Peer Reviewed
COVID-19 misinformation and the 2020 U.S. presidential election
Article Metrics
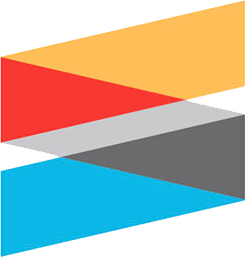
42
CrossRef Citations
Altmetric Score
PDF Downloads
Page Views
Voting is the defining act for a democracy. However, voting is only meaningful if public deliberation is grounded in veritable and equitable information. This essay investigates the politicization of public health practices during the Democratic primaries in the context of the 2020 U.S. presidential election, using a dataset of more than 67 million tweets. We find the public sphere on Twitter is politically heterogeneous and the majority—liberal and conservative alike—advocates for wearing masks and vote-by-mail. However, a small, but dense group of conservative users push anti-mask and voter fraud narratives.
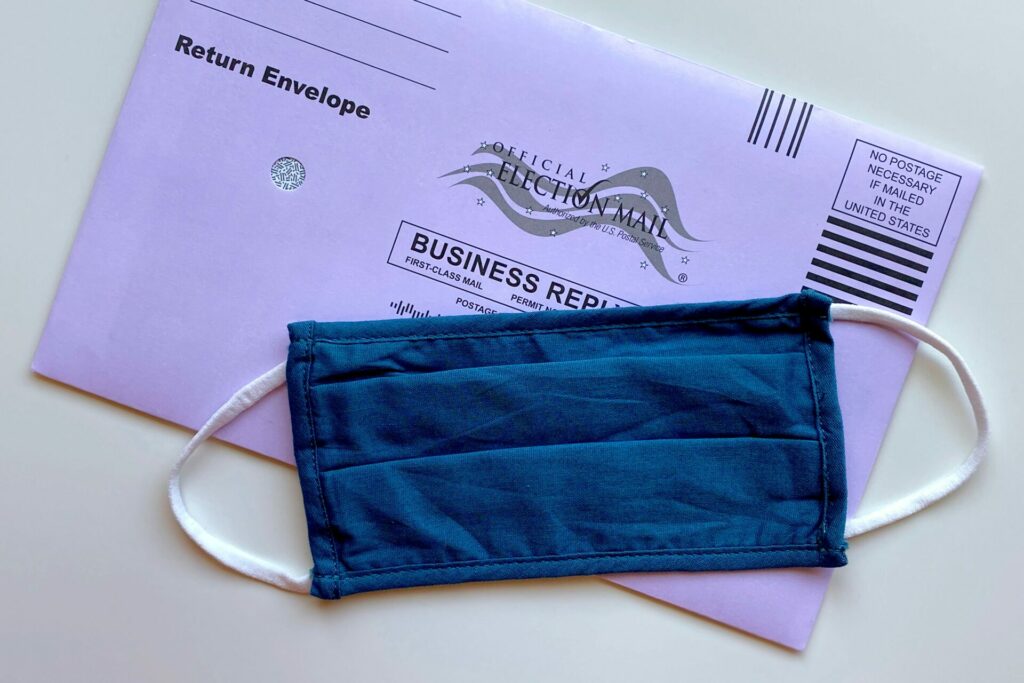
Research Questions
- What are the main COVID-19 misinformation narratives impacting the Democratic primaries and how do they evolve over time?
- To what extent do network topological differences vary based on party affiliation or intra-party tendency to share misinformation?
- Do we observe polarization at the network level? Is there political alignment in the spread of COVID-19 misinformation?
Essay Summary
- This essay explores different COVID-19 misinformation narratives occurring in the context of the 2020 U.S. Democratic primaries, using a subset of over 67 million tweets during the time frame March 1, 2020 through August 30, 2020 from an ongoing U.S. presidential elections and an ongoing COVID-19 Twitter dataset that we are collecting (Chen et al., 2020a; Chen et al., 2020b). We infer user geolocation (Jiang et al., 2020) and conduct temporal content analysis and network analysis on public health narratives originating in the United States.
- Two major misinformation narratives occur at the intersection of the COVID-19 pandemic and the 2020 U.S. Democratic primaries: the use of masks and the legitimacy of mail-in ballots. Whereas misinformation can arise from any community, health misinformation is associated with specific communities.
- A large and expansive cluster of politically heterogeneous users (both liberal and conservative) advocate for wearing masks and mail-in voting. A small but dense cluster of conservative users pushes misinformation about the inefficacy of masks and potential for voter fraud.
- This study identifies one of the sources of amplification of misinformation during the COVID-19 pandemic regarding public health practices and election integrity. We also suggest ways politicized health messages have impacted the most recent 2020 Democratic primaries.
- A narrative’s potential to be misinformation drives politicization of information just as much as misinformation itself does.
Implications
Voting is the defining act for a democracy. However, this action is only meaningful if public deliberation and decision-making are grounded in veritable and equitable information. Studying the possible effects of misinformation on voting behavior is thus a critical avenue of investigation even as we look back on the recent 2020 US presidential election cycle. This essay examines how health misinformation may be politicized – particularly how political alignment mediates the spread of COVID-19 misinformation.
It is useful to first disambiguate misinformation and disinformation, particularly in the context of politicization, or the use of information for political means. Misinformation is the spread of false information agnostic of intent, while disinformation is the intentional spread of false information. Disinformation campaigns often originate from specific institutions, such as intelligence agencies; however, these campaigns can also emerge spontaneously in online communities.
In this study, we focus on the politicization of COVID-19-related health misinformation and its spread and further analyze two of the most critical narratives during the 2020 US Democratic primary cycle:
- The legality and fraudulence of voting by mail
- The efficacy of masks
Although there have been widespread misinformation campaigns to convince the populace otherwise, the practice of mail-in voting has already been adopted by several U.S. states and has not been shown to be prone to or affected by significant fraud (Qiu, 2021; Spencer, 2020). The presence of disputed and disproven anti-mask rhetoric on popular social media platforms may adversely affect voter turnout due to health concerns and accessibility to mail-in ballot resources. Research investigating the interplay between misinformation and voting behavior reports conflicting results: for example, using random dial-in questionnaires, researchers found that both misinformation and factual information increase voter participation (White et al., 2006). Others focused on issues such as immigration, race, unemployment, and abortion. Automated phone calls (‘robocalls’) in Canada that contained misleading information about the location of polling stations resulted in a 3% average decrease in participation (Kessler et al., 2013).
