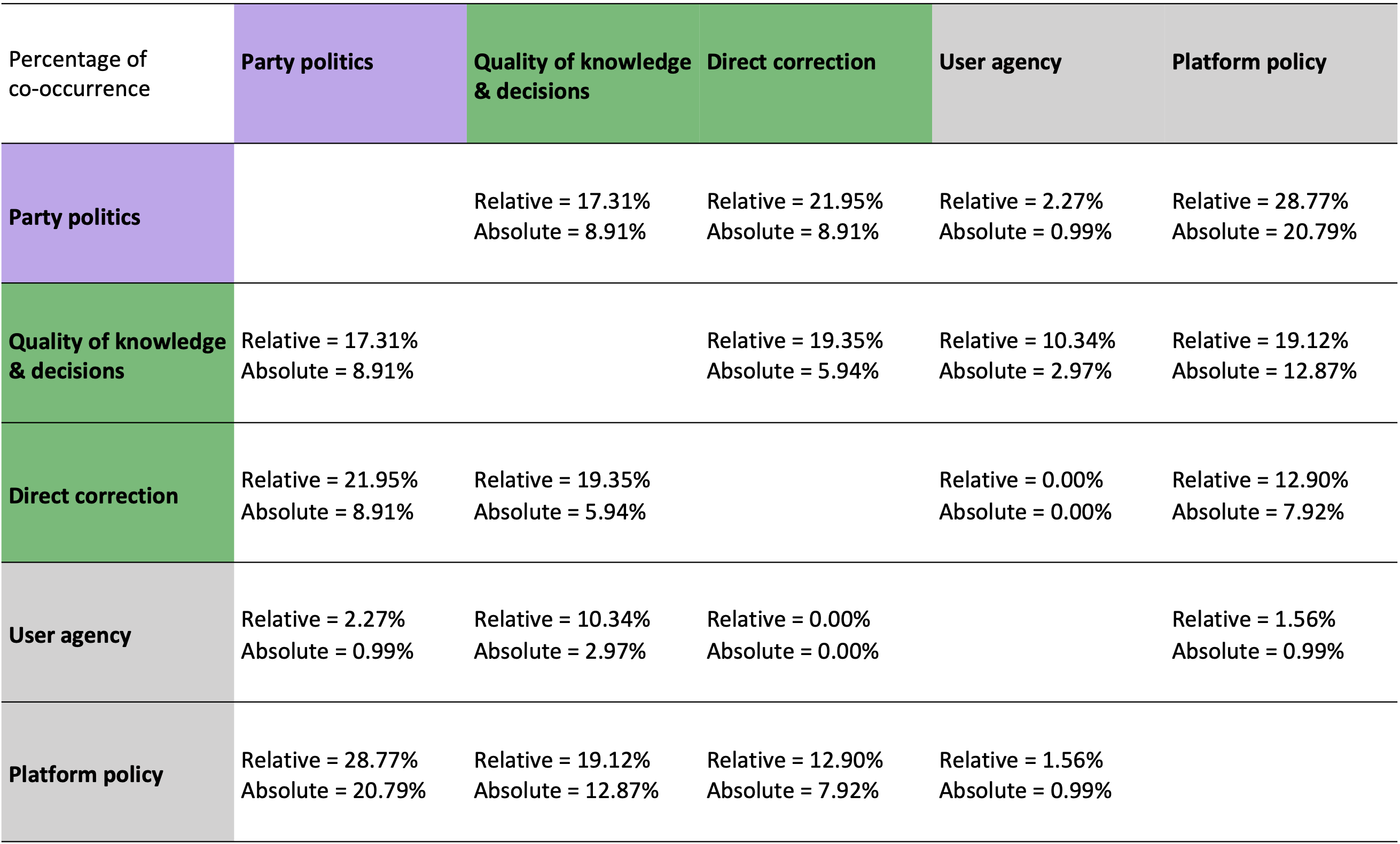
Further, the accuracy-based discussion about quality of knowledge and decisions also co-occurred with discussions about platform policy and party politics, suggesting that accuracy motivation, partisan motivation, and call-to-action can be concurrent rather than isolated considerations. For example, a segment from NBC News Today Show that aired on October 28, 2020, juxtaposed accuracy and partisan concerns when discussing platform policy:
JO LING KENT: Today, Mark Zuckerberg will face off with Congress yet again, preparing to defend the company’s strategy to protect voters in 2020 … The CEOs of Twitter and Google also testifying today saying they, too, are taking action to combat disinformation and post that may incite violence before and after Election Day. But on both sides of the aisle, some Facebook users are worried about how divisive it’s become. […]
JO LING KENT: Fifty-seven-year-old Romy Toussaint is mom to four sons. She used to love Facebook for staying in touch with family and friends, but now limits herself to fifteen minutes a day. How are you feeling about Facebook going into the 2020 election? How would you describe it?
