Peer Reviewed
Attitudes towards COVID-19 vaccines may have “spilled over” to other, unrelated vaccines along party lines in the United States
Article Metrics
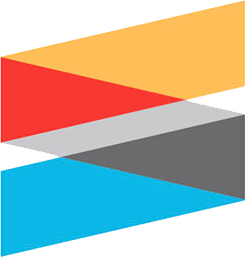
0
CrossRef Citations
Altmetric Score
PDF Downloads
Page Views
This study used data from pre- and post-COVID surveys to examine vaccine attitudes in the United States. We found evidence consistent with an ideological “spillover” effect: Liberals’ attitudes became more positive towards non-COVID vaccines (flu, MMR, HPV, chickenpox) and conservatives’ attitudes became more negative. These spillover effects are perplexing because the COVID-19 vaccines were developed more rapidly than the others and (some of them) were the first to use mRNA technology on a mass scale to achieve immunization. Hence, there were reasons to isolate one’s attitudes towards the COVID-19 vaccines rather than generalize them. This exacerbates current vaccine communication challenges.
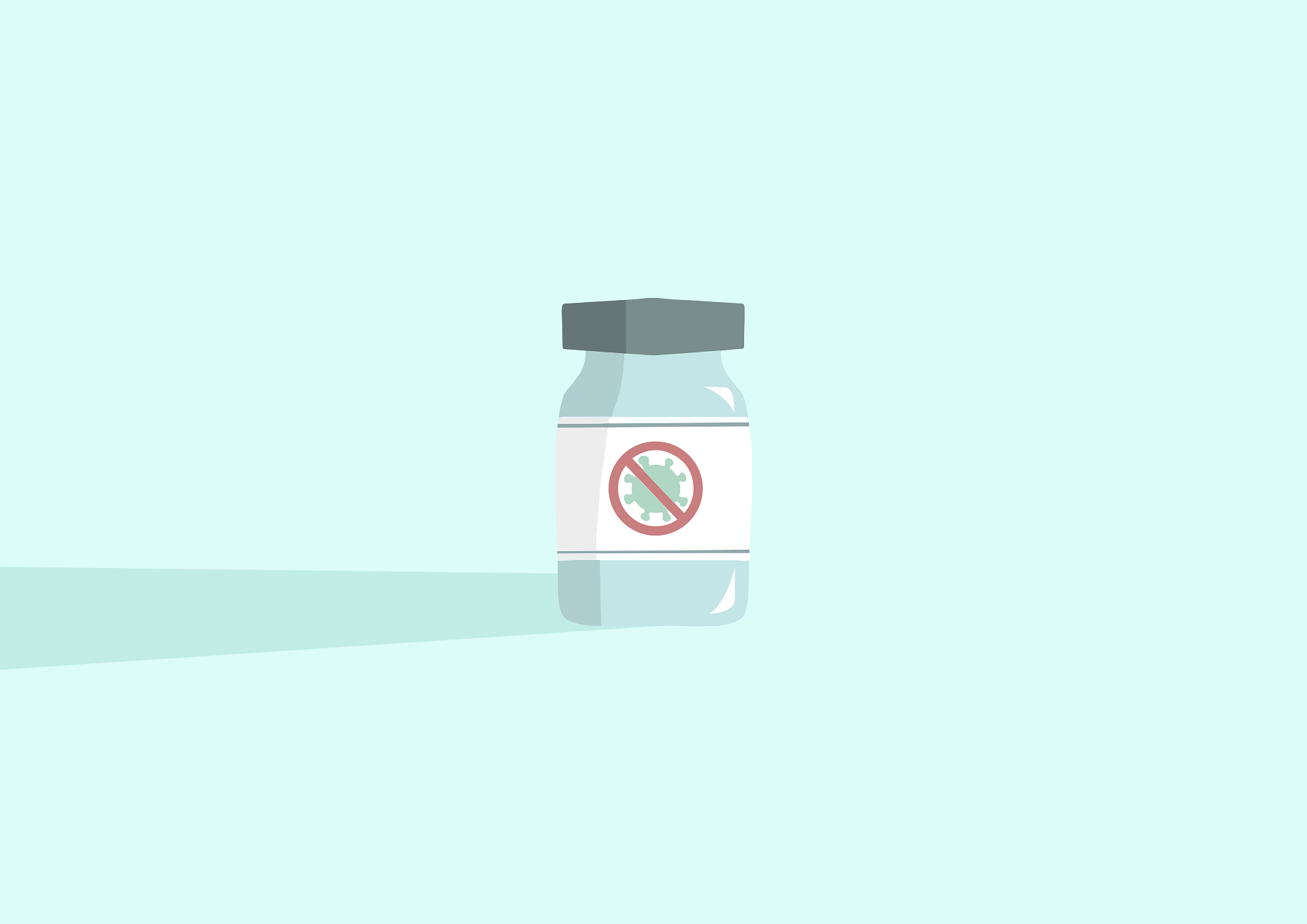
Research Question
- Did liberals and conservatives in the United States generalize their attitudes toward the COVID-19 vaccines to other, unrelated vaccines?
Essay Summary
- We compared political conservatives in the United States to liberals, measuring the degree to which their attitudes towards the COVID-19 vaccines extend to other, unrelated vaccines.
- Conservatives had far more negative attitudes towards the COVID-19 vaccines compared to liberals, but also had more negative attitudes towards the influenza, MMR, HPV, and chickenpox vaccines.
- We used an unsupervised machine learning algorithm to classify participants in a pre-pandemic survey into clusters of “likely liberals” and “likely conservatives.”
- This led to tentative results suggesting that conservatives in the United States spread their negative attitudes towards the COVID-19 vaccines to unrelated vaccines (flu, HPV, MMR, chickenpox).
- Liberals in the United States appeared to exhibit the opposite trend, generalizing their positive attitudes towards the COVID-19 vaccines to unrelated vaccines (flu, HPV, MMR, chickenpox).
Implications
Vaccines must meet rigorous safety standards before being deployed and save approximately 3.5 to 5 million lives per year (World Health Organization, 2019). From the beginning, however, vaccines have faced waves of negative reactions from the public (Allen, 2007). These attitudes manifest in several ways: vaccine refusal, hesitancy, and susceptibility to misinformation. For the sake of brevity, we refer to all manifestations as “negative vaccine attitudes” (NVAs).
The COVID-19 pandemic created strong, intricate changes in NVAs worldwide. On the one hand, several countries showed overall increases in NVAs (de Figueiredo et al., 2023; UNIFCEF, 2023). These increases were not uniform across cultures but resulted from complex interactions between political ideology (e.g., in France, Wang et al., 2020), science literacy (e.g., in Britain, Siani et al., 2022), and the salience of COVID-19’s possible zoonotic origins (e.g., in the United States, LaCour et al., 2022), to name just a few examples. On the other hand, a considerable number of people worldwide eagerly anticipated the opportunity to receive a COVID-19 vaccine once it was available (Lazarus et al., 2021; Lazarus et al., 2022).