These examples demonstrate the nuances in the effects of misinformation, as its transmission modality and type (e.g., political) may influence voter behavior. Today, the modality of interest has shifted from phones to social media, due to its ubiquitous presence. Efforts, spearheaded by the Russian Internet Research Agency (IRA) and others, to deliberately manipulate social media discourse have been well documented both in the 2016 U.S. presidential election (Bessi et al., 2016) and the 2017 French presidential election (Ferrara, 2017). The IRA appeared to have identified and targeted non-white voters (Badawy et al., 2019) months before the election with messages promoting racial identity (Dutt et al., 2018) that may have led to voter suppression (Kim et al., 2018), and certainly sowed division and conflict online (DiResta et al., 2019).
Many political scientists believe that an increase in information leads to electoral participation (Carpini et al., 1996). However, those who lack sufficient information tend to align with “opinion leaders” by following perceptions of knowledge or partisanship (Katz et al., 1966). Other factors, such as directionally motivated reasoning (Flynn et al., 2017), selective exposure (Guess et al., 2018), and correction-induced misperceptions (Nyhan et al., 2010), may also play a role in individual perception. With the COVID-19 pandemic characterized by uncertainty about the disease and best welfare practices, the public is vulnerable to partisan-driven misinformation at the intersection of public health and politics.
Recently, Jost and colleagues (Jost et al., 2018) noted that conservatives, in general, maintain more homogeneous social networks that are more conducive to the flow of misinformation, which would not only make them more vulnerable, but also generate dangerous cascade effects to the general public. Furthermore, prior studies have shown the elderly population engaging more with misinformation during the 2016 U.S. presidential election (Grinberg et al., 2019). As such, the elderly is one of the most susceptible populations to both digital misinformation and COVID-19 health complications.
The COVID-19 pandemic presents a novel chance to assess where health misinformation becomes political (Ferrara, 2020). While misinformation is the current label for our “narratives”, the importance in our study, beyond the truth value, is its political impact. The 1918 Spanish Flu has been shown to have generated political extremism that led to higher votes for the Nazi party in areas with more pandemic deaths. The term of choice at the time was propaganda, but the meaning is the same: the deliberate spread of (mis)information to influence elections. In fact, the term misinformation has become so prevalent that it has become core to candidates’ campaign strategies (such as Donald J. Trump’s use of “fake news” to discredit the media). These narratives may be misinformation, and that possibility, rather than factuality itself, is what makes them effective in politics.
In this study, we investigate two major narratives incubated within the COVID-19 discourse and their interplay with the Democratic primary online chatter on Twitter from March 1, 2020 through August 30, 2020. Upon isolating two health-related narratives prone to misinformation, namely the use of masks in public and the issue of mail-in ballots, we show how mask-related discourse grows with discourse about voting. We find that instances of health-related misinformation continue to circulate after their initial reporting, and a common strategy is to use true stories to drive larger misinformation narratives. Topologically, a large and expansive cluster of politically heterogeneous users constitutes the majority of the public sphere on Twitter, and this group, in general, advocates for wearing masks and mail-in voting. In contrast, a small but dense cluster of conservative users pushes misinformation about the inefficacy of masks and voter fraud. We show that while misinformation, in general, can arise from any point in the network, there is a clear division between communities that spread mail-in ballot and mask misinformation and those that do not.
Findings
Finding 1: Four overarching themes regarding health policies and voting procedures emerged in our data set.
- We first find, as expected, that Coronavirus discourse dominates much of the Democratic primary discussion during our observation period. This includes rulings by the United States Supreme Court surrounding religious gatherings to allegations that the Coronavirus is a hoax perpetrated by the Democratic party (Blue dotted line, Figure 1).
- We then identify a second narrative surrounding mail-in ballots and the role the United States Postal Service (USPS) played in the distribution and collection of these ballots. In August, The Washington Post, along with many other news organizations, reported that Postmaster General Louis DeJoy had restructured the postal office and reallocated funding, leading to slower ballot delivery and returns during the primaries, with ramifications stretching beyond the Democratic primaries into the presidential elections (Red solid line, Figure 1).
- We also find that there is general discourse surrounding imposed lockdowns, their efficacy, and constitutionality, as the United States faced a second wave during the summer of 2020 (Orange dotted line, Figure 2).
- Finally, we observe numerous tweets surrounding masks and face coverings, with a large number of tweets perpetuating the messaging that masks are a hoax and are ineffective (Purple solid line, Figure 2).