ROMY TOUSSAINT: I don’t trust anything that I see on Facebook, and I don’t think other people should. I don’t want for my speech to be censored but I do want for Facebook to take responsibility to let people know what they think is not true. […]
JO LING KENT: On the other side, twenty-eight-year-old Molly Felling feels Facebook is biased. Some of her posts about coronavirus theories and hydroxychloroquine have been either flagged as false by the company or taken down.
MOLLY FELLING: I was shocked. I couldn’t believe it. I think when it comes to an election, it’s important to have both sides.
JO LING KENT: Do you think that Facebook is targeting conservatives? Do you think that they go too far?
MOLLY FELLING: I feel like they’re a little more left leaning. You have to trust the public to be able to like inform themselves and make their own opinions. (NBC News Today Show, 2020)
Through one interviewee’s comment that “I do want for Facebook to take responsibility to let people know what they think is not true” and by pointing to the potential for misinformation to “incite violence before and after Election Day,” the news segment noted how misinformation can impact the quality of knowledge and decisions, justifying the necessity for platform policy to be based on accuracy considerations. Meanwhile, the segment “balanced” the first soundbite with a second soundbite. By asking “Do you think that Facebook is targeting conservatives? Do you think that they go too far?” the journalist used the party politics lens to interpret platform policies, propagating the frame that Facebook’s intervention on COVID-19 misinformation was ideologically biased and “left leaning.” Notably, the story did not weigh in about whether Molly Felling’s posts were accurate.
Finding 3: On Facebook and Twitter, platform policy and party politics still dominate the discussion, but user agency discussion takes a bigger share than in news.
Turning from news to Facebook and Twitter, the distributions of discussions largely held with minor differences (Figure 2). On social media, the party politics discussion constituted an even larger part of how social media users talk about misinformation than in news coverage (38.95%). Accuracy-based discussions, including quality of knowledge and decisions (22.13%) and direct corrections (12.31%) were relatively less frequent compared to the partisan-based discussion, replicating the pattern found in news. The platform policy discussion remained the most prevalent discussion (54.85%). Finally, the discussion on user agency, although still having a small share, was twice as frequent on social media (16.06%) than in news coverage (7.92%).