There is some evidence indicating that favorable attitudes towards COVID-19 vaccines also extended (or “spilled over” to) influenza vaccines (Domnich et al., 2021; Gallant et al., 2021; Goldman et al., 2021; Siani & Tranter, 2022) though not to childhood vaccines (Soveri et al., 2023). “Spillover” effects like these can be fortunate when they promote more widespread immunization. In the present study, we found evidence that liberals in the United States hold less negative attitudes not only towards the COVID-19 vaccines compared to conservatives but also towards unrelated vaccines (influenza, MMR, HPV, chickenpox).
On the other hand, “negative spillover” effects can still occur. The United States, for instance, experienced one of the most negative reactions to the COVID-19 vaccines, more negative than the global average, trailing only behind South Africa, Russia, Poland, Germany, and France (Lazarus et al., 2022). In the present study, we found that conservatives in the United States hold more negative attitudes not only towards the COVID-19 vaccines compared to liberals but also more negative attitudes towards unrelated vaccines.
We were not able to definitively establish a change in vaccine attitudes over time because we did not ask participants in the pre-pandemic survey about their political affiliation. However, we were able to use their demographic and geographical information to algorithmically categorize them as “likely liberals” or “likely conservatives.” While this methodology should be interpreted with caution (see below), the categories that emerged aligned with well-established demographic correlates of political ideology in the United States (Pew Research Center, 2023). The results were also consistent with a previous study (Fridman et al., 2021), which found that conservatives’ attitudes towards vaccines became more negative throughout the course of the pandemic. However, whereas Fridman et al. (2021) found no evidence that liberals changed their attitudes over time towards non-COVID vaccines, the current study did.
Negative spillover effects are unfortunate because people theoretically had reasons to isolate their attitudes towards the COVID-19 vaccines from other vaccines rather than generalize them. For one, the COVID-19 vaccines were developed more quickly than the others, which is one of the sources of COVID-19 NVAs in the general population (Kreps et al., 2021). Some COVID-19 vaccines were also the first to use mRNA to create immunity on a large scale (Anand & Stahel, 2021). The mRNA components in these vaccines are entirely safe and are quickly destroyed within a few days of injection. However, some members of the public mistakenly believe that mRNA-based vaccines can alter their DNA and many organizations (e.g., Centers for Disease Control and Prevention [2023]) have had to debunk this misconception.
Negative spillover effects are particularly regrettable because each vaccine already presented its own unique set of difficulties in terms of boosting vaccination rates. For example, some people harbor false beliefs that the MMR vaccines cause autism (Leask et al., 2012). By contrast, the HPV vaccine can prompt uncomfortable conversations about sexually transmitted diseases for children ages 11 to 12 years old (Daley et al., 2010). The chickenpox vaccine might seem unnecessary to many parents because they grew up during a period when children were intentionally infected with chickenpox, sometimes at “chickenpox parties” (Parad, 2012). Parents might, therefore, under-appreciate warnings from experts about the risks of chickenpox.
Post-pandemic vaccine advocacy
Since the COVID-19 pandemic, there have been two shifts in vaccine hesitancy that we think are particularly worth highlighting: a change in scale and a change in demography. First, there was a dramatic increase in vaccine refusal in the United States during and after the COVID-19 pandemic (Bolsen & Palm, 2022; Hart et al., 2020; Monroe & Savillo, 2021; Wood & Brumfiel, 2021). In fact, before the pandemic, inequalities in healthcare access likely overshadowed NVAs as a source of under-vaccination (Leask et al., 2012; Monais, 2019; Fuchs & Dicara, 2019).
The second major shift concerns demography. Before the pandemic, no single NVA group in the United States constituted a clear demographic majority. In many respects, NVA groups aligned demographically with liberals (Tomeny et al., 2017; Lubrano, 2019). Others had religious or philosophical motivations behind their NVAs, largely unrelated to politics (“A jab in time,” 2016). Before the pandemic, conservatives were just as likely to receive a flu vaccine as liberals (Enten, 2021). After the pandemic, conservatives appear to make up a majority of NVA groups in the United States (Wood & Brumfiel, 2021). This shift is likely due to political polarization surrounding the COVID-19 pandemic in the United States. Then-President Trump downplayed the severity of the virus (Oprysko, 2020) and conservative media outlets were overwhelmingly negative towards the COVID-19 vaccines, questioning their safety and necessity (Monroe & Savillo, 2021). Politically red states ended up having the lowest COVID-19 vaccination rates and the highest COVID-19 death tolls (Wood & Brumfiel, 2021). If there has indeed been a negative spillover effect among U.S. conservatives, this suggests that the previous obstacles related to specific vaccines are now amplified by partisanship and political identity.
Leveraging misinformation research
The primary findings of this study are consistent with a spillover effect, where people generalized their attitudes (good or bad) towards the COVID-19 vaccines to unrelated vaccines. There are a number of potential cognitive explanations for this. For instance, it might have occurred because of an inductive inference (Davis et al., 2017; Tapp et al., 2018; Davis et al., 2020). Some people may have thought, “If most vaccines are safe and effective, then the COVID-19 vaccine will be too.” By contrast, others might have inferred something along the lines of, “Since the COVID-19 vaccines are unsafe and ineffective, other vaccines must be too.”
A similar explanation is that people remember the “gist” of COVID-19 information (or misinformation) after forgetting the specific (verbatim) information (fuzzy-trace theory;1According to fuzzy-trace theory the mind has multiple systems for representing information, one that is “verbatim” (preserving exact details) and one that is “gist” (preserving overall meaning or summaries). The theory holds that people tend to rely on “gist” representations when performing a variety of cognitive tasks (e.g., problem solving, decision-making).Reyna et al., 2021). In other words, people with NVAs might have heard several specific statements about vaccines, but the details of these statements might have faded over time. All that is left over is the “gist” of this information: “Vaccines are bad” (or “good,” depending on one’s media diet). For someone with NVAs, then, no specific statement justifies their attitudes. Rather, the “gist” of many statements has left a generally negative impression. Thus, debunking specific claims in order to vindicate vaccines might fail to compensate for the influence left over from a larger number of negative, if poorly remembered, statements that seem to discredit said vaccines.
Negative spillover effects could also be explained by right-leaning media outlets repeating false or misleading information about the COVID-19 vaccines (Monroe & Savillo, 2021). The “illusory truth effect” occurs when false statements appear more plausible merely because they have been repeated. This is often attributed to subjective feelings of familiarity and ease of processing (cognitive fluency) caused by the repetitions (Brashier & Marsh, 2020). Thus, it is important to counter misinformation without repeating the original falsehoods.