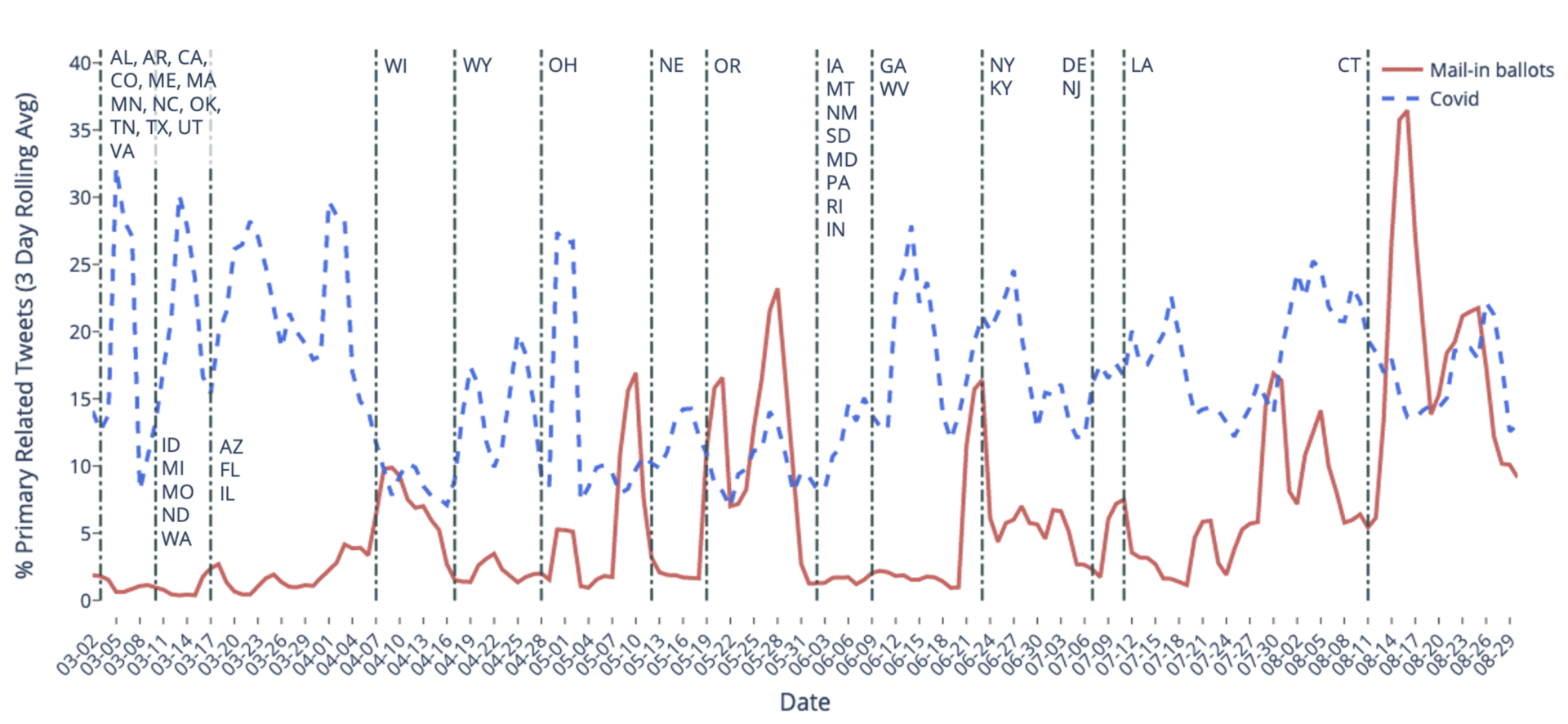
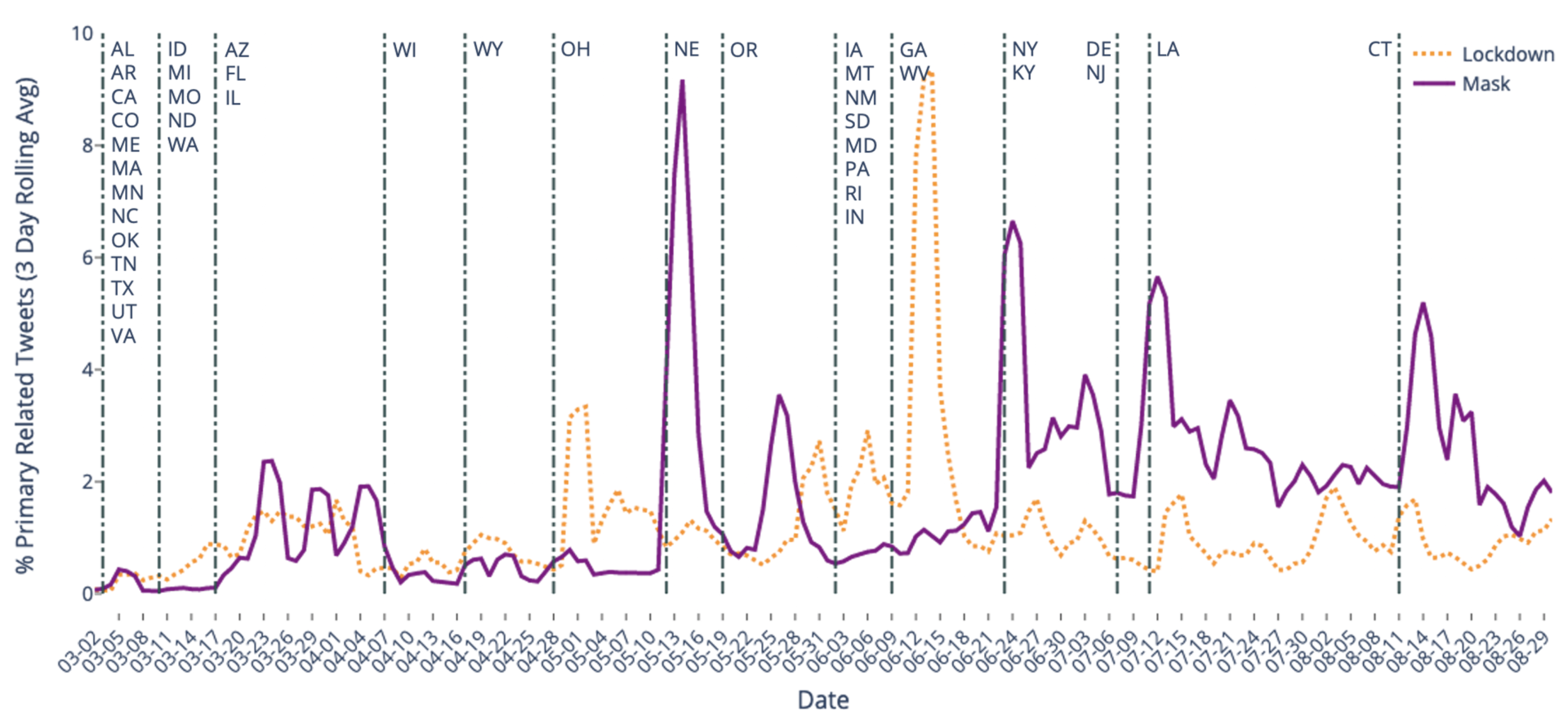
Due to the nature of our dataset and research questions, it is unsurprising that COVID-19 is salient throughout our dataset. Several narratives emerge under the umbrella of COVID-19, with some of the most vocal believing that COVID-19 is a hoax pushed by the Democratic party or that the threat of COVID-19 had already passed. We also find that Hydroxychloroquine (HCQ) and the injection of household disinfectants began to circulate, largely due to Trump announcing that he was actively taking the former as a preventative measure and suggesting that the latter might be worth further scientific investigation as a potential way to combat COVID-19 (Oprysko, 2020).