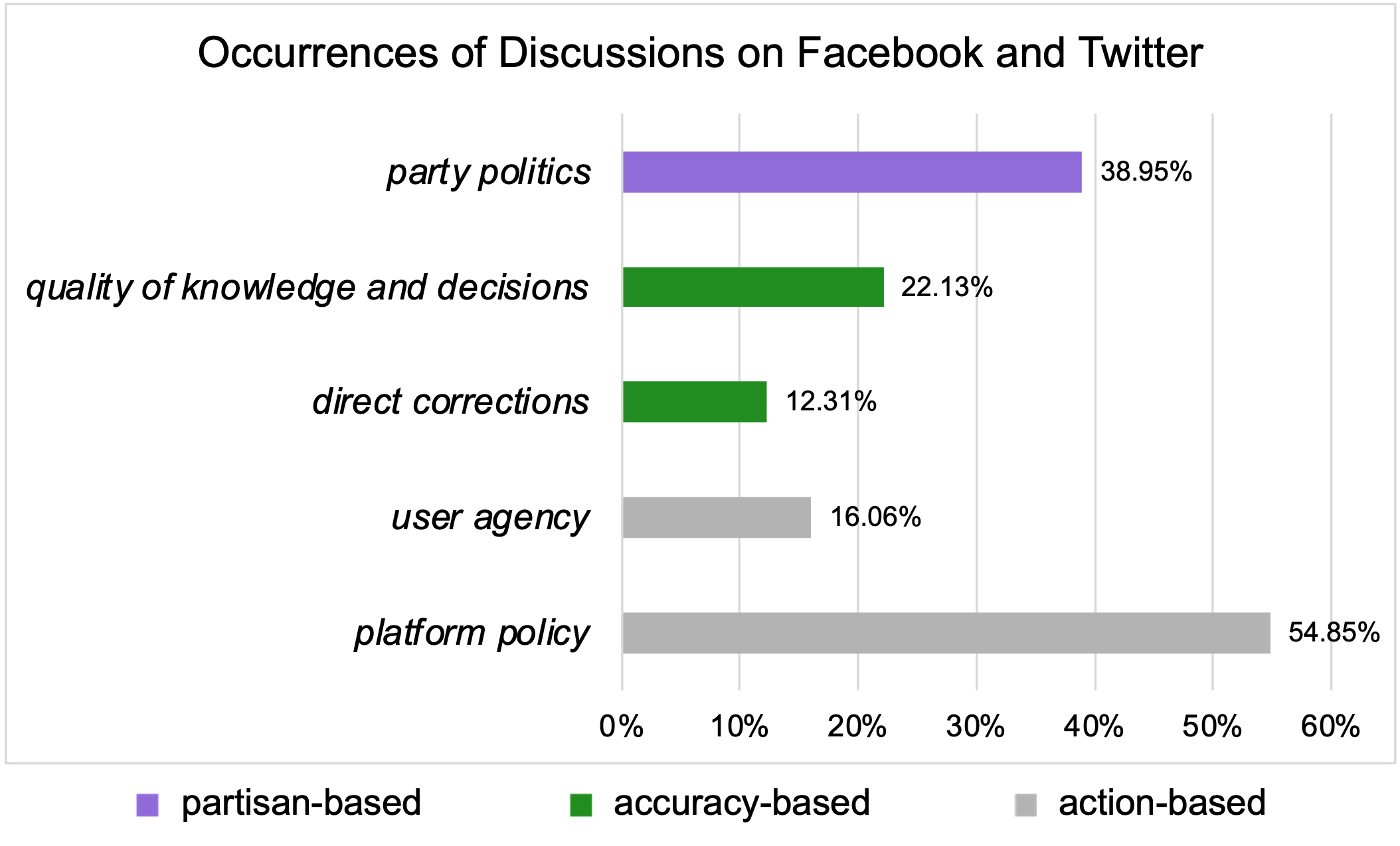
Finding 4: On Facebook and Twitter, platform policy and party politics still co-occur most frequently, while user agency and direct corrections are frequently discussed together.
Table 2 shows the ways in which different types of discussions co-occur with each other on Facebook and Twitter. The relative percentage of co-occurrence is measured by the Jaccard index (the number of posts where two types of discussions are both present divided by the number of posts where either type is present). The relative percentage should be the primary focus to interpret co-occurrence, as it takes account of the discussions being very rare in the whole dataset. The absolute percentage of co-occurrence is measured by the number of posts where two types of discussions are both present divided by total number of posts.
Similar to the pattern found in news coverage, we still observed the most frequent co-occurrence between platform policy and party politics on Facebook and Twitter (Table 2). For example, when quoting a tweet that said, “Twitter has not censored Joe Biden once but has censored Donald Trump more than 65 times,” a Twitter user wrote, “Because Donald Trump uses Twitter to spread venom, misinformation and lies. Joe Biden doesn’t. This isn’t hard.”