Researchers find it is best to focus on explanatory details when debunking false statements (Swire et al., 2017). People have mental models that help them make sense of the world. Communicators cannot usually remove a piece of explanatory information from these models without leaving an explanatory “gap.” Thus, it is important to supply correct information that explains the same phenomena the misinformation purported to explain (Ecker, 2017). Saying “mRNA vaccines cannot alter your DNA” debunks a statement but also repeats the statement. This can inadvertently make the statement more fluent. Communicators should use messages that do not repeat false statements but instead provide correct alternative information rich with explanatory details: “mRNA vaccines provide instructions directly to your body to produce a harmless piece of protein that is found on the surface of the virus that causes COVID-19. These instructions do not interact directly with your DNA, and once they are used, they are quickly eliminated by a natural process of degradation.”
While the current findings might lead to pessimism for some, there are also grounds for optimism. After all, only a relatively small minority of conservatives completely refused to vaccinate against COVID-19 (Newhagen & Bucy, 2020). The present study also builds on past research, which also harnessed socio-demographic data in order to pinpoint specific demographics and enhance the effectiveness of messages tailored to each group’s unique priorities and motivations (Enders et al., 2020). For instance, dispelling vaccine misinformation specifically for conservative audiences might be more effective if the messaging frames information accuracy as a patriotic duty or other traditional conservative values (Stagnaro et al., 2023).
Findings
Finding 1: After the pandemic, conservatives in the United States held substantially more negative attitudes toward a variety of vaccines compared to liberals.
Before the COVID-19 pandemic (August of 2018), we conducted a survey (n = 165) asking Americans about their attitudes towards the flu, HPV, MMR, and chickenpox vaccines. The objective was to examine which vaccines, relative to each other, were viewed most negatively. After the COVID-19 pandemic, this unpublished dataset was seen as an opportunity to compare attitudes towards a variety of vaccines pre- and post-pandemic. We, therefore, asked identical questions to a new set of participants (n = 243) after the pandemic (April of 2023) but with new questions included about the COVID-19 vaccines.
We begin by focusing on the post-pandemic survey. Figure 1 shows the degree of NVAs for each vaccine. Participants indicated their political leanings with a 7-point scale (1 = very liberal, 2 = liberal, 3 = somewhat liberal, 4 = neither liberal nor conservative, 5 = somewhat conservative, 6 = conservative, 7 = very conservative). In Figure 1, people are separated into liberal (3 or lower) and conservative (5 or higher) groups. Participants who described themselves as “neither liberal nor conservative” are not included in the graphic. Using general linear modeling, we confirmed there was a statistically significant association between partisanship and vaccine attitudes, regardless of whether political leanings are treated as a spectrum or polarized into binaries (for the full modeling details, see Methods section below).
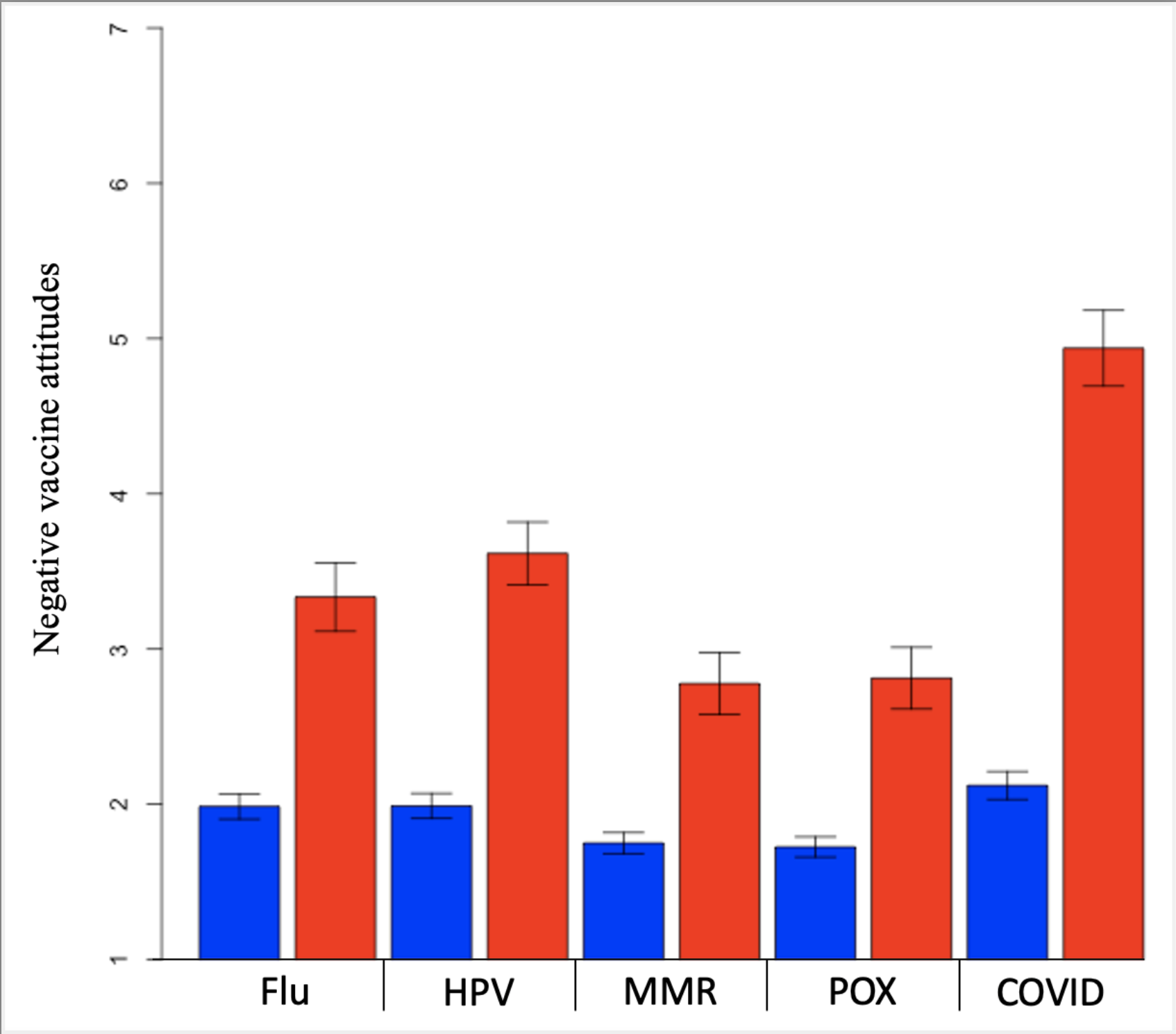
Finding 2: Evidence suggesting partisan spillover effects.
We asked participants in the pre- and post-pandemic surveys to answer identical questions about their attitudes towards the flu, HPV, MMR, and chickenpox vaccines. Because of this, we were able to analyze overall differences in NVAs across the two time points (see Figure 2).