The controversy around HCQ, in particular, emphasizes the constant evolution of the factuality of a claim. This also motivates our focus on politicization rather than on only factuality. In March, there had initially been suggestions that HCQ may have been effective against COVID-19, prompting the U.S. Food and Drug Administration (FDA) to issue an emergency use authorization (EUA) for HCQ. However, as more clinical reports and studies were conducted, it became apparent that the drug commonly used to treat malaria was not effective in treating COVID-19. The FDA rescinded its recommendation and eventually EUA in April and June respectively, and the World Health Organization removed it from their coronavirus treatment trials (Bull-Otterson et al., 2020; Edwards, 2020; World Health Organization, 2020). We note that the initial effectiveness of HCQ against COVID-19 was unclear due to lack of evidence, but as more evidence showed that HCQ was in fact ineffective, this mirrors the change in factuality of HCQ as a treatment in the context of COVID-19 over time. Despite this, this narrative’s political use was evident, regardless of its validity. This demonstrates the dangers of the spread of unverified health-related news stories on social media prior to reaching medical consensus regarding the validity of the story. We also see that the use of these narratives can continue long after their initial reporting.
We also find that the topic of mail-in ballots become more prominent throughout our observation period. During the pandemic, to mitigate transmission risks, many voters began to contemplate voting by mail instead of voting in person. However, after DeJoy’s changes to the USPS, Democrats began to call for investigations into these policy changes due to the potential implications they had on not only the primaries but also the U.S. presidential elections (Bogage et al., 2020). There were also many campaigns that claimed mail-in voting would increase voter fraud, a claim that has been deemed false by FactCheck multiple times since mid-April (Farley, 2020). This discourse increased in volume and representation in our dataset after Bernie Sanders conceded to Joe Biden on April 8, 2020, as the focus of the Democratic party shifted from the primaries to the upcoming presidential race.
Discourse surrounding social distancing, stay-at-home orders, and masks in the context of voting begins as early as mid-March and continues to attract attention over time. It then builds significant traction right after April, when multiple states held their primaries or decided to postpone them, implying that voting, social distancing, and mask discourse are largely event driven. The U.S. faced a second wave during the summer of 2020, which could explain the spikes in references to lockdowns and stay-at-home orders that initially beginning to relax but were reimposed in response to the summer spike in certain parts of the country (Wilson, 2020; “As U.S. Coronavirus Cases Hit 3.5 Million, Officials Scramble to Add Restrictions,” 2020).
Finding 2: We find that there exists a clear political and content polarization in the retweet user network topology.
We consider political polarization and a user’s history of spreading misinformation, as shown in Figure 3, below. In this network, we focus our attention on users, represented by nodes, who have tweeted about mail-in voting and mask-wearing. We constructed weighted directed edges between users, based on the number of interactions they had with each other (specifically retweets and original tweets). Figure 3 was generated first using node2vec (Grover and Leskovec, 2016), which represents social networks in high dimensional space. A two-dimensional layout was then extracted using the t-SNE algorithm (Maaten and Hinton, 2008).
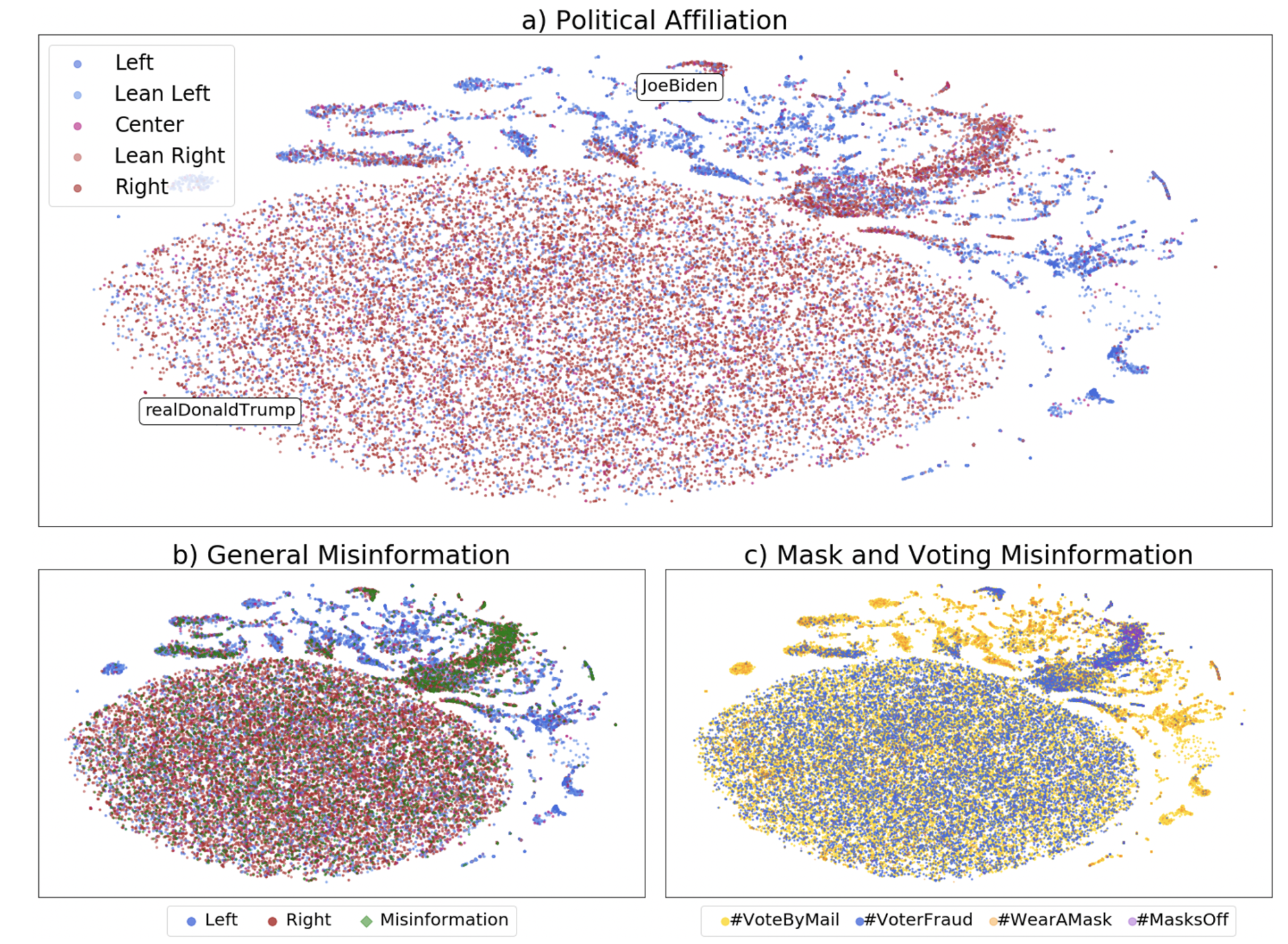
In Figure 3a), we observe a clear topological division between blue and red clusters in the top. By network topology, we refer to how nodes in the network are arranged, and how their embed-dings are spaced and clustered (such as when represented in a two-dimensional visualization). In much of the public Twitter sphere, there is a heterogeneous cluster of users that has a well-mixed political news diet. The appearance of multiple, homogenous clusters indicates the presence of extreme political polarization. Users predominantly identify as center and left-leaning, but there is a large cluster of conservative users in the upper right. This cluster is significantly denser and more homogeneous—we refer to this as the dense conservative cluster. Note that two nodes are plotted closer if they have a higher edge-weight (interact more frequently). As a result, groups of users with shared connections will be visualized closer together. While exact heterophily scores are possible, this would require labeling through community detection and merits the full scope of a separate study.