Quality of knowledge and decisions also had frequent co-occurrence with platform policy, replicating the pattern in news coverage (e.g., “Glad these false videos with misinformation about hydroxychloroquine are being taken down by Twitter, Facebook, and YouTube. Social media can amplify voices representing a very small minority, which in cases like this, can be deadly”). Again, these three types of discussions (platform policy, party politics, quality of knowledge and decisions) can co-occur together. For example, commenting about Facebook’s action to delete a post by Donald Trump based on its misinformation policy, a Twitter user said, “among the many errors/lies/falsehoods that Trump makes, this seems trifling. But there are a number of ways such stupidity can result in real harm, or even death. It is safer to assume that Trump is wrong, as a general rule.”
On the other hand, a difference emerged between news and social media co-occurrence findings. While the discussion about user agency did not co-occur with direct corrections in our news data, it was frequently used together with direct corrections among social media utterances. For example, one post from a public Facebook page said, “A few people have sent me a video of ‘America’s Frontline Doctors’ asking for my thoughts, so I decided to make a post. This video is dangerous. I will attempt to explain why.” It went on to offer direct corrections to hydroxychloroquine misinformation, “As more and more trials took place to study if hydroxychloroquine was effective, data started to demonstrate it was not, and it only increased risk of harm […] As of now, there is a pretty strong consensus in the medical community that hydroxychloroquine does not work for COVID and it is not a cure,” providing links to websites of National Institutes of Health and medical journals. Later, the post appealed to user agency by encouraging people to look for source credibility: “With all of that said, I invite you to consider the source of information […] When all else fails, always consider the source.” Similarly, another Facebook post offered direct corrections to election misinformation circulating in a local Facebook group claiming that polling locations will be shut down, explaining the availability of in-person voting. The post went on to discuss user agency by reminding social media users “to be extra vigilant about any voting or election information that does not come from a verified source like your county clerk.” Another post combined direct corrections and user agency by alerting people of impersonating social media accounts and asking social media users to act on it (e.g., “I need help from all of you to report these fake accounts”).
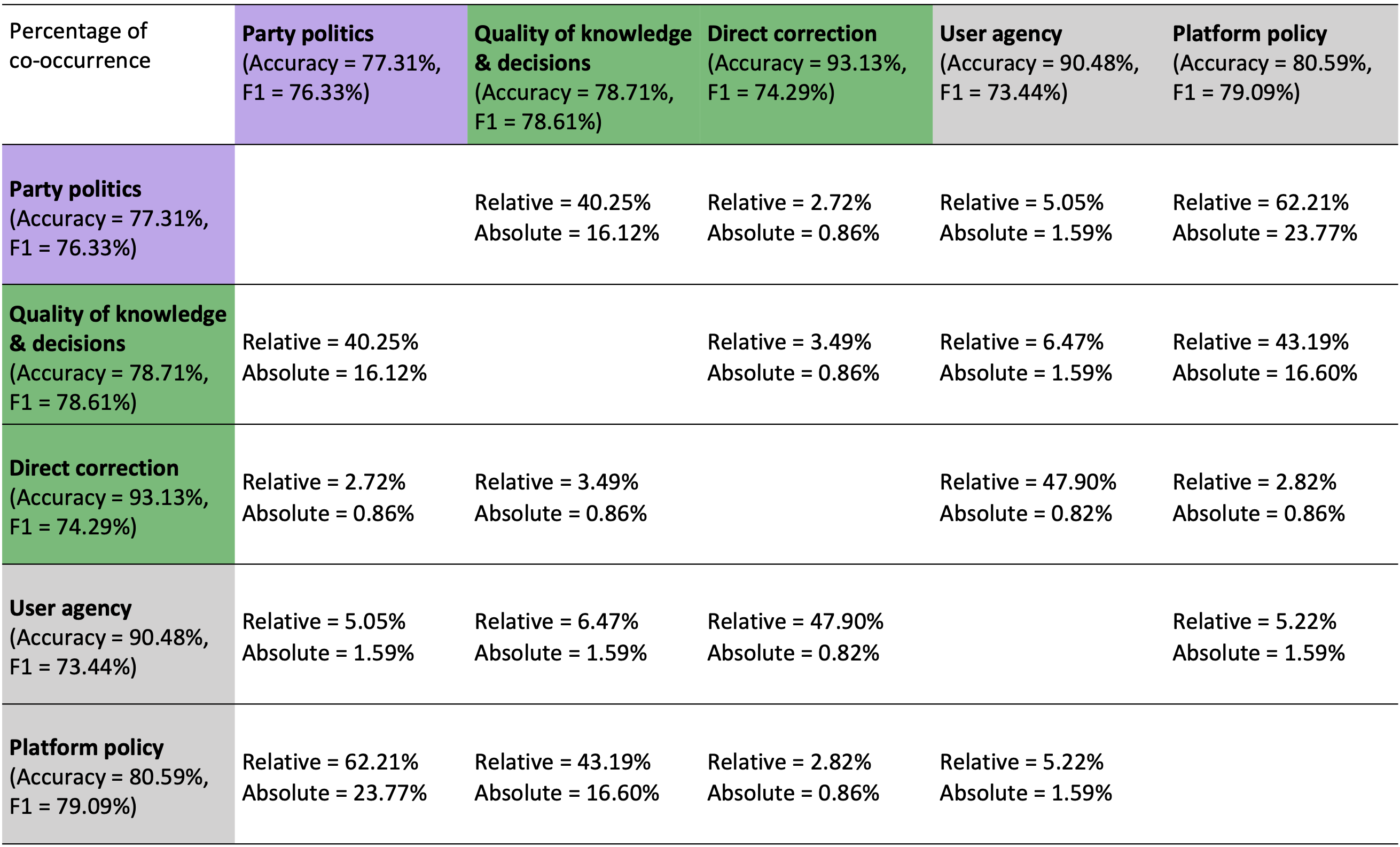
Methods