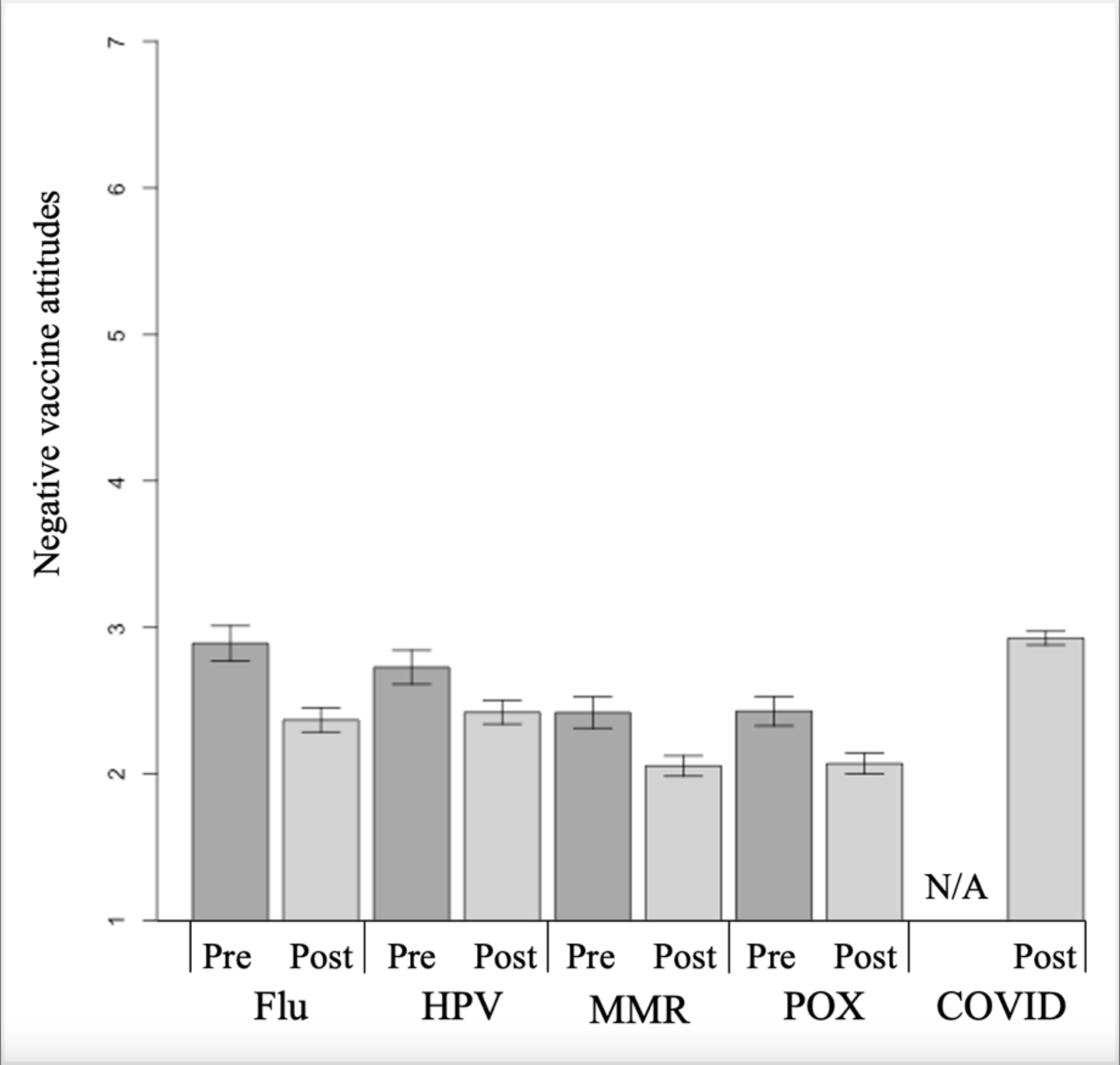
According to our statistical analyses, there was an overall decrease in NVAs over time. Since we did not explicitly ask the pre-COVID survey participants about their political affiliations, we were unable to directly analyze how pre- and post-pandemic NVAs potentially changed depending on participants’ political leanings. However, we did have substantial demographic and geographical information for the pre-COVID survey participants, including the ZIP code in which they grew up. We used participants’ age, gender, race, education level, whether they grew up in a red state, a swing state, the population of the district they grew up in, and whether that district voted mostly for Trump in the 2020 election to algorithmically sort participants into clusters. Notably, we did not use anyone’s vaccine attitudes to create these clusters. Two clusters emerged. One of these was characterized by people who tended to be older, predominantly male, white, have more formal education, come from a red state, a district with a smaller population, and one that voted mostly for Trump in the 2020 election. These cluster characteristics align with well-established demographic correlates of conservatism (Pew Research Center, 2023). This cluster also made up 25.66% of the overall sample, which was statistically indistinguishable from the 22.92% of participants in the second study who self-identified as conservative, χ2(1) = 0.21, p = .643. We, therefore, referred to participants in these two clusters as “likely liberals” and “likely conservatives.”
Figure 3 shows the average NVA for each vaccine for “likely liberals” (light blue) and “likely conservatives” (light red) in the pre-COVID survey, self-described liberals (dark blue) and self-described conservatives (dark red) in the post-COVID survey. To the extent the clustering algorithm can be relied upon, there appears to have been a positive spillover effect for liberals and a negative spillover effect for conservatives. Statistical analyses confirm that conservatives had higher NVAs in the post-pandemic survey compared to “likely liberals” and “likely conservatives” in the pre-pandemic survey (see Methods section for more details). The apparent negative spillover among conservatives aligns with previous research (Fridman et al., 2021), though the apparent positive spillover among liberals is novel to the present study.