Figures 3b) and 3c) show this data augmented with misinformation tags. Figure 3b) shows users (green) that have previously shared articles from questionable domains containing misinformation, as defined by Media Bias-Fact Check (Zandt, n.d.). We observe that misinformation is spread in both clusters and across a mixture of political affiliations; however, a significant amount arises from the conservative cluster on the upper right. Figure 3c) further shows the distribution of four narrative positions, best represented by the hashtags in Table A1 (see Appendix Part B, “Tagging public health misinformation”). As we discuss in the methods section, we leverage manual annotation to isolate misinformation and factual tweets, and then find co-occurring hashtags and terms to identify a larger set of tweets that align with the following positions:
- WearAMask (Coral): This policy position includes support for mask-wearing.
- MasksOff (Purple): This policy position rejects mask-wearing as necessary and purports that the usage of masks is detrimental to one’s health.
- VoteByMail (Gold): This policy position supports voting by mail.
- VoterFraud (Blue): This policy position suggests that increased voting by mail efforts leads to a subsequent and highly correlated increase in voter fraud.
In the discourse about mask-wearing and voting by mail, we observe a clearer division. Whereas most users are predominantly marked by advocacy for mask-wearing and voting by mail, the denser conservative cluster pushes almost exclusively anti-mask wearing discourse and equates voting by mail to voter fraud. It is important to not see this as a reductive division across partisan lines. Figure 3b) shows misinformation can be spread by any user; however, the conservative clusters spread significantly more misinformation. Figure 3c) shows the majority of users from across party lines advocate for confirmed public health practices and safety precautions around voting. Interestingly, even within the dense conservative cluster, sub-communities emerge for which anti-mask or voter fraud discourse takes precedent.
In sum, the public sphere of users on Twitter engaged in conversation on COVID-19 and the primaries take on a specific topology. There is a heterogeneous user-base comprising of a loosely connected majority. In contrast, a dense network of conservative users emerges, disjoint from the majority, which affirms Jost and colleagues’ observation that there exist higher levels of homogeneity amongst certain conservative populations (Jost et al., 2018). This dense group demonstrates a propensity to politicize health-related misinformation.
We find the top COVID-19 narratives, when tweeted during the 2020 Democratic primaries, to be highly politicized. We observe that it is not only the factual basis but also the potential for misinformation that contributes to the politicization of information online. For instance, one of the mask narratives stated that there was an N95 mask shortage in the US because the Obama administration had neglected to maintain the stockpile. This was denied by some left-leaning users but is actually true (Sherman, 2020). On the other hand, mask-related misinformation seemed to be pushed exclusively from the dense group of conservative users, which suggests selective exposure to fake news. In hindsight of the Democratic primaries and now the 2020 U.S. presidential elections, this paper provides a birds-eye view and warning on how misinformation and the potential to be perceived as misinformation may galvanize further politicization of surrounding public health policies.
Methods
Data curation
We leverage our public COVID-19 Twitter dataset (Chen et al., 2020) and U.S. presidential elections Twitter dataset (Chen et al., 2020) for this study, as Twitter provides a platform for users to engage in conversation surrounding events in real-time. Collection for the former dataset began in late January 2020, while the latter began in May 2019. At the time of this writing, we only had processed our elections data from March 2020 onwards, and so we chose to focus on tweets from both datasets that were posted between March 1, 2020 through August 30, 2020. The Democratic National Convention took place from August 17-20, marking the official shift from the primaries to the presidential election. For this study, we utilize release v2.12 from our COVID-19 dataset and release v1.3 from our U.S. presidential elections dataset. We tracked several related keywords and accounts for each dataset’s respective topic, a sampling of which can be found in Table 1.