To examine news coverage, we collected news transcripts discussing the topic of social media misinformation from three major broadcast networks, ABC, NBC, and CBS (n = 101) using relevant keywords.1(“social media” OR “Facebook” OR “Twitter”) AND (“misinformation” OR “disinformation” OR “fake news” OR “fake stories” OR “fake story” OR “fake information” OR “false news” OR “false stories” OR “false story” OR “false information” OR “made-up news” OR “made up news” OR “made-up stories” OR “made up stories” OR “made-up story” OR “made up story” OR “made-up information” OR “made up information”) These sources were chosen based on their large audience size as well as on being broadly recognized as “mainstream media” even among partisans (Pew Research Center, 2021; Shearer & Mitchell, 2021); future work should expand beyond these sources in the centrist and center-left media ecosystem (Benkler et al., 2018) and examine sources with a wider range of ideological perspectives.2Scholars disagree about the extent to which the three major networks are left-leaning and report considerable diversity between the three networks. Groseclose and Milyo’s (2005) influential work on media bias found, “The sixth and seventh most centrist outlets are ABC World News Tonight and NBC Nightly News” (p. 1221) and that the CBS Evening News was quite liberal. Groeling (2008) found evidence of left-leaning bias in NBC and CBS newscasts using favorable poll coverage of Bill Clinton, but found that ABC was more likely to report favorable polls for any president studied. Bernhardt et al. (2023) found more evidence of an anti-government bias than an ideological bias, with network news coverage becoming more critical of the occupant of the White House, which switched from George W. Bush to Barack Obama during the time period they analyzed. Hassell et al. (2020) found no evidence of liberal bias in what mainstream news media chose to cover.
To examine social media discussions, we collected posts discussing topics relevant to social media misinformation from public Facebook pages and groups (n = 22,578) and from Twitter (n = 302,404) using relevant keywords.3(“social media” OR “Facebook” OR “Twitter” OR “this platform”) AND (“misinformation” OR “disinformation” OR “fake news” OR “fakenews” OR “fake stories” OR “fake story” OR “fake information” OR “false news” OR “false stories” OR “false story” OR “false information” OR “made-up news” OR “made up news” OR “made-up stories” OR “made up stories” OR “made-up story” OR “made up story” OR “made-up information” OR “made up information”) Facebook posts containing keywords were collected from CrowdTangle, a Meta-owned research tool that archived all posts from Facebook pages and groups that were set as public. Twitter posts containing keywords were collected from Synthesio, a third-party commercial data vendor. We only collected English-language posts with a U.S. geographical location, but the keyword-based social media data were not representative of U.S. general population. We then eliminated content posted by news media and journalists from the social media data (see Appendix for details) and the final data consisted of 15,578 posts from public Facebook pages and groups and 301,334 posts from Twitter. The timeframe for both news and social media data was July 28 to December 3, 2020.