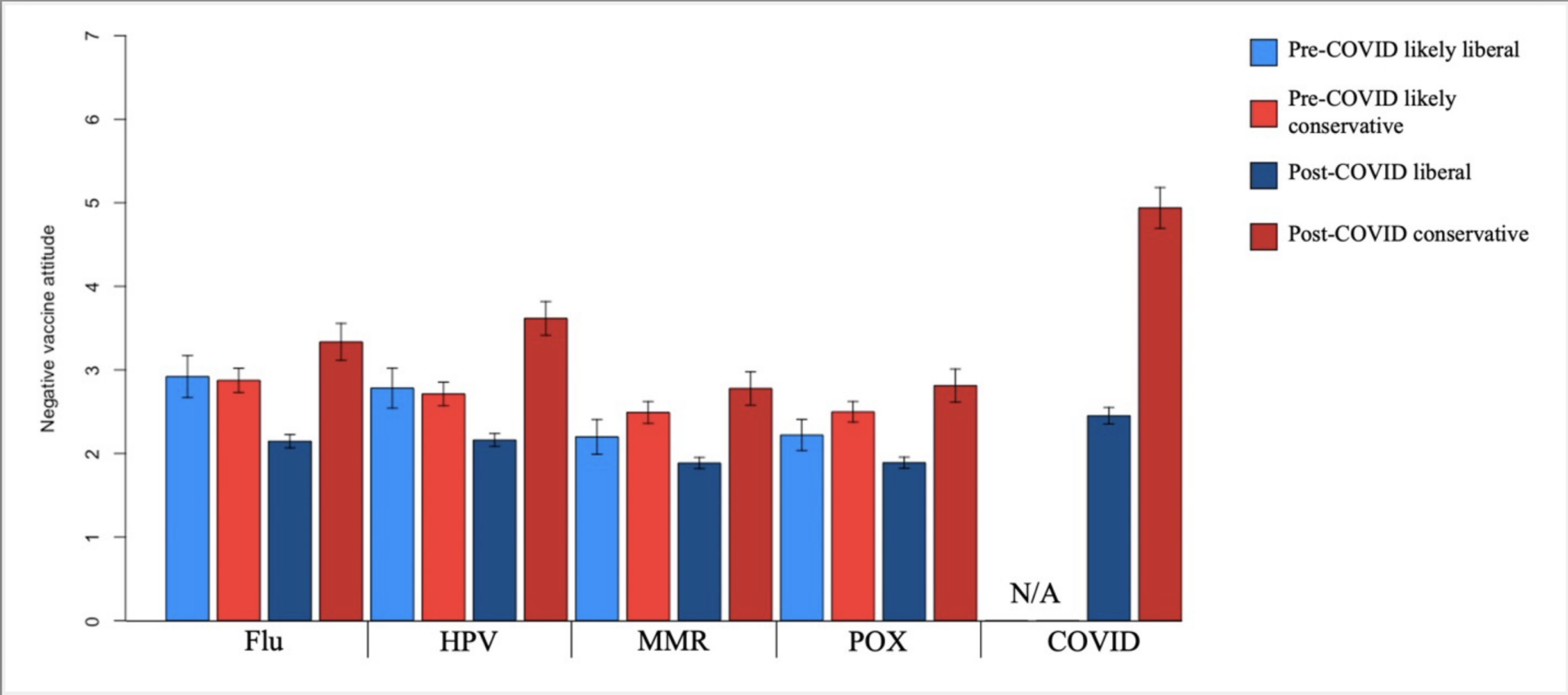
It is worth noting that the clustering algorithm sorted people into binary “likely liberal” and “likely conservative” categories. Thus, moderate voters were split between both clusters. By contrast, the post-pandemic data are split into binary categories (“liberal” or “conservative”) after excluding anyone who self-described as “neither liberal nor conservative.” Some of the ideological differences between timeframes could, therefore, be driven in part by removing moderate voters from the post-pandemic data, which is not possible to replicate in the pre-pandemic data.
Methods
Survey details
The pre-pandemic survey (n = 165) was conducted in August 2018 on Amazon’s Mechanical Turk platform. The post-pandemic survey (n = 243) was conducted in April 2023 on Prolific. Both samples consisted of people currently living in the United States. The demographic section of both surveys was identical, with two exceptions. First, the pre-pandemic survey asked, “In which ZIP code did you spend most of your childhood (if outside of the United States, list the country)?” This question was dropped from the first questionnaire in favor of “Which of the following best describes your political orientation?” The response options were: 1 = very liberal, 2 = liberal, 3 = somewhat liberal, 4 = neither liberal nor conservative, 5 = somewhat conservative, 6 = conservative, 7 = very conservative. Both surveys asked participants their age, sex, ethnicity, and highest level of education attained. Both surveys had sets of vaccine-specific questions. Namely, the statements in Table 1 were repeated with “flu,” “HPV,” “MMR,” “chickenpox,” and (for the post-pandemic survey) “COVID-19” substituted in. Thus, the pre-pandemic survey repeated these seven questions four times, while the post-pandemic survey repeated these seven questions five times. Additional details about the surveys and analyses discussed in the Methods section can be found in the Appendix and the Harvard Dataverse Repository at https://doi.org/10.7910/DVN/JVBA7F.
Question number | Question content |
1 | I worry about the short-term side effects of the __________vaccine. |
2 | I worry that the __________vaccine might have unknown long-term effects. |
3 | I feel uncertain about the potential side-effects of the __________vaccine. |
4 | I believe getting the __________vaccine is (very good, good, somewhat good, neither good nor bad, somewhat bad, bad, very bad). |
5 | Getting the __________vaccine is (very beneficial, beneficial, somewhat beneficial, neither beneficial nor harmful, somewhat harmful, harmful, very harmful). |
6 | Getting the __________vaccine is (very wise, wise, somewhat wise, neither wise nor foolish, somewhat foolish, foolish, very foolish). |
7 | How likely would you be to vaccinate your child with the __________ vaccine (Give a hypothetical answer, if necessary) (1 = very likely, 2 = likely, 3 = somewhat likely, 4 = neither likely nor unlikely, 5 = somewhat unlikely, 6 = unlikely, 7 = very unlikely). |
Participants rated their agreement to each statement on a 7-point Likert scale (1 = strongly disagree, 2 = disagree, 3 = somewhat disagree, 4 = neither agree nor disagree, 5 = somewhat agree, 6 = agree, 7 = strongly agree). NVAs were calculated for each vaccine by averaging the numbers selected for the seven questionnaire items shown in Table 1.
Each vaccine-specific section of the survey included a brief statement from a website (vaccineinformation.org) about the purpose of the vaccine, the symptoms of the disease, and the recommended vaccine schedule. As an example, the statement for chickenpox read, “According to vaccineinformation.org, chickenpox (varicella) is a disease caused by a virus. Most people with chickenpox get very itchy blisters and sores all over their body. All children should get two doses of chickenpox vaccine starting at age 1. Some teens and adults may also need this vaccine if they didn’t get two doses of the vaccine or chickenpox disease when they were younger.”
The original website from which we retrieved the vaccine information for the other section does not have an entry for COVID-19, so we wrote one in a style that is as similar as possible. It reads, “Coronavirus disease 2019 (COVID-19) is a contagious disease caused by a virus, the severe acute respiratory syndrome coronavirus 2 (SARS-CoV-2). The symptoms of COVID‑19 are variable but often include fever, cough, headache, fatigue, breathing difficulties, loss of smell, and loss of taste. CDC recommends everyone stay up to date with COVID-19 vaccines for their age group.” Some additional questions about whether people changed their minds about the COVID-19 vaccines throughout the course of the pandemic were included in the post-pandemic survey.
We conducted separate parallel analyses for each vaccine survey, then followed with an examination of the factor structure implied by each analysis. In every instance, there was an issue with a question about familiarity with the vaccine, “I am familiar with the varicella (chickenpox) vaccine, its benefits, and its risks.” Results indicate this question did not measure the same underlying construct as the remaining ones. After eliminating this question, the remaining items appeared to measure a single underlying construct. Cronbach’s alphas for the pre-pandemic survey were high for each vaccine: chickenpox = 0.93, MMR = 0.93, HPV = 0.94, influenza = 0.94. The post-pandemic survey also had generally high Cronbach’s alphas for each vaccine: chickenpox = 0.93, MMR = 0.93, HPV = 0.94, influenza = 0.94, COVID-19 = 0.96.