COVID-19-keyword | Tracked since | U.S. presidential elections keyword | Tracked since |
coronavirus | 1/21/2020 | @JoeBiden | 5/20/2019 |
CDC | 1/21/2020 | @CoryBooker | 5/20/2019 |
ncov | 1/21/2020 | @PeteButtigieg | 5/20/2019 |
covid-19 | 2/16/2020 | @JulianCastro | 5/20/2019 |
corona virus | 3/2/2020 | @BilldeBlasio | 5/20/2019 |
covid | 3/6/2020 | @JohnDelaney | 5/20/2019 |
sars-cov-2 | 3/6/2020 | @TulsiGabbard | 5/20/2019 |
socialdistancing | 3/13/2020 | @gillbrandny | 5/20/2019 |
lockdown | 3/16/2020 | @KamalaHarris | 5/20/2019 |
wear a mask | 6/28/2020 | @Hickenlooper | 5/20/2019 |
wearamask | 6/28/2020 | @JayInslee | 5/20/2019 |
We then filtered the general COVID-19 dataset for tweets related to the Democratic Primary using keywords of interest (Table 2). As we are interested in the U.S. Democratic primaries, we utilize user-specified locations included in each tweet’s metadata and normalized these locations (Jiang et al., 2020). We require all tweets to contain normalized location data that originates from the United States with an identifiable state attribution and be tagged as an English tweet by Twitter. We used Latent Dirichlet allocation (LDA) to cluster the tweets into 8 topics (this was selected based on the number of topics with the highest coherence score) and tagged tweets based on their nearest probable topic (Blei et al., 2003). We describe how we construct the final dataset in the Appendix (see Appendix Part A, “Constructing the dataset”).
Primary-related keywords | |
vote | primary |
democrat | bennet |
biden | bloomberg |
booker | bullock |
buttigieg | castro |
blasio | delaney |
gabbard | gravel |
gillibrand | harris |
hickenlooper | inslee |
klobuchar | messam |
moulton | ojeda |
rourke | patrick |
ryan | sanders |
sestak | steyer |
swalwell | warren |
williamson | yang |
mailin | mail in |
mail-in | ballot |
Narrative and community detection
We then focused on two narratives: mask-wearing and voting by mail, using tweets that contain mask or mail-in ballot-related keywords, as listed in Table 3. We remove quoted tweets, as we are interested in original content and the amplification of certain viewpoints, and quoted tweets (retweets with comments) may contain contrarian commentary relative to the retweeted tweet. This results in 5,211,071 vote-by-mail tweets and 1,014,751 mask tweets. With this dataset, we found relevant co-occurring hashtags from these tweets (see table A1 in Part B of the Appendix). Using these hashtags, we extracted tweets from the entire collection of primary-related tweets containing any of these hashtags. We also leverage specific hashtags that are indicative of stance to identify if a user has engaged in mask-wearing and voting by mail factual information or misinformation. Please refer to the Appendix Part B, “Tagging public health misinformation” for a more detailed discussion on how we determined hashtag ideology alignment and its surrounding discourse. To infer a user’s political affiliation, we matched user-shared URLs with domains from Media Bias-Fact Check to five categories: left, lean left, center, lean right, and right (Zandt, n.d.). For better accuracy, we only included users with more than 10 politically leaning URLs in our visualization. We find the majority URL political affiliation and tag the users as such; in the case of ties, one of the political classifications was chosen at random uniformly.
COVID-19-related keywords | Mail-in ballot-related keywords | Lockdown-related keywords | Mask-related keywords |
covid | ballot | lockdown | mask |
corona | mail-in | stayathome | face cover |
covd | mailin | stay at home | facecover |
sars-cov-2 | mail in | lock down | |
pandemic | ballot | stay-at-home | |
usps | social distanc |
Finally, we merge these two tags for each tweet based on the posting user and cluster the users into one of four categories describing a user’s political affiliation and their tendency to spread misinformation or factual information: 1) Democratic and fact, 2) Republican and fact, 3) Democratic and misinformation and 4) Republican and misinformation. This results in 1,253,022 unique users. The domains and the aggregate bias of the data are shown in Table 4 and Figure 4. The most frequent political affiliation of domains shared is from sources that are center left (or lean left), which is consistent with the labels the Pew Center assigns to the most reputable media outlets (Jurkowski et al, 2020). However, the most frequently retweeted individual domains include right-leaning media sources, Fox News, Dallas Morning News, and the Daily Caller. This suggests that conservative tweeters tend to have a more concentrated media diet.