Two undergraduate coders labeled a random sample of 1,168 unique social media posts and the entire corpus of 101 news transcripts. Krippendorff’s α was 0.85 for party politics, 0.94 for quality of knowledge and decisions, 0.92 for direct corrections, 0.76 for user agency, and 0.90 for platform policy. We used the Hopkins-King method to describe the proportions of discussions as research shows that it produces unbiased estimates for category proportions compared to individual classifiers (Hopkins & King, 2010). While the Hopkins-King method is superior in estimating category proportions, it does not offer information on how different discussions co-occur with each other because it does not classify individual documents. Thus, we tested three individual classifiers (Naïve Bayes, Support Vector Machine, Random Forest). Random Forest outperformed Support Vector Machine and Naïve Bayes in all discussions, producing an F1 score of 76.33% when classifying party politics, an F1 score of 78.61% when classifying quality of knowledge and decisions, an F1 score of 74.29% when classifying direct corrections, an F1 score of 73.44% when classifying user agency, and an F1 score of 79.09% when classifying platform policy.
Topics
Bibliography
Aghajari, Z., Baumer, E. P. S., & DiFranzo, D. (2023). Reviewing interventions to address misinformation: The need to expand our vision beyond an individualistic focus. Proceedings of the ACM on Human-Computer Interaction, 7(CSCW1), 1–34. https://doi.org/10.1145/3579520
Allen, J., Howland, B., Mobius, M., Rothschild, D., & Watts, D. J. (2020). Evaluating the fake news problem at the scale of the information ecosystem. Science Advances, 6(14), eaay3539. https://doi.org/10.1126/sciadv.aay3539
Barthel, M., Mitchell, A., and Holcomb, J. (2016, December 15). Many Americans believe fake news is sowing confusion. Pew Research Center. https://www.pewresearch.org/journalism/2016/12/15/many-americans-believe-fake-news-is-sowing-confusion/
Bennett, W. L. (1990). Toward a theory of press-state relations in the United States. Journal of Communication, 40(2), 103–127. https://doi.org/10.1111/j.1460-2466.1990.tb02265.x
Benkler, Y., Faris, R., & Roberts, H. (2018). Network propaganda: Manipulation, disinformation, and radicalization in American politics. Oxford University Press.
Bernhardt, L., Dewenter, R., & Thomas, T. (2023). Measuring partisan media bias in US newscasts from 2001 to 2012. European Journal of Political Economy, 78, 102360. https://doi.org/10.1016/j.ejpoleco.2023.102360
Bode, L., & Vraga, E. K. (2015). In related news, that was wrong: The correction of misinformation through related stories functionality in social media. Journal of Communication, 65(4), 619–638. https://doi.org/10.1111/jcom.12166
Bode, L., Vraga, E. K., & Tully, M. (2020). Do the right thing: Tone may not affect correction of misinformation on social media. Harvard Kennedy School (HKS) Misinformation Review, 1(4). https://doi.org/10.37016/mr-2020-026
Butler, L. H., Prike, T., Ecker, U. K. H. (2023). Nudge-based misinformation interventions are effective in information environments with low misinformation prevalence. Research Square. https://doi.org/10.21203/rs.3.rs-3736230/v1
CBS Evening News (2020, October 15). Hunter Biden tabloid story raises disinformation campaign fears [TV news transcript]. Nexis Uni. https://advance.lexis.com/api/document?collection=news&id=urn:contentItem:612Y-X761-DXH2-61R4-00000-00&context=1516831
de Cock Buning, M. (2018). A multi-dimensional approach to disinformation: Report of the independent high level group on fake news and online disinformation. Publications Office of the European Union. https://op.europa.eu/en/publication-detail/-/publication/6ef4df8b-4cea-11e8-be1d-01aa75ed71a1/language-en