Analytic details for Finding 1, where post-pandemic conservatives in the United States held substantially more negative attitudes towards a variety of vaccines compared to liberals
We first examined whether political ideology was associated with NVAs in the post-pandemic survey. Since each participant gave multiple measurements (for each vaccine type), we used a multilevel regression model. We used the lme4 package (Bates et al., 2015) in R (R Core Team, 2016). The dependent variable was negative vaccine attitudes (NVAs). Higher values represent more negative attitudes and lower values represent less negative attitudes. Each specific vaccine type was dummy-coded into the model as a fixed effect. We also included political ideology as a fixed effect along with its interaction with vaccine type. Subjects were included in the model as a random effect. See Table 2 for the model output.
Fixed effect | Coefficient (SE) | p-value | |
COVID-19 (baseline) | 0.79 (.14) | <.001 | * |
Flu | 0.54 (.13) | <.001 | * |
HPV | 0.34 (.13) | 0.008 | * |
MMR | 0.39 (.13) | 0.002 | * |
Chickenpox | 0.32 (.13) | 0.013 | * |
Conservatism | 0.71 (.04) | <.001 | * |
Flu x Conservatism | -0.35 (.04) | <.001 | * |
HPV x Conservatism | -0.26 (.04) | <.001 | * |
MMR x Conservatism | -0.41 (.04) | <.001 | * |
Chickenpox x Conservatism | -0.40 (.04) | <.001 | * |
The baseline category in the model was COVID-19 attitudes for people labeling themselves as “very liberal” (where 1 = “very liberal” and 7 = “very conservative”). Relative to COVID-19, “very liberal” participants had higher NVAs toward the influenza, HPV, MMR, and chickenpox vaccines. For each unit increase in the political spectrum question, NVAs toward the COVID-19 vaccines increased by 0.71 points. Each vaccine type had a statistically significant interaction with political affiliation with a negative sign. This suggests that the relationship between conservatism and NVAs was reduced for non-COVID vaccines. Note, however, that conservatives still had more negative attitudes towards the other vaccines overall, but less so compared to the COVID-19 vaccines. The marginal pseudo R2 value for the model was 0.36.
Political orientation could also be dichotomized into “liberal” (3 or lower) and “conservative” (5 or higher) groups, as we did when visualizing the data in Figures 1–3. When we repeated the above analysis under these conditions, the same trend was observed.
Analytic details for Finding 2, evidence consistent with partisan spillover effects
We sought to determine whether NVAs shifted between time periods. To acknowledge repeated attitude ratings nested within each participant, we used a multi-level regression model. The dependent variable and independent variables were identical to the previous model, except political affiliation was not included and time was. The model coefficients can be seen in Table 3.
Fixed effect | Coefficient (SE) | p-value | |
Influenza (baseline) | 2.94 (.10) | <.001 | * |
HPV | -0.16 (.11) | 0.137 | |
MMR | -0.47 (.11) | <.001 | * |
Chickenpox | -0.46 (.11) | <.001 | * |
Time | -0.55 (.10) | <.001 | * |
HPV x Time | 0.23 (.14) | 0.110 | |
MMR x Time | 0.15 (.14) | 0.308 | |
Chickenpox x Time | 0.14 (.14) | 0.312 |
The baseline category in the model was pre-pandemic influenza vaccines. NVAs were significantly lower for the MMR and chickenpox vaccines compared to influenza but not compared to HPV. The overall effect of time was a predicted decrease of 0.55 points in NVAs. The marginal pseudo R2 value for the model was .05. There was no evidence of any vaccine-by-time interactions. Rather than “affirm the null,” we compared a “null” model with only main effects against the current model, which allows vaccine-specific attitude changes across time points. We used an approximation of a Bayes factor (BF01) popularized by Wagenmakers (2007). Results suggest the “null” (main effects only) model was over 100 times more likely than our initial model. Thus, we concluded that the overall decrease in NVAs over time was fairly uniform across each vaccine type.
One possible issue with this analysis is that there was a different proportion of men and women between the pre- and post-COVID samples (see Appendix). Women in the United States have been drifting politically towards the left (Saad, 2024), which may have affected the results. To assess this possibility, we looked for time-by-gender interactions for each vaccine (except COVID). None of these interactions were statistically significant. We, therefore, found no evidence that gender was associated with differences in NVAs across the two time points.
We used k-means clustering on the demographic and geographical data from the pre-pandemic sample to see whether any latent sub-groups emerged that aligned with their political affiliation. K-means clustering is an algorithm that groups similar data points together based on their distance from each other in an N-dimensional space. Preliminary analyses suggested 2 clusters were likely present in the data. These clusters were based on participants’ age, sex (male or female), ethnicity (white, not-white), education, whether they were from a red state or swing state, the population of the ZIP code in which they grew up, and whether the corresponding district voted mostly for Trump in the 2020 election.
To examine shifts in NVAs across time points and political ideology (cluster-based, for the pre-pandemic sample), we conducted a multilevel regression model. The dependent variable was NVAs. Vaccine type was included as a fixed effect. We also had fixed effects for time, whether someone is a conservative (to any degree) and the interaction between time and conservatism. Participants were included as a random effect. The pseudo R2 for the model was .11. See Table 4 for the coefficient estimates.
Fixed effect | Coefficient (SE) | p-value | |
Influenza (baseline) | 2.86 (.09) | <.001 | * |
HPV | -0.02 (.07) | 0.815 | |
MMR | -0.38 (.07) | <.001 | * |
Chickenpox | -0.38 (.07) | <.001 | * |
Time (post-pandemic) | -0.64 (.07) | <.001 | * |
Conservative | -0.19 (.12) | 0.117 | |
Time x Conservative | 1.32 (.16) | <.001 | * |
There was a significant decrease in NVAs for the baseline group (liberals) between the pre- and post-pandemic surveys, as indicated by the significant coefficient for time. However, there was also significant (and large) time-by-conservative interaction, suggesting conservatives’ NVAs were much higher during the post-pandemic survey compared to liberals’ NVAs, even after adjusting for liberals’ attitude shifts over time and for vaccine type.