Domain | Frequency |
cnn | 10,922 |
dallasnews | 9,583 |
washingtonpost | 9,364 |
dailycaller | 5,943 |
foxnews | 4,580 |
nypost | 4,507 |
npr | 3,852 |
trib | 3,833 |
nbcnews | 3,492 |
rawstory | 2,959 |
nytimes | 2,790 |
apnews | 2,292 |
msn | 2,125 |
thehill | 2,036 |
yahoo | 1,937 |
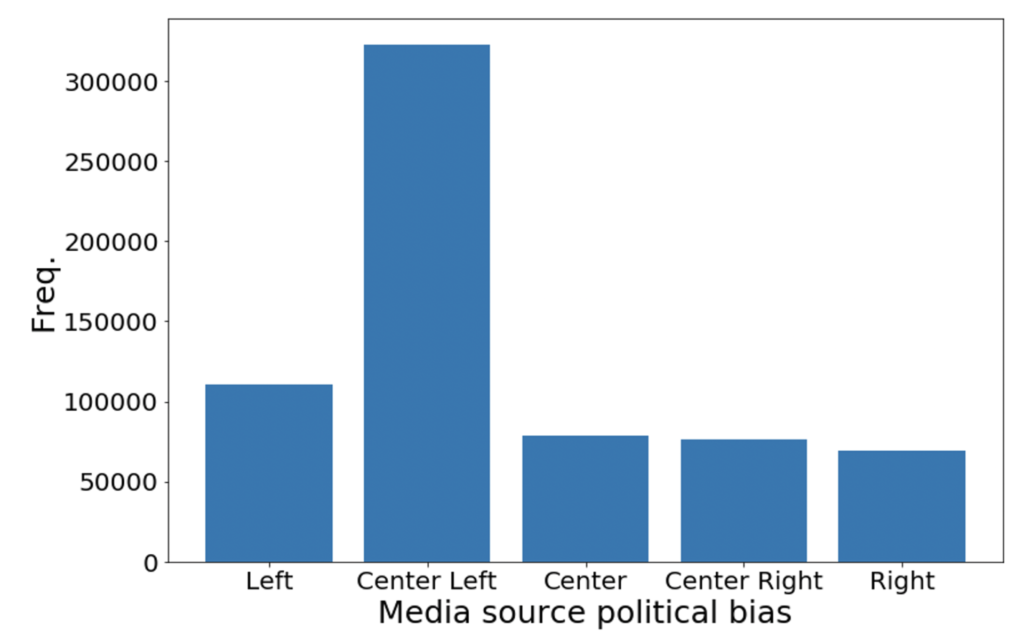
Given that there are more than 67 million tweets, visualizing user behavior in a meaningful way is a high-dimensional challenge. A network of social interaction was between Twitter users, where nodes are users and edges are the number of retweets. This is a directed graph, for which the original tweeter is the head. There were 1,028,742 unique users and 2,886,004 unique weighted edges.
From there, we applied node2vec, which represents the network in Euclidean space (Grover and Leskovec, 2008). The algorithm conducts random walks to explore “neighborhoods,” such that in the final representation nodes are preserved near their neighbors. We set the dimensions to 10 and the random walk length to 100—these were found through experimentation of visualization parameters. Next, we extract the two most prominent bases using the t-SNE algorithm (t-distributed stochastic neighbor embedding), which maps high dimensional data to lower dimensions by constructing Student t-distributions over the dataset. We set the dimensionality to two, as we want to visualize our networks in two dimensions. A discussion of the study limitations can be found in Part C of the Appendix.
Topics
Bibliography
As U.S. Coronavirus cases hit 3.5 million, officials scramble to add restrictions. (2020, July 15). The New York Times. https://www.nytimes.com/2020/07/15/world/coronavirus-updates.html
Badawy, A., Lerman, K., & Ferrara, E. (2019, May). Who falls for online political manipulation? Companion Proceedings of the 2019 World Wide Web Conference (pp. 162-168). https://doi.org/10.1145/3308560.3316494
Bessi, A. & Ferrara, E. (2016). Social bots distort the 2016 us presidential election online discussion. First Monday, 21(11-7). http://dx.doi.org/10.5210/fm.v21i11.7090
Blei, D. M., Ng, A. Y., & Jordan, M. I. (2003). Latent Dirichlet allocation. The Journal of Machine Learning Research, 3, 993-1022. https://jmlr.org/papers/volume3/blei03a/blei03a.pdf
Bogage, J., Rein, L., & Dawsey, J. (2020, August 20). Postmaster general eyes aggressive changes at Postal Service after election. The Washington Post. https://www.washingtonpost.com/business/2020/08/20/us-postal-service-louis-dejoy/
Bull-Otterson, L., Gray, E. B., Budnitz, D. S., Strosnider, H. M., Schieber, L. Z., Courtney, J., García, M. C., Brooks, J. T., Mac Kenzie, W. R., & Gundlapalli, A. V. (2020). Hydroxychloroquine and chloroquine prescribing patterns by provider specialty following initial reports of potential benefit for COVID-19 treatment – United States, January-June 2020. MMWR. Morbidity and Mortality Weekly Report, 69(35), 1210-1215. http://dx.doi.org/10.15585/mmwr.mm6935a4
Carpini, M., & Keeter, S. (1996). What Americans know about politics and why it matters. Yale University Press. http://www.jstor.org/stable/j.ctt1cc2kv1
Chen, E., Deb, A., & Ferrara, E. (2020a). #Election2020: The first public Twitter dataset on the 2020 US presidential election. ArXiv:2020.00600[cs.SI]. https://arxiv.org/abs/2010.00600
Chen, E., Lerman, K. & Ferrara, E. (2020b). Tracking social media discourse about the COVID-19 pandemic: Development of a public coronavirus Twitter data set. JMIR Public Health Surveillance, 6(2), e19273. https://doi.org/10.2196/19273
Deb, A., Luceri, L., Badaway, A. & Ferrara, E. (2019). Perils and challenges of social media and election manipulation analysis: The 2018 US midterms. Companion Proceedings of the 2019 World Wide Web Conference (pp. 237-247). https://doi.org/10.1145/3308560.3316486
DiResta, R., Shaffer, K., Ruppel, B., Sullivan, D., Matney, R., Fox, R., Albright, J. & Johnson, B. (2019). The tactics & tropes of the Internet Research Agency. https://digitalcommons.unl.edu/senatedocs/2/
Dutt, R., Deb, A., & Ferrara, E. (2018, December). “Senator, we sell ads”: Analysis of the 2016 Russian Facebook Ads Campaign. In Akoglu L., Ferrara E., Deivamani M., Baeza-Yates R., & Yogesh P. (Eds.), International Conference on Intelligent Information Technologies (pp. 151-168). Springer. https://doi.org/10.1007/978-981-13-3582-2_12
Edwards, E. (2020, June 17). World Health Organization halts hydroxychloroquine study. NBC News. https://www.nbcnews.com/health/health-news/world-health-organization-halts-hydroxychloroquine-study-n1231348
Farley, R. (2020, April 10). Trump’s latest voter fraud misinformation. FactCheck.org. https://www.factcheck.org/2020/04/trumps-latest-voter-fraud-misinformation/
Ferrara, E. (2017). Disinformation and social bot operations in the run up to the 2017 French presidential election. First Monday, 22(8). https://doi.org/10.5210/fm.v22i8.8005
Ferrara, E. (2020). What types of COVID-19 conspiracies are populated by Twitter bots? First Monday, 25(6). https://doi.org/10.5210/fm.v25i6.10633
Flynn, D. J., Nyhan, B., & Reifler, J. (2017). The nature and origins of misperceptions: Understanding false and unsupported beliefs about politics. Political Psychology, 38, 127-150. https://doi.org/10.1111/pops.12394
Grover, A., & Leskovec, J. (2016, August). node2vec: Scalable feature learning for networks. Proceedings of the 22nd ACM SIGKDD International Conference on Knowledge Discovery and Data Mining (pp. 855-864). https://doi.org/10.1145/2939672.2939754
Guess, A., Nyhan, B., & Reifler, J. (2018). Selective exposure to misinformation: Evidence from the consumption of fake news during the 2016 U.S. presidential campaign. European Research Council, 9(3), 4.