Duan, Z., Li, J., Lukito, J., Yang, K.-C., Chen, F., Shah, D. V., & Yang, S. (2022). Algorithmic agents in the hybrid media system: Social bots, selective amplification, and partisan news about COVID-19. Human Communication Research, 48(3), 516–542. https://doi.org/10.1093/hcr/hqac012
Dwoskin, E., & Timberg, C. (2021, January 16). Misinformation dropped dramatically the week after Twitter banned Trump and some allies. The Washington Post. https://www.washingtonpost.com/technology/2021/01/16/misinformation-trump-twitter/
González-Bailón, S., Lazer, D., Barberá, P., Zhang, M., Allcott, H., Brown, T., Crespo-Tenorio, A., Freelon, D., Gentzkow, M., Guess, A. M., Iyengar, S., Kim, Y. M., Malhotra, N., Moehler, D., Nyhan, B., Pan, J., Rivera, C. V., Settle, J., Thorson, E., … Tucker, J. A. (2023). Asymmetric ideological segregation in exposure to political news on Facebook. Science, 381(6656), 392–398. https://doi.org/10.1126/science.ade7138
Gotfredsen, S. G. & Mehta, D. (2023). journalists-twitter-activity. Github. https://github.com/TowCenter/journalists-twitter-activity
Groeling, T. (2008). Who’s the fairest of them all? An empirical test for partisan bias on ABC, CBS, NBC, and Fox News. Presidential Studies Quarterly, 38(4), 631–657. https://doi.org/10.1111/j.1741-5705.2008.02668.x
Groseclose, T., & Milyo, J. (2005). A measure of media bias. The Quarterly Journal of Economics, 120(4), 1191–1237. https://doi.org/10.1162/003355305775097542
Guess, A. M., Nyhan, B., & Reifler, J. (2020). Exposure to untrustworthy websites in the 2016 US election. Nature Human Behaviour, 4(5), 472–480. https://doi.org/10.1038/s41562-020-0833-x
Hassell, H. J. G., Holbein, J. B., & Miles, M. R. (2020). There is no liberal media bias in which news stories political journalists choose to cover. Science Advances, 6(14), eaay9344. https://doi.org/10.1126/sciadv.aay9344
Hopkins, D. J., & King, G. (2010). A method of automated nonparametric content analysis for social science. American Journal of Political Science, 54(1), 229–247. https://doi.org/10.1111/j.1540-5907.2009.00428.x
Jerzak, C. T., King, G., & Strezhnev, A. (2022). An improved method of automated nonparametric content analysis for social science. Political Analysis, 31(1), 42–58. https://doi.org/10.1017/pan.2021.36
Li, J., & Su, M.-H. (2020). Real talk about fake news: Identity language and disconnected networks of the US public’s “fake news” discourse on Twitter. Social Media + Society, 6(2), 2056305120916841. https://doi.org/10.1177/2056305120916841
Lwin, M. O., Lee, S. Y., Panchapakesan, C., & Tandoc, E. (2023). Mainstream news media’s role in public health communication during crises: Assessment of coverage and correction of COVID-19 misinformation. Health Communication, 38(1), 160–168. https://doi.org/10.1080/10410236.2021.1937842
McClure Haughey, M., Muralikumar, M. D., Wood, C. A., & Starbird, K. (2020). On the misinformation beat: Understanding the work of investigative journalists reporting on problematic information online. Proceedings of the ACM on Human-Computer Interaction, 4(CSCW2), 1–22. https://doi.org/10.1145/3415204
NBC News Today Show (2020, October 28). Facebook’s Mark Zuckerberg and other social media CEOs facing tough questions on Capitol Hill [TV news transcript]. Nexis Uni. https://advance.lexis.com/api/document?collection=news&id=urn:contentItem:61BW-5511-JB20-G371-00000-00&context=1516831
Nelson, J. L., & Lewis, S. C. (2023). Only “sheep” trust journalists? How citizens’ self-perceptions shape their approach to news. New Media & Society, 25(7), 1522–1541. https://doi.org/10.1177/14614448211018160
Pennycook, G., Epstein, Z., Mosleh, M., Arechar, A. A., Eckles, D., & Rand, D. G. (2021). Shifting attention to accuracy can reduce misinformation online. Nature, 592(7855), 590–595. https://doi.org/10.1038/s41586-021-03344-2