Bibliography
A jab in time. (2016, March 26). The Economist. https://www.economist.com/international/2016/03/26/a-jab-in-time
Allen, A. (2007). Vaccine: The controversial story of medicine’s greatest lifesaver. Norton. https://doi.org/10.1177/014107680710000515
Anand, P., & Stahel, V. P. (2021). The safety of Covid-19 mRNA vaccines: A review. Patient Safety in Surgery, 15(1). https://doi.org/10.1186/s13037-021-00291-9
Bates D., Mächler M., Bolker B., & Walker S. (2015). Fitting linear mixed-effects models using lme4. Journal of Statistical Software, 67(1), 1–48. https://doi.org/10.18637/jss.v067.i01
Bolsen, T., & Palm, R. (2022). Politicization and COVID-19 vaccine resistance in the U.S. Progress in Molecular Biology and Translational Science, 188(1), 81–100. https://doi.org/10.1016/bs.pmbts.2021.10.002
Brashier, N. M., & Marsh, E. J. (2020). Judging truth. Annual Review of Psychology, 71, 499–515. https://doi.org/10.1146/annurev-psych-010419-050807
Centers for Disease Control and Prevention. (2023). COVID-19: Myths & facts. https://www.cdc.gov/coronavirus/2019-ncov/vaccines/facts.html
Daley, M. F., Crane, L. A., Markowitz, L. E., Black, S. R., Beaty, B. L., Barrow, J., Babbel, C., Gottlieb, S. L., Liddon, N., Stokley, S., Dickingson, M., & Kempe, A. (2010). Human papillomavirus vaccination practices: A survey of US physicians 18 months after licensure. Pediatrics, 126(3), 425–433. https://doi.org/10.1542/peds.2009-3500
Davis, T., Goldwater, M. B., Ireland, M. E., Gaylord, N., & Van Allen, J. (2017). Can you catch Ebola from a stork bite? Inductive reasoning influences generalization of perceived zoonosis risk. PLOS ONE, 12(11), e0186969. https://doi.org/10.1371/journal.pone.0186969
Davis, T., LaCour, M., Goldwater, M., Hughes, B., Ireland, M. E., Worthy, D. A., Gaylord, N., & Van Allen, J. (2020). Communicating about diseases that originate in animals: Lessons from the psychology of inductive reasoning. Behavioral Science & Policy, 6(2), 1–11. https://doi.org/10.1353/bsp.2020.0010
de Figueiredo, A., Temfack, E., Tajudeen, R., & Larson, H. J. (2023). Declining trends in vaccine confidence across sub-Saharan Africa: A large-scale cross-sectional modeling study. Human Vaccines & Immunotherapeutics, 19(1). https://doi.org/10.1080/21645515.2023.2213117
Domnich, A., Grassi, R., Fallani, E., Spurio, A., Bruzzone, B., Panatto, D., Marozzi, B., Cambiaggi, M., Vasco, A., Orsi, A., & Icardi, G. (2021). Changes in attitudes and beliefs concerning vaccination and influenza vaccines between the first and second COVID-19 pandemic waves: A longitudinal study. Vaccines, 9(9), 1016. https://doi.org/10.3390/vaccines9091016
Ecker, U. K. (2017). Why rebuttals may not work: The psychology of misinformation. Media Asia, 44(2), 79–87. https://doi.org/10.1080/01296612.2017.1384145
Enders, A. M., Uscinski, J. E., Klofstad, C., & Stoler, J. (2020). The different forms of COVID-19 misinformation and their consequences. Harvard Kennedy School (HKS) Misinformation Review, 1(8). https://doi.org/10.37016/mr-2020-48
Enten, H. (2021, November 14). Flu shots uptake is now partisan. It didn’t use to be. CNN. https://www.cnn.com/2021/11/14/politics/flu-partisan-divide-analysis/index.html
Fridman, A., Gershon, R., & Gneezy, A. (2021). COVID-19 and vaccine hesitancy: A longitudinal study. PLOS ONE, 16(4), e0250123. https://doi.org/10.1371/journal.pone.0250123
Fuchs, N., & Dicara, L. S. (2019, September 21). Anti-vaxxers aren’t the main problem: Most unvaccinated children in Mass. simply fall through the cracks. CommonWealth Beacon. https://commonwealthmagazine.org/health-care/anti-vaxxers-arent-the-main-problem/
Gallant, A. J., Nicholls, L. A. B., Rasmussen, S., Cogan, N., Young, D., & Williams, L. (2021). Changes in attitudes to vaccination as a result of the COVID-19 pandemic: A longitudinal study of older adults in the UK. PLOS ONE, 16(12), e0261844. https://doi.org/10.1371/journal.pone.0261844
Goldman, R. D., McGregor, S., Marneni, S. R., Katsuta, T., Griffiths, M. A., Hall, J. E., Seiler, M., Klein, E. J., Cotanda, C. P., Gelernter, R., Hoeffe, J., Davis, A. L., Gualco, G., Mater, A., Manzano, S., Thompson, G. C., Ahmed, S., Ali, S., Brown, J. C., … Yue, E. L. (2021). Willingness to vaccinate children against influenza after the coronavirus disease 2019 pandemic. The Journal of Pediatrics, 228, 87–93. https://doi.org/10.1016/j.jpeds.2020.08.005
Hart, P. S., Chinn, S., & Soroka, S. (2020). Politicization and polarization in COVID-19 news coverage. Science Communication, 42(5), 679–697. https://doi.org/10.1177/1075547020950735
Hill, H. A., Elam-Evans, L. D., Yankey, D., Singleton, J. A., & Kang, Y. (2018). Vaccination coverage among children aged 19–35 months — United States, 2017. Morbidity and Mortality Weekly Report, 67(40), 1123–1128. https://doi.org/10.15585/mmwr.mm6740a4
Jolley, D., & Douglas, K. M. (2014). The effects of anti-vaccine conspiracy theories on vaccination intentions. PLOS ONE, 9(2), e89177. https://doi.org/10.1371/journal.pone.0089177
Kreps, S., Dasgupta, N., Brownstein, J. S., Hswen, Y., & Kriner, D. L. (2021). Public attitudes toward COVID-19 vaccination: The role of vaccine attributes, incentives, and misinformation. NPJ Vaccines, 6(1), 73. https://doi.org/10.1038/s41541-021-00335-2
LaCour, M., & Davis, T. (2020). Vaccine skepticism reflects basic cognitive differences in mortality-related event frequency estimation. Vaccine, 38(21), 3790–3799. https://doi.org/10.1016/j.vaccine.2020.02.052
LaCour, M., Hughes, B., Goldwater, M., Ireland, M., Worthy, D., Van Allen, J., Gaylord, N., Van-Hoosier, G., & Davis, T. (2022). The double bind of communicating about zoonotic origins: Describing exotic animal sources of COVID‐19 increases both healthy and discriminatory avoidance intentions. Risk Analysis, 42(3), 506–521. https://doi.org/10.1111/risa.13764
Lazarus, J. V., Ratzan, S. C., Palayew, A., Gostin, L. O., Larson, H. J., Rabin, K., Kimball, S., & El-Mohandes, A. (2021). A global survey of potential acceptance of a COVID-19 vaccine. Nature Medicine, 27(2), 225–228. https://doi.org/10.1038/s41591-020-1124-9
Lazarus, J. V., Wyka, K., White, T. M., Picchio, C. A., Rabin, K., Ratzan, S. C., Parsons Leigh, J., Hu, J., & El-Mohandes, A. (2022). Revisiting COVID-19 vaccine hesitancy around the world using data from 23 countries in 2021. Nature Communications, 13(1), 3801–3815. https://doi.org/10.1038/s41467-022-31441-x