Jiang, J., Chen, E., Yan, S., Lerman, K., & Ferrara, E. (2020). Political polarization drives online conversations about COVID-19 in the United States. Human Behavior and Emerging Technologies, 2(3), 200-211. https://doi.org/10.1002/hbe2.202
Jost, J. T., van der Linden, S., Panagopoulos, C., & Hardin, C. D. (2018). Ideological asymmetries in conformity, desire for shared reality, and the spread of misinformation. Current Opinion in Psychology, 23, 77-83. https://doi.org/10.1016/j.copsyc.2018.01.003
Jurkowitz, M., Mitchell, A., Shearer, E., & Walker, M. (2020). U.S. media polarization and the 2020 election: A nation divided. Pew Research Center: Journalism and Media. https://www.journalism.org/2020/01/24/u-s-media-polarization-and-the-2020-election-a-nation-divided/
Katz, E. & Lazarsfeld, P. F. (1966). Personal influence, the part played by people in the flow of mass communications. Transaction Publishers.
Kessler, A. S. & Cornwall, T. (2013). Does misinformation demobilize the electorate? Measuring the impact of alleged robocalls in the 2011 Canadian election. Centre for Economic Policy Research. https://cepr.org/active/publications/discussion_papers/dp.php?dpno=8945
Kim, Y. M., Hsu, J., Neiman, D., Kou, C., Bankston, L., Kim, S. Y., Heinrich, R., Baragwanath, R. & Raskutti, G. (2018). The stealth media? Groups and targets behind divisive issue campaigns on Facebook. Political Communication, 35(4), 515-541. https://doi.org/10.1080/10584609.2018.1476425
Maaten, L. V. D., & Hinton, G. (2008). Visualizing data using t-SNE. Journal of Machine Learning Research, 9(86), 2579-2605. https://jmlr.org/papers/volume9/vandermaaten08a/vandermaaten08a.pdf
Nyhan, B., & Reifler, J. (2010). When corrections fail: The persistence of political misperceptions. Political Behavior, 32(2), 303-330. https://doi.org/10.1007/s11109-010-9112-2
Oprysko, C. (2020, May 18). Trump says he’s taking hydroxychloroquine, despite scientists’ concerns. Politico. https://www.politico.com/news/2020/05/18/trump-says-hes-taking-unproven-anti-malarial-drug-265546
Qiu, L. (2021, January 5). Fact-checking falsehoods on mail-in voting. The New York Times. https://www.nytimes.com/article/fact-checking-mail-in-voting.html
Sherman, A. (2020, April 8). Trump said the Obama admin left him a bare stockpile. Wrong. Politifact. https://www.politifact.com/factchecks/2020/apr/08/donald-trump/trump-said-obama-admin-left-him-bare-stockpile-wro/
Spencer, S. H. (2020, December 11). Nine election fraud claims, none credible. FactCheck.org. https://www.factcheck.org/2020/12/nine-election-fraud-claims-none-credible/
World Health Organization. (2020, July 4). WHO discontinues hydroxychloroquine and lopinavir/ritonavir treatment arms for COVID-19. https://www.who.int/news/item/04-07-2020-who-discontinues-hydroxychloroquine-and-lopinavir-ritonavir-treatment-arms-for-covid-19
White, K. M., Binder, M., Ledet, R. & Hofstetter, C. R. (2006). Information, misinformation, and political participation. American Review of Politics, 27, 71-90. https://doi.org/10.15763/issn.2374-7781.2006.27.0.71-90
Wilson, C. (2020, October 25). The U.S. just set a new daily record for COVID-19 cases. Time. https://time.com/5903673/record-daily-coronavirus-cases/
Zandt, D. V. (n.d.) Media bias / Fact check – Search and learn the bias of news media. Media Bias Fact Check. https://mediabiasfactcheck.com/
Funding
The authors gratefully acknowledge support from the Defense Advanced Research Projects Agency (DARPA), contract #W911NF-17-C-0094 and the Air Force Office of Scientific Research Grant, grant #FA9550-17-1-0327. H.C. and E.F. are grateful to the Annenberg Foundation for their support.
Competing Interests
The authors declare no competing interests.
Ethics
This study leverages publicly available data and is registered as IRB exempt by the University of Southern California IRB (approved protocol UP-17-00610).
Copyright
This is an open access article distributed under the terms of the Creative Commons Attribution License, which permits unrestricted use, distribution, and reproduction in any medium, provided that the original author and source are properly credited.
Data Availability
The specific COVID-19 dataset version used in this study has been made publicly available via the Harvard Database: https://dataverse.harvard.edu/dataset.xhtml?persistentId=doi:10.7910/DVN/EBQ0E4. The maintained dataset (https://github.com/echen102/COVID-19-TweetIDs) is presented in Chen et al., 2020.
The specific US Presidential Elections dataset version used in this study has been made publicly available via the Harvard Dataverse: https://dataverse.harvard.edu/dataset.xhtml?persistentId=doi:10.7910/DVN/QYSSVA. The maintained dataset (https://github.com/echen102/us-pres-elections-2020) is presented in Chen et al., 2020.