Pew Reserch Center. (2021, July 13). Network news fact sheet. https://www.pewresearch.org/journalism/fact-sheet/network-news/
Saltz, E., Barari, S., Leibowicz, C., & Wardle, C. (2021). Misinformation interventions are common, divisive, and poorly understood. Harvard Kennedy School (HKS) Misinformation Review, 2(5). https://doi.org/10.37016/mr-2020-81
Shearer, E. & Mitchell, A. (2021, May 7). Broad agreement in U.S. – even among partisans – on which news outlets are part of the ‘mainstream media.’ Pew Research Center. https://www.pewresearch.org/short-reads/2021/05/07/broad-agreement-in-u-s-even-among-partisans-on-which-news-outlets-are-part-of-the-mainstream-media/
Shin, D., Kee, K. F., & Shin, E. Y. (2023). The nudging effect of accuracy alerts for combating the diffusion of misinformation: Algorithmic news sources, trust in algorithms, and users’ discernment of fake news. Journal of Broadcasting & Electronic Media, 67(2), 141–160. https://doi.org/10.1080/08838151.2023.2175830
Taber, C. S., & Lodge, M. (2006). Motivated skepticism in the evaluation of political beliefs. American Journal of Political Science, 50(3), 755–769. https://doi.org/10.1111/j.1540-5907.2006.00214.x
Tromble, R., & McGregor, S. C. (2019). You break it, you buy it: The naiveté of social engineering in tech —and how to fix it. Political Communication, 36(2), 324–332. https://doi.org/10.1080/10584609.2019.1609860
Vraga, E., Tully, M., & Bode, L. (2020). Empowering users to respond to misinformation about Covid-19. Media and Communication, 8(2), 475-479. https://doi.org/10.17645/mac.v8i2.3200
Xiang, H., Zhou, J., & Wang, Z. (2023). Reducing younger and older adults’ engagement with COVID-19 misinformation: The effects of accuracy nudge and exogenous cues. International Journal of Human–Computer Interaction, 1–16. https://doi.org/10.1080/10447318.2022.2158263
Zoizner, A. (2021). The consequences of strategic news coverage for democracy: A meta-analysis. Communication Research, 48(1), 3–25. https://doi.org/10.1177/0093650218808691
Funding
This research was assisted by the Social Science Research Council’s Social Data Research and Dissertation Fellowships, with funds provided by Omidyar Network. This research was also supported by the John S. and James L. Knight Foundation.
Competing Interests
The authors declare no competing interests.
Ethics
Institutional Review Board approval was not required for this study. This project collects publicly available data on news channels, Twitter, and public Facebook groups and pages. Data are reported on the aggregate level and anonymously. To avoid reverse identification of social media users, quotes from Twitter and Facebook posts are presented with minor editing in language and light paraphrasing while keeping substantial content.
Copyright
This is an open access article distributed under the terms of the Creative Commons Attribution License, which permits unrestricted use, distribution, and reproduction in any medium, provided that the original author and source are properly credited.
Data Availability
All materials needed to replicate this study are available via the Harvard Dataverse: https://doi.org/10.7910/DVN/KZYJF6.
Following Twitter’s and Facebook’s data sharing policy, we only share Tweet IDs and public Facebook post IDs (collected from public Facebook pages and public Facebook groups via CrowdTangle) for academic research purposes. Regarding mainstream news media data, TV news transcripts are not shared due to copyright restrictions.
Acknowledgements
The authors gratefully acknowledge insightful and helpful comments from Dhavan V. Shah, Sijia Yang, Chris Cascio, Michael Xenos, and Katherine J. Cramer.