Leask, J., Kinnersley, P., Jackson, C., Cheater, F., Bedford, H., & Rowles, G. (2012). Communicating with parents about vaccination: A framework for health professionals. BMC Pediatrics, 12(1). https://doi.org/10.1186/1471-2431-12-154
Lubrano, A. (2019, April 10). Anti-vaccine parents are often white, college-educated, ‘Whole Foods moms.’ The Philadelphia Inquirer. https://www.inquirer.com/news/middle-class-working-class-vaccine-anti-vaxxers-measles-cdc-20190410.html
Monais, L. (2019, December 4). We must talk more about measles — and less about anti-vaxxers. The Washington Post. https://www.washingtonpost.com/outlook/2019/12/04/we-must-talk-more-about-measles-less-about-anti-vaxxers/
Monroe, T., & Savillo, R. (2021, July 16). Fox has undermined vaccination efforts in nearly 60% of all vaccination segments in a 2-week period. Media Matters. https://www.mediamatters.org/fox-news/fox-has-undermined-vaccination-efforts-nearly-60-all-vaccination-segments-2-week-period
Newhagen, J. E., & Bucy, E. P. (2020). Overcoming resistance to COVID-19 vaccine adoption: How affective dispositions shape views of science and medicine. Harvard Kennedy School (HKS) Misinformation Review, 1(6). https://doi.org/10.37016/mr-2020-44
Oprysko, C. (2020, November 1). Trump adviser doubles down on baseless Trump claim doctors are profiting off coronavirus deaths. Politico. https://www.politico.com/news/2020/11/01/doctors-profit-coronavirus-deaths-trump-miller-433793
Parad, J. E. (2012). A psychological critique of the public health response to chicken pox parties. Society, 49(6), 495–499. https://doi.org/10.1007/s12115-012-9591-3
Pew Research Center (2023, July 7). Demographic profiles of Republican and Democratic voters. https://www.pewresearch.org/politics/2023/07/12/demographic-profiles-of-republican-and-democratic-voters/
R Core Team. (2016). R: A language and environment for statistical computing. R Foundation for Statistical Computing. http://www.R-project.org/
Reyna, V. F. (2021). A scientific theory of gist communication and misinformation resistance, with implications for health, education, and policy. Proceedings of the National Academy of Sciences, 118(15), e1912441117. https://doi.org/10.1073/pnas.1912441117
Saad, L. (2024, February 7). U.S. women have become more liberal; men mostly stable. Gallup. https://news.gallup.com/poll/609914/women-become-liberal-men-mostly-stable.aspx#:~:text=Trends%20in%20Liberal%20Identification%20Among%20U.S.%20Men%2C%20by%20Age&text=Fewer%20men%20in%20each%20age,.%2025%25%20of%20men).
Siani, A., Carter, I., & Moulton, F. (2022). Political views and science literacy as indicators of vaccine confidence and COVID-19 concern. Journal of Preventive Medicine and Hygiene, 63(2), E257. https://doi.org/10.15167%2F2421-4248%2Fjpmh2022.63.2.2320
Siani, A., & Tranter, A. (2022). Is vaccine confidence an unexpected victim of the COVID-19 pandemic? Vaccine, 40(50), 7262–7269. https://doi.org/10.1016/j.vaccine.2022.10.061
Soveri, A., Karlsson, L. C., Antfolk, J., Mäki, O., Karlsson, L., Karlsson, H., Nolvi, S., Karukivi, M., Lindfelt, M., & Lewandowsky, S. (2023). Spillover effects of the COVID-19 pandemic on attitudes to influenza and childhood vaccines. BMC Public Health, 23(1). https://doi.org/10.1186/s12889-023-15653-4
Stagnaro, M., Pink, S., Rand, D. G., & Willer, R. (2023). Increasing accuracy motivations using moral reframing does not reduce Republicans’ belief in false news. Harvard Kennedy School (HKS) Misinformation Review, 4(6). https://doi.org/10.37016/mr-2020-128
Swire, B., Ecker, U. K. H., & Lewandowsky, S. (2017). The role of familiarity in correcting inaccurate information. Journal of Experimental Psychology: Learning, Memory, and Cognition, 43(12), 1948–1961. https://doi.org/10.1037/xlm0000422
Tapp, W. N., Miller, M. F., Gaylord, N., Goldwater, M. B., Ireland, M. E., Van Allen, J., & Davis, T. (2018). The impact of beliefs about cross-species disease transmission on perceived safety of wild game meat: Building a psychological approach to meat safety. Meat and Muscle Biology, 1(2). https://doi.org/10.22175/rmc2016.004
Tomeny, T. S., Vargo, C. J., & El-Toukhy, S. (2017). Geographic and demographic correlates of autism-related anti-vaccine beliefs on Twitter, 2009–15. Social Science & Medicine, 191, 168–175. https://doi.org/10.1016/j.socscimed.2017.08.041
UNICEF. (2023, April 20). New data indicates declining confidence in childhood vaccine of up to 44 percentage points in some countries during the COVID-19 pandemic [Press release]. https://www.unicef.org/rosa/press-releases/new-data-indicates-declining-confidence-childhood-vaccines-44-percentage-points-some
Wagenmakers, E. J. (2007). A practical solution to the pervasive problems of p values. Psychonomic Bulletin & Review, 14(5), 779–804. https://doi.org/10.3758/BF03194105
Wang, Q., Hu, S., Du, F., Zang, S., Xing, Y., Qu, Z., Zhang, X., Lin, L., & Hou, Z. (2022). Mapping global acceptance and uptake of COVID-19 vaccination: A systematic review and meta-analysis. Communications Medicine, 2(1), 113. https://doi.org/10.1038/s43856-022-00177-6
Wood, D., & Brumfiel, G. (2021, December 5). Pro-Trump counties now have far higher COVID death rates. Misinformation is to blame. NPR. https://www.npr.org/sections/health-shots/2021/12/05/1059828993/data-vaccine-misinformation-trump-counties-covid-death-rate
World Health Organization. (2019). Vaccines and immunization. https://www.who.int/health-topics/vaccines-and-immunization#tab=tab_1
Funding
No funding has been received to conduct this research.
Competing Interests
The authors declare no competing interests.
Ethics
The research protocol employed was approved by the institutional review boards of both universities where the studies were conducted. All participants provided informed consent. Ethnicity, gender, and other demographic categories were defined by the researchers and were collected to assess the generalizability of the results across various demographic groups.
Copyright
This is an open access article distributed under the terms of the Creative Commons Attribution License, which permits unrestricted use, distribution, and reproduction in any medium, provided that the original author and source are properly credited.
Data Availability
All materials needed to replicate this study are available via the Harvard Dataverse: https://doi.org/10.7910/DVN/JVBA7F